Deep Reinforcement Learning for
Wireless Communications and Networking: Theory, Applications and Implementation Dinh Thai Hoang
Visit to download the full and correct content document: https://ebookmass.com/product/deep-reinforcement-learning-for-wireless-communicat ions-and-networking-theory-applications-and-implementation-dinh-thai-hoang/
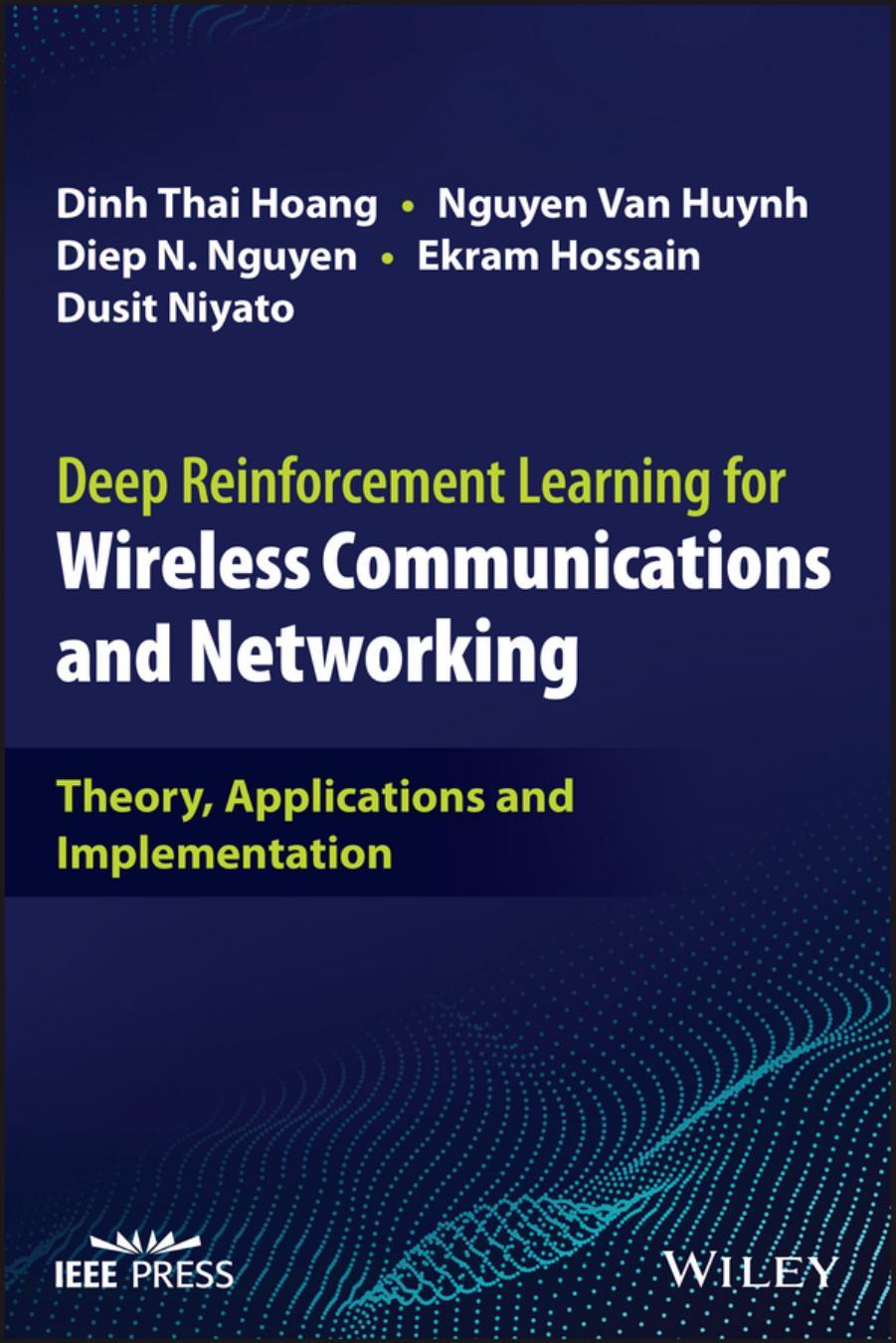
More products digital (pdf, epub, mobi) instant download maybe you interests ...
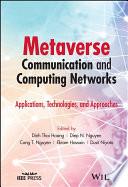
Metaverse Communication and Computing Networks: Applications, Technologies, and Approaches Dinh Thai Hoang
https://ebookmass.com/product/metaverse-communication-andcomputing-networks-applications-technologies-and-approaches-dinhthai-hoang/
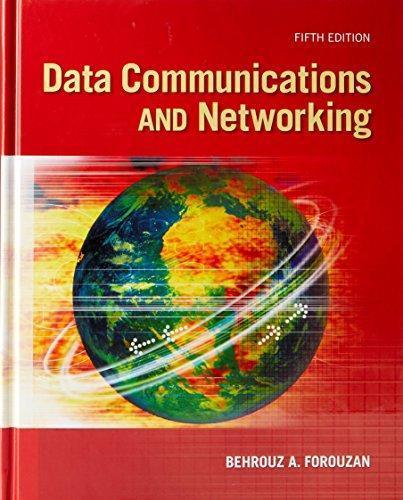
Data Communications and Networking 5th Edition
https://ebookmass.com/product/data-communications-andnetworking-5th-edition/
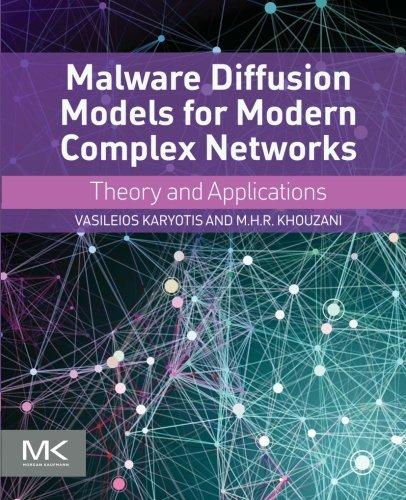
Malware Diffusion Models for Wireless Complex Networks. Theory and Applications 1st Edition Karyotis
https://ebookmass.com/product/malware-diffusion-models-forwireless-complex-networks-theory-and-applications-1st-editionkaryotis/
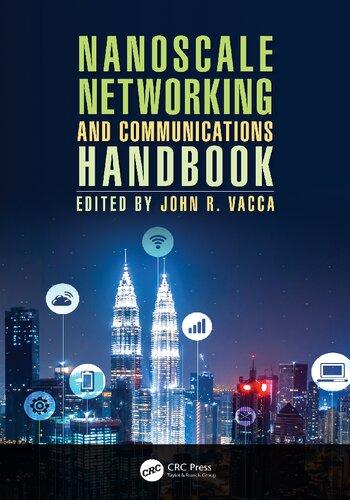
Nanoscale Networking and Communications Handbook John R. Vacca
https://ebookmass.com/product/nanoscale-networking-andcommunications-handbook-john-r-vacca/
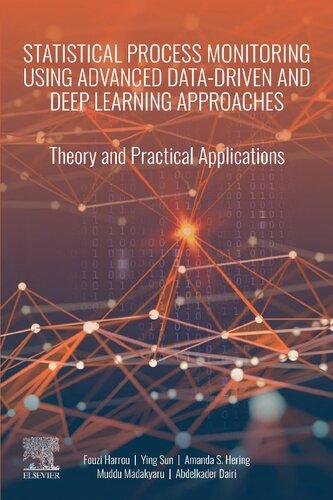
Statistical Process Monitoring Using Advanced DataDriven and Deep Learning Approaches: Theory and Practical Applications 1st Edition Fouzi Harrou
https://ebookmass.com/product/statistical-process-monitoringusing-advanced-data-driven-and-deep-learning-approaches-theoryand-practical-applications-1st-edition-fouzi-harrou/

Risk Modeling: Practical Applications of Artificial Intelligence, Machine Learning, and Deep Learning
Terisa Roberts
https://ebookmass.com/product/risk-modeling-practicalapplications-of-artificial-intelligence-machine-learning-anddeep-learning-terisa-roberts/
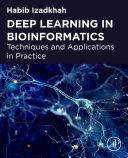
Deep Learning in Bioinformatics: Techniques and Applications in Practice Habib Izadkhah
https://ebookmass.com/product/deep-learning-in-bioinformaticstechniques-and-applications-in-practice-habib-izadkhah/
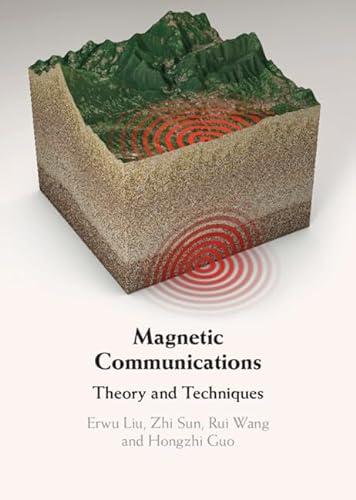
Magnetic Communications: Theory and Techniques Liu
https://ebookmass.com/product/magnetic-communications-theory-andtechniques-liu/
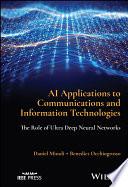
AI Applications to Communications and Information Technologies: The Role of Ultra Deep Neural Networks
Daniel Minoli
https://ebookmass.com/product/ai-applications-to-communicationsand-information-technologies-the-role-of-ultra-deep-neuralnetworks-daniel-minoli/
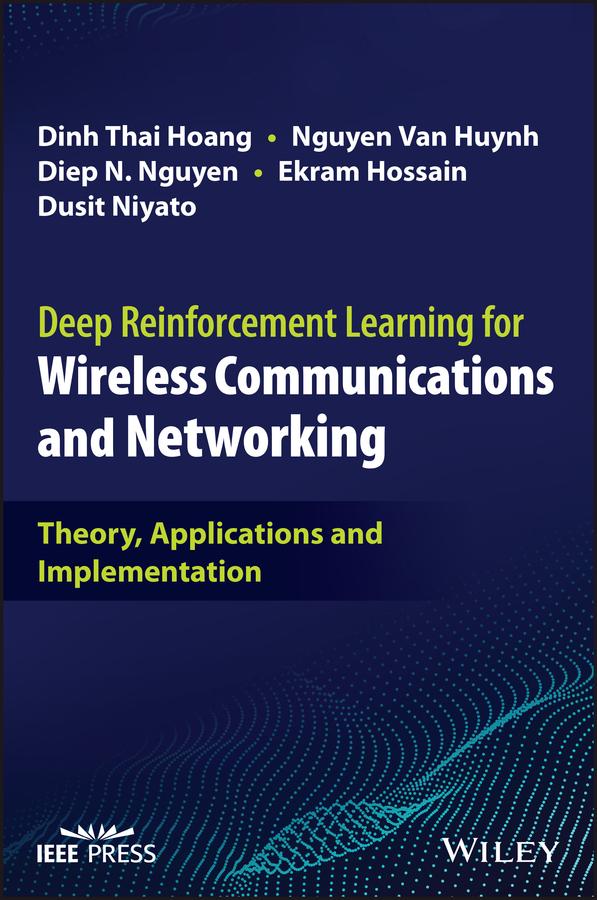
IEEEPress
445HoesLane
Piscataway,NJ08854
IEEEPressEditorialBoard
SarahSpurgeon, EditorinChief
JónAtliBenediktsson
AnjanBose
JamesDuncan
AminMoeness
DesineniSubbaramNaidu
BehzadRazavi
JimLyke
HaiLi
BrianJohnson
JeffreyReed
DiomidisSpinellis
AdamDrobot
TomRobertazzi
AhmetMuratTekalp
DeepReinforcementLearningforWireless CommunicationsandNetworking
Theory,Applications,andImplementation
DinhThaiHoang
UniversityofTechnologySydney,Australia
NguyenVanHuynh
EdinburghNapierUniversity,UnitedKingdom
DiepN.Nguyen
UniversityofTechnologySydney,Australia
EkramHossain UniversityofManitoba,Canada
DusitNiyato
NanyangTechnologicalUniversity,Singapore
Copyright©2023byTheInstituteofElectricalandElectronicsEngineers,Inc.Allrights reserved.
PublishedbyJohnWiley&Sons,Inc.,Hoboken,NewJersey.
PublishedsimultaneouslyinCanada.
Nopartofthispublicationmaybereproduced,storedinaretrievalsystem,ortransmittedinany formorbyanymeans,electronic,mechanical,photocopying,recording,scanning,orotherwise, exceptaspermittedunderSection107or108ofthe1976UnitedStatesCopyrightAct,without eitherthepriorwrittenpermissionofthePublisher,orauthorizationthroughpaymentofthe appropriateper-copyfeetotheCopyrightClearanceCenter,Inc.,222RosewoodDrive,Danvers, MA01923,(978)750-8400,fax(978)750-4470,oronthewebatwww.copyright.com.Requeststo thePublisherforpermissionshouldbeaddressedtothePermissionsDepartment,JohnWiley& Sons,Inc.,111RiverStreet,Hoboken,NJ07030,(201)748-6011,fax(201)748-6008,oronlineat http//www.wiley.com/go/permission.
TrademarksWileyandtheWileylogoaretrademarksorregisteredtrademarksofJohnWiley& Sons,Inc.and/oritsaffiliatesintheUnitedStatesandothercountriesandmaynotbeused withoutwrittenpermission.Allothertrademarksarethepropertyoftheirrespectiveowners. JohnWiley&Sons,Inc.isnotassociatedwithanyproductorvendormentionedinthisbook.
LimitofLiability/DisclaimerofWarrantyWhilethepublisherandauthorhaveusedtheirbest effortsinpreparingthisbook,theymakenorepresentationsorwarrantieswithrespecttothe accuracyorcompletenessofthecontentsofthisbookandspecificallydisclaimanyimplied warrantiesofmerchantabilityorfitnessforaparticularpurpose.Nowarrantymaybecreatedor extendedbysalesrepresentativesorwrittensalesmaterials.Theadviceandstrategiescontained hereinmaynotbesuitableforyoursituation.Youshouldconsultwithaprofessionalwhere appropriate.Neitherthepublishernorauthorshallbeliableforanylossofprofitoranyother commercialdamages,includingbutnotlimitedtospecial,incidental,consequential,orother damages.Further,readersshouldbeawarethatwebsiteslistedinthisworkmayhavechanged ordisappearedbetweenwhenthisworkwaswrittenandwhenitisread.Neitherthepublisher norauthorsshallbeliableforanylossofprofitoranyothercommercialdamages,includingbut notlimitedtospecial,incidental,consequential,orotherdamages.
Forgeneralinformationonourotherproductsandservicesorfortechnicalsupport,please contactourCustomerCareDepartmentwithintheUnitedStatesat(800)762-2974,outsidethe UnitedStatesat(317)572-3993orfax(317)572-4002.
Wileyalsopublishesitsbooksinavarietyofelectronicformats.Somecontentthatappearsin printmaynotbeavailableinelectronicformats.FormoreinformationaboutWileyproducts, visitourwebsiteatwww.wiley.com.
LibraryofCongressCataloging-in-PublicationDataappliedfor:
HardbackISBN:9781119873679
CoverDesign:Wiley CoverImage:©Liuzishan/Shutterstock
Setin9.5/12.5ptSTIXTwoTextbyStraive,Chennai,India
Tomyfamily–DinhThaiHoang
Tomyfamily–NguyenVanHuynh
ToVeronicaHaiBinh,PaulSonNam,andThuy–DiepN.Nguyen
Tomyparents–EkramHossain
Tomyfamily–DusitNiyato
Contents
NotesonContributors xiii
Foreword xiv
Preface xv
Acknowledgments xviii
Acronyms xix
Introduction xxii
PartIFundamentalsofDeepReinforcementLearning 1
1DeepReinforcementLearningandItsApplications 3
1.1WirelessNetworksandEmergingChallenges 3
1.2MachineLearningTechniquesandDevelopmentofDRL 4
1.2.1MachineLearning 4
1.2.2ArtificialNeuralNetwork 7
1.2.3ConvolutionalNeuralNetwork 8
1.2.4RecurrentNeuralNetwork 9
1.2.5DevelopmentofDeepReinforcementLearning 10
1.3PotentialsandApplicationsofDRL 11
1.3.1BenefitsofDRLinHumanLives 11
1.3.2FeaturesandAdvantagesofDRLTechniques 12
1.3.3AcademicResearchActivities 12
1.3.4ApplicationsofDRLTechniques 13
1.3.5ApplicationsofDRLTechniquesinWirelessNetworks 15
1.4StructureofthisBookandTargetReadership 16
1.4.1MotivationsandStructureofthisBook 16
1.4.2TargetReadership 19
1.5ChapterSummary 20
References 21
2MarkovDecisionProcessandReinforcementLearning 25
2.1MarkovDecisionProcess 25
2.2PartiallyObservableMarkovDecisionProcess 26
2.3PolicyandValueFunctions 29
2.4BellmanEquations 30
2.5SolutionsofMDPProblems 31
2.5.1DynamicProgramming 31
2.5.1.1PolicyEvaluation 31
2.5.1.2PolicyImprovement 31
2.5.1.3PolicyIteration 31
2.5.2MonteCarloSampling 32
2.6ReinforcementLearning 33
2.7ChapterSummary 35 References 35
3DeepReinforcementLearningModelsandTechniques 37
3.1Value-BasedDRLMethods 37
3.1.1DeepQ-Network 38
3.1.2DoubleDQN 41
3.1.3PrioritizedExperienceReplay 42
3.1.4DuelingNetwork 44
3.2Policy-GradientMethods 45
3.2.1REINFORCEAlgorithm 46
3.2.1.1PolicyGradientEstimation 46
3.2.1.2ReducingtheVariance 48
3.2.1.3PolicyGradientTheorem 50
3.2.2Actor-CriticMethods 51
3.2.3AdvantageofActor-CriticMethods 52
3.2.3.1AdvantageofActor-Critic(A2C) 53
3.2.3.2AsynchronousAdvantageActor-Critic(A3C) 55
3.2.3.3GeneralizedAdvantageEstimate(GAE) 57
3.3DeterministicPolicyGradient(DPG) 59
3.3.1DeterministicPolicyGradientTheorem 59
3.3.2DeepDeterministicPolicyGradient(DDPG) 61
3.3.3DistributedDistributionalDDPG(D4PG) 63
3.4NaturalGradients 63
3.4.1PrincipleofNaturalGradients 64
3.4.2TrustRegionPolicyOptimization(TRPO) 67
3.4.2.1TrustRegion 69
3.4.2.2Sample-BasedFormulation 70
3.4.2.3PracticalImplementation 70
3.4.3ProximalPolicyOptimization(PPO) 72
3.5Model-BasedRL 74
3.5.1VanillaModel-BasedRL 75
3.5.2RobustModel-BasedRL:Model-EnsembleTRPO(ME-TRPO) 76
3.5.3AdaptiveModel-BasedRL:Model-BasedMeta-PolicyOptimization (MB-MPO) 77
3.6ChapterSummary 78 References 79
4ACaseStudyandDetailedImplementation 83
4.1SystemModelandProblemFormulation 83
4.1.1SystemModelandAssumptions 84
4.1.1.1JammingModel 84
4.1.1.2SystemOperation 85
4.1.2ProblemFormulation 86
4.1.2.1StateSpace 86
4.1.2.2ActionSpace 87
4.1.2.3ImmediateReward 88
4.1.2.4OptimizationFormulation 88
4.2ImplementationandEnvironmentSettings 89
4.2.1InstallTensorFlowwithAnaconda 89
4.2.2Q-Learning 90
4.2.2.1CodesfortheEnvironment 91
4.2.2.2CodesfortheAgent 96
4.2.3DeepQ-Learning 97
4.3SimulationResultsandPerformanceAnalysis 102
4.4ChapterSummary 106 References 106
PartIIApplicationsofDRLinWirelessCommunications andNetworking 109
5DRLatthePhysicalLayer 111
5.1Beamforming,SignalDetection,andDecoding 111
5.1.1Beamforming 111
5.1.1.1BeamformingOptimizationProblem 111
5.1.1.2DRL-BasedBeamforming 113
5.1.2SignalDetectionandChannelEstimation 118
5.1.2.1SignalDetectionandChannelEstimationProblem 118
5.1.2.2RL-BasedApproaches 120
x Contents
5.1.3ChannelDecoding 122
5.2PowerandRateControl 123
5.2.1PowerandRateControlProblem 123
5.2.2DRL-BasedPowerandRateControl 124
5.3Physical-LayerSecurity 128
5.4ChapterSummary 129 References 131
6DRLattheMACLayer 137
6.1ResourceManagementandOptimization 137
6.2ChannelAccessControl 139
6.2.1DRLintheIEEE802.11MAC 141
6.2.2MACforMassiveAccessinIoT 143
6.2.3MACfor5GandB5GCellularSystems 147
6.3HeterogeneousMACProtocols 155
6.4ChapterSummary 158 References 158
7DRLattheNetworkLayer 163
7.1TrafficRouting 163
7.2NetworkSlicing 166
7.2.1NetworkSlicing-BasedArchitecture 166
7.2.2ApplicationsofDRLinNetworkSlicing 168
7.3NetworkIntrusionDetection 179
7.3.1Host-BasedIDS 180
7.3.2Network-BasedIDS 181
7.4ChapterSummary 183 References 183
8DRLattheApplicationandServiceLayer 187
8.1ContentCaching 187
8.1.1QoS-AwareCaching 187
8.1.2JointCachingandTransmissionControl 189
8.1.3JointCaching,Networking,andComputation 191
8.2DataandComputationOffloading 193
8.3DataProcessingandAnalytics 198
8.3.1DataOrganization 198
8.3.1.1DataPartitioning 198
8.3.1.2DataCompression 199
8.3.2DataScheduling 200
8.3.3TuningofDataProcessingSystems 201
8.3.4DataIndexing 202
8.3.4.1DatabaseIndexSelection 202
8.3.4.2IndexStructureConstruction 203
8.3.5QueryOptimization 205
8.4ChapterSummary 206 References 207
PartIIIChallenges,Approaches,OpenIssues,and EmergingResearchTopics 213
9DRLChallengesinWirelessNetworks 215
9.1AdversarialAttacksonDRL 215
9.1.1AttacksPerturbingtheStatespace 215
9.1.1.1ManipulationofObservations 216
9.1.1.2ManipulationofTrainingData 218
9.1.2AttacksPerturbingtheRewardFunction 220
9.1.3AttacksPerturbingtheActionSpace 222
9.2MultiagentDRLinDynamicEnvironments 223
9.2.1Motivations 223
9.2.2MultiagentReinforcementLearningModels 224
9.2.2.1Markov/StochasticGames 225
9.2.2.2DecentralizedPartiallyObservableMarkovDecisionProcess (DPOMDP) 226
9.2.3ApplicationsofMultiagentDRLinWirelessNetworks 227
9.2.4ChallengesofUsingMultiagentDRLinWirelessNetworks 229
9.2.4.1NonstationarityIssue 229
9.2.4.2PartialObservabilityIssue 229
9.3OtherChallenges 230
9.3.1InherentProblemsofUsingRLinReal-WordSystems 230
9.3.1.1LimitedLearningSamples 230
9.3.1.2SystemDelays 230
9.3.1.3High-DimensionalStateandActionSpaces 231
9.3.1.4SystemandEnvironmentConstraints 231
9.3.1.5PartialObservabilityandNonstationarity 231
9.3.1.6MultiobjectiveRewardFunctions 232
9.3.2InherentProblemsofDLandBeyond 232
9.3.2.1InherentProblemsofDL 232
9.3.2.2ChallengesofDRLBeyondDeepLearning 233
9.3.3ImplementationofDLModelsinWirelessDevices 236
9.4ChapterSummary 237 References 237
10DRLandEmergingTopicsinWirelessNetworks 241
10.1DRLforEmergingProblemsinFutureWirelessNetworks 241
10.1.1JointRadarandDataCommunications 241
10.1.2AmbientBackscatterCommunications 244
10.1.3ReconfigurableIntelligentSurface-AidedCommunications 247
10.1.4RateSplittingCommunications 249
10.2AdvancedDRLModels 252
10.2.1DeepReinforcementTransferLearning 252
10.2.1.1RewardShaping 253
10.2.1.2IntertaskMapping 254
10.2.1.3LearningfromDemonstrations 255
10.2.1.4PolicyTransfer 255
10.2.1.5ReusingRepresentations 256
10.2.2GenerativeAdversarialNetwork(GAN)forDRL 257
10.2.3MetaReinforcementLearning 258
10.3ChapterSummary 259 References 259
Index 263
NotesonContributors
DinhThaiHoang SchoolofElectricalandData
Engineering
UniversityofTechnologySydney
Australia
NguyenVanHuynh SchoolofComputing,Engineeringand theBuiltEnvironment
EdinburghNapierUniversity
UK
DiepN.Nguyen SchoolofElectricalandData
Engineering
UniversityofTechnologySydney
Australia
EkramHossain DepartmentofElectricaland ComputerEngineering UniversityofManitoba
Canada
DusitNiyato SchoolofComputerScienceand Engineering NanyangTechnologicalUniversity
Singapore
Foreword
Prof.MerouaneDebbah,Integratingdeepreinforcementlearning(DRL)techniquesinwirelesscommunicationsandnetworkinghaspavedthewayfor achievingefficientandoptimizedwirelesssystems.Thisground-breakingbook providesexcellentmaterialforresearcherswhowanttostudyapplicationsofdeep reinforcementlearninginwirelessnetworks,withmanypracticalexamplesand implementationdetailsforthereaderstopractice.Italsocoversvarioustopicsat differentnetworklayers,suchaschannelaccess,networkslicing,andcontent caching.Thisbookisessentialforanyonelookingtostayaheadofthecurvein thisexcitingfield.
Prof.VincentPoor,Manyaspectsofwirelesscommunicationsandnetworkingare beingtransformedthroughtheapplicationofdeepreinforcementlearning(DRL) techniques.Thisbookrepresentsanimportantcontributiontothisfield,providingacomprehensivetreatmentofthetheory,applications,andimplementation ofDRLinwirelesscommunicationsandnetworking.Animportantaspectofthis bookisitsfocusonpracticalimplementationissues,suchassystemdesign,algorithmimplementation,andreal-worlddeploymentchallenges.Bybridgingthe gapbetweentheoryandpractice,theauthorsprovidereaderswiththetoolsto buildanddeployDRL-basedwirelesscommunicationandnetworkingsystems. Thisbookisausefulresourceforthoseinterestedinlearningaboutthepotential ofDRLtoimprovewirelesscommunicationsandnetworkingsystems.Itsbreadth anddepthofcoverage,practicalfocus,andexpertinsightsmakeitasingularcontributiontothefield.
Preface
Reinforcementlearningisoneofthemostimportantresearchdirectionsof machinelearning(ML),whichhashadsignificantimpactsonthedevelopment ofartificialintelligence(AI)overthelast20years.Reinforcementlearningis alearningprocessinwhichanagentcanperiodicallymakedecisions,observe theresults,andthenautomaticallyadjustitsstrategytoachieveanoptimal policy.However,thislearningprocess,evenwithprovenconvergence,oftentakes asignificantamountoftimetoreachthebestpolicyasithastoexploreand gainknowledgeofanentiresystem,makingitunsuitableandinapplicableto large-scalesystemsandnetworks.Consequently,applicationsofreinforcement learningareverylimitedinpractice.Recently,deeplearninghasbeenintroducedasanewbreakthroughMLtechnique.Itcanovercomethelimitationsof reinforcementlearningandthusopenaneweraforthedevelopmentofreinforcementlearning,namely deepreinforcementlearning (DRL).DRLembraces theadvantageofdeepneuralnetworks(DNNs)totrainthelearningprocess, therebyimprovingthelearningrateandtheperformanceofreinforcement learningalgorithms.Asaresult,DRLhasbeenadoptedinnumerousapplications ofreinforcementlearninginpracticesuchasrobotics,computervision,speech recognition,andnaturallanguageprocessing.
Intheareasofcommunicationsandnetworking,DRLhasbeenrecentlyused asaneffectivetooltoaddressvariousproblemsandchallenges.Inparticular, modernnetworkssuchastheInternet-of-Things(IoT),heterogeneousnetworks(HetNets),andunmannedaerialvehicle(UAV)networksbecomemore decentralized,ad-hoc,andautonomousinnature.NetworkentitiessuchasIoT devices,mobileusers,andUAVsneedtomakelocalandindependentdecisions, e.g.spectrumaccess,datarateadaption,transmitpowercontrol,andbasestation association,toachievethegoalsofdifferentnetworksincluding,e.g.throughputmaximizationandenergyconsumptionminimization.Inuncertainand stochasticenvironments,mostofthedecision-makingproblemscanbemodeled asaso-called Markovdecisionprocess (MDP).Dynamicprogrammingandother
algorithmssuchasvalueiteration,aswellasreinforcementlearningtechniques, canbeadoptedtosolvetheMDP.However,modernnetworksarelarge-scaleand complicated,andthusthecomputationalcomplexityofthetechniquesrapidly becomesunmanageable,i.e.curseofdimensionality.Asaresult,DRLhasbeen developingasanalternativesolutiontoovercomethechallenge.Ingeneral,the DRLapproachesprovidethefollowingadvantages:
● DRLcaneffectivelyobtainthesolutionofsophisticatednetworkoptimizations, especiallyincaseswithincompleteinformation.Thus,itenablesnetwork entities,e.g.basestations,inmodernnetworkstosolvenon-convexand complexproblems,e.g.jointuserassociation,computation,andtransmission schedule,toachieveoptimalsolutionswithoutcompleteandaccuratenetwork information.
● DRLallowsnetworkentitiestolearnandbuildknowledgeaboutthecommunicationandnetworkingenvironment.Thus,byusingDRL,thenetworkentities, e.g.amobileuser,canlearnoptimalpolicies,e.g.basestationselection,channelselection,handoverdecision,caching,andoffloadingdecisions,without knowingapriorichannelmodelandmobilitypattern.
● DRLprovidesautonomousdecision-making.WiththeDRLapproach,network entitiescanmakeobservationsandobtainthebestpolicylocallywithminimumorwithoutinformationexchangeamongeachother.Thisnotonlyreduces communicationoverheadsbutalsoimprovesthesecurityandrobustnessofthe networks.
● DRLsignificantlyimprovesthelearningspeed,especiallyinproblemswithlarge stateandactionspaces.Thus,inlarge-scalenetworks,e.g.IoTsystemswith thousandsofdevices,DRLallowsthenetworkcontrollerorIoTgatewaysto controldynamicallyuserassociation,spectrumaccess,andtransmitpowerfor amassivenumberofIoTdevicesandmobileusers.
● Severalotherproblemsincommunicationsandnetworkingsuchas cyber-physicalattacks,interferencemanagement,anddataoffloadingcan bemodeledasgames,e.g.thenon-cooperativegame.DRLhasbeenrecently extendedandusedasanefficienttooltosolvecompetitor,e.g.findingtheNash equilibrium,withoutcompleteinformation.
Clearly,DRLwillbethekeyenablerforthenextgenerationofwirelessnetworks. Therefore,DRLisofincreasinginteresttoresearchers,communicationengineers, computerscientists,andapplicationdevelopers.Inthisregard,weintroducea newbook,titled“DeepReinforcementLearningforWirelessCommunicationsandNetworking:Theory,Applications,andImplementation”,which willprovideafundamentalbackgroundofDRLandthenstudyrecentadvancesin DRLtoaddresspracticalchallengesinwirelesscommunicationsandnetworking. Inparticular,thisbookfirstgivesatutorialonDRL,frombasicconceptsto
Preface
advancedmodelingtechniquestomotivateandprovidefundamentalknowledge forthereaders.Wethenprovidecasestudiestogetherwithimplementation detailstohelpthereadersbetterunderstandhowtopracticeandapplyDRLto theirproblems.Afterthat,wereviewDRLapproachesthataddressemerging issuesincommunicationsandnetworking.Theissuesincludedynamicnetwork access,dataratecontrol,wirelesscaching,dataoffloading,networksecurity,and connectivitypreservation,whichareallimportanttonext-generationnetworks suchas5Gandbeyond.Finally,wehighlightimportantchallenges,openissues, andfutureresearchdirectionsforapplyingDRLtowirelessnetworks.
Acknowledgments
Theauthorswouldliketoacknowledgegrant-awardingagenciesthatsupported partsofthisbook.ThisresearchwassupportedinpartbytheAustralianResearch CouncilundertheDECRAprojectDE210100651andtheNaturalSciencesand EngineeringResearchCouncilofCanada(NSERC).
TheauthorswouldliketothankMr.CongThanhNguyen,Mr.HieuChiNguyen, Mr.NamHoaiChu,andMr.KhoaVietTranfortheirtechnicalassistanceand discussionsduringthewritingofthisbook.
Acronyms NoAcronymsTerms
1A3Casynchronousadvantageactor-critic
2ACKacknowledgmentmessage
3AIartificialintelligence
4ANNartificialneuralnetwork
5APaccesspoint
6BERbiterrorrate
7BSbasestation
8CNNconvolutionalneuralnetwork
9CSIchannelstateinformation
10D2Ddevice-to-device
11DDPGdeepdeterministicpolicygradient
12DDQNdoubledeepQ-network
13DLdeeplearning
14DNNdeepneuralnetwork
15DPGdeterministicpolicygradient
16DQNdeepQ-learning
17DRLdeepreinforcementlearning
18eMBBenhancedmobilebroadband
19FLfederatedlearning
20FSMCfinite-stateMarkovchain
21GANgenerativeadversarialnetwork
22GPUgraphicsprocessingunit
23IoTInternet-of-Things
NoAcronymsTerms
24ITSintelligenttransportationsystem
25LTELong-termevolution
26M2Mmachine-to-machine
27MACmediumaccesscontrol
28MARLmulti-agentRL
29MDPMarkovdecisionprocess
30MECmobileedgecomputing
31MIMOmultiple-inputmultiple-output
32MISOMulti-inputsingle-output
33MLmachinelearning
34mMTCmassivemachinetypecommunications
35mmWavemillimeterwave
36MUmobileuser
37NFVnetworkfunctionvirtualization
38OFDMAorthogonalfrequencydivisionmultipleaccess
39POMDPpartiallyobservableMarkovdecisionprocess
40PPOproximalpolicyoptimization
41PSRpredictivestaterepresentation
42QoEQualityofExperience
43QoSQualityofService
44RANradioaccessnetwork
45RBresourceblock
46RFradiofrequency
47RISreconfigurableintelligentsurface
48RLreinforcementlearning
49RNNrecurrentneuralnetwork
50SARSAstate-action-reward-state-action
51SDNsoftware-definednetworking
52SGDstochasticgradientdescent
53SINRsignal-to-interference-plus-noiseratio
54SMDPsemi-Markovdecisionprocess
55TDtemporaldifference
56TDMAtime-divisionmultipleaccess
57TRPOtrustregionpolicyoptimization
NoAcronymsTerms
58UAVunmannedaerialvehicle
59UEuserequipment
60ULuplink
61URLLCultra-reliableandlow-latencycommunications
62VANETvehicularadhocNETworks
63VNFvirtualnetworkfunction
64WLANwirelesslocalareanetwork
65WSNwirelesssensornetwork
Introduction
Deepreinforcementlearning(DRL)empoweredbydeepneuralnetworks(DNNs) hasbeendevelopingasapromisingsolutiontoaddresshigh-dimensionaland continuouscontrolproblemseffectively.TheintegrationofDRLintofuturewirelessnetworkswillrevolutionizeconventionalmodel-basednetworkoptimization withmodel-freeapproachesandmeetvariousapplicationdemands.Byinteracting withtheenvironment,DRLprovidesanautonomousdecision-makingmechanismforthenetworkentitiestosolvenon-convex,complex,model-freeproblems, e.g.spectrumaccess,handover,scheduling,caching,dataoffloading,andresource allocation.Thisnotonlyreducescommunicationoverheadbutalsoimproves networksecurityandreliability.ThoughDRLhasshowngreatpotentialtoaddress emergingissuesincomplexwirelessnetworks,therearestilldomain-specific challengesthatrequirefurtherinvestigation.Thechallengesmayincludethe designofproperDNNarchitecturestocapturethecharacteristicsof5Gnetwork optimizationproblems,thestateexplosionindensenetworks,multi-agent learningindynamicnetworks,limitedtrainingdataandexplorationspacein practicalnetworks,theinaccessibilityandhighcostofnetworkinformation,as wellasthebalancebetweeninformationqualityandlearningperformance.
ThisbookprovidesacomprehensiveoverviewofDRLanditsapplicationsto wirelesscommunicationandnetworking.Itcoversawiderangeoftopicsfrom basictoadvancedconcepts,focusingonimportantaspectsrelatedtoalgorithms, models,performanceoptimizations,machinelearning,andautomationforfuture wirelessnetworks.Asaresult,thisbookwillprovideessentialtoolsandknowledge forresearchers,engineers,developers,andgraduatestudentstounderstandand beabletoapplyDRLtotheirwork.Webelievethatthisbookwillnotonlybeof greatinteresttothoseinthefieldsofwirelesscommunicationandnetworkingbut alsotothoseinterestedinDRLandAImorebroadly.
1.1WirelessNetworksandEmergingChallenges
Overthepastfewyears,communicationtechnologieshavebeenrapidlydevelopingtosupportvariousaspectsofourdailylives,fromsmartcitiesandhealthcareto logisticsandtransportation.Thiswillbethebackboneforthefuture’sdata-centric society.Nevertheless,thesenewapplicationsgenerateatremendousamount ofworkloadandrequirehigh-reliabilityandultrahigh-capacitywirelesscommunications.Inthelatestreport[1],Ciscoprojectedthenumberofconnected devicesthatwillbearound29.3billionby2023,withmorethan45%equipped withmobileconnections.Thefastest-growingmobileconnectiontypeislikely machine-to-machine(M2M),asInternet-of-Things(IoT)servicesplayasignificantroleinconsumerandbusinessenvironments.Thisposesseveralchallenges infuturewirelesscommunicationsystems:
● Emergingservices(e.g.augmentedreality[AR]andvirtualreality[VR])require high-reliabilityandultrahighcapacitywirelesscommunications.However, existingcommunicationsystems,designedandoptimizedbasedonconventionalcommunicationtheories,significantlypreventfurtherperformance improvementsfortheseservices.
● Wirelessnetworksarebecomingincreasinglyadhocanddecentralized,in whichmobiledevicesandsensorsarerequiredtomakeindependentactions suchaschannelselectionsandbasestationassociationstomeetthesystem’s requirements,e.g.energyefficiencyandthroughputmaximization.Nonetheless,thedynamicsanduncertaintyofthesystemspreventthemfromobtaining optimaldecisions.
● Anothercrucialcomponentoffuturenetworksystemsisnetworktrafficcontrol. Networkcontrolcandramaticallyimproveresourceusageandtheefficiency ofinformationtransmissionthroughmonitoring,checking,andcontrolling dataflows.Unfortunately,theproliferationofsmartIoTdevicesandultradense DeepReinforcementLearningforWirelessCommunicationsandNetworking: Theory,Applications,andImplementation,FirstEdition. DinhThaiHoang,NguyenVanHuynh,DiepN.Nguyen,EkramHossain,andDusitNiyato. ©2023TheInstituteofElectricalandElectronicsEngineers,Inc.Published2023byJohnWiley&Sons,Inc.
radionetworkshasgreatlyexpandedthenetworksizewithextremelydynamic topologies.Inaddition,theexplosivegrowingdatatrafficimposesconsiderable pressureonInternetmanagement.Asaresult,existingnetworkcontrol approachesmaynoteffectivelyhandlethesecomplexanddynamicnetworks.
● Mobileedgecomputing(MEC)hasbeenrecentlyproposedtoprovidecomputingandcachingcapabilitiesattheedgeofcellularnetworks.Inthisway,popularcontentscanbecachedatthenetworkedge,suchasbasestation,end-user devices,andgatewaystoavoidduplicatetransmissionsofthesamecontent, resultinginbetterenergyandspectrumusage[2,3].Onemajorchallengein futurecommunicationsystemsisthestragglingproblemsatbothedgenodes andwirelesslinks,whichcansignificantlyincreasethecomputationdelayof thesystem.Additionally,thehugedatademandsofmobileusersandthelimited storageandprocessingcapacitiesarecriticalissuesthatneedtobeaddressed.
Conventionalapproachestoaddressingthenewchallengesanddemandsof moderncommunicationsystemshaveseverallimitations.First,therapidgrowth inthenumberofdevices,theexpansionofnetworkscale,andthediversityof servicesintheneweraofcommunicationsareexpectedtosignificantlyincrease theamountofdatageneratedbyapplications,users,andnetworks[1].However, traditionalsolutionsmaybeunabletoprocessandutilizethisdataeffectivelyto improvesystemperformance.Second,existingalgorithmsarenotwell-suitedto handlethedynamicanduncertainnatureofnetworkenvironments,resultingin poorperformance[4].Finally,traditionaloptimizationsolutionsoftenrequire completeinformationaboutthesystemtobeeffective,butthisinformation maynotbereadilyavailableinpractice,limitingtheapplicabilityofthese approaches.Deepreinforcementlearning(DRL)hasthepotentialtoovercome theselimitationsandprovidepromisingsolutionstothesechallenges.
DRLleveragesthebenefitsofdeepneuralnetworks(DNNs),whichhaveproven effectiveintacklingcomplex,large-scaleengines,speechrecognition,medical diagnosis,andcomputervision.ThismakesDRLwellsuitedformanagingthe increasingcomplexityandscaleoffuturecommunicationnetworks.Additionally, DRL’sonlinedeploymentallowsittoeffectivelyhandlethedynamicsand unpredictablenatureofwirelesscommunicationenvironments.
1.2MachineLearningTechniquesandDevelopment ofDRL
1.2.1MachineLearning
Machinelearning(ML)isaproblem-solvingparadigmwhereamachine learnsaparticulartask(e.g.imageclassification,documenttextclassification, speechrecognition,medicaldiagnosis,robotcontrol,andresourceallocationin
Figure1.1 Adata-drivenMLarchitecture.
communicationnetworks)andperformancemetric(e.g.classificationaccuracy andperformanceloss)usingexperiencesordata[5].Thetaskgenerallyinvolves afunctionthatmapswell-definedinputstowell-definedoutputs.Theessenceof data-drivenMListhatthereisapatterninthetaskinputsandtheoutcomewhich cannotbepinneddownmathematically.Thus,thesolutiontothetask,which mayinvolvemakingadecisionorpredictinganoutput,cannotbeprogrammed explicitly.Ifthesetofrulesconnectingthetaskinputsandoutput(s)wereknown, aprogramcouldbewrittenbasedonthoserules(e.g.if-then-elsecodes)tosolve theproblem.Instead,anMLalgorithmlearnsfromtheinputdataset,which specifiesthecorrectoutputforagiveninput;thatis,anMLmethodwillresult inaprogramthatusesthedatasamplestosolvetheproblem.Adata-drivenML architecturefortheclassificationproblemisshowninFigure1.1.Thetraining moduleisresponsibleforoptimizingtheclassifierfromthetrainingdatasamples andprovidingtheclassificationmodulewithatrainedclassifier.Theclassificationmoduledeterminestheoutputbasedontheinputdata.Thetrainingand classificationmodulescanworkindependently.Thetrainingproceduregenerally takesalongtime.However,thetrainingmoduleisactivatedonlyperiodically. Also,thetrainingprocedurecanbeperformedinthebackground,whilethe classificationmoduleoperatesasusual.
TherearethreecategoriesofMLtechniques,includingsupervised,unsupervised,andreinforcementlearning.
● Supervisedlearning:Givenadataset D ={(x�� , y1 ), (x�� , y2 ), , (xn , yn )} ⊆ ℝn × ,asupervisedlearningalgorithmpredicts y thatgeneralizestheinput–output mappingin D toinputs x outside D.Here, ℝn isthe n-dimensionalfeature space , xi istheinputvectorofthe ithsample, yi isthelabelofthe ithsample, and isthelabelspace.Forbinaryclassificationproblems(e.g.spamfiltering), ={0,1} or ={−1,1}.Formulticlassclassification(e.g.faceclassification), ={1,2, , K }(K ≥ 2).Ontheotherhand,forregressionproblems(e.g. predictingtemperature), = ℝ.Thedatapoints (xi , yi ) aredrawnfroma (unknown)distribution (X , Y ).Thelearningprocessinvolveslearningafunction h suchthatforanewpair (x, y)∽ ,wehave h(x)= y withhighprobability (or h(x)≈ y).Alossfunction(orriskfunction),suchasthemeansquared
errorfunction,evaluatestheerrorbetweenthepredictedprobabilities/values returnedbythefunction h(xi ) andthelabels yi onthetrainingdata.
Forsupervisedlearning,thedataset D isusuallysplitintothreesubsets: DTR asthetrainingdata, DVA asthevalidationdata,and DTE asthetestdata.The function h(⋅) isvalidatedon DVA :ifthelossistoosignificant, h(⋅) willberevised basedon DTR andvalidatedagainon DVA .Thisprocesswillkeepgoingback andforthuntilitgivesalowlosson DVA .Thestandardsupervisedlearning techniquesincludethefollowing:Bayesianclassification,logisticregression, K -nearestneighbor(KNN),neuralnetwork(NN),supportvectormachine (SVM),decisiontree(DT)classification,andrecommendersystem.Notethat supervisedlearningtechniquesrequiretheavailabilityoflabeleddatasets.
● Unsupervisedlearning techniquesareusedtocreateaninternalrepresentation oftheinput,e.g.toformclusters,extractfeatures,reducedimensionality, estimatedensity.Unlikesupervisedlearning,thesetechniquescandealwith unlabeleddatasets.
● Reinforcementlearning(RL) techniquesdonotrequireapriordataset.WithRL, anagentlearnsfrominteractionswithanexternalenvironment.Theideaof learningbyinteractingwithadomainisanimitationofhumans’naturallearningprocess.Forexample,atthepointwhenanewbornchildplays,e.g.waves hisarmsorkicksaball,his/herbrainhasadirectsensorimotorconnectionwith itssurroundings.Repeatingthisprocessproducesessentialinformationabout theimpactofactions,causesandeffects,andwhattodotoreachthegoals.
Deeplearning(DL),asubsetofML,hasgainedpopularitythankstoitsDNN architecturestoovercomethelimitationsofML.DLmodelsareabletoextractthe keyfeaturesofdatawithoutrelyingonthedata’sstructure.The“deep”indeep learningreferstothenumberoflayersintheDNNarchitecture,withmorelayers leadingtoadeepernetwork.DLhasbeensuccessfullyappliedinvariousfields, includingfaceandvoicerecognition,texttranslation,andintelligentdriverassistancesystems.Ithasseveraladvantagesovertraditionalalgorithmsasfollows[6]:
● Noneedforsystemmodeling:Thesystemmustbewellmodeledintraditional optimizationapproachestoobtaintheoptimalsolution.Nevertheless,allinformationaboutthesystemmustbeavailabletoformulatetheoptimizationproblem.Inpractice,thismaynotbefeasible,especiallyinfuturewirelessnetworks whereusers’behaviorsandnetworkstatesarediverseandmayrandomlyoccur. Eveniftheoptimizationproblemiswelldefined,solvingitisusuallychallenging duetononconvexityandhigh-dimensionalproblems.DLcanefficientlyaddress alltheseissuesbyallowingustobedata-driven.Inparticular,itobtainstheoptimalsolutionbytrainingtheDNNwithsufficientdata.
● Supportsparallelanddistributedalgorithms:Inmanycomplexsystems,DLmay requirealargevolumeoflabeleddatatotrainitsDNNtoachievegoodtraining
performance.DLcanbeimplementedinparallelanddistributedtoaccelerate thetrainingprocess.Specifically,insteadoftrainingwithsinglecomputinghardware(e.g.graphicsprocessingunit[GPU]),wecansimultaneouslyleveragethe computingpowerofmultiplecomputers/systemsforthetrainingprocess.There aretwotypesofparallelisminDL:(i)modelparallelismand(ii)dataparallelism. Fortheformer,differentlayersinthedeeplearningmodelcanbetrainedin parallelonothercomputingdevices.Thelatterusesthesamemodelforevery executionunitbuttrainsthemodelwithdifferenttrainingsamples.
● Reusable:Thetrainedmodelcanbereusedinothersystems/problemseffectively withDL.Usingwell-trainedmodelsbuiltbyexpertscansignificantlyreduce thetrainingtimeandrelatedcosts.Forexample,AlexNetcanbereusedinnew recognitiontaskswithminimalconfigurations[6].Moreover,thetrainedmodel canbetransferredtoadifferentbutrelatedsystemtoimproveitstrainingusing thetransferlearningtechnique.Thetransferlearningtechniquecanobtaina goodtrainingaccuracyforthetargetsystemwithafewtrainingsamplesasit canleveragethegainedknowledgeinthesourcesystem.Thisisveryhelpfulas collectingtrainingsamplesiscostlyandrequireshumanintervention.
ThereareseveraltypesofDNNs,suchasartificialneuralnetworks(ANNs)(i.e. feed-forwardneuralnetworks),convolutionalneuralnetworks(CNNs),andrecurrentneuralnetworks(RNNs).However,theyconsistofthesamecomponents:(i) neurons,(ii)weights,(iii)biases,and(iv)functions.Typically,layersareinterconnectedvianodes(i.e.neurons)inaDNN.Eachneuronhasanactivationfunction tocomputetheoutputgiventheweightedinputs,i.e.synapsesandbias[7].During thetraining,neuralnetworkparametersareupdatedbycalculatingthegradient ofthelossfunction.
1.2.2ArtificialNeuralNetwork
AnANNisatypicalneuralnetworkknownasafeed-forwardneuralnetwork.In particular,ANNconsistsofnonlinearprocessinglayers,includinganinputlayer, severalhiddenlayers,andanoutputlayer,asillustratedinFigure1.2.Ahidden layerusestheoutputsofitspreviouslayerastheinput.Inotherwords,ANNpasses informationinonedirectionfromtheinputlayertotheoutputlayer.Ingeneral, ANNcanlearnanynonlinearfunction;thus,itisoftenreferredtoasauniversal functionapproximator.Theessentialcomponentofthisuniversalapproximation isactivationfunctions.Specifically,theseactivationfunctionsintroducenonlinear propertiestothenetworkandthushelpittolearncomplexrelationshipsbetween inputdataandtheiroutputs.Inpractice,therearethreemainactivationfunctions widelyadoptedinDLapplications:(i)sigmoid,(ii)tanh,and(iii)relu[6,8].Due toitseffectivenessandsimplicity,ANNisthemostpopularneuralnetworkused inDLapplications.
1.2.3ConvolutionalNeuralNetwork
AnothertypeofdeepneuralnetworkisCNN,designedmainlytohandleimage data.Todothat,CNNintroducesnewlayers,includingconvolution,RectifiedLinearunit(Relu),andpoolinglayers,asshowninFigure1.3.
● Convolutionlayer deploysasetofconvolutionalfilters,eachofwhichhandles certainfeaturesfromtheimages.
● Relulayer canmapnegativevaluestozeroandmaintainpositivevaluesduring training,andthusitenablesfasterandmoreeffectivetraining.
● Poolinglayer isdesignedtoreducethenumberofparametersthatthenetwork needstolearnbyperformingdown-samplingoperations.
ItisworthnotingthataCNNcancontaintensorhundredsoflayersdepending onthegivenproblem.Thefilterscanlearnsimplefeaturessuchasbrightnessand edgesandthenmovetocomplexpropertiesthatuniquelybelongtotheobject.In general,CNNperformsmuchbetterthanANNinhandlingimagedata.Themain reasonisthatCNNdoesnotneedtoconvertimagestoone-dimensionalvectors beforetrainingthemodel,whichincreasesthenumberoftrainableparameters andcannotcapturethespatialfeaturesofimages.Incontrast,CNNusesconvolutionallayerstolearnthefeaturesofimagesdirectly.Asaresult,itcaneffectively
learnallthefeaturesofinputimages.Intheareaofwirelesscommunications, CNNisapromisingtechniquetohandlenetworkdataintheformofimages,e.g. spectrumanalysis[9–11],modulationclassification[12,13],andwirelesschannel featureextraction[14].
1.2.4RecurrentNeuralNetwork
AnRNNisaDLnetworkstructurethatleveragespreviousinformationtoimprove thelearningprocessforthecurrentandfutureinputdata.Todothat,RNNis equippedwithloopsandhiddenstates.AsillustratedinFigure1.4,byusingthe loops,RNNcanstorepreviousinformationinthehiddenstateandoperatein sequence.Inparticular,theoutputoftheRNNcellattime t 1willbestoredin thehiddenstate ht andwillbeusedtoimprovethetrainingprocessoftheinputat time t.ThisuniquepropertymakesRNNsuitablefordealingwithsequentialdata suchasnaturallanguageprocessingandvideoanalysis.
Inpractice,RNNmaynotperformwellwithlearninglong-termdependencies asitcanencounterthe“vanishing”or“exploding”gradientproblemcausedbythe backpropagationoperation.Longshort-termmemory(LSTM)isproposedtodeal withthisissue.AsillustratedinFigure1.5,LSTMusesadditionalgatestodecide