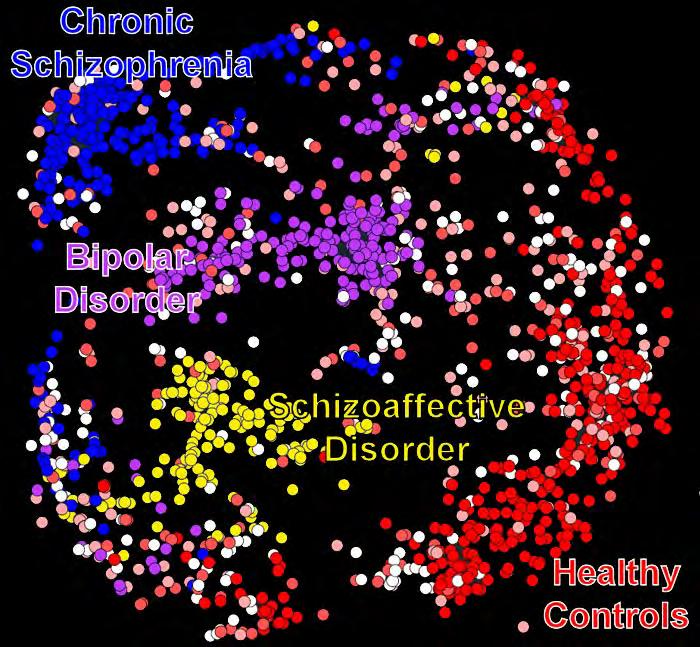
6 minute read
THE PROMISE OF IMAGING FOR
from CerebrumWinter2021
Knowledge gleaned from big data and advances in neuroimaging have provided new insights into the workings of the brain. OUR AUTHOR, founding director of the Center for Translational Research in Neuroimaging and Data Science and a Georgia Research Alliance eminent scholar in brain health and image analysis, traces the evolution of these two evolving fields and how it may have an impact on mental health in the not-too-distant future.
BY VINCE C ALHOUN, Ph
Advertisement
Mental Health
H E Grebztr
Leinadyb
Ental And Neurodegenerative Disorders Are Among
the most costly and common causes of disability in society today. Because the brain is the most complex organ in the human body, diagnosing and treating problems when things go wrong poses enormous challenges. Even before the 1990s was designated the Decade of the Brain, the potential of neuroimaging—the technology that makes it possible to see inside the working brain—was a major focus in psychiatry. Since that time, expectations have been high that neuroimaging would move the needle forward in unraveling the mystery of mental illness.
Living in a time of such national programs as the BRAIN Initiative and Human Connectome Project, we’ve become accustomed to hearing terms such as artificial intelligence, virtual reality, and brain-machine interface. In light of the exponential increase in computational and algorithmic power, one can only assume that we have made great progress via psychiatric neuroimaging. But just how far have we actually come in the last half-century? How close are we to having neuroimaging-based tools that can be used in the clinic? Have we learned anything about diagnosis or mental illness from the vast trove of neuroimaging data that has been collected over the years? I wish I could point to specific examples where the widening use of neuroimaging is beginning to help the mentally ill, but we are just not there yet.
As an undergraduate electrical engineering student at the University of Kansas in 1991, I became enthralled with magnetic resonance imaging (MRI), which makes it possible to see inside the body, noninvasively and safely, using magnetic fields and radio waves. This was the beginning of a career in brain imaging that led me to spend 12 years in a psychiatry department at Johns Hopkins where, I like to joke, I was an engineer “studying the psychiatrists.” In reality, it was a fascinating experience, as I learned the complexity of the problems under study, saw the potential for applying engineering and signal processing principles, and gravitated to the emerging specialty of psychiatric neuroimaging.
It didn’t take me long to realize that the study of psychiatric disorders is extremely difficult, in part, since the assessment of the individual is mainly based on their medical history and symptoms they are experiencing at that moment. And while these assessments can be reliable up to a point, they largely lack biological validity. If someone is feeling depressed, for example, they may be diagnosed with depression. If, however, a few months later they are experiencing hallucinations or their thoughts are confused, the diagnosis is likely to be schizophrenia or bipolar disorder. There are no objective tests to confirm or rule out any of these disorders. Nor are there cures, although existing treatments may mitigate symptoms. But even determining appropriate treatment can be a formidable challenge, often requiring sequential effort to determine, and the wrong treatment can muddle diagnosis and, in some cases, the wrong medication can make a condition worse.
The Promise of Magnetic Imaging
MRI has been used to visualize the size (i.e., volume) of various brain structures for over 40 years now. And for almost 30 years we have been able to see fluctuations in brain activity using a technique called functional MRI (fMRI), which tracks magnetic signals that reflect changes in blood flow and oxygenation level associated with neuronal activity. The discovery of fMRI raised hopes for a clinical breakthrough in assessment and treatment. Beyond the challenges that lay before them, the psychiatric research community saw the potential that neuroimaging held for helping patients whose lives were disrupted by a malfunctioning brain.
We believed we were on the verge of better understanding how the brain worked, and developing tools and treatments to address what are now understood to be brain disorders, including depression, schizophrenia, bipolar disorder, and attention-deficit/hyperactivity disorder. Using neuroimaging to identify disrupted brain regions could provide information useful to develop and evaluate new treatments, enable prediction of individual response to such treatments, and help subtype individuals (e.g., schizophrenia and schizoaffective disorder). The obstacles, however, turned out to be more formidable, and progress slower than we would have liked. Our challenges and successes might be best understood through the evolution of five different eras.
Depth 1 Depth 2
FIGURE 1: The above figure shows the potential benefits of more powerful analysis of neuroimaging data. Each dot represents an individual’s brain data, and the dots are color-coded by their diagnosis. The model flexibility increases with increasing depth, and it is clear that this model is better able to separate the disorders with the greatest depth (depth 3, largest panel). Two conclusions we can draw from this are: 1) more complex models separate diagnoses better and highlight the information contained in neuroimaging data, 2) the relationship between diagnoses is complex and there is still a lot of overlap between them. The latter is likely due to imprecision in the diagnostic categories as well as individual differences.
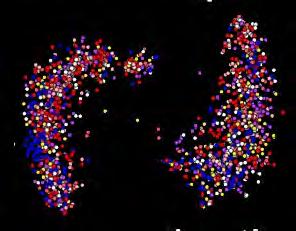
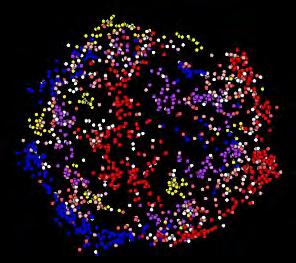
FIGURE 2: The above figure shows us how different brain networks are functioning together. The lines measure the strength of communication among various networks and represent a unique “fingerprint” of our brains. By using such displays, we can evaluate what is called the human functional connectome—and also how mental illness is disrupted.
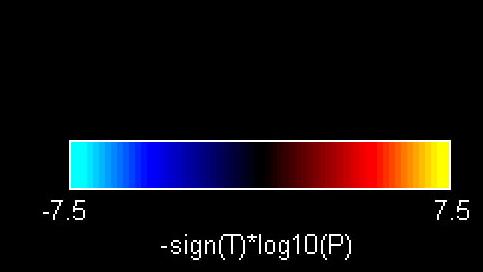
The “Small N” Era
In the early days of neuroimaging studies, we used a small number of subjects (called “small Ns”). In this era, even though structural and functional MRI studies were initially quite small (e.g., using a "small N" of only 5 to 20 subjects), each promised a potentially revolutionary finding. Initial functional studies had individuals perform a number of carefully designed tasks and tracked how the brain responded to these tasks. But while many studies highlighted specific deficits associated with various mental disorders, differences tended to be relatively small and most studies lacked sufficient controls for the many possible confounds, such as medication or patient movement within the scanner.
Studies that followed often yielded promising findings that pointed toward various brain regions that appeared to be important contributors to brain disorders or symptoms. Many of these findings turned out to be hard to replicate. In some cases, the tasks were difficult for those impacted by mental illness to perform, which made it hard to know if the observed changes were due to the disorder itself or were different simply because the tasks were not being performed. So, the small N problem became a large problem, compounded by the heterogeneity of mental disorders.
To better understand what researchers faced, consider the development of a vaccine for Covid-19, where 30,000 individuals are required for a study. Testing the efficacy and safety of a vaccine is a much simpler problem than treating depression, for example, since the outcome measures are clear (someone gets ill or not). With psychiatric disorders, by contrast, we are simultaneously trying to clarify the diagnosis and understand how the brain is impacted—which regions and what mechanisms are involved.
Despite the challenges, we learned much about how to model neuroimaging data during the small N era, including how to efficiently administer tasks, control for statistical complexities, and compare data. The “dead salmon” paper, which was presented at the Human Brain Mapping conference in 2009, light-heartedly highlighted the already well-known statistical corrections that are needed when studying brain images. The presenters were making an important scientific point regarding the “multiple comparisons problem.” If one does a lot of different statistical tests, some of them will, just by chance, give interesting results. With this, we gained important information about how mental illness impacts the brain. But this was not enough.
The “Large Group” Era
In the next phase of neuroimaging (referred to as the “large group” era), researchers focused on increasing group sizes (typically to hundreds of individuals or more) to increase confidence that detected effects were not just due to noise and to better characterize the heterogeneity of psychiatric disorders. During this era, we learned with greater confidence which brain regions were activated by tasks, as well as the degree to which regional responses differed in those with mental illness (e.g., auditory oddball or working memory task deficits in schizophrenia).
Likewise, we learned more about how brain structure was impacted by disease (e.g., individuals with schizophrenia consistently show reduced temporal lobe and medial frontal gray matter). Approaches based on meta-analysis offered tools to pool results from many small studies, in order to provide more reliable statistical summaries. During this era, the focus was still very much on isolating specific brain regions, rather than considering the brain as a highly interconnected system.
The “Network” Era
A major shift occurred when our attention turned to brain networks, both at rest and during task performance (referred to as the “network” era). The mathematical tools used to study networks can also be applied to study networks between brain regions. The same approaches Google and Facebook use to leverage the concept of networks to improve search engines and social interactions began to be applied to the brain, which can be thought of as a network of networks. The idea was to identify specific networks of brain regions that are linked to, or correlated with, one another. Among other advantages, this approach enabled us to study the brain even when individuals were resting, rather than performing specified tasks.
By mitigating the formidable challenge of ensuring that everyone was performing the same task the same way, the approach allowed researchers to scale up to much larger numbers, combining data across many imaging centers. In