Intelligent Data Mining and Analysis in Power and Energy Systems : Models and Applications for Smarter Efficient Power Systems 1st Edition Zita A. Vale
Visit to download the full and correct content document: https://ebookmass.com/product/intelligent-data-mining-and-analysis-in-power-and-en ergy-systems-models-and-applications-for-smarter-efficient-power-systems-1st-editio n-zita-a-vale/
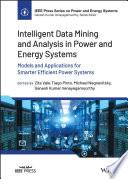
More products digital (pdf, epub, mobi) instant download maybe you interests ...
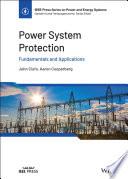
Power System Protection: Fundamentals and Applications (IEEE Press Series on Power and Energy Systems) 1st Edition Ciufo
https://ebookmass.com/product/power-system-protectionfundamentals-and-applications-ieee-press-series-on-power-andenergy-systems-1st-edition-ciufo/
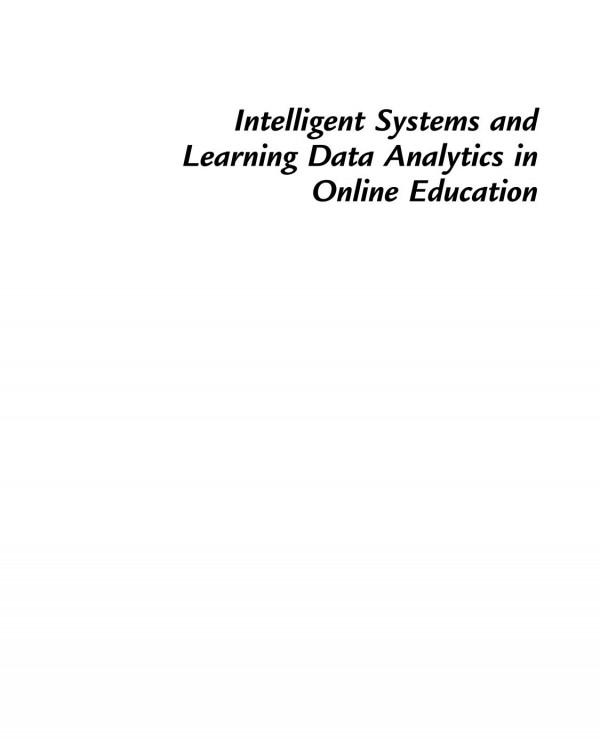
Intelligent Systems and Learning Data Analytics in Online Education: A volume in Intelligent Data-Centric Systems Santi Caballé
https://ebookmass.com/product/intelligent-systems-and-learningdata-analytics-in-online-education-a-volume-in-intelligent-datacentric-systems-santi-caballe/
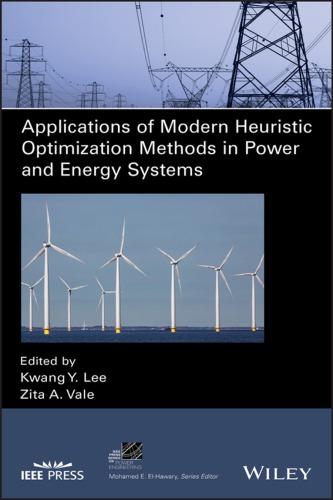
Applications of modern heuristic optimization methods in power and energy systems Lee Kwang Y.
https://ebookmass.com/product/applications-of-modern-heuristicoptimization-methods-in-power-and-energy-systems-lee-kwang-y/
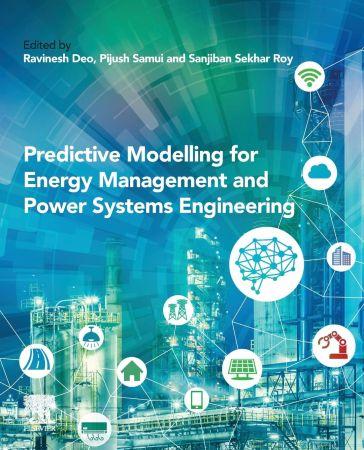
Predictive Modelling for Energy Management and Power Systems Engineering Ravinesh Deo
https://ebookmass.com/product/predictive-modelling-for-energymanagement-and-power-systems-engineering-ravinesh-deo/
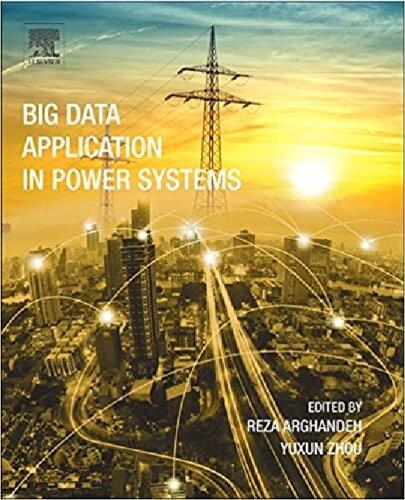
https://ebookmass.com/product/big-data-application-in-powersystems-reza-arghandeh/

Electrochemical Power Sources: Fundamentals, Systems, and Applications Tom Smolinka
https://ebookmass.com/product/electrochemical-power-sourcesfundamentals-systems-and-applications-tom-smolinka/
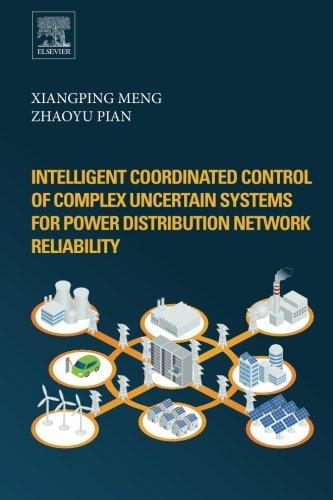
Intelligent Coordinated Control of Complex Uncertain Systems for Power Distribution and Network Reliability
1st Edition Meng Xiangping
https://ebookmass.com/product/intelligent-coordinated-control-ofcomplex-uncertain-systems-for-power-distribution-and-networkreliability-1st-edition-meng-xiangping/
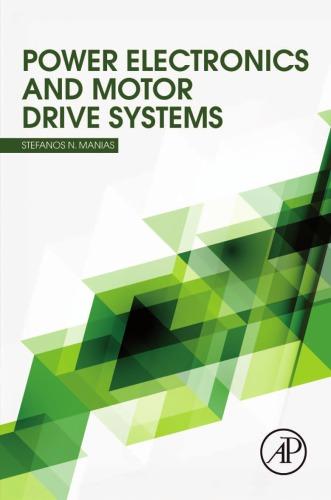
Power Electronics, Motor Drive Systems: Fundamentals, Experiments and Applications Manias
https://ebookmass.com/product/power-electronics-motor-drivesystems-fundamentals-experiments-and-applications-manias/
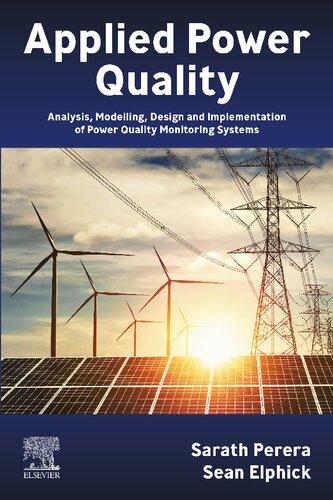
Applied Power Quality: Analysis, Modelling, Design and Implementation of Power Quality Monitoring Systems
Sarath Perera
https://ebookmass.com/product/applied-power-quality-analysismodelling-design-and-implementation-of-power-quality-monitoringsystems-sarath-perera/
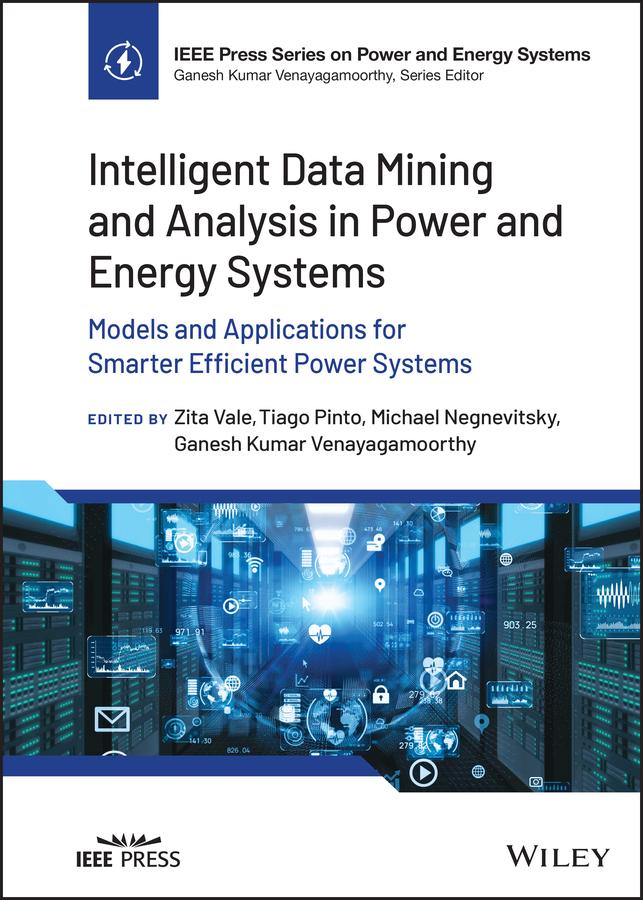
IEEEPress
445HoesLane Piscataway,NJ08854
IEEEPressEditorialBoard
SarahSpurgeon, EditorinChief
JónAtliBenediktssonAndreasMolischDiomidisSpinellis
AnjanBoseSaeidNahavandiAhmetMuratTekalp
AdamDrobot
Peter(Yong)Lian
JeffreyReed ThomasRobertazzi
IntelligentDataMiningandAnalysisinPowerand EnergySystems
ModelsandApplicationsforSmarterEfficientPowerSystems
Editedby ZitaVale
GECADResearchGrouponIntelligentEngineeringandComputingforAdvancedInnovation andDevelopment(GECAD)PolytechnicInstituteofPorto(ISEP/IPP)Porto,Portugal
TiagoPinto
GECADResearchGrouponIntelligentEngineeringandComputingforAdvancedInnovation andDevelopment(GECAD)PolytechnicInstituteofPorto(ISEP/IPP)Porto,Portugaland UniversityofTrás-os-MonteseAltoDouroVilaReal,Portugal
MichaelNegnevitsky
SchoolofEngineering,UniversityofTasmaniaHobart,Tasmania,Australia
GaneshKumarVenayagamoorthy
HolcombeDepartmentofElectricalandComputerEngineering,Real-TimePowerand IntelligentSystemsLaboratoryClemsonUniversityClemson,SC,USAandSchoolof EngineeringUniversityofKwaZulu-NatalDurban,SouthAfrica
Copyright©2023byTheInstituteofElectricalandElectronicsEngineers,Inc.
PublishedbyJohnWiley&Sons,Inc.,Hoboken,NewJersey.Allrightsreserved. PublishedsimultaneouslyinCanada.
Nopartofthispublicationmaybereproduced,storedinaretrievalsystem,ortransmittedinanyformorbyany means,electronic,mechanical,photocopying,recording,scanning,orotherwise,exceptaspermittedunderSection 107or108ofthe1976UnitedStatesCopyrightAct,withouteitherthepriorwrittenpermissionofthePublisher,or authorizationthroughpaymentoftheappropriateper-copyfeetotheCopyrightClearanceCenter,Inc.,222 RosewoodDrive,Danvers,MA01923,(978)750-8400,fax(978)750-4470,oronthewebatwww.copyright.com. RequeststothePublisherforpermissionshouldbeaddressedtothePermissionsDepartment,JohnWiley&Sons, Inc.,111RiverStreet,Hoboken,NJ07030,(201)748-6011,fax(201)748-6008,oronlineathttp://www.wiley.com/ go/permission.
Trademarks:WileyandtheWileylogoaretrademarksorregisteredtrademarksofJohnWiley&Sons,Inc.and/or itsaffiliatesintheUnitedStatesandothercountriesandmaynotbeusedwithoutwrittenpermission.Allother trademarksarethepropertyoftheirrespectiveowners.JohnWiley&Sons,Inc.isnotassociatedwithanyproduct orvendormentionedinthisbook.
LimitofLiability/DisclaimerofWarranty:Whilethepublisherandauthorhaveusedtheirbesteffortsinpreparing thisbook,theymakenorepresentationsorwarrantieswithrespecttotheaccuracyorcompletenessofthecontents ofthisbookandspecificallydisclaimanyimpliedwarrantiesofmerchantabilityorfitnessforaparticularpurpose. Nowarrantymaybecreatedorextendedbysalesrepresentativesorwrittensalesmaterials.Theadviceand strategiescontainedhereinmaynotbesuitableforyoursituation.Youshouldconsultwithaprofessionalwhere appropriate.Neitherthepublishernorauthorshallbeliableforanylossofprofitoranyothercommercialdamages, includingbutnotlimitedtospecial,incidental,consequential,orotherdamages.Further,readersshouldbeaware thatwebsiteslistedinthisworkmayhavechangedordisappearedbetweenwhenthisworkwaswrittenandwhenit isread.Neitherthepublishernorauthorsshallbeliableforanylossofprofitoranyothercommercialdamages, includingbutnotlimitedtospecial,incidental,consequential,orotherdamages.
Forgeneralinformationonourotherproductsandservicesorfortechnicalsupport,pleasecontactourCustomer CareDepartmentwithintheUnitedStatesat(800)762-2974,outsidetheUnitedStatesat(317)572-3993orfax(317) 572-4002.
Wileyalsopublishesitsbooksinavarietyofelectronicformats.Somecontentthatappearsinprintmaynotbe availableinelectronicformats.FormoreinformationaboutWileyproducts,visitourwebsiteatwww.wiley.com.
LibraryofCongressCataloging-in-PublicationData
Names:Vale,Zita,editor.|Pinto,Tiago,PhD,editor.|Negnevitsky, Michael,editor.|Venayagamoorthy,GaneshKumar,editor.
Title:Intelligentdataminingandanalysisinpowerandenergysystems: modelsandapplicationsforsmarterefficientpowersystems/editedby ZitaVale,TiagoPinto,MichaelNegnevitsky,GaneshKumar Venayagamoorthy.
Description:Hoboken,NewJersey:Wiley-IEEEPress,[2023]|Series:IEEE pressseriesonpowerandenergysystems
Identifiers:LCCN2022043311(print)|LCCN2022043312(ebook)|ISBN 9781119834021(cloth)|ISBN9781119834038(adobepdf)|ISBN 9781119834045(epub)
Subjects:LCSH:Electricpowersystems.|Datamining.
Classification:LCCTK1001.I5772023(print)|LCCTK1001(ebook)|DDC 621.31–dc23/eng/20220909
LCrecordavailableathttps://lccn.loc.gov/2022043311
LCebookrecordavailableathttps://lccn.loc.gov/2022043312
CoverDesign:Wiley
CoverImage:©metamorworks/Shutterstock
Setin9.5/12.5ptSTIXTwoTextbyStraive,Chennai,India
“ToourdearParentsandChildren”
Contents
AbouttheEditors xix
ListofContributors xxi
Foreword xxvii
Introduction 1 References 3
PartIDataMiningandAnalysisFundamentals 5
1Foundations 7
AnselY.Rodríguez-González,AngelDíaz-Pacheco,RamónAranda,andMiguelÁ. Álvarez-Carmona
Acronyms 7
1.1DataMining:WhyandWhat? 7
1.2DataMiningintoKDD 8
1.3TheDataMiningProcess 9
1.3.1DataCleaning 10
1.3.2DataIntegration 10
1.3.3DataReduction 11
1.3.4DataTransformation 12
1.4DataMiningTaskandTechniques 12
1.4.1Techniques 14
1.4.1.1Techniquesinthe“Description”Branch 14
1.4.1.2RegressionTechniques 14
1.4.1.3ClassificationTechniques 15
1.4.2Applications 17
1.5DataMiningIssuesandConsiderations 18
1.5.1ScalabilityofAlgorithms 18
1.5.2HighDimensionality 18
1.5.3ImprovingInterpretability 18
1.5.4HandlingUncertainty 19
1.5.5PrivacyandSecurityConcerns 19
1.6Summary 19 References 20
2DataMiningandAnalysisinPowerandEnergySystems:AnIntroductionto AlgorithmsandApplications 25 FernandoLezama Acronyms 25
2.1Introduction 25
2.2DataMiningTechnologies 26
2.2.1SupervisedMethods 26
2.2.1.1Regression-BasedMethods 27
2.2.1.2Classification-BasedMethods 27
2.2.2UnsupervisedMethods 27
2.2.2.1AssociationRuleMining 28
2.2.2.2Clustering-BasedMethods 28
2.3DataMiningApplicationsinPowerSystems 28
2.3.1Profiling 29
2.3.2Forecasting 31
2.3.3FaultDetectionandDiagnosis 33
2.3.4OtherApplications 34
2.4DiscussionandFinalRemarks 35 References 37
3DeepLearninginIntelligentPowerandEnergySystems 45 BrunoMota,TiagoPinto,ZitaVale,andCarlosRamos Acronyms 45
3.1Introduction 46
3.2DeepLearning 49
3.2.1RegressionProblems 49
3.2.1.1PhotovoltaicEnergyForecast 49
3.2.1.2WindPowerForecast 50
3.2.1.3BuildingEnergyConsumptionPrediction 50
3.2.1.4ElectricityPriceForecast 51
3.2.1.5OtherRegressionWorks 52
3.2.2ClassificationProblems 52
3.2.2.1PowerQualityDisturbancesDetection/Classification 53
3.2.2.2FaultDetection/Classification 54
3.2.2.3FeatureEngineering 55
3.2.2.4OtherClassificationWorks 55
3.2.3Decision-MakingProblems 56
3.2.3.1EnergyManagement 56
3.2.3.2DemandResponse 57
3.2.3.3ElectricityMarket 57
3.2.3.4OtherDecision-MakingWorks 58
3.3Accomplishments,Limitations,andChallenges 58
3.4Conclusions 60 References 60
PartIIClustering 69
4DataMiningTechniquesAppliedtoPowerSystems 71 SérgioRamos,JoãoSoares,ZahraForouzandeh,andZitaVale Acronyms 71
4.1Introduction 71
4.1.1DataSelection 72
4.1.2DataPre-processing 73
4.1.3DataMining 73
4.1.4AnalysisandInterpretation 74
4.2DataMiningTechniques 75
4.2.1ClusteringAlgorithms 76
4.2.2ClusteringValidityIndices 79
4.2.3ClassificationAlgorithms 80
4.3DataMiningTechniquesAppliedtoPowerSystems 82
4.3.1ElectricalConsumersCharacterization 83
4.3.1.1TypicalLoadProfile 83
4.3.2ElectricalConsumersCharacterization–Classification 86
4.3.3Conclusions 89
4.4ElectricalTariffsDesignBasedonDataMiningTechniques 90
4.4.1ElectricalTariffsDesign 90
4.4.2Conclusions 93
4.5DataMiningContributionstoCharacterizeZonalPrices 93
4.5.1ZonalPricesCharacterization 93
4.5.2Conclusions 97
4.6DataMining-BasedMethodologyforWindForecasting 98
4.6.1WindForecasting 98
4.6.2Conclusions 100
4.7FinalRemarks 101 References 101
5SynchrophasorDataAnalyticsforAnomalyandEventDetection, Classification,andLocalization 105 SajanK.Sadanandan,ArmanAhmed,ShikharPandey,andAnuragK.Srivastava
5.1Introduction 105
5.2SynchrophasorDataQualityIssuesandChallenges 106
5.2.1PMUDataFlow:DataQualityIssues 107
5.2.2PMUDataAnomalies 108
5.3ML-BasedAnomalyDetection,Classification,andLocalization(ADCL)OverData DriftingMultivariateSynchrophasorDataStreams 108
5.3.1DataDriftinSynchrophasorMeasurements 109
5.3.2PMUNETFramework 110
5.3.2.1DataPre-Processing(DPP)Module 110
5.3.2.2Data-Drift(DD)Module 110
5.3.2.3Save-Load(SL)Module 112
5.3.3AnomalyDetector(AD)Module 112
5.3.3.1AnomalyClassification 113
5.3.3.2AnomalyLocalization 113
5.3.4DistributedDeepAutoencoderLearning 113
5.4SynchrophasorDataAnomalyandEventDetection,Localization,and Classification(SyncAED) 114
5.4.1SynchrophasorDataAnomalyDetection(SyncAD) 114
5.4.1.1BaseDetectors 115
5.4.1.2EnsembleMethod 115
5.4.1.3Prony-BasedTransientWindowEstimation 115
5.4.2EventDetection,Classification,andLocalization 116
5.4.2.1EventDetection 117
5.4.2.2EventClassification 117
5.4.2.3EventLocalization 117
5.5Test-BedandTestCases 119
5.5.1Cyber-PowerTest-BedArchitecture 119
5.5.1.1TestCase 119
5.6ResultsandDiscussion 120
5.6.1SimulationResultsforPMUNET 120
5.6.1.1PerformanceEvaluationMetrics 120
5.6.1.2ExperimentalAnalysis 121
5.6.2SimulationResultsforSyncAED 122
5.6.2.1AnomalyDetection 122
5.6.2.2EventDetectionandClassificationUsingClusteringandDecisionTree 122
5.7Summary 125 Acknowledgments 125 References 125
6ClusteringMethodsfortheProfilingofElectricityConsumersOwningEnergy StorageSystem 129
CátiaSilva,PedroFaria,JuanM.Corchado,andZitaVale Acronyms 129
6.1Introduction 129
6.2MethodologyDefinition 131
6.3ClusteringofConsumerswithESS 135
6.3.1OptimalNumberofClusters 135
6.3.1.1AverageSilhouetteMethod 136
6.3.1.2ElbowMethod 136
6.3.1.3GapStatisticMethod 137
6.3.2ClusteringMethods 137
6.3.2.1PartitionalClustering 138
6.3.2.2FuzzyClustering 141
6.3.2.3HierarchicalClustering 142
6.4Conclusion 145
Acknowledgments 146 References 146
PartIIIClassification 149
7ANovelFrameworkforNTLDetectioninElectricDistributionSystems 151 Chia-ChiChu,NelsonFabianAvila,GerardoFigueroa,andWen-KaiLu Acronyms 151
7.1Introduction 151
7.1.1State-of-the-Art 152
7.1.2ProposedFramework 153
7.2DataAcquisitionandPre-Processing 154
7.2.1DataAcquisition 154
7.2.2Pre-Processing 155
7.3FeatureExtraction 156
7.3.1Overview 156
7.3.2MODWPT 156
7.3.3FeatureExtractionMechanism 156
7.4ClassificationStrategies 158
7.4.1RandomUnder-Sampling(RUS)andRandomOver-Sampling(ROS)Techniques 158
7.4.2AdaptiveBoostingAlgorithm 158
7.4.3RandomUnder-SamplingBoostingAlgorithm 159
7.5Evaluation 160
7.6Experiments 161
7.6.1OutlierDetectionUsingSmoothingSplines 161
7.6.2MODWPT-BasedSignalDecomposition 163
7.6.3RusBoostNTLDetectionTechnique 163
7.6.4ComparisonwithExistingApproaches 164
7.7Conclusion 166 References 167
8ElectricityMarketParticipationProfilesClassificationforDecisionSupportin MarketNegotiation 171 TiagoPintoandZitaVale Acronyms 171
8.1Introduction 171
8.2BilateralNegotiation 172
8.3DecisionSupportforBilateralNegotiations 174
8.3.1ClusteringofPlayersProfiles 176
8.3.2ClassificationofNewPlayers 177
8.3.2.1ArtificialNeuralNetworks 177
8.3.2.2SupportVectorMachines 177
8.4IllustrativeResults 178
8.5Conclusion 183 References 184
9Socio-demographic,Economic,andBehavioralAnalysisofElectric Vehicles 187 RúbenBarreto,TiagoPinto,andZitaVale Acronyms 187
9.1Introduction 187
9.2ElectricVehicleOutlook 188
9.2.1ElectricMobilityMarket 188
9.2.2EconomicAspects 189
9.2.3Socio-demographicAspects 190
9.2.4RecommendationsforPolicymakers 191
9.3DataMiningModelsforEVs 191
9.3.1ChargingBehavior 191
9.3.2EVUserBehavior 192
9.3.3DrivingRange 193
9.3.4Speed 194
9.3.5ElectricVehicleBattery 195
9.3.6ChargingStationPlanning 195
9.3.7Summary 196
9.4Conclusions 197 References 197
PartIVForecasting 201
10AMultivariateStochasticSpatiotemporalWindPowerScenario ForecastingModel 203 WenleiBai,DueheeLee,andKwangY.Lee Acronyms 203 Nomenclature 203
10.1Introduction 204
10.2GeneralizedDynamicFactorModel 206
10.2.1DerivationoftheGDFM 206
10.2.2EstimationoftheGDFM 208
10.2.3ForecastoftheGDFM 210
10.2.4VerificationoftheGDFM 212
10.2.5ApplicationoftheGDFM 216
10.3Conclusion 219 References 221
11SpatiotemporalSolarIrradianceandTemperatureDataPredictive Estimation 223
ChirathPathiravasamandGaneshKumarVenayagamoorthy Acronyms 223
11.1Introduction 223
11.2VirtualWeatherStations 225
11.3DistributedWeatherForecasting 227
11.3.1SpatiotemporalPredictionNetwork 227
11.3.2ComputationalUnits 228
11.4ResultsandDiscussion 228
11.4.1WeatherDataEstimation 229
11.4.2WeatherDataPrediction 230
11.5Summary 232 Acknowledgment 234 References 234
12ApplicationofDecomposition-BasedHybridWindPowerForecastingin IsolatedPowerSystemswithHighRenewableEnergyPenetration 237 EvgeniiSemshikov,MichaelNegnevitsky,JamesHamilton,andXiaolinWang
12.1Introduction 237
12.2DecompositionTechniques 238
12.2.1VariationalModeDecomposition 239
12.2.2DecompositionofWindPowerTimeSeries 239
12.3Decomposition-BasedNeuralNetworkForecasting 241
12.3.1TheoryBehindLSTM 242
12.3.2VMD-LSTMforWindPowerForecasting 242
12.4Forecast-BasedDispatchinIsolatedPowerSystems 243
12.4.1ControlStrategy 244
12.4.2RegulationandLoadFollowingReserves 246
12.5CaseStudies 249
12.5.1KingIslandIsolatedPowerSystem 249
12.5.2CaseStudyI(ControlStrategywithNoREForecast) 250
12.5.3CaseStudyII(ControlStrategyInvolvingPersistenceModelREForecast) 251
12.5.4CaseStudyIII(ControlStrategyInvolvingVMD-LSTM-BasedRE Forecast) 251
12.5.5EconomicAssessmentOveraYearofOperation 252
12.6ConclusionsandDiscussions 253 References 253
PartVDataAnalysis 257
13HarmonicDynamicResponseStudyofOverheadTransmissionLines 259 DharmbirPrasad,RudraP.Singh,IrfanKhan,andSushriMukherjee Acronyms 259 Nomenclature 259
13.1IntroductiontoMethodology 260
13.1.1General 261
13.1.2SelectionAspectsofDampers 261
13.1.3LiteratureReview 262
13.2ProblemFormulation 264
13.2.1DesignAspect 265
13.2.2MathematicalModeling 265
13.3NumericalAnalysis 266
13.3.1SimulationInputs 267
13.3.1.1ModelDescription 267
13.3.1.2LoadExcitation 268
13.3.1.3SpanWisePhaseLag 268
13.3.2AnalysisFindings 271
13.4Conclusion 273
13.AAppendix 274
References 277
14EvaluationofShortestPathtoOptimizeDistributionNetworkCostand PowerLossesinHillyAreas:ACaseStudy 281 SubhoUpadhyay,RajeevK.Chauhan,andMahendraP.Sharma Acronyms 281
14.1Introduction 282
14.2DesignofPowerDistributionNetwork 282
14.3DigitalElevationMap 283
14.4PlacementofGeneratorsandLoadCenters 283
14.5SingleLineDiagramof9-BusSystem 285
14.6FindingShortestPathBetweenLoad/GeneratingCenters 286
14.6.1ObjectiveFunction 287
14.6.2DistributionNetworkDistance 289
14.7SelectionofConductorUsingNewtonRaphsonMethod 290
14.7.1EstimationofConductorCost 292
14.8CalculationofCO2 EmissionCostSaving 293
14.9OverallCostEstimationofDistributionSystem 294
14.10SensitivityAnalysis 294
14.10.1ChangeinDieselFuelPrice 295
14.10.2ChangeinSolarRadiation 295
14.10.3ChangeinDemand 295
14.10.4ChangeinEnergyIndexRatio 295
14.11Conclusion 295 References 296
15IntelligentApproachestoSupportDemandResponseinMicrogrid Planning 299
RahmatKhezri,AminMahmoudi,andHirohisaAki Acronyms 299
15.1Introduction 299
15.2MicrogridPlanning 300
15.2.1ProblemOverview 301
15.2.2ObjectiveFunctions 302
15.2.3DataAnalysis 303
15.2.3.1WeatherData 304
15.2.3.2LoadData 304
15.2.3.3ElectricityPrice 304
15.2.4MicrogridComponents 305
15.2.4.1DistributedEnergyResources 305
15.2.4.2EnergyStorageSystems 305
15.2.5MicrogridOperation 306
15.3DemandResponseinMicrogrids 306
15.3.1OverviewonDemandResponseApplicationforMicrogrids 306
15.3.2DemandResponse:TypesandCharacteristics 307
15.3.3IncentiveDR 308
15.3.4Time-BasedDR 309
15.4IntelligentApproachestoSupportDemandResponse 309
15.4.1DataMiningMethodsinDR 310
15.4.1.1SupervisedDataMining 310
15.4.1.2UnsupervisedDataMining 312
15.4.2FuzzyLogic-BasedDR 313
15.4.3ApplicationsinMicrogridPlanning 313
15.4.3.1CostReduction 313
15.4.3.2ResiliencyEnhancement 314
15.4.3.3FlexibilityImprovement 314
15.4.3.4BatteryCapacityReduction 314
15.5Conclusion 315 References 315
16SocioeconomicAnalysisofRenewableEnergyInterventions:Developing AffordableSmall-scaleHouseholdSustainableTechnologiesin NorthernUganda 319
JensB.Holm-Nielsen,AchoraP.O.Mamur,andSamsonMasebinu Acronyms 319
16.1Introduction 319
16.2RenewableEnergyTechnologies 321
16.2.1Bio-oil 321
16.2.2Bio-pellets 322
16.2.3Biogas 322
16.2.4SolarCookers 322
16.2.5SolarPV 323
16.3Methodology 323
16.3.1DriverPressureImpactStateResponseFramework 323
16.3.2Cost–BenefitAnalysis 324
16.4ApplicationoftheMethod 324
16.5CaseStudyResultsforProductDevelopment 327
16.5.1FieldStudy 327
16.5.2SourceofEnergyforCooking 328
16.5.3SourceofEnergyforLighting 328
16.5.4HouseholdIncomeLevel 328
16.5.5ChallengesforFirewoodandCharcoalUse 329
16.5.6TheRankofAdoptionTowardSustainableRenewableEnergyTechnologies 329
16.5.7HouseholdOpinionsforModernEnergyTechnologies 330
16.5.8LevelofAwarenessofthePopulation 331
16.5.9MediumofInformation 331
16.5.10PromotiontoPurchaseAlternativeRenewableEnergyTechnologies 331
16.5.11SourcesofFundforInvestmentinNorthernUgandaTowardRenewableEnergy TechnologiesforHouseholds 332
16.6Cost–BenefitAnalysis(CBA) 333
16.6.1BenefitstoBetterHealth 333
16.6.2BenefitsonGreenhouseGasEmissionsReduction 334
16.6.3BenefitsoftheDistrictForestResourcesPreservation 334
16.6.4OutcomesofCost–BenefitAnalysis 334
16.7Conclusion 337 References 338
PartVIOtherMachineLearningApplications 343
17Non-IntrusiveLoadMonitoringUsingAParallelBidirectionalLong Short-TermMemoryModel 345 VictorAndreanandKuo-LungLian Nomenclature 345
17.1Introduction 346
17.1.1Optimization-BasedApproach 346
17.1.2Learning-BasedApproach 347
17.2NILMSystemandDataPreprocessing 349
17.2.1DataScaling 349
17.2.2WindowLengthSelection 350
17.2.3Input-to-OutputRelation(IOR) 350
17.2.3.1IOR1 351
17.2.3.2IOR2 351
17.2.3.3IOR3 351
17.2.3.4IOR4 351
17.3ProposedMethod 352
17.3.1FeatureExtractor 353
17.3.2ElementsofthePBLSTM 355
17.3.2.1ConvolutionNeuralNetwork(CNN) 355
17.3.2.2BidirectionalLongShort-TermMemory(BLSTM) 356
17.3.2.3DenseLayer 357
17.3.2.4DeepNeuralNetworkTraining 358
17.4Validation 358
17.5Conclusion 368 References 368
18ReinforcementLearningforIntelligentBuildingEnergyManagement SystemControl 371 OliveraKotevskaandPhilippAndelfinger ChapterObjectives 371
18.1Introduction 371
18.2ReinforcementLearning 372
18.2.1DeepReinforcementLearning 374
18.2.2AdvancedReinforcementLearning 375
18.3ApplicationsofDeepReinforcementLearninginBuildingEnergyManagement SystemsControl 376
18.3.1Heating,Ventilation,andAirConditioning 377
18.3.2WaterHeater 379
18.3.3OtherDevices 380
18.4ChallengesandResearchDirections 380
18.5Conclusions 383 References 383
19FederatedDeepLearningTechniqueforPowerandEnergySystems DataAnalysis 387
HamedMoayyed,ArashMoradzadeh,BehnamMohammadi-Ivatloo,and RezaGhorbani
Nomenclature 387
Acronyms 387
Symbols 387
19.1Introduction 388
19.2FederatedLearning(FL) 388
19.2.1FederatedLearningMotivation 389
19.2.2PerformanceEvaluationMetrics 390
19.2.3FederatedLearningvs.DistributedMachineLearningApproaches 391
19.2.4TheFederatedAveragingAlgorithm 391
19.2.5ApplicationsofFederatedLearning 393
19.2.6ChallengesofFederatedLearning 395
19.3PowerSystemsChallengesandthePerformanceofArtificialIntelligence TechniquesinIt 396
19.3.1AI-BasedForecastinginPowerSystems 396
19.3.2AI-BasedConditionMonitoringinPowerSystems 397
19.4ApplicationofFederatedDeepLearninginPowerandEnergySystems 399
19.4.1ElectricVehicleNetworks 399
19.4.2FalseDataInjectionAttacksinSolarFarms 399
19.4.3SolarIrradiationForecasting 400
19.4.4HeatingLoadDemandForecasting 400
19.5Conclusion 400 References 401
20DataMiningandMachineLearningforPowerSystemMonitoring, Understanding,andImpactEvaluation 405
XindaKe,HuiyingRen,QiuhuaHuang,PavelEtingov,andZhangshuanHou Acronyms 405
20.1Introduction 406
20.2PowerSystemMonitoringwithPhasorMeasurementUnitData 407
20.2.1PMUAnomalyDetectionFramework 407
20.2.2AnomalyDetectionandClassification 408
20.3PowerSystemMechanisticandPredictiveUnderstanding 411
20.3.1SpatiotemporalPatternRecognitioninPMUSignals 412
20.3.1.1TimeSeriesPatternRecognition 412
20.3.1.2SimilaritiesandVariationsAcrossUnits 415
20.3.1.3Similarities/DiscrepanciesBetweenDays/Months 417
20.3.2EventsClassificationandLocalizationThroughConvolutionalNeuralNetwork 417
20.3.2.1PolishSystemTestbedandDataPreparation 417
20.3.2.2FaultTypesandImplementation 419
20.3.2.3CNNModelDevelopment 419
20.3.2.4CNNModelEvaluation 420
20.3.2.5FaultLocalization 421
20.3.2.6FaultClassification 422
20.4CharacterizationandModellingofWeatherandPowerExtremes 423
20.4.1DataSources 424
20.4.2SpatiotemporalAnalysis 425
20.4.3ProbabilisticModellingofLinesOutage 428
20.5Conclusion 430 References 430
Conclusions 433 ZitaVale,TiagoPinto,MichaelNegnevitsky,andGaneshKumarVenayagamoorthy
Index 435
AbouttheEditors
ZitaVale,IEEESeniorMember,graduatedinelectricalengineeringin1986,receivedthePhD degreeinelectricalandcomputerengineeringin1993,andtheAgregaçãotitle(Habilitation)in 2003fromtheUniversityofPorto,Portugal.SheisafullprofessorintheSchoolofEngineering, PolytechnicofPorto.SheleadstheresearchactivitiesonIntelligentPowerandEnergySystems atGECAD–ResearchGrouponIntelligentEngineeringandComputingforAdvancedInnovationandDevelopment.Shehasbeeninvolvedinmorethan60R&Dprojectsandpublished morethan200papersininternationalscientificjournals.Herscientificresearchactivities mainlyfocusonPowerandEnergySystemsOperation,ElectricityMarkets,DemandResponse, Renewables,ElectricVehicles,andDistributedGenerationandStorage.Shehasbeendeveloping artificial-intelligence-basedmodels,methods,andapplicationsforpowerandenergy,usingagents andmultiagentsystems,knowledge-basedsystems,semantics,machinelearning,datamining, andevolutionarycomputation.
Sheactivelyparticipatesinseveraltechnicalworkinggroupsandcommittees.Sheisthechair oftheIEEEPESIntelligentDataAnalysisandMining(IDMA)WorkingGroupandoftheOpen DataSets(ODS)TaskForce.SheisthechairoftheboardofdirectorsofISAP–IntelligentSystems ApplicationtoPowerSystems.Sheisinvolvedineditingactivitiesfordifferentjournalsandbooks andisaregularreviewerandevaluatorforpapersandforprojectproposalsandmonitoringfrom differentfundingagenciesaroundtheworld.
TiagoPinto isanassistantprofessorattheUniversidadedeTrásosMonteseAltoDouro (UTAD),Portugal,andresearcheratINESC-TEC.HehasconcludedtheBScandMSc,bothat theSchoolofEngineeringofthePolytechnicofPorto,wherehehasalsodevelopedhisresearch workformorethan10years,namelyatGECAD–ResearchGrouponIntelligentEngineering andComputingforAdvancedInnovationandDevelopment.HegotthePhDfromUTADin2016, afterwhichheengagedinapostdocattheUniversityofSalamanca,incollaborationwiththe UniversityofOxfordandENGIE.Hismainresearchinterestsfocusonartificialintelligenceand itsapplicationinpowerandenergysystems,particularlymachinelearning,multi-agentsystems, decisionsupportsystems,andmetaheuristicoptimization.Throughtheinvolvementinmorethan 30researchprojectsinthesefields,hehasauthoredover200publicationsininternationaljournals andconferences,andhasco-editedseveralbooksandspecialissuesinjournalsrelatedtopower andenergysystemsandartificialintelligence.
MichaelNegnevitsky receivedtheBE(Hons)inElectricalEngineeringdegreeandPhDdegree fromByelorussianUniversityofTechnology,Minsk,Belarus,in1978and1983,respectively.From 1978to1980,heworkedattheElectricalMaintenance,ConstructionandCommissioningCompany,andfrom1984to1991,hewasSeniorResearchFellowattheDepartmentofElectricalEngineering,ByelorussianUniversityofTechnology,Minsk.AfterhisarrivaltoAustralia,heworked
atMonashUniversity,Melbourne,andthentheUniversityofTasmania.CurrentlyheisProfessor andChairinPowerEngineeringandComputationalIntelligence,andDirectoroftheCentrefor RenewableEnergyandPowerSystems.
Hisresearchinterestsincludepowersystemanalysisandcontrol,micro-gridswithdistributed andrenewableenergyresources,smartgrids,powerqualityandapplicationsofartificialintelligenceinpowersystems.Hehaspublishedmorethan450papersinhigh-qualityjournalsand refereedconferenceproceedings,authored14chaptersinseveralbooks,andreceived4patents forinventions.Hisbook ArtificialIntelligence (AddisonWesley2002,2005,2011)hasbeentranslatedintoMandarin,Cantonese,Korean,Greek,andVietnameseandadoptedinmanyuniversities aroundtheworld.
HeisFellowofEngineersAustralia,FellowoftheJapanSocietyforthePromotionofScience, MemberofCIGREAPC4(SystemTechnicalPerformance)andAPC6(DistributionSystemsand DispersedGeneration),AustralianTechnicalCommittee.Dr.NegnevitskyhasservedasSecretary andDeputyChairofIEEEPESEnergyDevelopmentandPowerGenerationCommittee,Chair ofIEEEPESInternationalPracticesSubcommittee,andChairoftheIEEEPESWorkingGroup onHighRenewableEnergyPenetrationinRemoteandIsolatedPowerSystems.In2018,he receivedtheJointAustralasianCommitteeforPowerEngineeringandCIGREAustraliaAward for“outstandingcareer-longcontributionstoresearchandteachinginelectricpowerengineering aswellascontributiontoindustryandCIGREactivities.”
GaneshKumarVenayagamoorthy istheDukeEnergyDistinguishedProfessorofPowerEngineeringandProfessorofElectricalandComputerEngineeringatClemsonUniversitysinceJanuary 2012.Priortothat,hewasaProfessorofElectricalandComputerEngineeringattheMissouri UniversityofScienceandTechnology(MissouriS&T),Rolla,USA,wherehewasfrom2002to2011. Dr.VenayagamoorthywasaSeniorLecturerinDepartmentofElectronicEngineering,DurbanUniversityofTechnology,Durban,SouthAfrica,wherehewasfrom1996to2002.Dr.Venayagamoorthy istheFounderandDirectoroftheReal-TimePowerandIntelligentSystemsLaboratoryatMissouri S&TandClemsonUniversity.
Dr.VenayagamoorthyreceivedhisPhDandMScEngdegreesinElectricalEngineeringfrom theUniversityofNatal,Durban,SouthAfrica,inApril2002andApril1999,respectively.He receivedhisBEngdegreewithaFirst-ClassHonorsinElectricalandElectronicsEngineeringfrom AbubakarTafawaBalewaUniversity,Bauchi,Nigeria,inMarch1994.HeholdsanMBAdegreein EntrepreneurshipandInnovationfromClemsonUniversity,SC(August2016).
Dr.Venayagamoorthy’sinterestsareinresearch,developmentandinnovationofpowersystems, smartgrid,andartificialintelligencetechnologies.Dr.VenayagamoorthyisaFellowoftheIEEE, IET(UK),theSouthAfricanInstituteofElectricalEngineers(SAIEE)andAsia-PacificArtificial IntelligenceAssociation(AAIA),andaSeniorMemberoftheInternationalNeuralNetwork Society.
ListofContributors
A.Ahmed SmartGridDemonstrationandResearch InvestigationLab WashingtonStateUniversity Pullman,WA USA
HirohisaAki FacultyofEngineering,Informationand Systems UniversityofTsukuba Tsukuba,Ibaraki Japan
PhilippAndelfinger InstituteforVisualandAnalyticComputing UniversityofRostock Rostock Germany
VictorAndrean DepartmentofElectricalEngineering NationalTaiwanUniversityofScienceand Technology Taipei Taiwan
RamónAranda TepicTechnologyTransferUnit,Centerfor ScientificResearchandHigherEducationof Ensenada Tepic,Nayarit,Mexico and
NationalCouncilofScienceandTechnology MexicoCity,MexicoCity Mexico
NelsonF.Avila IndependentElectricitySystemOperator Toronto,ON Canada WenleiBai OracleEnergyandWater Austin,TX USA
RúbenBarreto ResearchGrouponIntelligentEngineering andComputingforAdvancedInnovationand Development(GECAD)Instituteof EngineeringPolytechnicofPorto Porto Portugal
MiguelA.Carmona TepicTechnologyTransferUnit,Centerfor ScientificResearchandHigherEducationof Ensenada Tepic Nayarit Mexico and NationalCouncilofScienceandTechnology MexicoCity MexicoCity Mexico
RajeevK.Chauhan DepartmentofElectricalEngineering,Faculty ofEngineering DayalbaghEducationalInstitute Agra,UttarPradesh
India
Chia-ChiChu DepartmentofElectricalEngineering NationalTsingHuaUniversity HsinChu
Taiwan,R.O.C.
JuanM.Corchado BISITEresearchgroup UniversityofSalamanca Salamanca Spain
PavelEtingov PacificNorthwestNationalLaboratory Richland,WA
USA
PedroFaria
GECAD–ResearchGrouponIntelligent EngineeringandComputingforAdvanced InnovationandDevelopment Porto
Portugal
GerardoFigueroa SentianceNV Antwerpen
Belgium
ZahraForouzandeh
GECAD–ResearchGrouponIntelligent EngineeringandComputingforAdvanced InnovationandDevelopment,Polytechnicof Porto,SchoolofEngineering(ISEP) Porto
Portugal
RezaGhorbani RenewableEnergyDesignLaboratory (REDLab),DepartmentofMechanical Engineering UniversityofHawaiiatManoa Honolulu,HI USA
JamesHamilton SchoolofEngineering,UniversityofTasmania Hobart
Tasmania
Australia
JensB.Holm-Nielsen DepartmentofEnergyTechnology CenterforBioenergyandGreenEngineering AalborgUniversity
Esbjerg
Denmark
ZhangshuanHou PacificNorthwestNationalLaboratory Richland,WA USA
QiuhuaHuang PacificNorthwestNationalLaboratory Richland,WA USA
XindaKe PacificNorthwestNationalLaboratory Richland,WA USA
IrfanKhan Supreme&Co.Pvt.Ltd. Kolkata,WestBengal India
RahmatKhezri CollegeofScienceandEngineering FlindersUniversity
Adelaide,SA
Australia
OliveraKotevska ComputerScienceandMathematics OakRidgeNationalLaboratory Tennessee OakRidge USA
DueheeLee ElectricalEngineeringDepartment KonkukUniversity
Seoul Korea
KwangY.Lee ElectricalandComputerEngineering Department BaylorUniversity Waco,TX USA
FernandoLezama PolytechnicofPorto(ISEP/IPP),Research GrouponIntelligentEngineeringand ComputingforAdvancedInnovationand Development(GECAD)
Porto
Portugal
Kuo-LungLian DepartmentofElectricalEngineering NationalTaiwanUniversityofScienceand Technology
Taipei Taiwan
Wen-KaiLu DepartmentofInformationManagement NationalTaiwanUniversity
Taipei
Taiwan,R.O.C.
AminMahmoudi CollegeofScienceandEngineering,Flinders University
Adelaide,SA
Australia
AchoraP.O.Mamur FacultyofSociology,Environmentaland BusinessEconomics UniversityofSouthernDenmark Esbjerg
Denmark
SamsonMasebinu DepartmentofEnergyTechnology,Centerfor BioenergyandGreenEngineering AalborgUniversity Esbjerg
Denmark
HamedMoayyed GECAD–ResearchGrouponIntelligent EngineeringandComputingforAdvanced InnovationandDevelopment,Polytechnicof Porto(P.PORTO)
Porto
Portugal
BehnamMohammadi-Ivatloo FacultyofElectricalandComputer Engineering UniversityofTabriz
Tabriz
Iran
ArashMoradzadeh FacultyofElectricalandComputer Engineering UniversityofTabriz
Tabriz
Iran
BrunoMota GECADResearchGrouponIntelligent EngineeringandComputingforAdvanced InnovationandDevelopment(GECAD) PolytechnicInstituteofPorto(ISEP/IPP)
Porto
Portugal
SushriMukherjee IndianInstituteofTechnologyDelhi HauzKhas NewDelhi India
MichaelNegnevitsky
SchoolofEngineering,UniversityofTasmania Hobart Tasmania Australia
AngelD.Pacheco
TepicTechnologyTransferUnit,Centerfor ScientificResearchandHigherEducationof Ensenada Tepic Nayarit Mexico
S.Pandey SmartGridandTechnology ComEd OakbrookTerrace,IL USA
ChirathPathiravasam
HolcombeDepartmentofElectricaland ComputerEngineering,Real-TimePowerand IntelligentSystemsLaboratory ClemsonUniversity Clemson,SC USA and DepartmentofElectricalEngineering UniversityofMoratuwa Katubedda SriLanka
TiagoPinto
GECADResearchGrouponIntelligent EngineeringandComputingforAdvanced InnovationandDevelopment(GECAD) PolytechnicInstituteofPorto(ISEP/IPP)
DharmbirPrasad
AsansolEngineeringCollege Asansol,WestBengal India
CarlosRamos
GECADResearchGrouponIntelligent EngineeringandComputingforAdvanced InnovationandDevelopment(GECAD) PolytechnicInstituteofPorto(ISEP/IPP)
Porto
Portugal
SérgioRamos
Porto Portugal and UniversityofTrás-os-MonteseAltoDouro VilaReal Portugal
GECAD–ResearchGrouponIntelligent EngineeringandComputingforAdvanced InnovationandDevelopment,Polytechnicof Porto,SchoolofEngineering(ISEP) Porto Portugal
HuiyingRen
PacificNorthwestNationalLaboratory Richland,WA USA
AnselY.RodríguezGonzález TepicTechnologyTransferUnit,Centerfor ScientificResearchandHigherEducationof Ensenada Tepic Nayarit Mexico and NationalCouncilofScienceandTechnology MexicoCity
MexicoCity
Mexico
SajanK.Sadanandan SmartGridIntegration R&DCenter,DubaiElectricity&Water Authority(DEWA)
Dubai
UAE
EvgeniiSemshikov SchoolofEngineering,UniversityofTasmania Hobart,Tasmania
Australia
MahendraP.Sharma DepartmentofHydroandRenewableEnergy IndianInstituteofTechnology Roorkee,Uttarakhand
India
CátiaSilva
GECAD–ResearchGrouponIntelligent EngineeringandComputingforAdvanced InnovationandDevelopment
Porto
Portugal
RudraP.Singh AsansolEngineeringCollege Asansol,WestBengal
India
JoãoSoares
GECAD–ResearchGrouponIntelligent EngineeringandComputingforAdvanced InnovationandDevelopment,Polytechnicof Porto,SchoolofEngineering(ISEP)
Porto
Portugal
AnuragK.Srivastava SmartGridResiliencyandAnalyticsLab WestVirginiaUniversity Morgantown,WV
USA
SubhoUpadhyay DepartmentofElectricalEngineering,Faculty ofEngineering DayalbaghEducationalInstitute Agra,UttarPradesh
India
ZitaVale GECADResearchGrouponIntelligent EngineeringandComputingforAdvanced InnovationandDevelopment(GECAD) PolytechnicInstituteofPorto(ISEP/IPP)
Porto
Portugal
GaneshKumarVenayagamoorthy HolcombeDepartmentofElectricaland ComputerEngineering,Real-TimePowerand IntelligentSystemsLaboratory ClemsonUniversity Clemson,SC
USA and SchoolofEngineering UniversityofKwaZulu-Natal
Durban
SouthAfrica
XiaolinWang SchoolofEngineering,UniversityofTasmania Hobart,Tasmania
Australia
Foreword
Recentmachinelearninganddataanalyticsmethodshaveproliferatedintomostareasofscience, engineering,andcommerce.Thereareexcellentreasonsfortheirincreasingpopularityandapplications.Manyreal-worldproblemsaretoocomplextocomeupwithclosed-formanalyticalsolutions.However,suchchallengesdidnotmakepractitionersidle;instead,theyhavecreatedworking models,prototypesandevenbuiltsystemswithacarefulunderstandingofcriticalcomponentsof thesystemsasafirststep.Thedatageneratedfromsuchsystemsarethenanalyzedbymachine learninganddataanalyticsmethodstohaveamorecomprehensiveunderstandingofthesystems.
Thisbooktitled IntelligentDataMiningandAnalysisinPowerandEnergySystems makesahuge leapinthisdirectioninprovidingabetterunderstandingofpowerandenergysystems.Compiled byZitaVale,TiagoPinto,MichaelNegnevitsky,andGaneshKumarVenayagamoorthy,thebook beginswithanintroductiontomachinelearninganddataanalyticsmethodsandthenlaysout state-of-the-artmethodsinaddressingvarioustopicsinpowerandenergysystemdesign,clustering,classification,forecasting,andanalysiswithlatestmachinelearninganddataanalytics methods.
Thebookisself-containedandwrittenforbothnoviceandexpertsonthetopic.Thetopicsare discussedinsimplemannerwithadequatereferencesanddetails,sothatreaderscanunderstand thecurrentstate-of-the-artandalsofindrelevantpaststudiesinasinglevolume.
Ifyouareworkinginpowerandenergysystemseitherasaresearcherorapractitioner,thisisa must-havebooktostayaheadinthegame.Authorsareexpertsintheirownfields.Thebookwill saveyoureffortsinsearchingformaterialsonthetopic,provideyouwiththelatestmethodologies, anddirectyoutoothersimilarpaststudies.
Kudostotheeditorsforthiscompilationandauthorsfortheircontributions.
KalyanmoyDeb
UniversityDistinguishedProfessor
WithrowSeniorDistinguishedResearchScholar
KoenigEndowedChairProfessor
IEEECISEvolutionaryComputationPioneer
IEEEFellow
DepartmentofElectricalandComputerEngineering
MichiganStateUniversity,EastLansing,MI,USA
Introduction
Inaneraofever-increasingdata,thereisalsoanincreasingneedforthedevelopmentofsuitable intelligentdataminingandanalysissolutionsthatenabletakingthevalueoutofthesedata. Fosteredbythisincreasingneedandalsoboostedbytherecentworldwideboominartificial intelligenceinterestanddevelopment,wehavebeenwitnessingasignificantdevelopmentofa widearrayofnewadvanceddataminingmodelsandmethods.Thesemodelsandmethodologies havebeeninstrumentalindealingwithrealproblems,especiallyinhighlycomplexdomainssuch aspowerandenergysystems[1].
Thechallengesinpowerandenergysystemshavechangedcompletelyduringthepastyears, especiallybecauseoftheincreaseinthedistributedrenewableenergysourcesandtheconsequently requiredtransformationsinpowersystems’operation,management,andplanning,andalsoin electricitymarkets[2].Newplayersareemerging,suchasprosumers,electricvehicles,newtypesof aggregators,energycommunities,newlocalmarketoperators,energymanagersofdifferentkinds, amongmanyothers[3,4].Consequently,newbusinessmodelsarealsobeingproposed,experimented,andimplementedasthewaytoinvolvesuchnewplayersinthesectorinanactiveway whilecreatinganewvaluefortheseplayersandforthesystem,e.g.throughtheenhancementof theuseoflocalgenerationandthefosteringofconsumptionandgenerationflexibilitytrading[5].
Suchsignificantchangesinatraditionallyconservativesectorrequireanunprecedentedadaptationandforesightcapacityfromtheentireenergyvaluechain,includingfrompolicymakers, regulators,operators,planners,andevenfromthesmallerplayers.Thisiswheretheroleofnew andintelligentdataminingandanalysismodelsandmethodologiesbecomecrucial,contributing toovercomingmultipleproblemswithdistinctcharacteristics.Somerelevantexamplesarepower systemplanning,stateestimation,energyresourceprofiling,aggregationandforecasting,market negotiation,andenergymanagementatmultiplelevels,includingbuilding,microgrid,smartgrid, energycommunity,anddistributiongridlevels.
Thisbookprovidesacomprehensivereviewofintelligentdataminingandanalysisapplications inpowerandenergysystems.Thisbookisorganizedinsixcomplementaryparts,eachfocusing onaspecifictopicwithinthedataminingandanalysisdomain,namely,dataminingandanalysisfundamentals,clustering,classification,forecasting,dataanalysis,andothermachinelearning applications.Eachofthesixpartsisbrieflydescribedasfollows:
PartI: Dataminingandanalysisfundamentalsprovideanoverviewondataminingandanalysisfoundationsasameanstointroducethereadertothemainconceptsofthedomainand facilitatethedeeperunderstandingoftheworksdescribedintherestofthebook.Besidesthe mainconceptsbehinddataminingandanalysis,thefirstchapterisdedicatedtohighlighting theimportanceofdatapre-processingandfeatureengineeringasameanstoenableasuitable
IntelligentDataMiningandAnalysisinPowerandEnergySystems:ModelsandApplicationsforSmarterEfficient PowerSystems,FirstEdition.EditedbyZitaVale,TiagoPinto,MichaelNegnevitsky,andGaneshKumarVenayagamoorthy. ©2023TheInstituteofElectricalandElectronicsEngineers,Inc.Published2023byJohnWiley&Sons,Inc.
applicationofthestate-of-the-artmodelsdedicatedtothediversetraditionalproblemsrelated todatamining.Thisintroductoryoverviewprovidesthemeansforadeeperunderstandingof dataminingandanalysis,bridgingthereaderintothepowerandenergysystemsapplication domainthroughthepresentationoftwosystematicreviews,namely,ondataminingandanalysisapplicationsinpowerandenergysystemsandonthecontributionsofdeeplearninginpower systemproblems.Thesetwochaptersaddressdifferentproblemswithinpowerandenergysystems,whichbenefitfromtheadvancesofdataminingmodelsrelatedtoclustering,classification, forecasting,andothercommonapproaches.
PartII: Clusteringpresentsadescriptionofworksthatapplyclusteringmodelsandmethodsto addresspowerandenergysystemproblems.Theseincludestandardclusteringapproaches,as wellasthecombinationofclusteringwithothermodels,e.g.classification-based,tosolvedifferenttypesofproblems.Specifically,thepowerandenergysystemproblemsaddressedbythis partincludeconsumer-directedproblemsrelatedtoconsumerclusteringanddemandprofiling.Aggregationproblemsconsideringnotonlytheaggregationofconsumersbutalsooftheir consumptionflexibilityareexploredasameanstosolvedemandresponsechallengesinwider scales.Synchrophasordataanalyticstakingadvantageonclusteringmodelsandtheircombinationwithotherapproachesarealsoaddressed,aimingatanomalydetection,localization,and classification.
PartIII: Classificationincludesthedescriptionofworksthatapplyclassificationmodelssuchas artificialneuralnetworks,supportvectormachines,K-nearestneighbors,amongothers,tosolve problemsusinglabeleddata.Theapplicationcasesoftheseworksarerelatedtonon-technical lossdetectioninelectricdistributionsystems,electricalvehicleintegrationinthepowersystemundermultipleworldwideperspectivesandconsideringdifferenttypesoftechnologies,and electricitymarketparticipationanddecisionsupport,namely,inthescopeofbilateralcontract negotiationsusinghistoricandoverserveddatafrommultiplenegotiators’negotiationprocess.
PartIV: Forecastingisdevotedtothedescriptionofworksrelatedtotheforecastingofenergy resourceswithdistinctcharacteristics.Theseworksaremainlyfocusedontheforecastingof highlyvariablerenewableenergysources;besides,theapplicationoftraditionalregression-based algorithmsdescribestheadvantagesofspecificapproachessuchasmultivariatestochasticmodels,spatiotemporalmodels,anddecomposition-basedmodels.Theforecastingmodelsare appliedtosolarirradianceandtemperatureestimationandtowindandsolarpowerforecasting underdistinctscenariosregardinghistoricaldata,powersystemcharacteristics,andoverall renewableenergypenetration.
PartV: Dataanalysispresentstheapplicationofdataanalysismodelsofdistinctnaturestoaddress differenttypesofpowersystem-relatedproblems.Theseproblemsconcernissuessuchasthe vibrationoftransmissionlineconductorsthroughtheanalysisofharmonicdynamicresponse andthedesignofpowerdistributionnetworkinhillyareaswiththepurposeofenablingoff-grid electrification.Theapplicationofintelligentdemandresponsemodelsaspartofmicrogridplanningisanotheroftheaddressedproblems.Thispartisfinalizedwithachapterfocusingon socioeconomicanalysisofrenewableenergyinterventionstowardaffordableandsustainable householdtechnologies.
PartVI: Othermachinelearningapplicationsdescribeapplicationsthatusedistincttypesof machinelearningapproachessuchasreinforcementlearning,federatedlearning,andprobabilisticmodeling,addressingavariedsetofchallengesofnatures.Suchchallengesincludethe stateestimationofpowerelectronicconverters,usingbothwhiteboxandblackboxapproaches. Theproblemofintelligentbuildingenergymanagementandcontrolisaddressedusing reinforcementlearning.Federateddeeplearningisappliedtogenerateglobalsupermodelsfor
powersystemdataanalysis,andriskassessmentofpowersystemoutagesisperformedthrough probabilisticmodeling,consideringweatherandclimateextremes.
Overall,thisbookcomprisesthedescriptionofawidesetofintelligentdataminingandanalysis models,methodologies,andapplications,addressingproblemsofdistinctnatureswithinthefield ofpowerandenergysystems,whilehighlightingtheadvantagesofthealreadyachievedbreakthroughsinthedomainandpointingoutthemaingapsthathavenotyetbeensolved,aspointers forfuturepathsofcontinuousresearchanddevelopment.
ZitaVale PolytechnicofPorto,Portugal TiagoPinto PolytechnicofPorto,Portugal UniversityofTrás-os-MonteseAltoDouro Portugal MichaelNegnevitsky UniversityofTasmania,Australia GaneshKumarVenayagamoorthy ClemsonUniversity,USA
References
1 Ibrahim,M.S.,Dong,W.,andYang,Q.(2020).Machinelearningdrivensmartelectricpowersystems:currenttrendsandnewperspectives. AppliedEnergy 272:115237.
2 Pinto,T.,Vale,Z.,Widergren,S.,andeditors.(2021). LocalElectricityMarkets,1e.Academic Press384pp.https://www.elsevier.com/books/local-electricity-markets/pinto/978-0-12-820074-2.
3 Koirala,B.P.,Koliou,E.,Friege,J.etal.(2016).Energeticcommunitiesforcommunityenergy:a reviewofkeyissuesandtrendsshapingintegratedcommunityenergysystems. Renewableand SustainableEnergyReviews 56:722–744.
4 deSão,J.D.,Faria,P.,andVale,Z.(2021).Smartenergycommunity:asystematicreviewwith metanalysis. EnergyStrategyReviews 36:100678.
5 Hall,S.andRoelich,K.(2016).Businessmodelinnovationinelectricitysupplymarkets:therole ofcomplexvalueintheUnitedKingdom. EnergyPolicy 92:286–298.