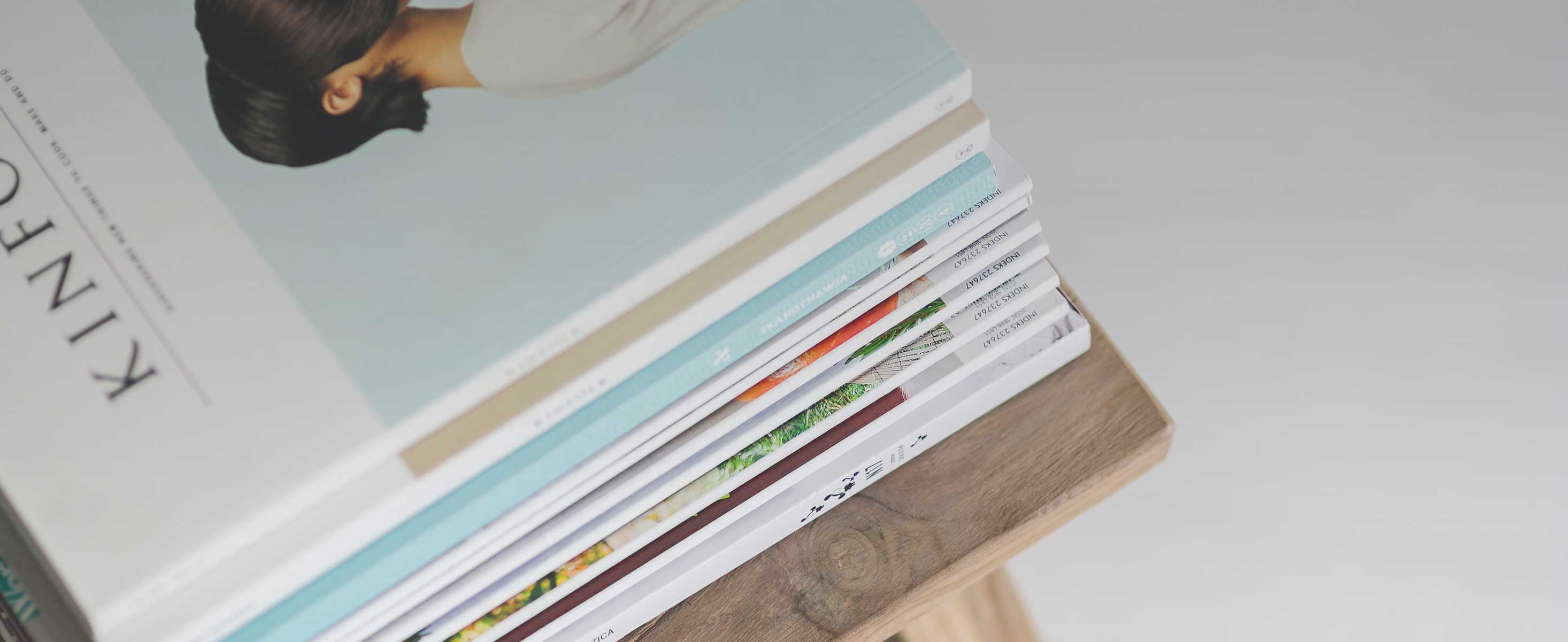
5 minute read
The same approaches Google and Facebook use to
from CerebrumWinter2021
predict response to antidepressants versus mood stabilizers in adolescents with mood disorders).
addition, fancy new analytical tools that can assess brain activity coming from many regions at once (e.g., multivariate methods and techniques based on graph theory), similar to those used by many software engineering companies, were introduced and are now being used with increasing regularity. But although these approaches have taught us much about how psychiatric disorders impact brain connectivity, they have not yet led to clinical tools. For that we need more precise and individualized information.
Advertisement
The “Prediction” Era
The vast majority of brain imaging researchers focus on describing central tendencies and group results, rather than findings with individual subjects, and the case studies that are reported typically concentrate on explaining the data at hand rather than predicting unseen data from an individual (i.e., Can I use brain imaging to predict a future diagnosis, or to determine if that individual will respond well to a certain medication?). While this may seem like a small distinction, it is quite critical, as the results for these two approaches (studying averages versus studying individuals) often differ. A focus on individual level prediction and forecasting of future trajectories relevant to an individual person is arguably the most important goal if brain imaging is to translate into practical solutions to improve the quality of life and enhance technological development.
Prediction studies typically utilize advanced computational approaches and algorithms that can learn from data (i.e., machine learning). The field has experienced a large growth in studies using machine-learning approaches to make individualized predictions (i.e., informed guesses) of symptoms, cognitive scores, medication response information, and more. Studies of brain function and structure that focus on these estimates have revealed whole brain patterns that show potential to be able to predict and identify mental disorders and to predict treatment response (e.g., using resting fMRI to
Beyond these patterns, they also appear to be predictive of risk for psychiatric disorders. However, we are still only scratching the surface in the prediction era, as these approaches tend to require large amounts of data, and algorithms are still not able to learn well without a good “ground truth” (i.e., If we already know the answer it is not hard to train a computer to recognize it, but with mental illness we are still unsure about even the diagnostic categories). Existing techniques with the data we have are still unable to tell us clearly whether the psychiatric diagnoses accurately reflect the underlying disorder. Thankfully, algorithms are getting smarter, and more data are arriving all the time.
The Era of Big Data
We are now firmly in the era of big data for neuroimaging and psychiatry. The number of large, shared data sets has dramatically increased over the past few years. Several studies, e.g., Aging Brain Cognition and Development and UK Biobank, are scanning tens of thousands of individuals over time (although these are mostly individuals without psychiatric problems, but the assessed measures can be used to study psychiatric issues as a spectrum).
There is interest in considering mental illness as manifested by otherwise everyday human traits that lie outside typical ranges of behavior and process. For example, someone may be anxious about an upcoming deadline, but when anxiety becomes constant and independent of circumstances, it impacts our quality of life and might be considered a psychiatric disorder.
To study mental illness requires vast amounts of data and flexible models that can handle all its complexity. A promising class of powerful models (deep learning models), like those that were used to beat international experts in the game of Go, or that Alexa uses to recognize your commands, have been shown to be very powerful. But they also require a lot of data. The application of deep learning (deep artificial neural networks) to neuroimaging has shown great promise and will likely be a major force in advancing our knowledge and understanding of the data. These approaches require considerable computational resources as the complexity of models and the amount of data continue to grow.
Challenges, Testing, and Discovery
Given that the criteria used to decide who has a mental disorder are largely based on self-reported symptoms and not biologically based, we have a wicked chicken-and-egg problem. Do predictions of mental disorder based on nonbiological characterizations provide useful and actionable information?” The fact is that the brain is incredibly complex; psychiatric illness is complex and multifaceted, including an overlap among existing diagnosis and prediction models that are relatively simple. This creates a perfect storm of difficulty for computational approaches and can lead to a “garbage in—garbage out” problem: We are trying to predict something that is not well defined in the first place. At the same time, the dual challenge of unclear diagnostic categories and the desire to make predictions presents an opportunity.
The use of deep neural network models offers the flexibility to capture relationships that are not yet well understood, including dynamic changes and multimodal contributions. The accelerating pace of algorithmic innovation can only expand this potential, providing tools for 1) predicting existing diagnostic categories, 2) identifying new categories, and 3) combining the two, all based on biological data. The use of hypothesis-based and data-discovery approaches should work together in a “virtuous cycle” in which we learn from new data, update our models accordingly, and learn more by applying these models for finer-tuned analysis.
In many ways, this evolution parallels the development of big data genomics models that have highlighted the polygenic nature of psychiatric disorders by scaling up to deal with extremely large studies. Early larger scale imaging studies also suggest a “polyregional” brain disruption, impacting brain connectivity at a systemic or network level. However, the neuroimaging field has some additional challenges to confront, while also presenting some unique advantages.
Neuroimaging studies require brain scans. Extremely large genomics studies are more easily extended to hundreds of thousands of data sets since these only require saliva or in some cases a blood draw. In contrast, neuroimaging provides greater potential for characterization of mental disorders due to its ability to capture changes over time, as well as a valuable tool for studies focused on intervention or brain stimulation. In addition, neuroimaging changes related to mental disorders tend to be larger and more detectable than those based on genomic studies of these highly polygenic disorders. While it remains to be seen what place neuroimaging and genomics will ultimately occupy in clinical decision-making, it does seem clear that they will be part of the landscape.
Where to Now?
While we have learned a lot, we still have a long way to go before we can fully leverage neuroimaging data to understand psychiatric disorders and help people address their mental health difficulties. In the near-term, predicting medication response is a promising goal, as this bypasses diagnostic criteria in identifying the best treatment for an individual. For example, one might use fMRI to predict the response to antidepressants versus mood stabilizers or to determine which antidepressant is likely to have the best effect. While this is only a small step, it does have promise as a support to decision-making in the clinic.
Another key question will be how to benefit from large amounts of data while protecting privacy. New data management infrastructure (i.e., neuroinformatics) to support the use of neuroimaging will need to incorporate the ability to safeguard sensitive information. Decentralized or federated approaches may provide a way forward here, combining intermittent neuroimaging with the regular capture of information from a mobile device in a way that preserves privacy and which, for example, has considerable potential to treat disorders such as depression or cognitive decline by detecting mental health patterns. A recent example of this is reflected in some of the Covid-19 tracing apps which are designed with privacy in mind.
While we have not advanced as quickly as we might have hoped in explicating the mysteries of mental illness, there is considerable reason to be optimistic about the not-so-distant future. The brain is an amazing organ, but its complexity, individuality, and role in shaping who we are is also the reason the problems are so challenging. As the author and scholar C.S. Lewis once said: “There are no ordinary people.” Despite such challenges, I remain firmly hopeful and believe we are moving towards the needed solutions. l