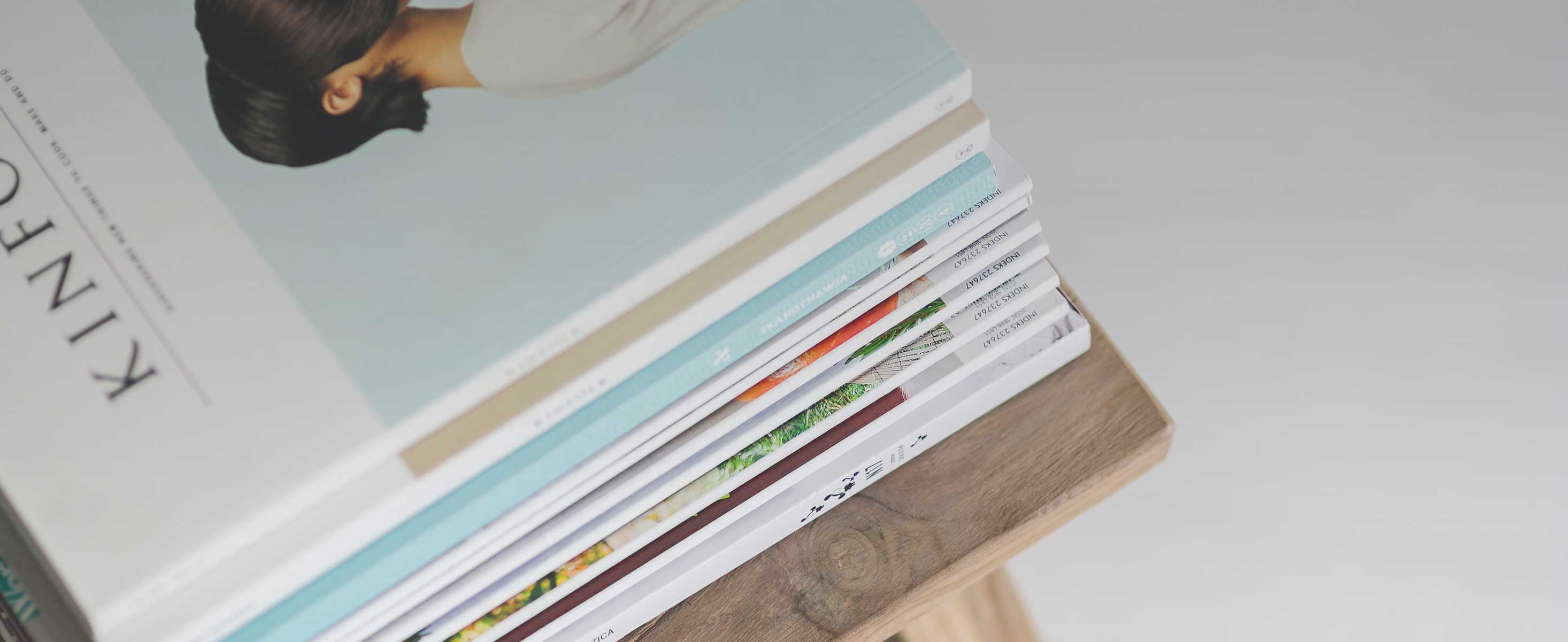
2 minute read
AI Research Roundup
The Division of Artificial Intelligence in Medicine at Cedars-Sinai is using AI to help close gaps in understanding and treatment of major human disease conditions.
Chugh Laboratory
Advertisement
The Chugh Laboratory at the Smidt Heart Institute focuses on arrhythmia research. The team investigates mechanisms of ventricular arrhythmias with a view to improve prediction, prevention and management of sudden cardiac arrest.
Dey Laboratory
The Dey Laboratory, affiliated with the Biomedical Imaging Research Institute and the Department of Biomedical Sciences, focuses on automated derivation of imaging measures from noninvasive cardiac image data and clinical implementation of novel automated computerprocessing algorithms.
ONLY 12% OF HEALTHCARE ORGANIZATIONS operate mature artificial intelligence (AI) programs. The AI gold standard integrates algorithms into a health system’s framework that are consistently vetted for bias and methodically monitored for compliance. The lack of trustworthy AI tools that translate between institutions can breed imbalanced, incomplete and skewed data.
Clean, AI-derived data is hard to come by. Less than half of 1% of studies that mine information from electronic health records (EHRs) harvest anything other than structured data fields, such as dates or diagnostic codes. This narrow approach excludes valuable context found in unstructured clinical notes and risks study results that don’t reflect actual population health.
“When data is not analyzed correctly, and the wrong conclusions are reached and the wrong actions are taken, that defeats the purpose of research and renders it harmful,” says
Natural-language processing can make all the difference in establishing a real understanding of the actual landscape of disease. Dr. Gonzalez-Hernandez points to a 2016 paper published in Diabetes Research and Clinical Practice comparing big-data strategies in the study of how often Type 2 diabetes patients experienced hypoglycemia. Researchers at Optum Epidemiology and Merck & Co. found that AI that utilized natural-language processing methods to read EHRs, combined with standard approaches, revealed a much higher prevalence of hypoglycemia than standard AI approaches alone.
A 2009 federal mandate requires physicians to use EHRs to report clinical data and quality measures. The rule was intended to standardize the capture of such information, facilitate its exchange, and improve research and care. But the effort has fallen far short, in part, because large-scale population health studies ignore unstructured EHR data, Dr. Gonzalez-Hernandez says.
“We’ve just begun to tap into this promise of using many records together to take advantage of cumulative knowledge to uncover patterns and come up with better ways to treat people,” she says. “After all these years, we’re still barely using EHRs, even though the data is all there.”
Ouyang Laboratory
The Ouyang Laboratory in the Smidt Heart Institute focuses on cardiology and cardiovascular imaging, working on applications of deep learning, computer vision and the statistical analysis of large data sets. The team applies deep learning for precision phenotyping in cardiac ultrasound and researches the deployment of AI models.
Slomka Laboratory
The Slomka Laboratory focuses on developing innovative methods for fully automated analysis of cardiac imaging data using novel algorithms and machine-learning techniques and on developing integrated motion-corrected analysis of PET/CT and CT angiography imaging.
Zhang Laboratory
The Zhang Laboratory develops automated deep-learning methods to accommodate rapid biotechnology development. These models help elucidate causal effects of genetic variations in epigenetics, transcription, post-transcriptional regulation, genome editing and various diseases.