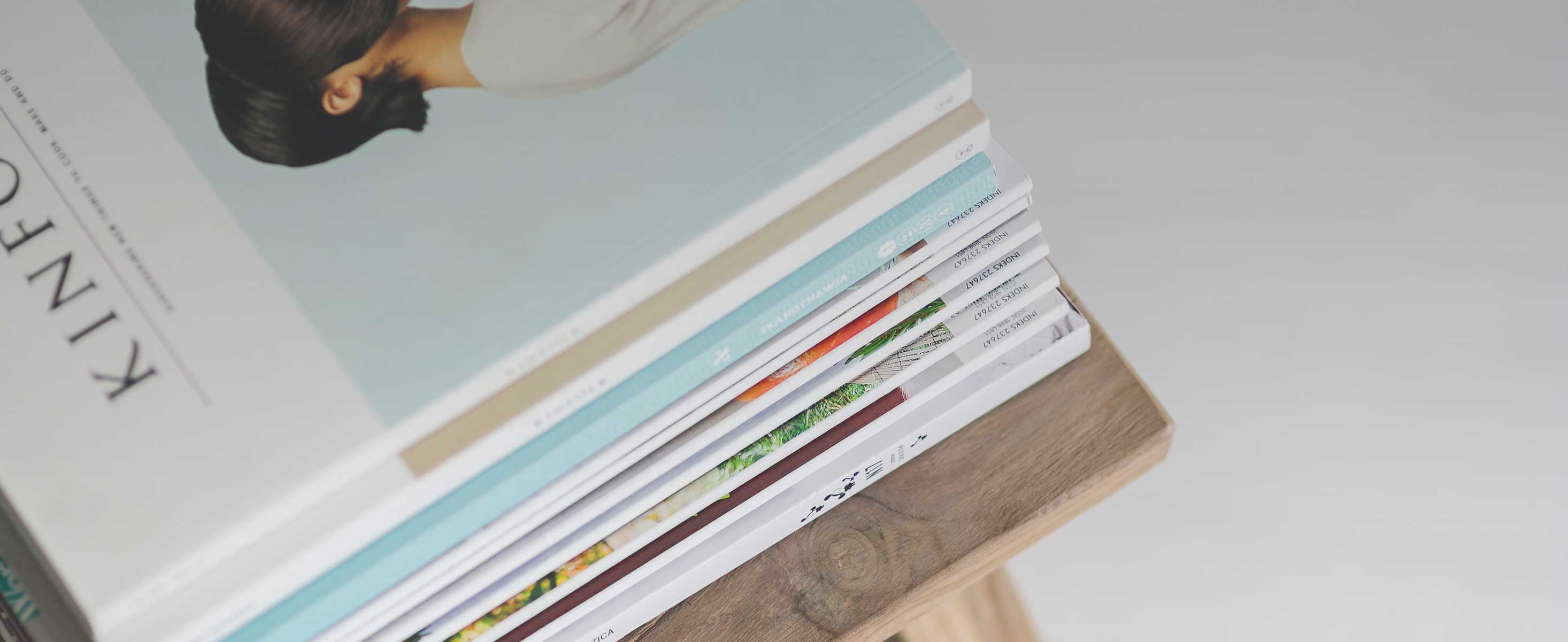
4 minute read
Implementation
mated unit costs for one device is €50 to €60. Currently, the project has been delayed due to COVID-19 and trade restrictions between the U.S. and parts of the Middle and Far East.
The project supports the following Sustainable Development Goals (SDGs):
Advertisement
1. SDG 3 “Good Health and Well-being”: By improving public health through safer and cleaner environmental conditions. 2. SDG 6 “Water and Sanitation”: By improving water quality and reducing pollution. 3. SDG 9 “Industry, Innovation and Infrastructure”: By developing high-quality, reliable and resilient infrastructures through enhanced technology and access to information. 4. SDG 11 “Sustainable Cities and Communities”: By ensuring access to sustainable basic services.
Technical framework
First, a requirements analysis was created and the AI was then developed accordingly. The AI uses a self-developed convolutional neural network (CNN) and computer vision on a microscopic camera as an optical solution for detecting dangerous particles and bacteria in water. It works in almost the same way as security camera (CCTV) footage.
The development and setup can be summarized in following steps:
1. Water flows underneath the camera. Particles and bacteria are scanned by the lens and recorded for real-time analysis by the
CNN. 2. In the initial developing steps, the project prototype used a laptop computer with a neural computing stick connected to a microscopic camera. 3. In the next iteration, the solution was developed as an IoT device. In its current state, Clean Water AI is as self-containing device (the size of a shoebox) that can be easily installed in areas where monitoring is required.
Supervised learning, a subfield of machine learning, was used for image classification. Two existing open-source models, CaffeNet and Mobilnet, were used in the development of the system and software libraries. Tensorflow and caffe were used to train the model. The company mobilized the expertise required to develop the AI application locally and a Titan RTX was used to ensure sufficient computing power. The deep learning neural network enables users of Clean Water AI to analyse water contamination in near real time based on the shape of bacteria at microscopic level. If new bacteria types need to be detected, the CNN can be trained and automatically updated.
Risk management
Clean Water AI covers a niche area between cities with none to very little developed water monitoring in place and smart cities that run a (modern) water monitoring system. It can detect leaks in sewage systems (enabling operators to quickly shut-off of a branch in the system, for example), it cannot purify the water itself. The developer of Clean Water AI considers most of China to be an appropriate market for the application. China has both the political will to introduce new technologies and the budget to buy the devices. At the same time, Chinese infrastructure is often too large (in some regions) to introduce chlorine purification, so the Clean Water AI application would close the gap until a nationwide introduction of chlorine purification is possible, i.e. the monitoring can be used as an early warning system to indicate water contamination and protect water consumers of an area or within a building.
Governance & project management
The system is integrated in the existing client’s technical infrastructure and used constantly to monitor the water quality within the network. In terms of maintenance, the system health of the IoT camera devices can be monitored automatically. The Clean Water AI software can be maintained by the company on a contractual basis.
Subsequent use
Transferability
The application example can be transferred to other contexts such as developing and emerging countries. The most important aspects here include a city’s ability to invest in the technology and the presence of a functioning or possibility to install a water purification system that will work more seamlessly with Clean Water AI in future. The AI solution is not a viable option for cities and regions that do not have any purification system in place as the software only monitors water quality, it cannot improve it.
Data management
This project uses data gathered by monitoring water systems (for example, videos and images of water) from utilities such as water management and treatment companies, or from city governments. As long as only water data and no personal data is required, there are no legal or privacy (GDPR) issues. In addition to this, there is no need for an agreement or framework on data sharing provided the data is not shared with third parties by Clear Water AI and is handled and managed by the client. The client, for example a city’s municipal administration department, may decide to make aggregated data publicly available, or just use them internally for maintenance purposes only.
Cost benefit & business model
The project’s ability to automatically monitor water quality in near real time is a key factor of its success. As part of its business