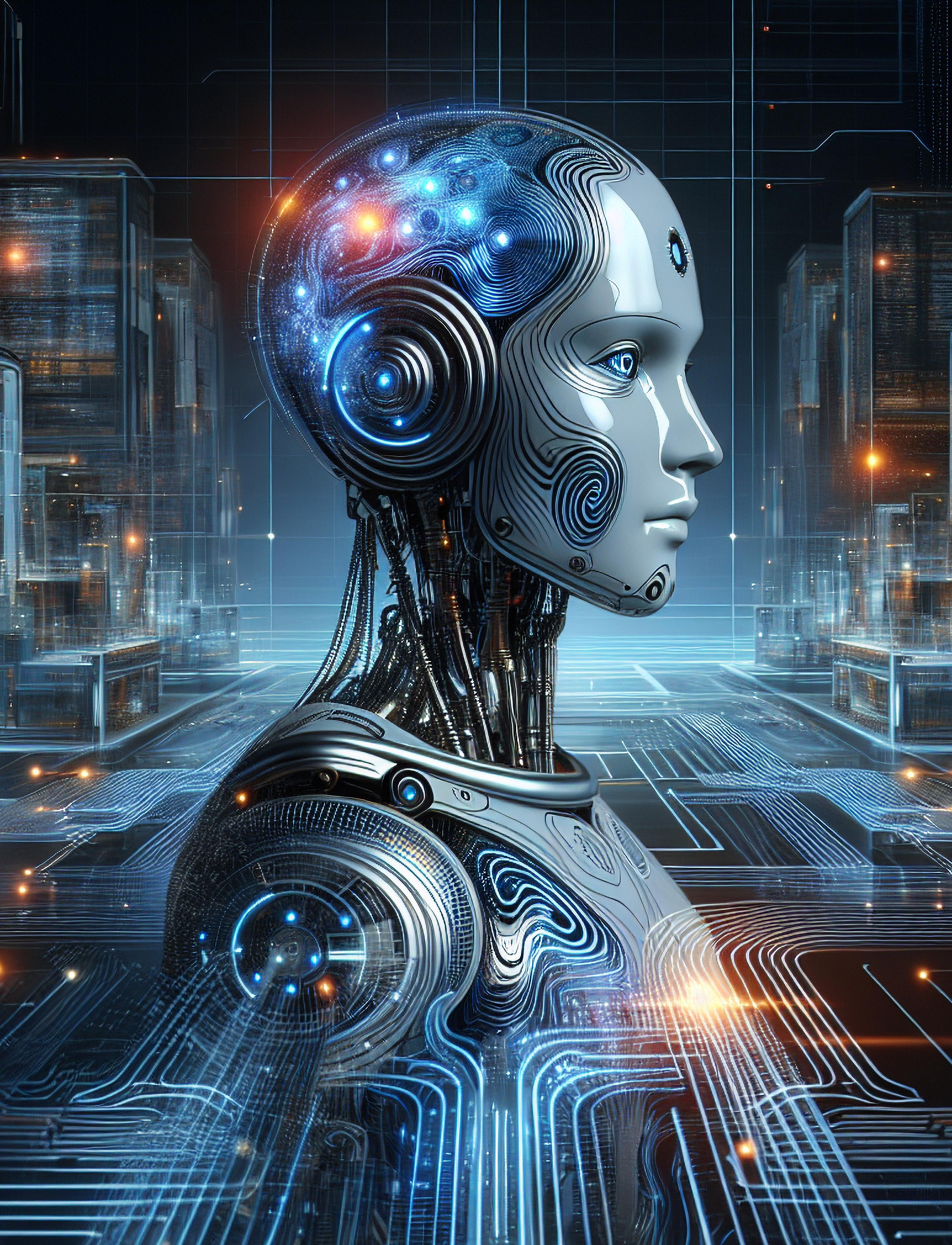
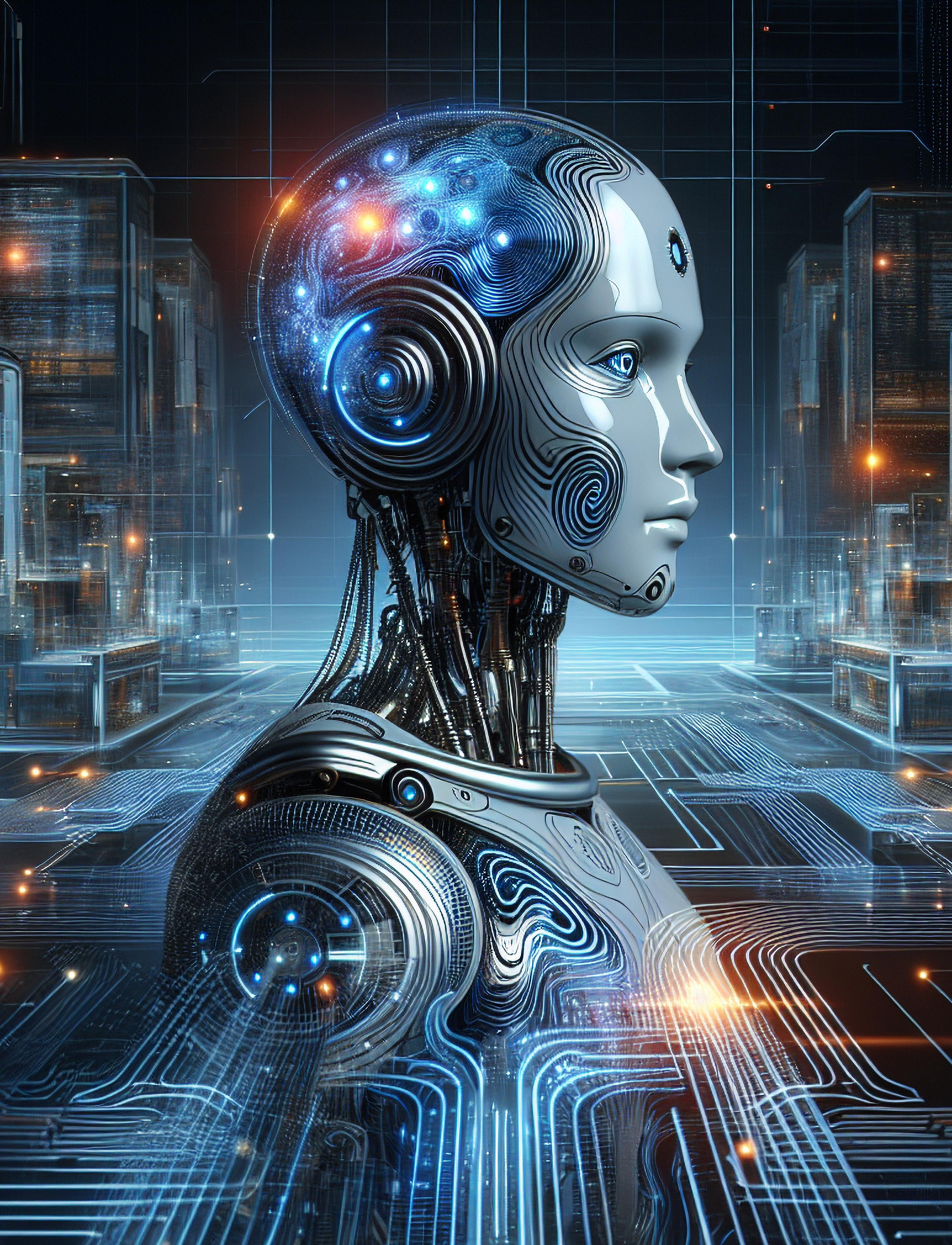
BlueVerve
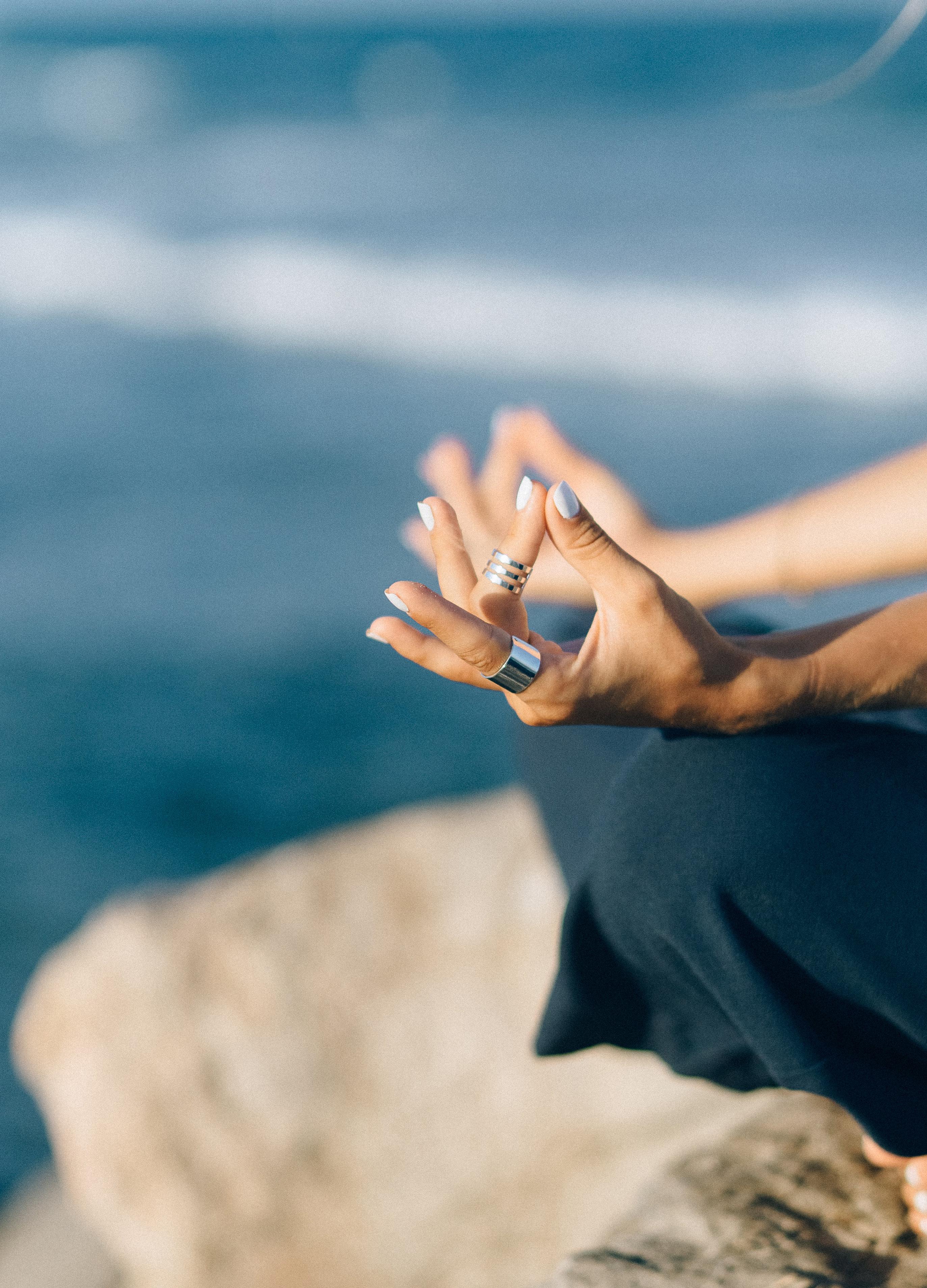
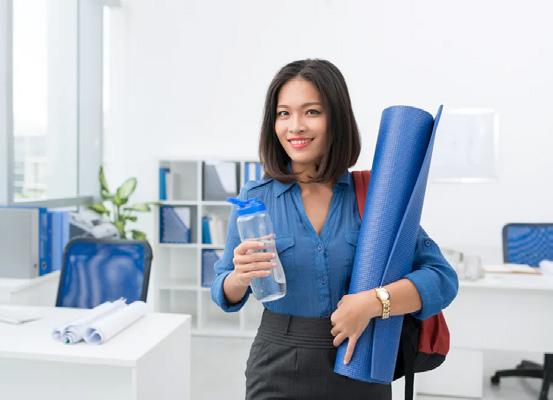
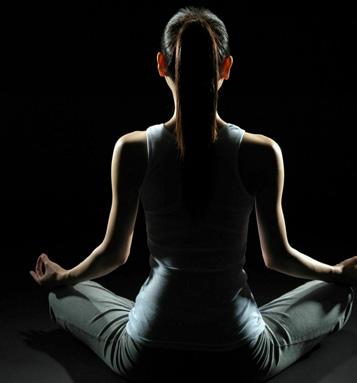
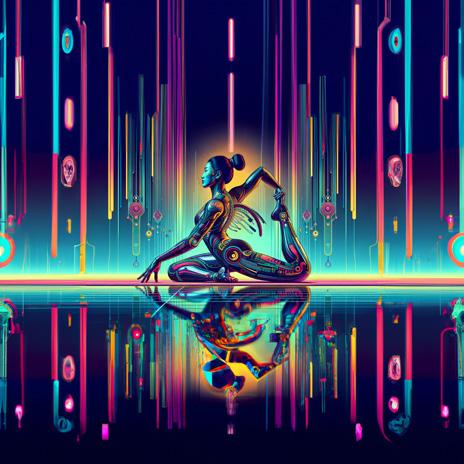
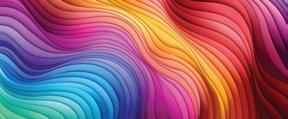
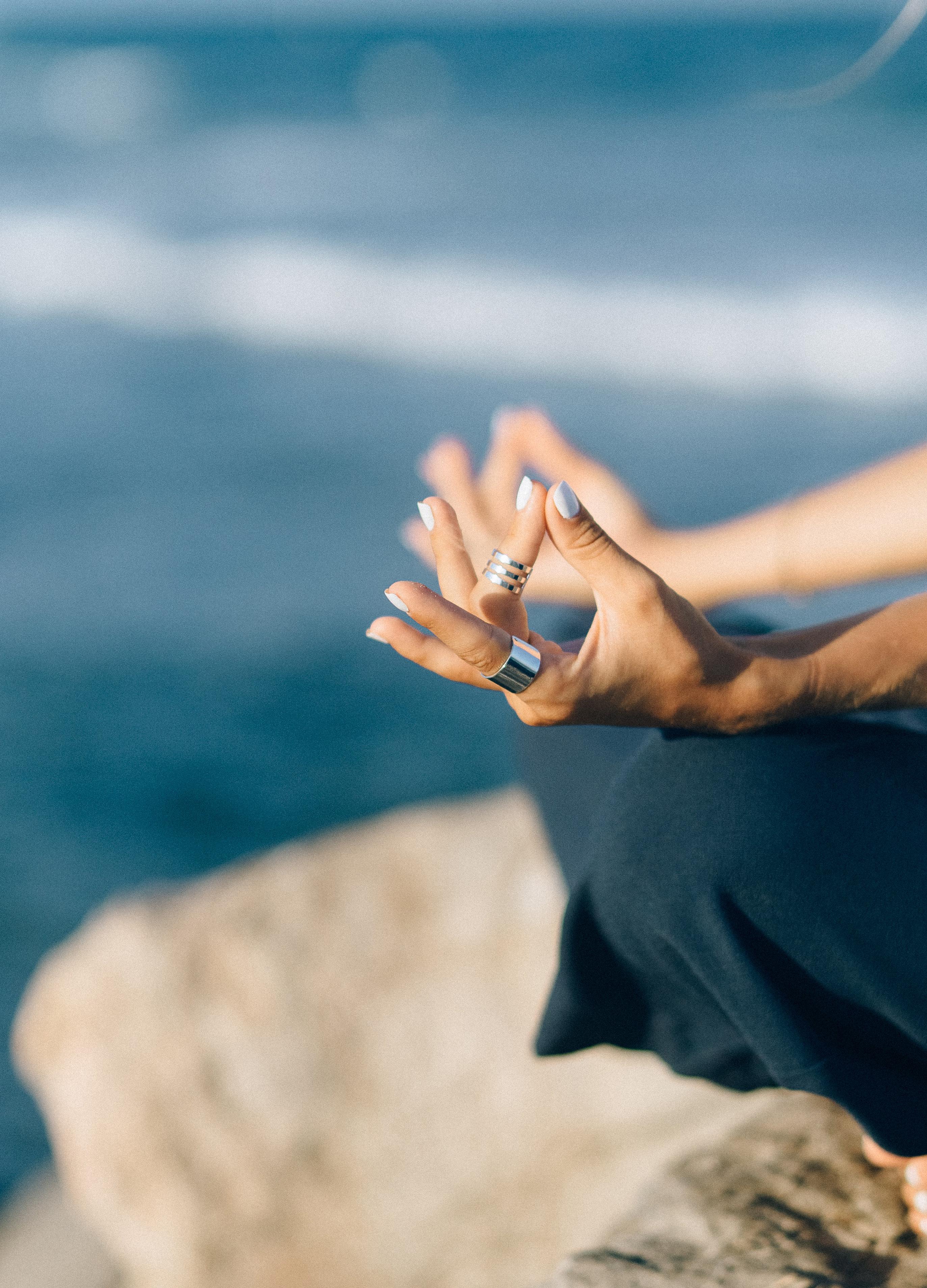
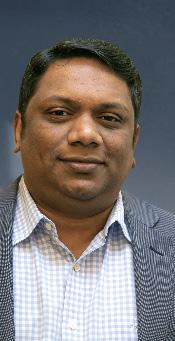
The rise of agents
Artificial intelligence is entering a new era with the rise of agentic AI, a groundbreaking advancement that enables AI systems to autonomously solve complex, multi-step problems through sophisticated reasoning and iterative planning. Unlike traditional AI, which primarily relies on predefined instructions, agentic AI can make decisions, adapt to changing environments, and optimize processes in real-time. This evolution is set to revolutionize productivity and operations across industries.
How will agentic AI change the way we work? It has the potential to redefine workflows and decision-making by automating strategic problem-solving. It allows businesses to move beyond simple automation, empowering AI agents to analyze challenges, develop solutions, and take independent action. This shift will free up human talent to focus on innovation and high-value work while AI handles routine and time-intensive tasks.
Now, you might wonder—isn’t this what RPA promises? Traditional RPA, however, depends on pre-defined scripts that excel in handling predictable processes but struggle when faced with changing variables. In contrast, Agentic AI adapts dynamically in real time.
This raises an important question—what do enterprises need to achieve this level of AI autonomy?
To fully leverage agentic AI, organizations must establish a strong foundation, beginning with a well-structured data infrastructure. Highquality, real-time data is essential for AI systems to make intelligent, autonomous decisions. This requires seamless cloud integration to ensure data is processed efficiently and securely across distributed environments. Enterprises must also invest in scalable computing resources, allowing AI to analyze vast datasets and respond dynamically to new information.
The applications of agentic AI are limited only by imagination. From generating content and managing enterprise workflows to orchestrating entire digital ecosystems, agentic AI is poised to become an indispensable tool across industries. As companies continue to innovate and refine AI capabilities, we can expect a future where AI not only supports human decision-making but actively drives business success.
Embracing agentic AI today means staying ahead in an increasingly automated world. The question is no longer if AI will transform industries—but how quickly businesses can adapt to its game-changing potential.
JeevanThankappan
JEEVAN THANKAPPAN
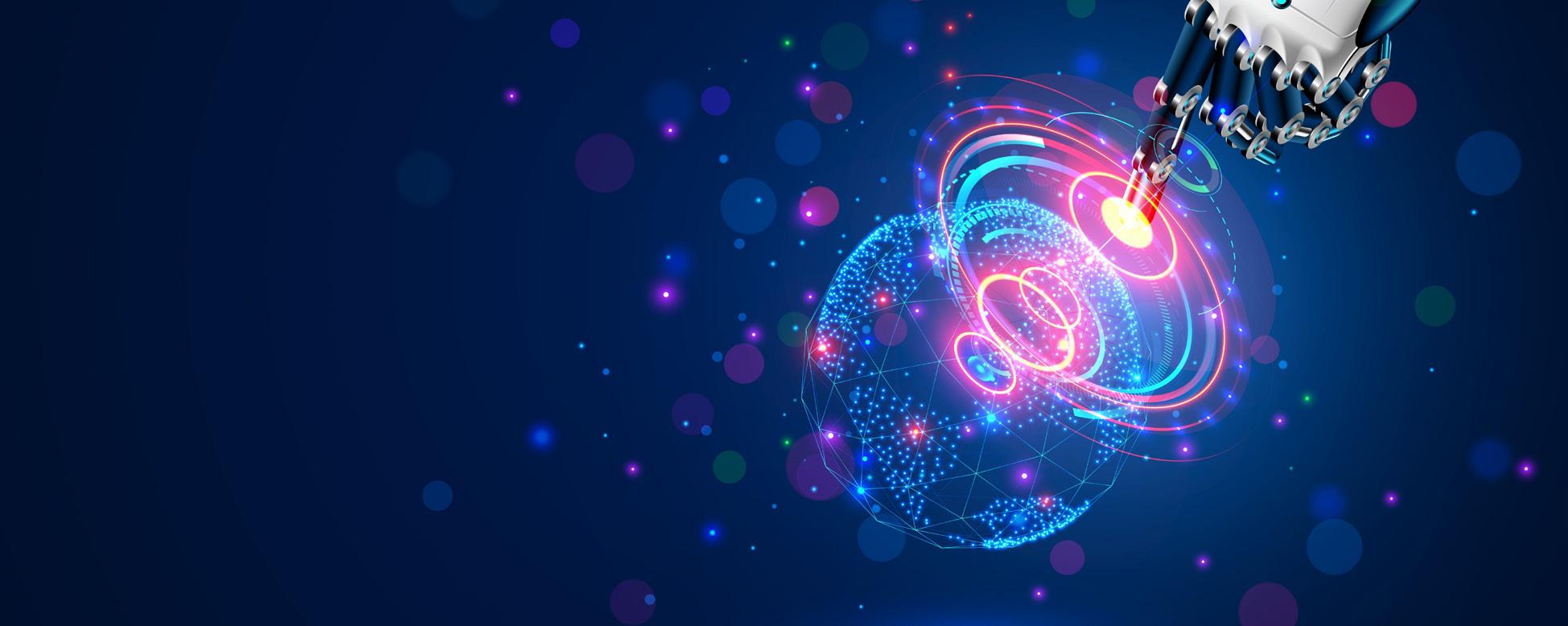
12 Building the future.
Ihab Tarazi, CTO and Sr. VP at Dell Technologies Infrastructure Solutions Group, discusses how the company is powering the next wave of AI innovation.
14 Global ambitions
Thomas Pramotedham, CEO of Presight, explains how the homegrown company is exporting Abu Dhabi’s AI expertise.
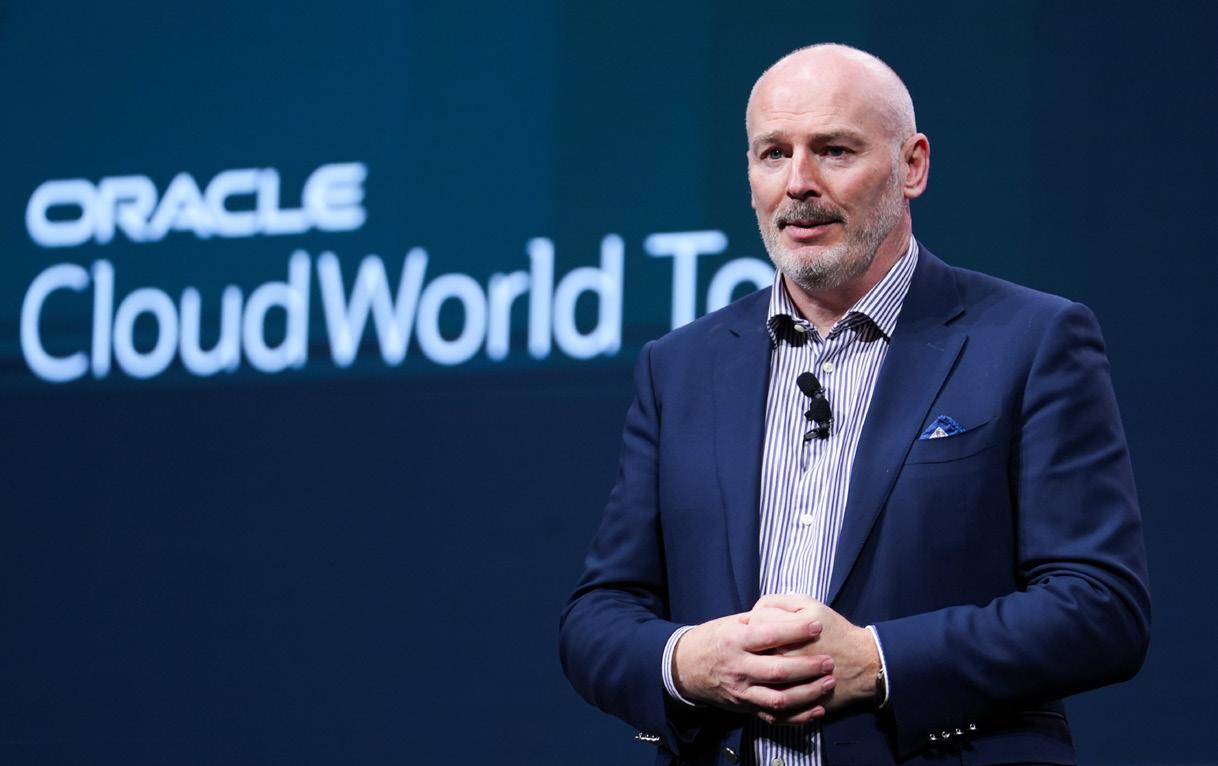
Navigating the future of cloud and AI.
An exclusive interview with Cormac Watters, EVP – Business Applications, EMEA at Oracle.
The path to responsible AI. Lee McLean, Legal Counsel and AI Specialist, Zebra Technologies, on how can we define Responsible Ai and turn
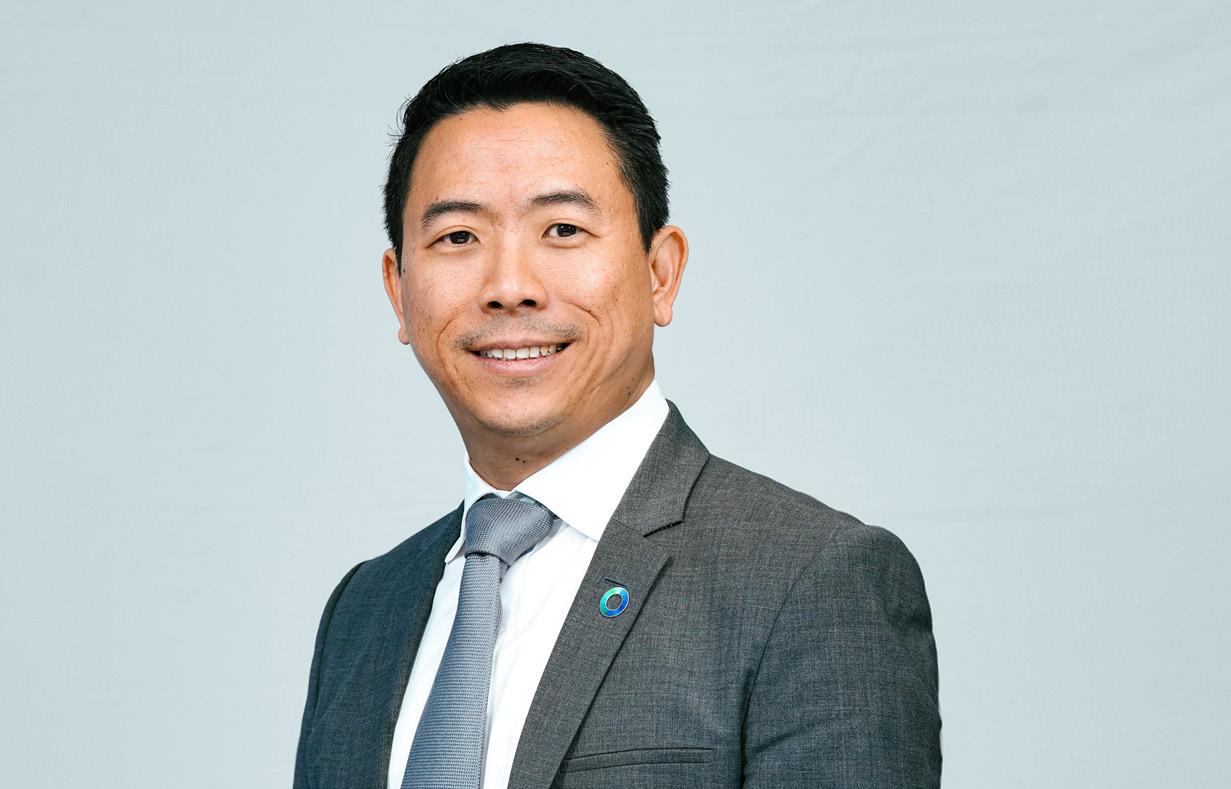
26 AI showdown.
An in-depth analysis of four leading AI tools in 2025, focusing on their architectures, performance, use cases, and business implications.
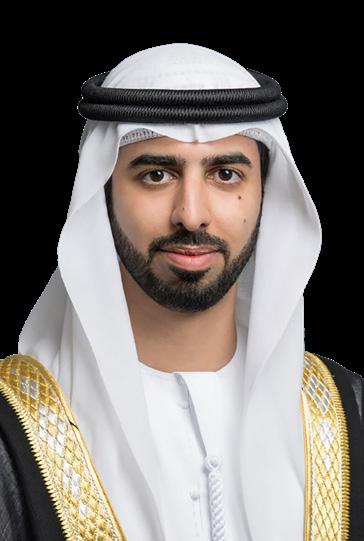
42
How the UAE is leading the AI revolution.
Excerpts from a speech delivered by H.E. Omar Sultan Al Olama, UAE’s Minister of State for Artificial Intelligence, at Oracle CloudWorld.
NORTH STAR COUNCIL
Our North Star Council serves as the editorial guiding light of the AI Times, providing strategic direction and ensuring our content remains on the cutting edge of AI innovation.
Our Members
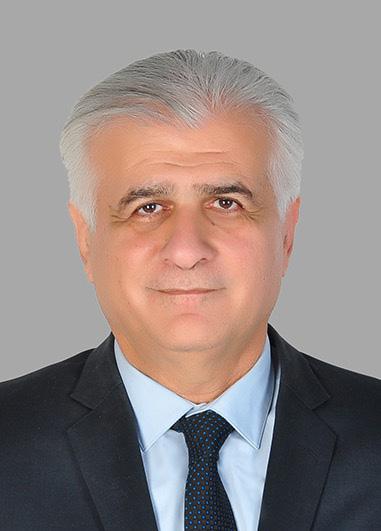
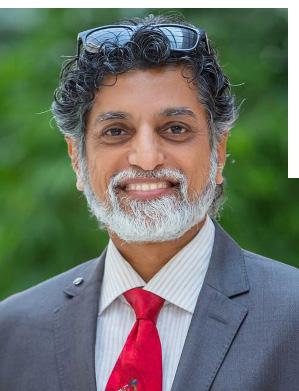
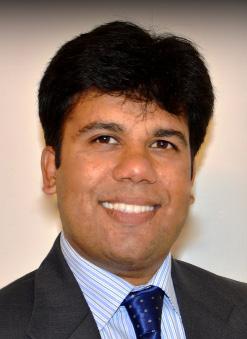
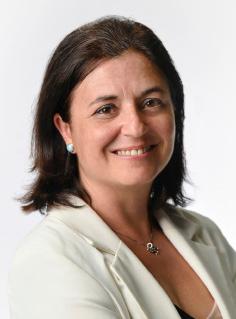
Dr. Jassim Haji President of the International Group of Artificial Intelligence
Venkatesh Mahadevan Founding Board Member CAAS
Jayanth N Kolla Founder & Partner Convergence Catalyst
Idoia Salazar Founder & President OdiseIA
If you would like to be a part of our North Star Council, please reach out to us at jeevan@gecmediagroup.com
PUBLISHER TUSHAR SAHOO tushar@gecmediagroup.com
CO-FOUNDER & CEO RONAK SAMANTARAY ronak@gecmediagroup.com
MANAGING EDITOR Jeevan Thankappan jeevan@gcemediagroup.com
ASSISTANT EDITOR SEHRISH TARIQ sehrish@gcemediagroup.com
GLOBAL HEAD, CONTENT AND STRATEGIC ALLIANCES ANUSHREE DIXIT anushree@gecmediagroup.com
CHIEF COMMERCIAL OFFICER RICHA S richa@gecmediagroup.com
PROJECT LEAD JENNEFER LORRAINE MENDOZA jennefer@gecmediagroup.com
SALES AND ADVERTISING sales@gecmediagroup.com
Content Writer
KUMARI AMBIKA
IT MANAGER VIJAY BAKSHI
DESIGN TEAM CREATIVE LEAD AJAY ARYA
SR. DESIGNER SHADAB KHAN DESIGNERS
JITESH KUMAR, SEJAL SHUKLA
PRODUCTION
RITURAJ SAMANTARAY
S.M. MUZAMIL
PRODUCTION CIRCULATION, SUBSCRIPTIONS info@gecmediagroup.com
DESIGNED BY

SUBSCRIPTIONS info@gecmediagroup.com
PRINTED BY Al Ghurair Printing & Publishing LLC. Masafi Compound, Satwa, P.O.Box: 5613, Dubai, UAE
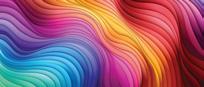
(UAE) Office No #115 First Floor , G2 Building Dubai Production City Dubai, United Arab Emirates Phone : +971 4 564 8684
(USA) 31 FOXTAIL LAN, MONMOUTH JUNCTION, NJ - 08852 UNITED STATES OF AMERICA Phone :+ 1 732 794 5918
Globant partners with Red Sea Global
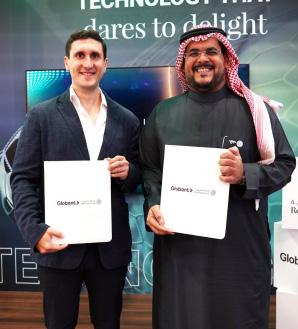
Globant has partnered with Red Sea Global (RSG) to create a cutting-edge
digital program designed to transform the connected visitor experience at one of Saudi Arabia’s most ambitious tourism destinations.
The collaboration leverages Globant’s expertise in digital innovation to deliver a seamless, immersive, and personalized journey for every visitor to Red Sea Global’s world-class destination, The Red Sea. This initiative aligns with Saudi Arabia’s Vision 2030 strategy, showcasing the kingdom’s commitment to sustainable and technologically advanced tourism.
Sultan Moraished, Group Head of Technology and Corporate Excellence of Red Sea Global, noted, “The Red Sea destination represents a bold vision for the future of tourism, one that combines luxury, technology, and sustainability in perfect harmony. With Globant as our partner, we are taking an important step toward delivering a connected experience that will serve as a benchmark not just for the region but for destinations around the world”.
The program centers around a robust, digitally enabled ecosystem that integrates advanced technologies such as artificial intelligence, IoT, and data analytics. This connected visitor experience will provide intuitive, real-time interactions tailored to individual preferences, ensuring a truly memorable stay at The Red Sea’s luxury resorts, islands, and attractions.
LEAP 2025 kicks off with record $14.9B AI investment announcement
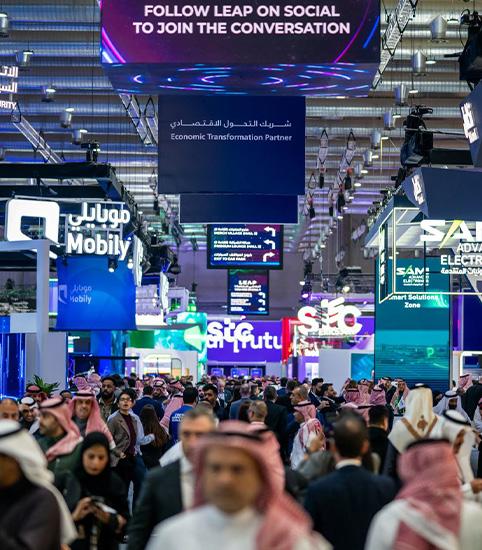
LEAP 2025 has announced a record-breaking US$14.9 billion in new Artificial Intelligence (AI) investments that further cement the Kingdom’s status as a worldleading AI hub.
Revealed on the opening day of this year’s four-day event, the new announcements increase the total amount of technologyrelated infrastructure investments in Saudi Arabia to more than US$42.4bn since LEAP debuted in 2022.
The new investments in the Kingdom included an announcement between
Groq and Aramco Digital confirming a US$1.5bn plan to expand AI-powered inference infrastructure and cloud computing; ALAT and Lenovo committing US$2bn to establish an advanced manufacturing and technology centre integrating AI and robotics; Google introducing new AI-driven digital infrastructure and the launch of a powerful computing cluster to meet regional and global demand; Qualcomm confirming the availability of its ALLAM language model on Qualcomm AI Cloud.
New NTT DATA Report Exposes the AI Responsibility Crisis
New research from NTT DATA reveals that businesses are racing to adopt AI, yet a responsibility gap threatens to undermine progress. More than 80% of executives acknowledge that leadership, governance, and workforce readiness are failing to keep pace with AI advancements—putting investment, security, and public trust at risk.
The report, The AI Responsibility Gap: Why Leadership is the Missing Link, draws insight from more than 2,300 C-suite leaders and decision-makers across 34 countries, uncovering the urgent need for a leadershipdriven mandate to align AI innovation with ethical responsibility.
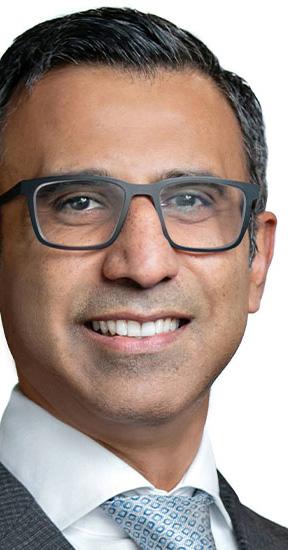
“The enthusiasm for AI is undeniable, but our findings show that innovation without responsibility is a risk multiplier,” said Abhijit Dubey, Chief Executive Officer, NTT DATA, Inc. “Organizations need leadership-driven AI governance strategies to close this gap— before progress stalls and trust erodes.”
The widening AI responsibility gap is becoming a critical challenge for businesses, as executives struggle to balance innovation with ethical responsibility. The C-suite remains divided, with one-third prioritizing responsibility, another third emphasizing innovation, and the rest valuing both equally. However, regulatory uncertainty is stifling AI growth, with over 80% of leaders citing unclear government policies as a major barrier to investment and implementation. Security and ethics are also falling behind AI ambitions—while 89% of executives are concerned about AI security risks, only 24% of CISOs believe their organizations have a strong risk-management framework.
Vertiv Leads Discussions on Advanced Cooling Solutions for AI at Data Center Nation in Riyadh
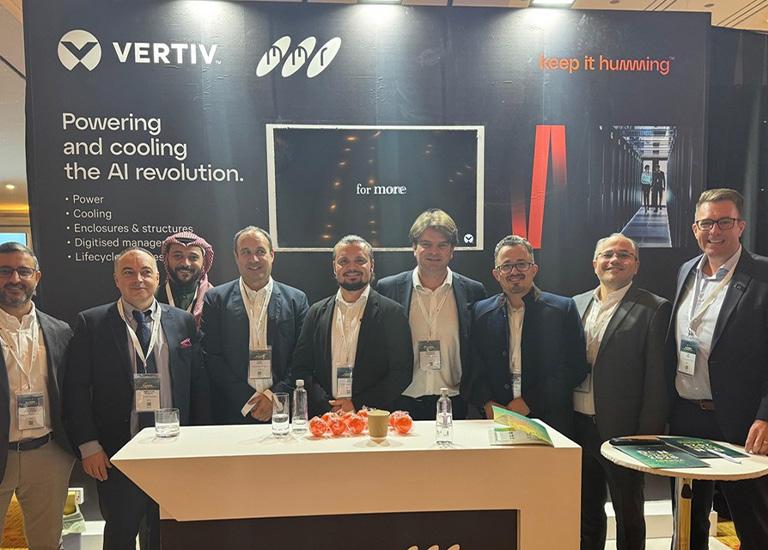
Vertiv played a prominent role at the inaugural Data Center Nation held recently in Riyadh. The event served as the leading platform for the data center industry in Saudi Arabia and brought together key players, thought leaders, and innovators in the field. Vertiv, in collaboration with Mohammed Mansour Al-Rumaih (MMR KSA), participated as a gold sponsor, highlighting their joint commitment to advancing data center infrastructure and innovation in the region.
During the event, Vertiv led an insightful panel discussion titled “Cooling the AI Era,” moderated by Piergiorgio Tagliapietra, Director of Application Engineering Thermal Management for EMEA, Vertiv. The session delved deep into the transformative power of Artificial Intelligence (AI) and its profound implications for data center infrastructure.
Attendees at Vertiv’s booth experienced the innovative Vertiv™ 360AI platform, which integrates liquid and aircooling technologies for high-density deployments of up to 132 kW per rack. This solution delivers unparalleled heat management, operational efficiency, and future readiness.
Tassos Peppas, Regional Director METCA, Vertiv said, “We were thrilled to be part of the inaugural Data Center Nation event in Riyadh, an emerging hub for data center innovation. Vertiv has always been at the forefront of technological evolution, and our commitment to advancing industry conversations remains steadfast. Engaging in forums like this, alongside our strategic partners and regional thought leaders from across the region, builds on our successes at similar events in the UAE, Poland, and Italy.
ServiceNow and Google Cloud Expand Partnership to Deliver AIpowered Tools to Millions of Users
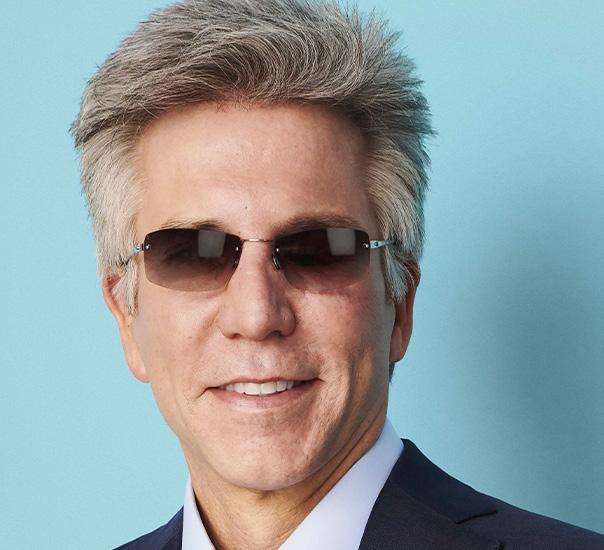
ServiceNow and Google Cloud have announced a major expansion of their partnership to maximize the value of generative AI across every layer of the enterprise technology stack. ServiceNow will bring its Now Platform and full suite of workflows to customers on Google Cloud Marketplace and also make its Customer Relationship Management (CRM), IT Service Management (ITSM), and Security Incident Response (SIR) solutions available on Google Distributed Cloud (GDC).
The companies share a vision for transforming enterprise work with gen AI. Making the ServiceNow platform and workflows across IT, CRM, and HR available on Google Cloud will allow ServiceNow to bring AI enhanced experiences to millions
of new and existing users. New end to end integrations will enable ServiceNow customers to use BigQuery to connect their enterprise data to AI; extend these AI powered insights to Google Workspace, where users can do things like easily access ServiceNow data directly within Google Sheets and Chat; build gen AI applications on top of their data foundation with Vertex AI; and more.
Bringing the Now Platform and ServiceNow’s full suite of workflows, including CRM, ITSM, and SIR solutions, to Google Cloud Marketplace will make it easier for businesses to combine their ServiceNow data with Google Cloud’s AI, data analytics, and productivity technology.
Riverbed Launches Smart OTel
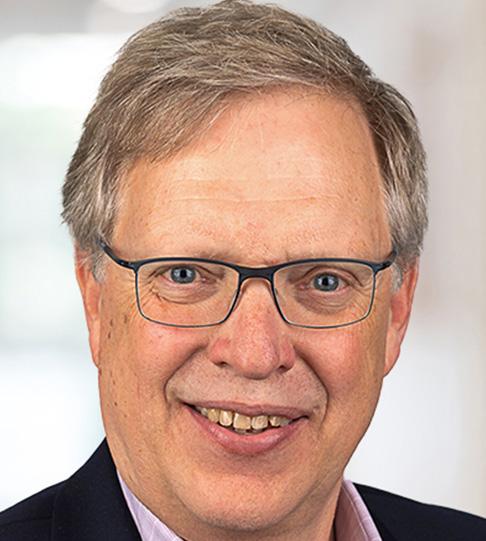
Riverbed has announced the general availability of Riverbed Smart OTelTM (OpenTelemetry), a game-changing approach to OpenTelemetry, delivering the right data at the right time for better insights and decision making. Available as part of the Riverbed Platform, Smart OTel supports Riverbed Aternity and Network Observability solutions, as well as third-party applications. Unlike traditional OpenTelemetry solutions, Smart OTel leverages platform-level data collection for
smarter analytics, automation and troubleshooting. With Smart OTel, once an event or alert is triggered, the patented Riverbed Data Store surfaces only pertinent data required for that specific event or business use case, and exports that data to any standard OTel collector. Customers can also leverage Riverbed AI and AI automations to further process the data prior to exporting, adding even more precision filtering and decision capability.
Bill McDermott, Chairman and CEO, ServiceNow
Richard Tworek, Chief Technology Officer, Alluvio, at Riverbed
Deloitte launches Silicon2-Service Offering
Deloitte has launched its comprehensive Silicon-2-Service (S2S) offering in the Middle East. This innovative service offering aims to provide end-to-end strategy, design, deployment, optimization and management of Sovereign AI capabilities for its most strategic clients. Unveiled at LEAP, the S2S offering’s all-encompassing AI approach blends industry best practices in production, deployment, and business integration. The service offering draws on Deloitte’s deep expertise in private cloud infrastructure, hardware optimization and management, AI software integration, data science, and data management, leveraging powerful technology platforms such as NVIDIA.
Zoom Uses Oracle Cloud Infrastructure to Power its AI-first Work Platform
Zoom Communications is using Oracle Cloud Infrastructure (OCI) to support its next-generation AI assistant, Zoom AI Companion, in Saudi Arabia. OCI is helping Zoom keep customer data in-region and deliver AI-first solutions. This provides users with AI personal assistants that help prioritize their work and deliver contextdriven responses to help prepare for, execute, and follow up after meetings. Zoom AI Companion helps users streamline their workdays by helping with a range of different tasks, including summarizing meetings, chat threads, and phone conversations, drafting chat messages, creating searchable smart recordings, answering meeting questions, and more, within the Zoom Workplace app.
HPE introduces nextgen ProLiant Servers engineered for AI automation
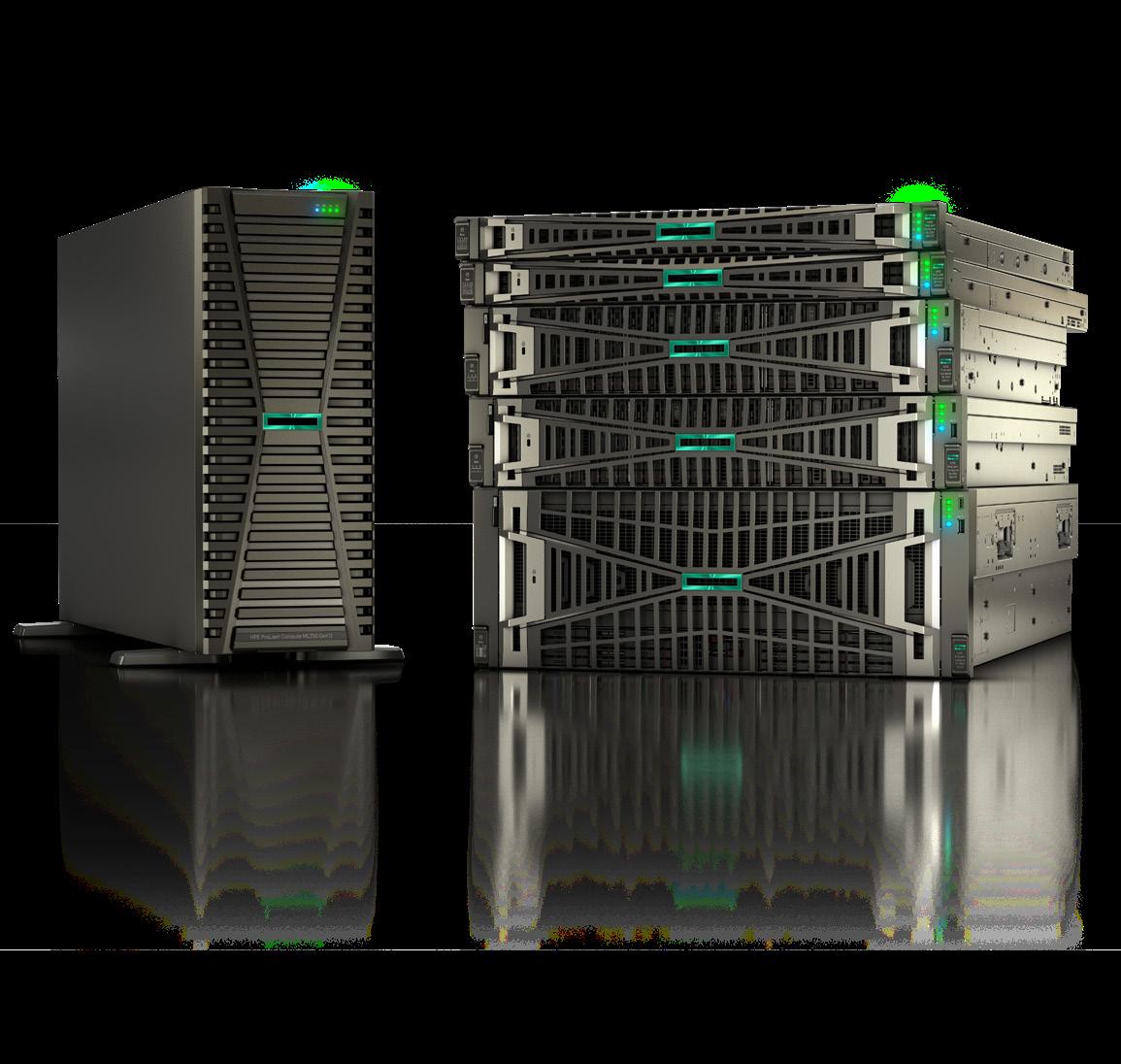
Hewlett Packard Enterprise (HPE) has unveiled eight new HPE ProLiant Compute Gen12 servers, designed to enhance security, optimize performance for complex workloads, and improve productivity with AI-driven management. Featuring upcoming Intel Xeon 6 processors, these servers cater to data center and edge environments.
The new servers introduce chip-to-cloud security with HPE iLO 7, which includes a dedicated secure enclave—a proprietary security processor offering quantum-resistant readiness and FIPS 140-3 Level 3 certification. This technology ensures an unbreakable chain of trust from manufacturing to decommissioning, protecting against firmware threats.
HPE Compute Ops Management enhances automation, security, and energy efficiency with AI-powered predictive insights. A new global map view provides real-time server health monitoring, reducing downtime by up to 4.8 hours per server annually. Power usage forecasting helps control costs and carbon emissions, aligning with sustainability goals.
These AI-driven management tools, including third-party integrations, are also available for HPE ProLiant Gen10 and newer servers. Additionally, HPE Power Advisor assists customers in evaluating energy costs and emissions before deployment.
Rapid GenAI Application Adoption Drives New Era of Application and Infrastructure Modernization
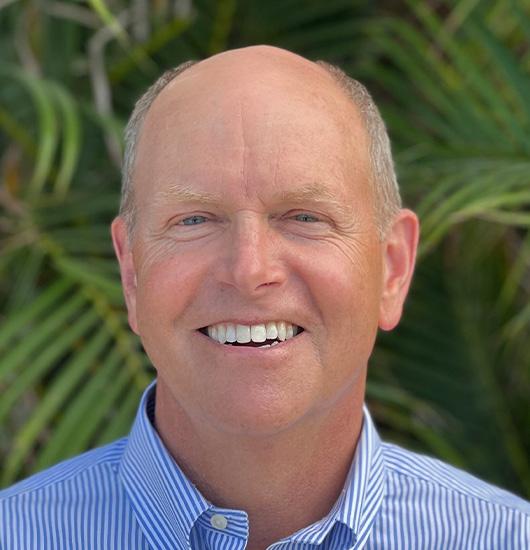
Nutanix has announced the findings of its seventh annual Enterprise Cloud Index (ECI) survey and research report, which measures global enterprise progress with cloud adoption. This year’s report sheds light on Generative Artificial Intelligence (GenAI) adoption, investment priorities, and benefits along with key challenges organizations face to meet the demands of these emerging workloads.
As GenAI application adoption and implementation move at a blazing pace, the ECI uncovered that while the majority of organizations have already implemented a GenAI strategy, implementation targets vary significantly. Organizations are eager to leverage GenAI for productivity, automation, and innovation, but they also face critical hurdles in the form of data security, compliance, and IT infrastructure modernization. Further, 90% of respondents expect their IT costs to rise due to GenAI and modern application implementation. But promisingly, 70% of organizations expect to make a return on their investment from GenAI projects over the next two to three years.
“Many organizations have reached an inflection point with GenAI implementation and deployment,” said Lee Caswell, SVP, Product and Solutions Marketing at Nutanix. “This year’s ECI revealed key trends that we’re hearing from customers as well, including challenges with scaling GenAI workloads from development to production, new requirements GenAI creates for data governance, privacy, and visibility, and integration with existing IT infrastructure. To successfully unlock ROI with GenAI projects, organizations need to take a holistic approach to modernizing applications and infrastructure and embrace containerization.”
Ai Everything Global 2025 wraps up as a leading AI
innovation hub
Ai Everything Global 2025 concluded in the UAE after two days of intense debates, groundbreaking discussions, and world-first AI application showcases.
Held at the Dubai Exhibition Centre, Expo City, the event featured top global AI experts exploring
how artificial intelligence can drive technological progress while benefiting society. Panel discussions tackled critical issues such as data sovereignty, ethical AI, and equitable access to technology.
A key topic was Sovereign AI— ensuring that AI systems align
with national values and support economic growth.
Another major discussion point was the need for equitable AI access. With one-third of the global population still offline, barriers remain in making AI a universal resource.
Lee Caswell, SVP, Product and Solutions Marketing at Nutanix
Hub71 expands Abu Dhabi’s tech ecosystem with new cohort
Hub71, Abu Dhabi’s global tech ecosystem, has welcomed 27 startups as part of Cohort 16 across Hub71’s three programs, Access, Hub71+ ClimateTech and Hub71+ Digital Assets. This new addition increases the total number of ventures supported by Hub71 to 357. The latest cohort includes startups across key priority sectors, reinforcing Hub71’s commitment to diversifying Abu Dhabi’s economy through technology and innovation.
Collectively, Cohort 16 startups have raised over USD 145 million (AED 532 million) in funding, averaging USD 4.9 (AED 18 million) million per startup. This achievement reflects Hub71’s track record in attracting high-potential companies that advance technological innovation across Abu Dhabi’s priority sectors. By joining Hub71, these startups are establishing their operations in Abu Dhabi, gaining access to a robust network of capital, mentorship, and resources to scale their businesses and drive impactful growth.
Cohort 16 was selected from over 1,300 applications, with 63% of chosen startups headquartered in leading technology markets such as the USA, UK and Germany. Among the notable additions to Hub71’s Access programis Vivan Therapeutics, a UK-based precision medicine company pioneering cancer research, using AI and fruit fly models to identify personalized treatments, that has raised USD 10 million (AED 36 million) in funding.
Meanwhile, Theion, a German startup developing sustainable sulfur-based batteries that store up to three times more energy than traditional batteries will join the Hub71+ ClimateTech program. South Korean startup Redbrick, a cloud-based 3D creation engine that uses AI and blockchain, has raised USD 16.3 million (AED 59.8 million) in funding and will join the Hub71+ Digital Assets program.
Furthermore, one in three startups in the cohort is based in the UAE, showcasing the nation’s growing status as a global entrepreneurship hub.
SolarWinds to be acquired by Turn/ River Capital
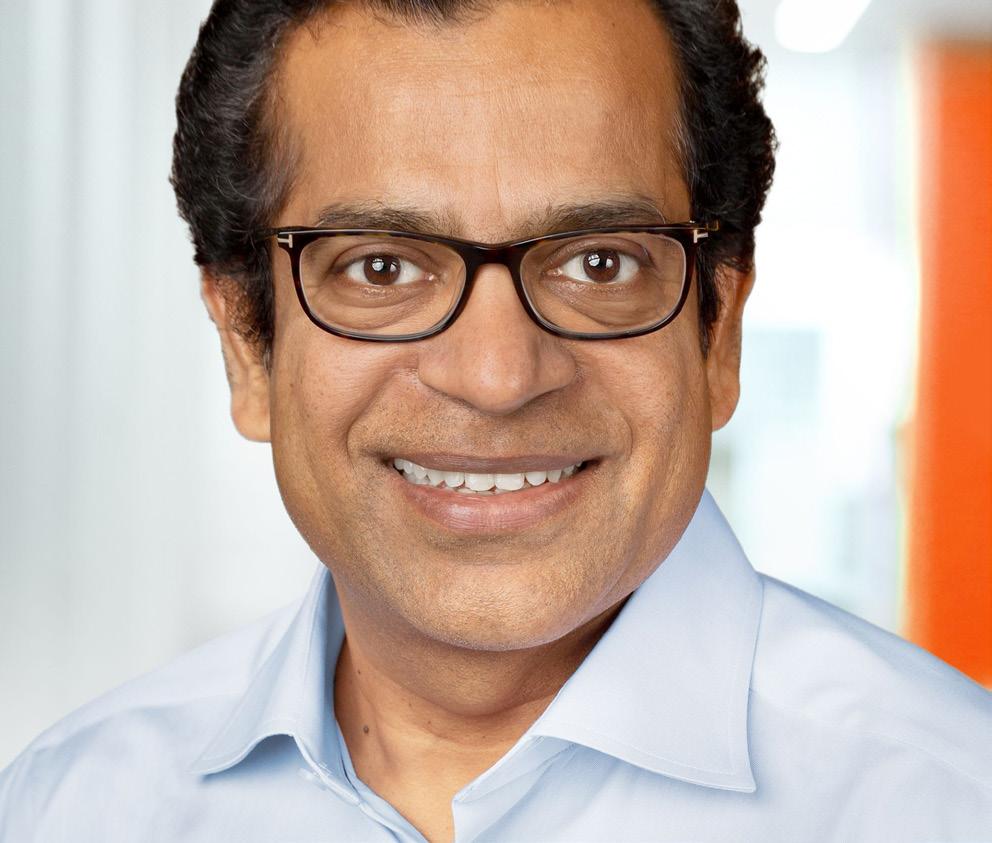
SolarWinds has announced that it has entered into a definitive agreement to be acquired by Turn/River Capital in an all-cash transaction for $18.50 per share or approximately $4.4 billion. The per-share price represents a premium of approximately 35% to the volume-weighted average closing price of SolarWinds stock for the 90 trading days ended on February 6, 2025.
“We have built a great track record of helping customers accelerate business transformations through simple, powerful, secure solutions designed for hybrid and multicloud environments. We now look forward to partnering with Turn/River to deliver operational resilience solutions for our customers on our SolarWinds Platform, leveraging our premier observability, monitoring, and service desk solutions,” said Sudhakar Ramakrishna, President and CEO of SolarWinds.
“This successful transaction and exciting partnership are testaments to our employees’ outstanding work of building exceptional solutions and delivering great customer success,” Ramakrishna continued. “We are confident that Turn/River’s expertise and growth orientation will help us ensure SolarWinds continues to drive innovation and deliver even greater value for customers and stakeholders.”
“SolarWinds is a global leader in software that helps a wide range of businesses securely manage and optimize their systems, networks, and IT infrastructure. Their deep commitment to understanding and solving customer needs has led to decades of innovation, impact, and consistent growth,” said Dominic Ang, Founder and Managing Partner of Turn/River Capital. “We are incredibly excited to partner with SolarWinds. By pairing our team of software operators and investors with their relentless focus on customer success, together we aim to accelerate growth and further innovation.”
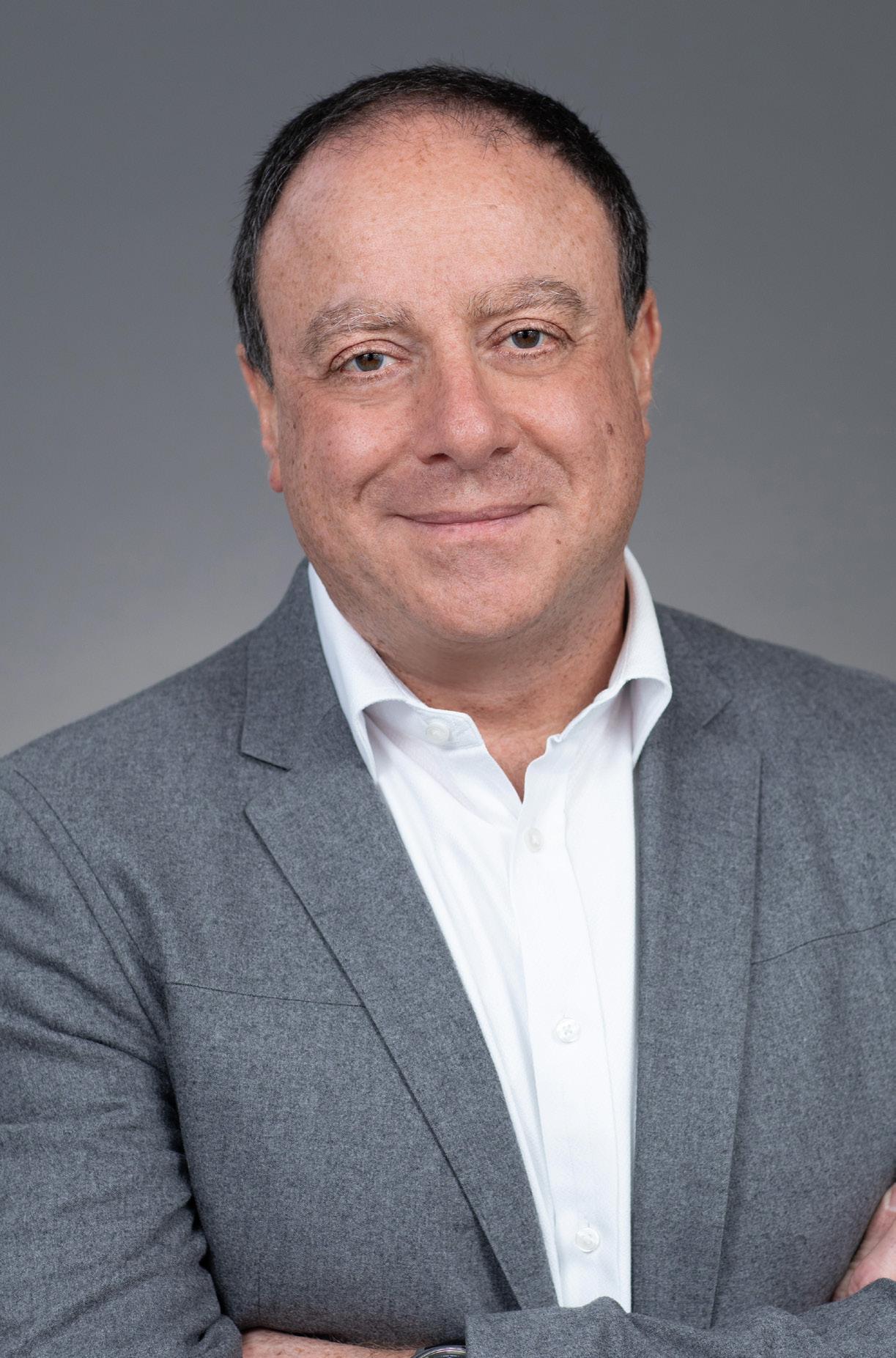
INTERVIEW
IHAB TARAZI
CHIEF TECHNOLOGY OFFICER AND SR. VICE PRESIDENT AT DELL TECHNOLOGIES, INFRASTRUCTURE SOLUTIONS GROUP, DISCUSSES HOW DELL IS POWERING THE NEXT WAVE OF AI INNOVATION.
“Building the future”
Let us talk about Dell’s AI Factory. Is it metaphorical, or is it a literal production strategy?
That’s an excellent question. It’s actually a literal, physical production strategy. We call it an AI Factory because it follows a different architecture than traditional IT. In IT, there’s something called hyper-converged infrastructure (HCI)—an AI Factory is essentially the AI equivalent of that.
For example, when we announced the AI Factory with NVIDIA, it included all the Dell components, physical infrastructure, software tools, and NVIDIA’s software—all packaged into one AI Factory.
What makes it a factory is that we don’t just provide the hardware—we test, integrate, design, and validate all the components to ensure they work seamlessly as a single system.
What are the core components of an AI Factory?
Let me break it down piece by piece.
Compute requires specialized GPUs. Some of the latest models integrate up to eight GPUs in a single box, which completely changes the compute architecture.
Networking is another crucial component, as AI workloads have immense network requirements. We use specialized software to optimize how data flows between GPUs, ensuring efficient GPU-toGPU communication.
Storage plays a critical role in AI performance. AI workloads require fast, high-density storage, and the challenge is feeding data to GPUs efficiently for both inference and training. If the storage layer is not optimized, it results in low GPU utilization, network bottlenecks, or
insufficient storage—all of which can be costly, given that GPUs are expensive.
If you attempt to build an AI infrastructure using a traditional plug-andplay IT approach, you will likely encounter performance inefficiencies, outages, and unnecessary expenses. That is why AI Factories focus on fine-tuning these components to work together seamlessly.
You mentioned inference servers. How do they fit into the AI Factory model?
Yes, inference servers are a crucial part of the AI Factory. They work alongside AI frameworks such as PyTorch, TensorRT, and AMD ROCm. The software running on these inference servers is just as important as the GPU hardware itself.
Each AI workload requires different precision levels—FP6, FP8, FP16—and every GPU must be optimized accordingly. Some of the latest GPUs provide as much of a performance boost from software optimizations as they do from hardware improvements.
That’s why an AI Factory must continuously update and integrate the latest software from GPU providers. Additionally, AI workloads require a specialized software stack, which includes:
• Operating systems (OS)
• Kubernetes clusters (starting with vanilla Kubernetes but increasingly optimized for inference)
• Inference server optimizations If these components aren’t optimized together, you won’t get the full performance benefits from your AI infrastructure.
Gartner predicts that about 30% of GenAI use cases will be abandoned after proof of concept by the end of 2025. How do you help your customers realize AI’s full potential?
The best way to answer it is to look at the biggest challenges companies face when implementing AI. Most companies struggle with data. Sometimes the required data doesn’t even exist—it has to be collected first. Even when data is available, it must be properly structured and optimized for AI processing.
Additionally, GPUs process data extremely fast, so they need a continuous, high-speed data pipeline. This requires data orchestration, which is where technologies like data lakehouses come in. We’re working with partners like Starburst to address these challenges.
Another key component is metadata management. When organizations work with AI-generated data (AG), they need to track versions and maintain an up-to-date dataset. Without proper metadata management, large-scale AI deployments become difficult to manage.
For example, let’s say a company wants to search its entire database for regulatory compliance documents. Without proper metadata tracking, finding the right files at scale would be nearly impossible.
The second challenge is around Security and Data Governance. Many organizations have strict policies preventing them from sending sensitive data to public cloud services. They need to keep their AI workloads on-premises.
For example, some companies prohibit employees from sharing proprietary data with ChatGPT or other cloud-based AI models. As a result, they must establish clear internal data governance policies before fully adopting AI.
These two challenges—data management and security—are by far the biggest hurdles for AI adoption.
What has been the feedback from early adopters of AI Factory?
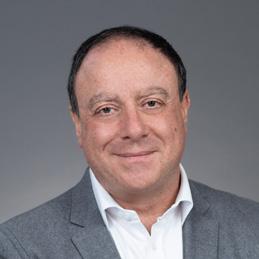
Yes, AI Factory customers are very diverse. The feedback we receive depends largely on the specific use cases.
What differentiates us is that we don’t just sell AI technology— we help customers use it effectively.
Another key component is metadata management. When organizations work with AI-generated data (AG), they need to track versions and
maintain an up-to-date dataset. Without proper metadata management, large-scale AI deployments become
difficult to manage.
Through our service organization and CTO office, we guide customers on how to integrate AI into their business strategies.
For example, we don’t just sell GPUs and leave them to figure it out. We work with them to optimize AI infrastructure, ensure smooth implementation, and align AI projects with their business goals.
This is what truly sets us apart. Having the best technology in the world is one thing, but helping customers leverage that technology for real business impact is what makes the difference.
INTERVIEW
Global ambitions
Thomas Pramotedham, CEO of Presight, explains why the homegrown company is exporting Abu Dhabi’s AI expertise.
Can you tell us more about Presight’s international expansion plans?
We are expanding not just our footprint in the UAE but also across the region, as our focus is on the Global South. And, of course, you’ve seen us establish our presence in Kazakhstan and expand our work in Africa. More recently, we’ve started engaging with governments in Southeast Asia.
A big part of this expansion is the fact that what has proven to work in Abu Dhabi serves as a strong testament to what can be exported from Abu Dhabi. If you’ve been following us, you know that our mandate is international. We are a homegrown company, building systems for the Abu Dhabi government and the UAE, which we then export—along with the broader ecosystem. For example, Jais – the Arabic LLM – was developed utilizing computational resources from G42. We take all of this under the
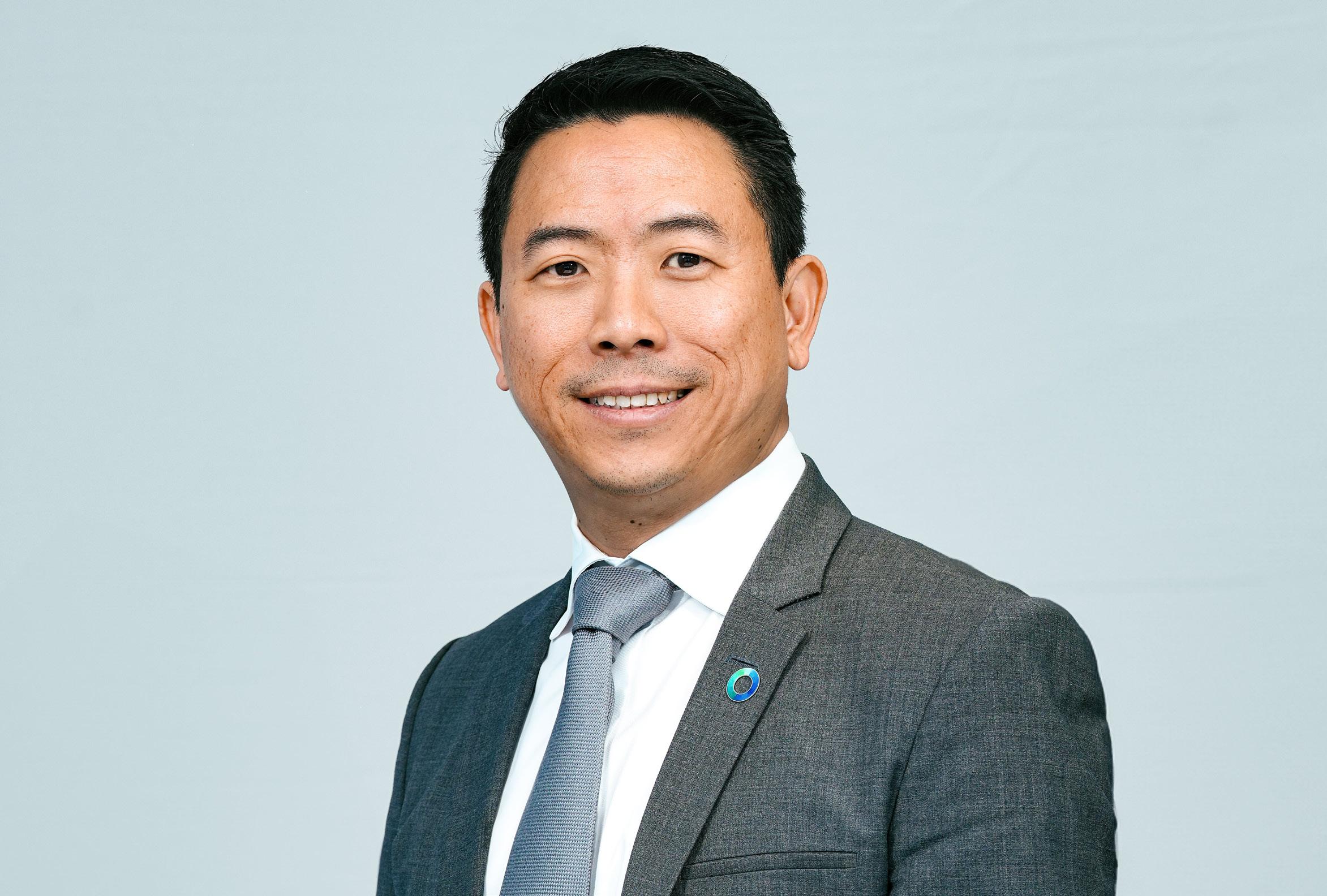
CEO OF PRESIGHT
THOMAS PRAMOTEDHAM

umbrella of “Abu Dhabi Inc.”
We are optimistic about sustaining this momentum and are witnessing a greater diversification of our revenue streams, with an increasing balance between UAE-based and international business. This shift is a strong indicator of where we are headed. I’m sure you’ll hear more updates in the coming weeks.
The way AI is being used varies significantly between developed and developing countries. In developed nations, it is applied differently—often in the medical sector. Meanwhile, in developing countries, AI is being integrated into transport systems, e-government services, and even smart grids. A recent success story is our work in Azerbaijan, where we helped modernize the energy grid for AzeriGas. Our approach is twofold:
1. Creating National Platforms – Unlike enterprise-level AI solutions, our focus is on large-scale government and state-owned enterprise projects.
2. Developing Agile, Scalable AI Products – Last year, we launched an enterprise AI suite, a comprehensive suite of AI assistants designed to make AI adoption more tangible. One of the biggest challenges with AI today— beyond tools like Copilot and ChatGPT— is that AI solutions are often difficult to purchase and implement effectively. We aim to simplify this process for executives, helping them quantify AI’s impact.
Last year, we acquired a majority share in AIQ from ADNOC. AIQ had already been driving AI adoption within ADNOC, and under its leadership, it uncovered $500 million in cost savings through AI-driven applications. Following the acquisition, we launched Energy AI, a large language model designed specifically for upstream energy operations. Within just 90 days, we released a report demonstrating multiple efficiencies, from resource optimization to time savings. One notable example is seismic cube analysis: a process that previously required two engineers five months to complete can now be done with 70% accuracy within a week. This is the real impact of AI on organizations and governments, and it’s what excites us. We are well-positioned to continue applying these proven AI use cases in new markets, expanding our reach and impact globally.
If you look at many AI projects in the region, they are in the PoC stage. How do
you help clients achieve tangible benefits from their AI use cases?
The key is to start with the outcomes. If you interact with my local team—or any of my team members—you’ll see that we run a structured program, beginning with a boot camp for the client. There’s no technology talk at this stage. Instead, we focus on the core questions: What do you want to achieve? Which areas do you want to improve? Is it HR? Resourcing? Logistics?
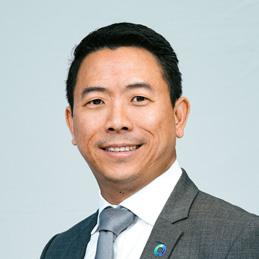
“One of the biggest challenges with AI today—beyond tools like Copilot and ChatGPT—is that AI solutions are often difficult to purchase and implement effectively. We aim to simplify this process for executives, helping them quantify AI’s impact.”
For example, with our work at Etihad Airways, they aimed to enhance predictive maintenance. With Masdar, they wanted a comprehensive view of their assets worldwide. These organizations don’t come to us saying, “I need AI,” or “I need a large language model.” Instead, they define the outcome they want to achieve, and we then design a solution that delivers on that goal. Rather than using the term POC, we use IOC (Initial Operating Capability). If a client presents a use case and knows what they want to achieve, we prove—using the best available technology—that it works. From there, they must move towards Full Operating Capability (FOC). This approach ensures that projects stay focused on delivering results. Yes, sometimes POCs fail—that’s true across all technological evolutions. But sometimes, POCs are necessary as an investment in education. Even if a POC doesn’t lead to full deployment, it can leave the client better informed. However, my team prioritizes value-based outcomes— choosing the right technology to achieve measurable impact.
We have been using AI long before Generative AI became mainstream. We have applied big data analytics, foundational AI models, NLPs, machine learning, and computer vision for years. The key is right-sizing AI applications to address realworld problems. When you solve a problem effectively, monetization follows.
What are the key differentiators for Presight?
First, we are truly Emirati—that’s one key differentiator. Second, we were born out of the Abu Dhabi ecosystem. Third, we are highly focused on applied technology and real-world solutions.
AGENTIC AI
The rise of agentic AI
A NEW KIND OF INTELLIGENCE IS EMERGING—ONE THAT DOESN’T JUST ANSWER QUESTIONS BUT ASKS ITS OWN.
Unlike the rigid, task-specific AI of the past, a new breed of artificial intelligence—Agentic AI—is emerging. These systems don’t just respond to commands; they think, plan, and act independently, making complex decisions in real time. From negotiating business deals to orchestrating emergency response operations, agentic AI is redefining what it means to have an artificial mind at work. It integrates advanced artificial intelligence, including large language models (LLMs), traditional AI like machine learning, and enterprise automation to create autonomous AI agents. These agents can analyze data, set goals, and take action with minimal human oversight, and solve complex problems dynamically.
Sascha Giese, Global Tech Evangelist, Observability, SolarWinds, says Agentic AI is not a new concept. “It was mentioned for the first time in the early 2000s, but we simply referred to it by different names in the past. In general, when we talk about AI or consume any science-fiction media, we are almost always referring to agentic AI at its core: a system that works autonomously and makes its own decisions.”
Sid Bhatia, Area VP & General Manager, Middle East, Turkey & Africa at Dataiku, says defines agentic AI refers to artificial intelligence systems designed to operate with a degree of autonomy, enabling them to make decisions and solve problems
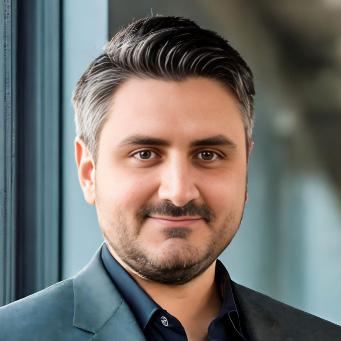
“In general, when we talk about AI or consume any sciencefiction media, we are almost always referring to agentic AI at its core: a system that works autonomously and makes its own decisions. ”
ARTURO BUZZALINO,
EPICOR
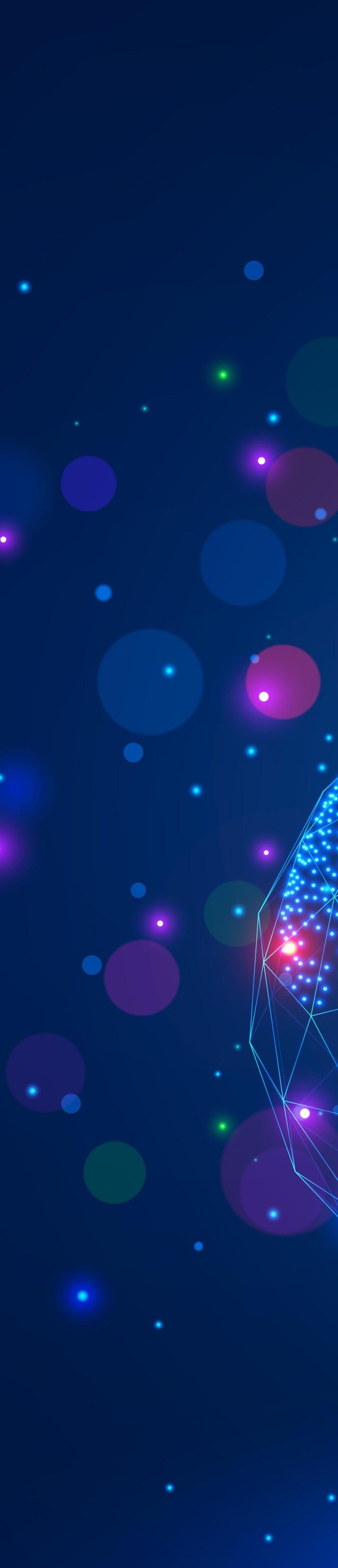
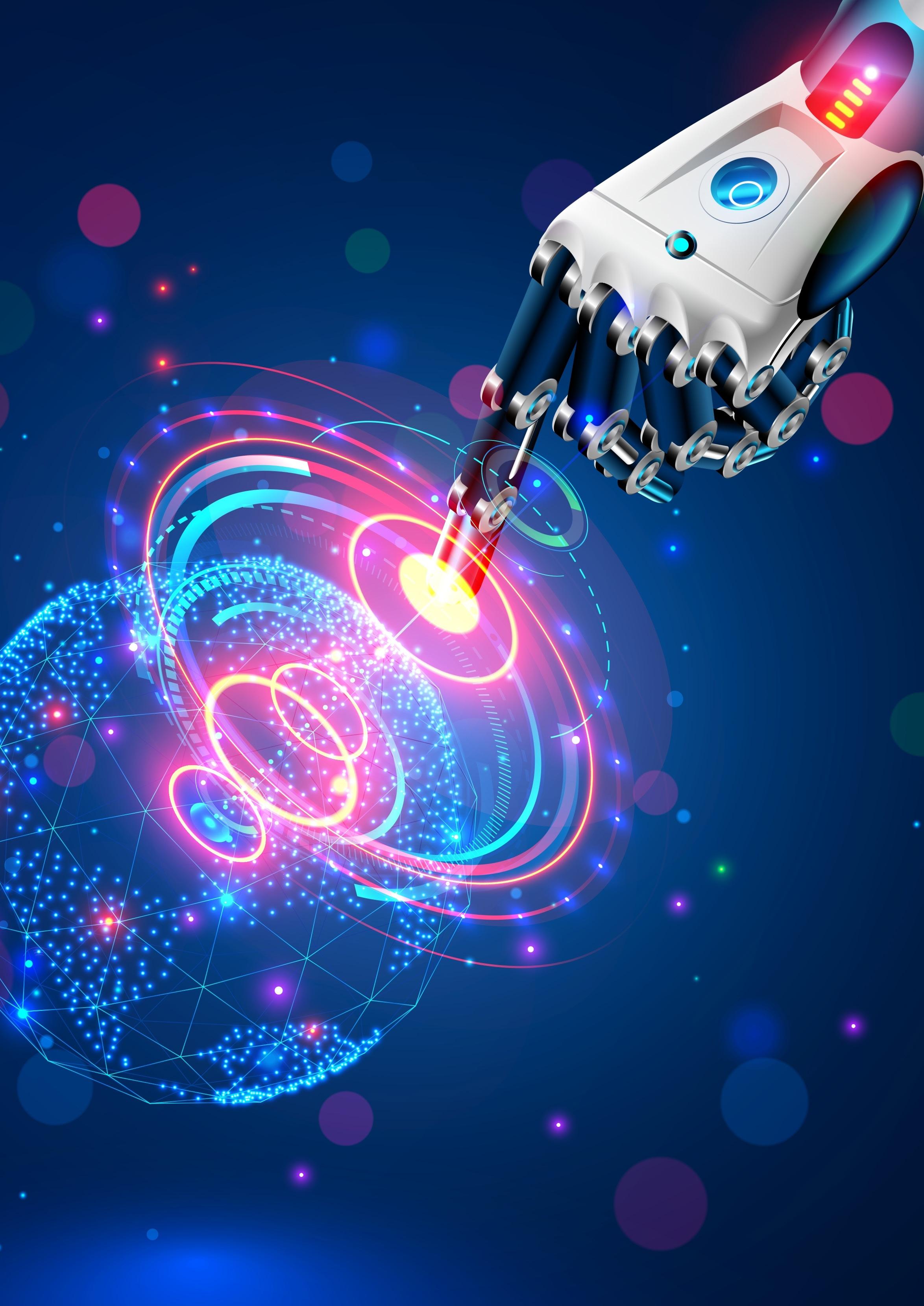
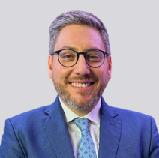
“It processes data from multiple sources, identifies patterns, and uses this insight to execute tasks like supplier negotiations or contract management. Machine learning enables it to refine its decisionmaking over time, while feedback mechanisms ensure continuous improvement.”
FRANCESCO COLAVITA, VP PRESALES CONSULTING, JAGGAER,
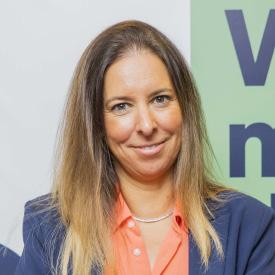
independently. Unlike traditional AI, which works within predefined rules and parameters, or generative AI, which focuses on creating outputs like text, images, or code, agentic AI is goal-oriented and capable of adaptive decision-making. It combines real-time feedback, long-term planning, and contextual understanding, making it more versatile in complex, dynamic environments.
According to Stefan Leichenauer, VP Engineering, SandboxAQ, traditional AI and generative AI are both tools used directly by human users: we supply an input and we get an output that is directly related to that input. An agentic AI system can act autonomously and take independent actions without explicit direction from a human user.
The agent may be able to observe its environment to gather data, either through physical or software-based sensors, and it may have a set of tools that it can use to accomplish tasks. For example, an agent may be able to send emails, or search the internet, or execute code, or control a robotic arm.
Jessica Constantinidis, Innovation Officer EMEA, ServiceNow, highlights that agentic AI functions similarly to the human brain, possessing cognitive abilities such as reasoning, learning, and understanding relationships. It can set its own goals and reason independently, without needing explicit instructions from humans. Once it starts reasoning, Agentic AI can adapt and modify its thinking, optimizing its algorithms based on new insights. It also has contextual awareness, allowing it to adjust its reasoning as new information is introduced.
“Like the human brain, it learns from past experiences and adapts its thinking for future tasks. In contrast, traditional AI systems and generative AI require human guidance and instructions to perform specific tasks. They cannot reason or learn autonomously like Agentic AI.”
“Like the human brain, it learns from past experiences and adapts its thinking for future tasks. In contrast, traditional AI systems and generative AI require human guidance and instructions to perform specific tasks. They cannot reason or learn autonomously like Agentic AI. ”
JESSICA CONSTANTINIDIS, SERVICENOW
Now this begs the question: How does agentic AI achieve autonomy in decision-
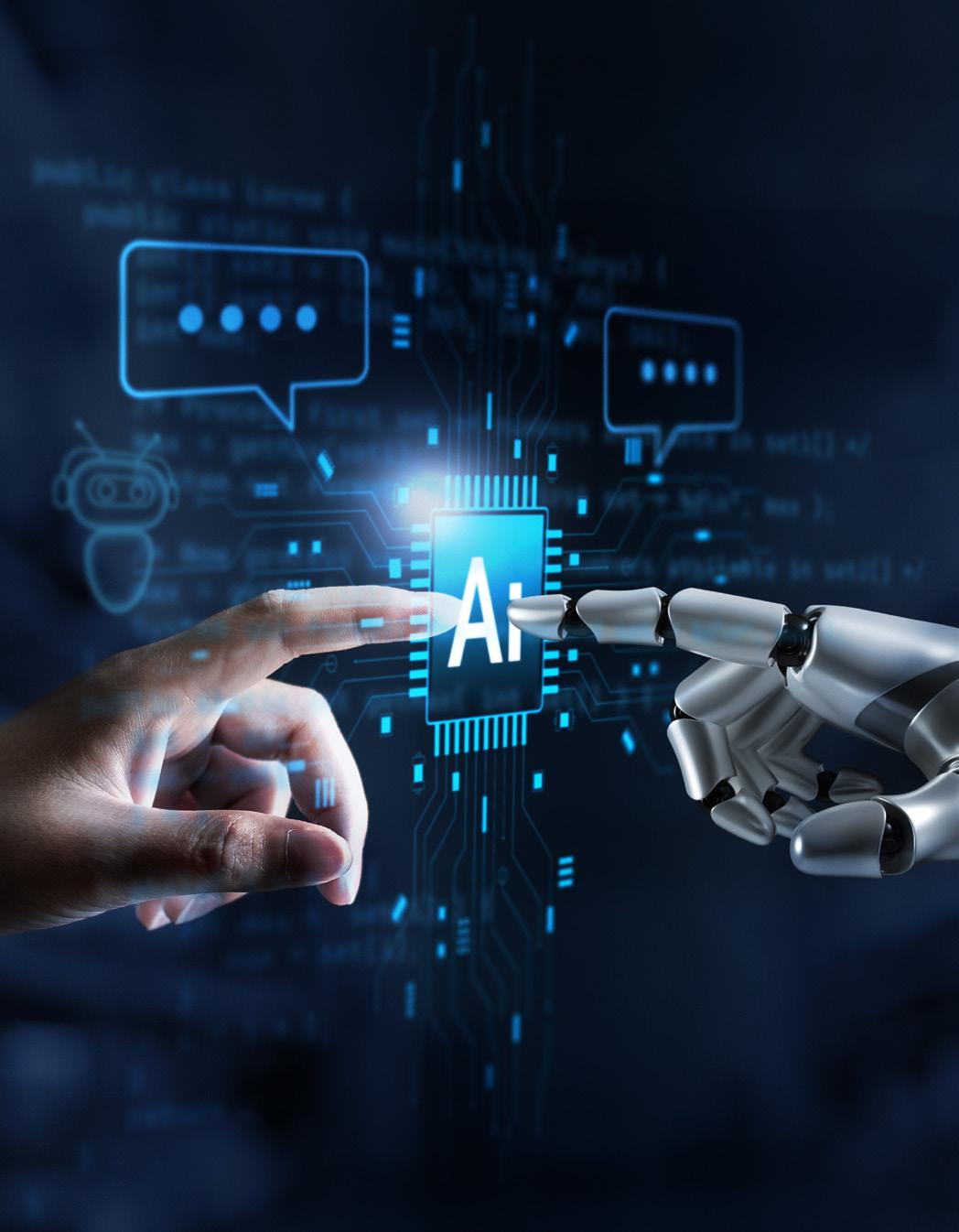
making, and what are the boundaries of its “agency”?
Joe Dunleavy, Global SVP & Head of AI Pod, Endava, says agentic AI realizes a higher level of autonomy through a few key characteristics. Unlike a typical generative AI, which is essentially a prompt-response engine, agentic AI is given a task or set of tasks, context about the environment in which it’s operating, and parameters as to its authority.
“Through careful articulation of the “rules of engagement”, it is able to function more independently than other implementations of AI, and when combined with other agentic processes, accurately simulates the kind of activity we come to expect from human operators in the real world. The key idea is that it is provided the guidelines (and in some cases, guardrails) up front and is bound within that rule set as it works,” he says.
Francesco Colavita, VP Presales Consulting, JAGGAER, explains that agentic AI achieves autonomy by combining data analysis, machine learning, and feedback loops. It processes data from multiple sources, identifies patterns, and uses this insight to execute tasks like supplier negotiations or contract management.

Machine learning enables it to refine its decision-making over time, while feedback mechanisms ensure continuous improvement.
However, its autonomy is bounded by predefined rules set by human operators, ensuring it operates within ethical, legal, and strategic constraints. For example, it must comply with procurement regulations and respect organisational risk tolerance.
“The effectiveness of agentic AI may also be limited by how well it integrates with existing systems and processes. Just as a human employee couldn’t effectively complete a task without access to the necessary resources, so too an AI agent would be limited by such constraints,” he adds.
TECHNICAL HURDLES IN DEVELOPING TRULY AGENTIC AI SYSTEMS
Generalization is a significant hurdle, as the AI must be capable of transferring its learning across diverse tasks and domains. Explainability is another critical challenge; systems must articulate the reasoning behind their decisions to ensure trust and transparency.
High-quality, unbiased, and comprehensive training data are essential for the AI to function effectively. Also, realtime adaptation requires algorithms capable of processing and reacting to vast amounts of data instantaneously.
Sid from Dataiku says integrating agentic AI into existing infrastructures without causing disruption is also complex, and robust safety mechanisms must be developed to prevent unintended consequences.
Arturo Buzzalino, Group Vice President and Chief Innovation Officer, Epicor, says major technical hurdles revolve around collecting and structuring vast amounts of data, ensuring real-time responsiveness, and creating systems that can interpret and adapt to changing conditions. Scalability, reliability, and transparent decision-making are also critical challenges—all of which must be addressed to unlock the full potential of truly agentic AI.
Computing power and cost and data copying limitations are currently at the forefront of Agentic AI development constraints, according to Jessica from ServiceNow. High transactional costs increase as the AI requires more computing and reasoning capacity. While newer chips,
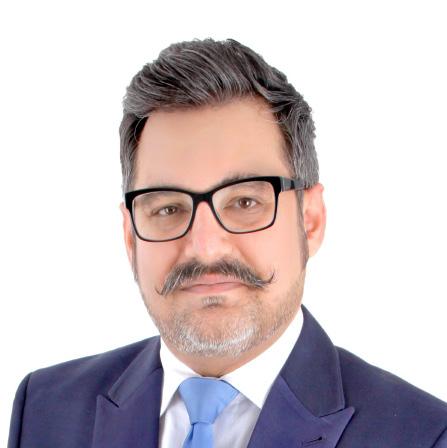
such as those from NVIDIA, are improving processing speed, more power and easier access to data are still needed for true agentic AI. Additionally, governance and data classification pose significant challenges.
“Data needs to be properly classified to ensure the AI understands which information it can use and which it cannot, but much data remains improperly labeled or unclassified. This lack of proper data tagging, limits
“The effectiveness of agentic AI may also be limited by how well it integrates with existing systems and processes. Just as a human employee couldn’t effectively complete a task without access to the necessary resources, so too an AI agent would be limited by such constraints.”
SID BHATIA, VP GM - MIDDLE EAST TURKEY, DATAIKU
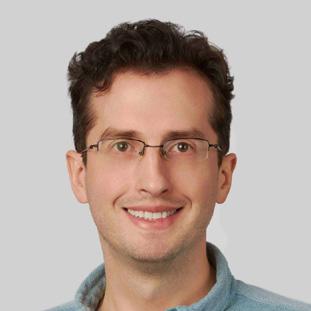
“Traditional AI and generative AI are both tools used directly by human users: we supply an input and we get an output that is directly related to that input. An agentic AI system can act autonomously and take independent actions without explicit direction from a human user.”
STEFAN LEICHENAUER, VP ENGINEERING AT SANDBOXAQ
the AI’s ability to function effectively and securely. A mature data classification system must be established as a standard to address these issues,” she says.
ADVICE FOR BUSINESSES
CONSIDERING THE ADOPTION OF AGENTIC AI SYSTEMS
The adoption of AI should be guided by clear business needs rather than an overemphasis on the tools themselves.
Sid from Dataiku says ideal opportunities for AI automation include complex workflows and repetitive tasks — for example, a cybersecurity team could use it to detect intrusions. Applications that would benefit from the real-time analysis of large data volumes are also prime candidates. This could be the marketing department using AI agents to identify market patterns and customer behaviours, or the finance team leveraging predictive analytics to forecast revenue trends and optimise budgeting decisions.
“Start by being crystal clear about the challenges you’re trying to address and the outcomes you want to achieve. For Agentic AI to be effective, businesses need highquality, frequently updated datasets and a solid strategy to guid its use. It’s also crucial to understand that while Agentic AI can improve decision-making and operational autonomy, it should always complement human oversight – especially in areas that demand ethical judgment or nuanced thinking,” concludes Thomas Pramotedham, CEO of Presight.
INTERVIEW
“Navigating the future of cloud and AI”
An exclusive interview with Cormac Watters, EVP – Business Applications, EMEA at Oracle.
Fusion Cloud and market differentiation? OThere are several key differentiators. Every vendor claims to have competitive features, and within each module, we do certain things better than others. For example, we have an excellent pricing feature and credit collection system, which are important. While there’s a lot of competition with strong features, our real strength lies elsewhere.
I’ve been in this industry for a long time, and I remember when integrated ERP became a priority 30 years ago. Back then, companies realized that having separate best-of-breed solutions for accounts receivable, accounts payable, and fixed assets was inefficient. Instead, they needed a fully integrated ERP system.
Today, cloud ERP follows the same principle. A fully integrated end-to-end suite makes sense, and this is where we stand out. We are one of the only vendors with
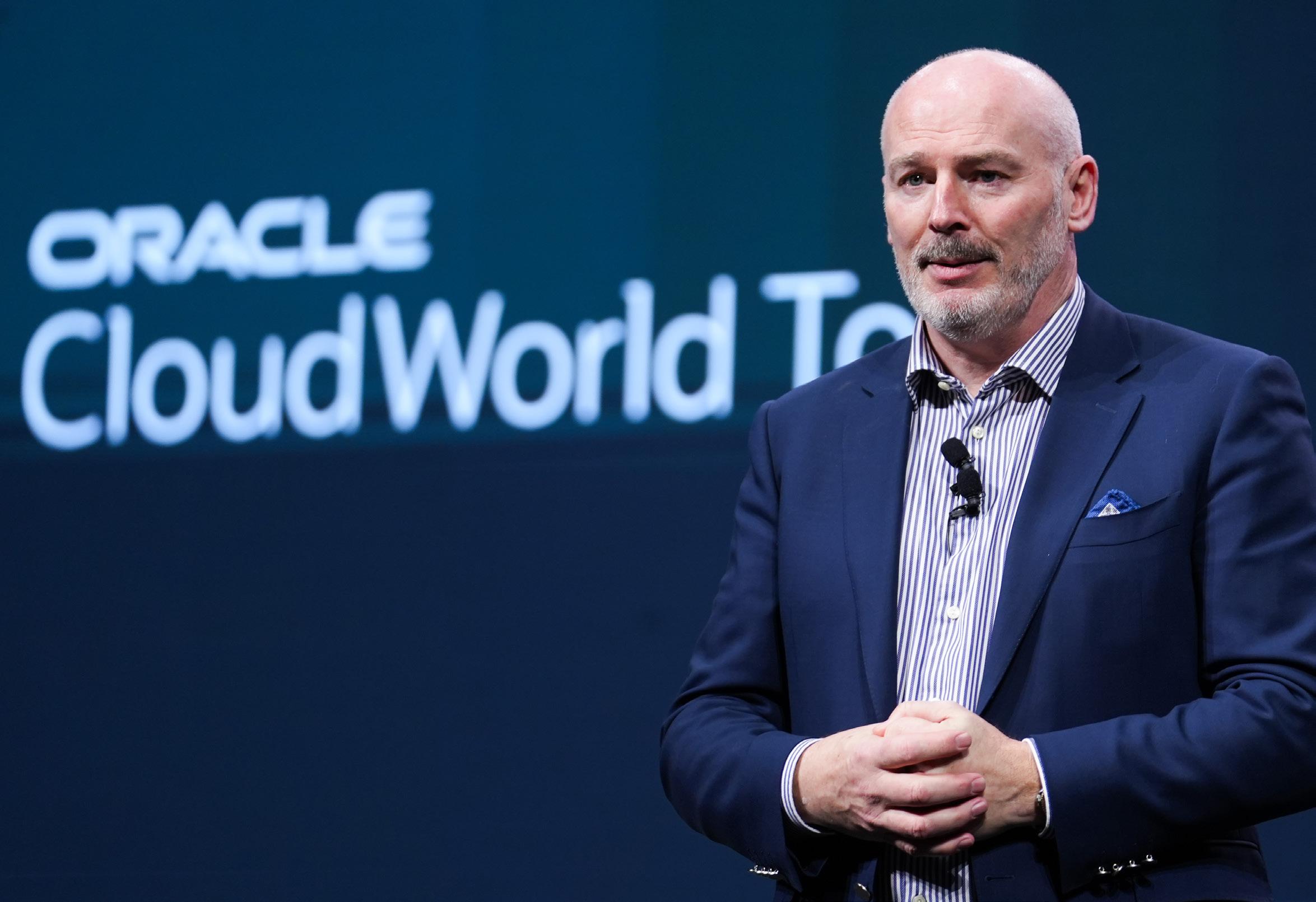

a truly integrated platform, where HCM, supply chain, and finance all run on the same data model. Some competitors offer cloud solutions, but their HCM, supply chain, and finance modules are separate products, leading to inefficiencies. Customers prefer a single unified system where everything works together seamlessly.
AI and the power of data
AI is transformative, but its effectiveness depends on data. If your data is fragmented across multiple platforms, how can you efficiently harness AI’s power?
Our integrated, single data model is a unique advantage. It enables better AIdriven insights, more automation, and seamless data flow across the entire enterprise.
Technology, security, and performance
Another key differentiator is that Fusion Cloud runs on Oracle Cloud Infrastructure (OCI). This gives us significant advantages in security, AI capabilities, and integration. Our applications influence the development of the platform, and in turn, the platform enhances our applications. This synergy ensures better security, performance, uptime, and faster response times—especially when using Autonomous Database.
When customers sign a contract with us, they get a single, fully integrated solution instead of managing multiple vendors. This simplicity, breadth of functionality, and performance optimization make us more secure, reliable, and efficient than competitors.
We have a saying: “The power of Oracle is in its entirety.” Each component is individually competitive, whether it’s our database, fixed assets, or OCI against AWS. But when everything works together, that’s when we truly differentiate ourselves.
The unique nature of Oracle Cloud
Many customers assume “moving to the cloud” simply means migrating workloads. But we educate them on what cloud actually means for their business. Are they just doing hosting? Is the application running on the same cloud as the infrastructure? Are there multiple control planes? Who is responsible for updates and security?
Our cloud is unique because there is no universal definition of what a cloud should be. Customers have the flexibility to deploy in a private cloud or a public region. Most of our customers opt for public deployment,
while governments tend to use sovereign clouds for regulatory compliance.
Data security and data sovereignty are always concerns, so we walk customers through how our model works. Once they understand how their data remains secure, they are comfortable with public cloud options. Governments and large enterprises may prefer dedicated private clouds, but most other businesses are happy with public cloud deployment.
Expanding cloud deployments
We are rapidly expanding our cloud infrastructure, with nearly 200 cloud regions deployed—171 currently live, with a goal of reaching 200+.
Our technical teams have developed the capability to deploy a full cloud data center with a much smaller footprint. The goal is to reduce this to just eight racks while maintaining full functionality.
This has created a new market where large telecom companies are adopting Oracle Alloy, using our cloud as a white-label solution to sell cloud services. This approach allows them to offer SaaS applications alongside infrastructure services, with Oracle managing SaaS updates across all deployment models—Alloy, public, and sovereign clouds.
How Oracle Alloy customers deploy
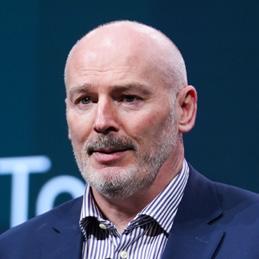
“Our technical teams have developed the capability to deploy a full cloud data center with a much smaller footprint. The goal is to reduce this to just eight racks while maintaining full functionality.”
Typically, large telecoms host Oracle Alloy within their own data centers, acting as cloud providers. This allows them to resell cloud services while benefiting from full Oracle capabilities. And yes, OCI is available as a service— this is not a “slimmeddown” cloud but a full-scale Oracle Cloud deployment. Our engineering teams have even enabled air-gapped deployments for highly secure environments, though most organizations don’t require that level of isolation.
NetSuite’s role in expanding the market
With NetSuite now part of our portfolio, we have a stronger presence in the mid-market segment. NetSuite adoption in the Middle East has been growing, particularly among smaller and mid-sized businesses.
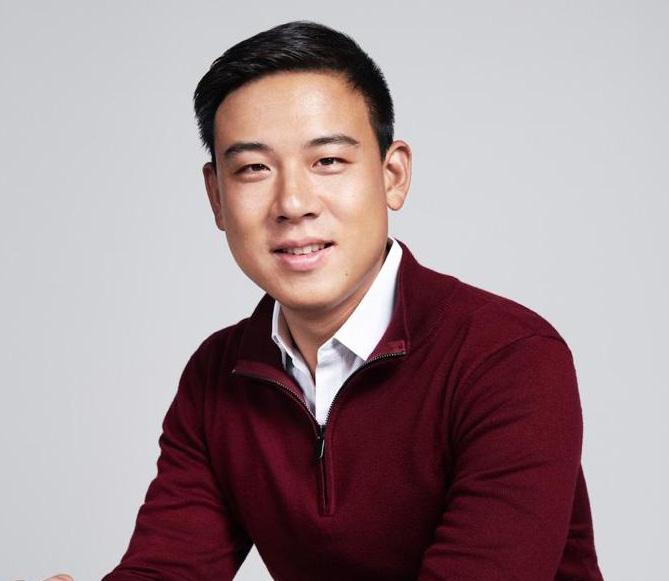
“Where is AI headed?”
Can you share a bit about your background and journey in the venture capital and AI investment space?
I’m the managing partner and co-founder of Mangusta Capital. We are an AI-focused venture capital fund investing in early-stage, application-layer AI companies, from preseed through Series A, and supporting their journey beyond.
Before this, I spent nearly 10 years at SoftBank as part of the Vision Fund investment team, investing in AI companies across various sectors, from supply chain and cybersecurity to vertical software. I grew up in Silicon Valley, so I’m a Cupertino native and have been surrounded by technological advancements for the last 20 to 30 years.
What brought you here?
I think the Middle East is a fascinating region, given its rapid development and increasing investment in technology and AI. I am somewhat familiar with the region due to my time at SoftBank, where we partnered with various local and regional governments, from Abu Dhabi to Saudi Arabia.
We continue to maintain strong relationships here, and I wanted to explore
INTERVIEW
KEVIN JIANG
CO-FOUNDER AND CHIEF INVESTMENT OFFICER AT MANGUSTA CAPITAL, SHARES HIS INSIGHTS INTO AI INVESTMENTS AND INDUSTRY TRENDS.
the region further, build relationships with investors, companies, and ecosystem partners, and find ways to collaborate. This is an incredibly dynamic and fastgrowing region, and I don’t want to miss any opportunities. That’s a big reason why I came here. Additionally, the event organizers invited me to speak, flew me out, and I wanted to be part of this exciting event.
Can you talk about your company’s regional focus?
We are a global VC firm, meaning we can invest in companies from any country worldwide. However, due to the concentration of AI talent, most of the companies we invest in are based in the U.S., where the majority of our portfolio lies today.
That said, we have also invested in Asian and European-based companies. If we find compelling opportunities in the GCC region, we are open to investing here as well. Our focus is on identifying the most promising entrepreneurs and opportunities, regardless of geography.
How fascinated are you with AI, and what are your predictions for its future?
AI is evolving at a rapid pace. To provide some context, at Mangusta Capital, we focus on the application layer of AI because we believe that infrastructure and AI models will become increasingly commoditized and highly competitive over time. This has been our thesis since we launched the fund about seven months ago.
DeepSeek is a great example that highlights this shift—demonstrating how infrastructure is becoming more competitive and efficient.
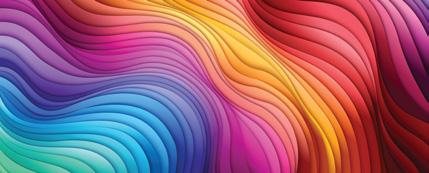
GUEST ARTICLE
The path to responsible AI
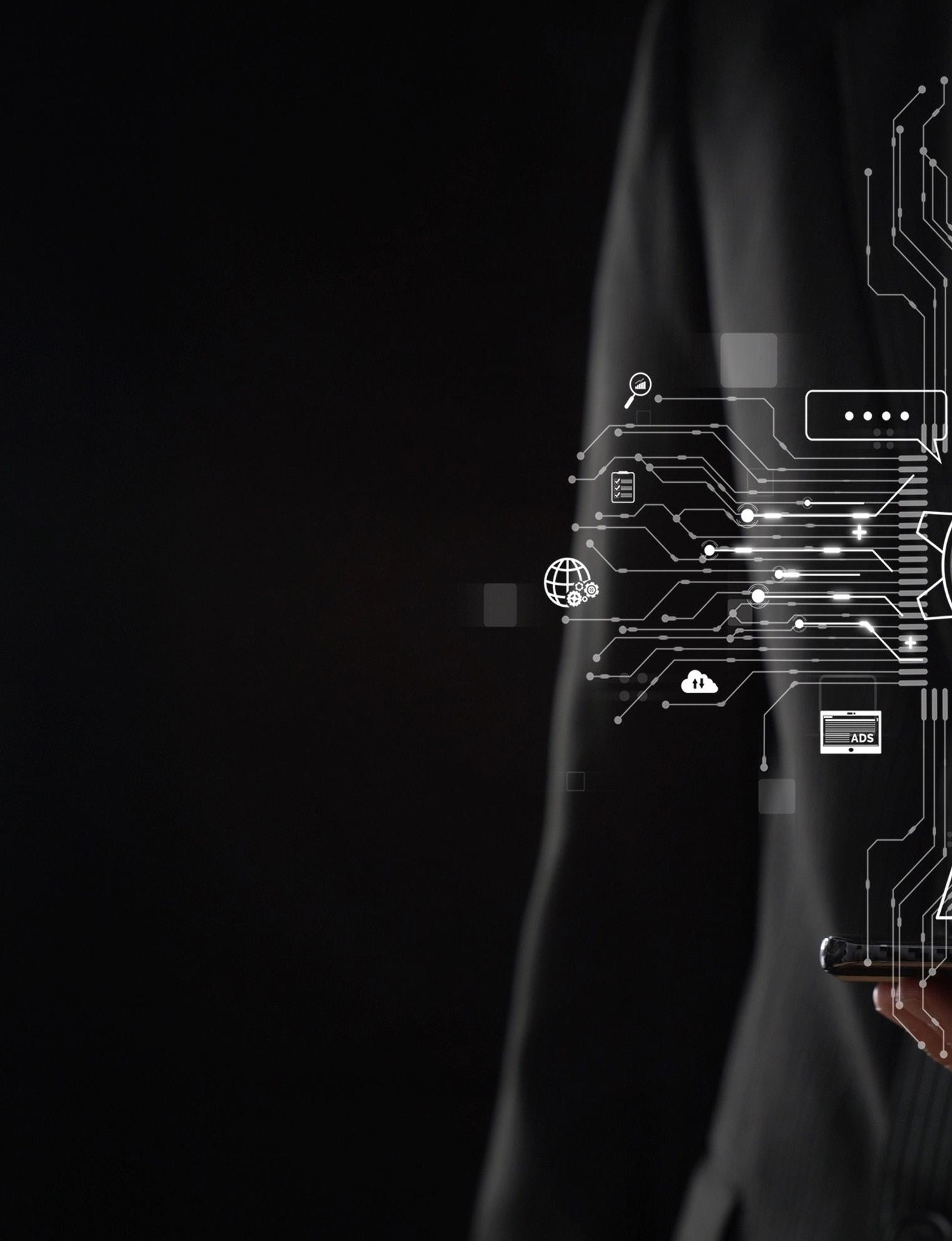
The ethos – and existential urgency –driving responsible AI policies is rooted in themes such as privacy, security, fairness, and transparency. Many headlines blur AI concepts such as machine learning, adaptive algorithms, deep learning, natural language processing, and generative AI (GenAI) technologies that are driving the current AI boom. People are worried about their data being misused, their words misconstrued, and their work misrepresented. These concerns are creating an environment of fear, uncertainty, and doubt.
How do we maintain control over AI so it doesn’t mislead, misinform, or harm humans? How do we ensure the AI models being integrated into products are not infringing on copyrighted content, imparting bias, or otherwise detrimentally interfering with livelihoods? How do we provide AI with the necessary level of self-sufficiency and autonomy while also protecting both consumers and business?
These are questions that are not so straightforward to answer, especially as the value of AI seemingly increases and potential use cases multiply. The good news is these are among the questions that many AI engineers, academics, legal experts, policy makers, and business leaders are actively sorting through as new regulations seek to balance responsible AI with innovation. But before we can address the question of the day – how can companies put responsible AI into practice? – we first need to ask and answer
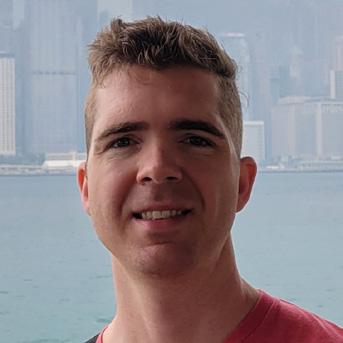
LEE MCLEAN
Legal Counsel and AI Specialist, Zebra Technologies, on how can we define Responsible Ai and tur it into action.
another question: What is responsible AI?
CAN WE DEFINE ‘RESPONSIBLE AI’?
There are many different definitions that generally align, but the International Organisation for Standardisation (ISO) provides a solid base-level definition. ISO states that “Responsible AI is an approach to developing and deploying artificial intelligence from both an ethical and legal standpoint. The goal is to employ AI in a safe, trustworthy and ethical way. Using AI responsibly should increase transparency while helping reduce issues such as AI bias.”
Though the intent of responsible AI is pretty straightforward, putting this theory into practice is where stakeholders struggle to find consensus. As Tess Valbuena, interim CEO of Humans in the Loop has said, the need for AI oversight – and the magnitude of oversight – is not as objective as many would probably like it to be.
Currently, it’s up to companies and individuals to develop responsible AI frameworks and determine how to comply with the responsible AI ethics standards and oversight processes. However, many standards organisations, governmental regulatory agencies, and professional licensing boards are attempting to provide guiding frameworks.
For example, in the U.S., an October 2023 Executive Order on the Safe, Secure, and Trustworthy Development and Use of AI (“EO 14110”) set the stage for the continued development of AI risk management
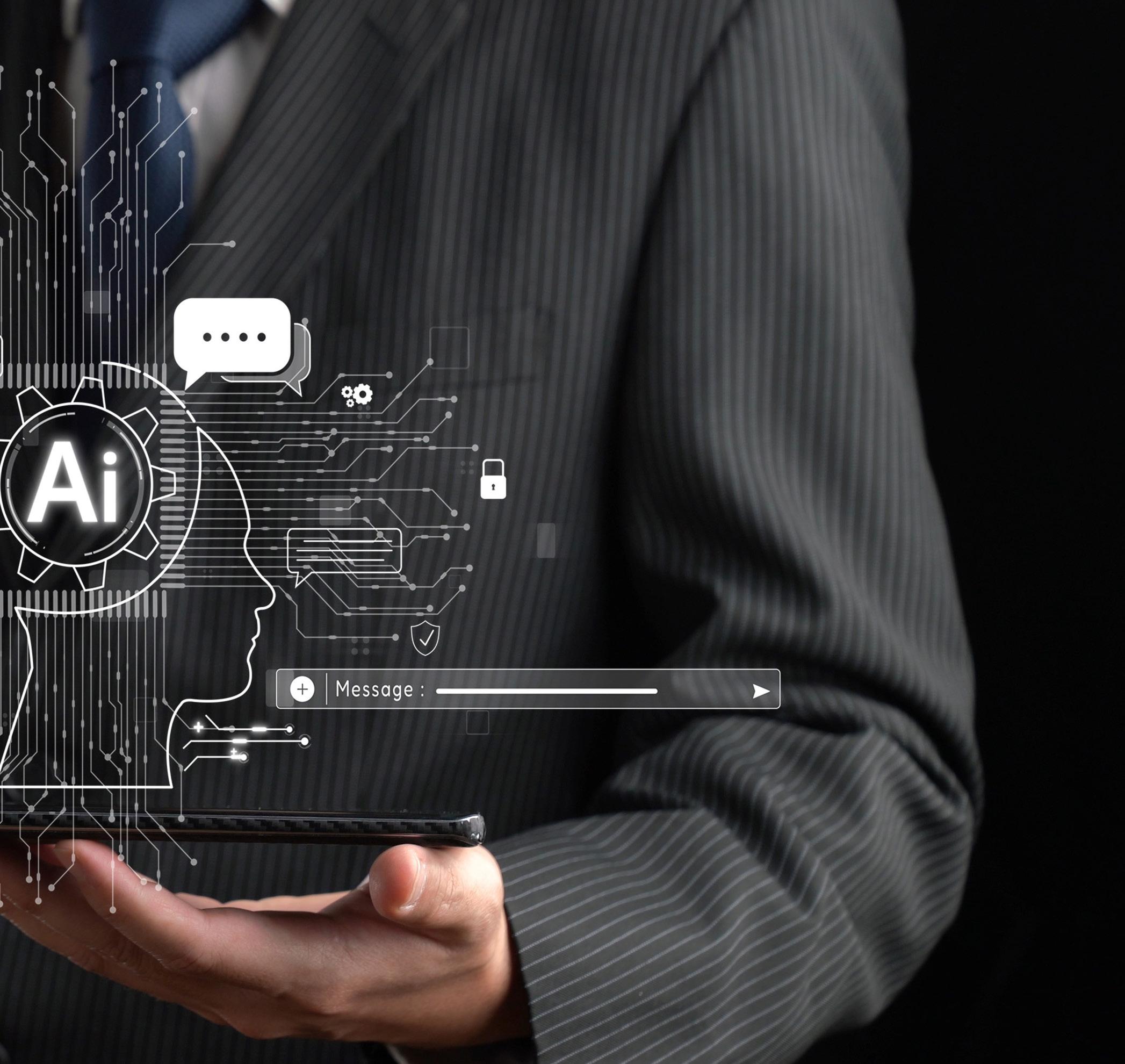
in place to prevent non-public or confidential information from being shared outside the model’s instance or organisation? Can a company trust the data source if the model uses thirdparty inputs to render services?
• Keep humans-in-the-loop (HITL)HITL is a concept outlined in many of the resources noted above and is discussed more in depth in this podcast episode. However, I want to stress how important it is to have human oversight of AI systems. The insertion of human judgment and intervention being integrated into the AI decisionmaking process can be critical to enhancing safety, reliability, and ethical compliance.
Also in July 2024, the European Union (EU) enacted a pioneering piece of legislation – the EU AI Act – that is the first comprehensive legal framework aiming to regulate AI development and use.
PUTTING A DEFINITION INTO PRACTICE IN THE WORKPLACE
Even welltrained, low-risk AI models can be flawed in their outputs (just like humans). It is generally a best practice to implement an HITL approach to assist in minimising hallucination risks. standards. One of EO 14110’s aims directed the National Institute of Standards and Technology (NIST) to develop standards for generative AI within 270 days of EO 14110’s release. In July 2024, NIST released the AI Risk Management Framework (RMF): Generative AI Profile (NIST AI 600-1), a companion piece to NIST’s AI RMF, which was previously published in January 2023.
What other steps should companies take to ensure they’re engaging with and/or following responsible AI practices? This is a bit difficult to answer without knowing the specific type of AI being leveraged and how companies are applying it (as the “easy example” describes above). However, the following are some general best practices:
• Confirm and vet the source of the AI model or tool - understand the ethical principles, policies, and practices of the AI’s provider and any other party involved in its training or ongoing oversight. Did they act responsibly during the model’s development and training? What are their current and long-term intentions with the model?
• Understand the source of the inputs used to train the AI model and how inputs are handled - are there controls
• Understand the risk of hallucinations with any AI model and have guidance in place for output verifications - do not disseminate or use AI outputs without confirming – and being completely confident in – their accuracy. Even well-trained, low-risk AI models can be flawed in their outputs (just like humans). It is generally a best practice to implement an HITL approach to assist in minimising hallucination risks.
• Confirm the right to input thirdparty data into the AI model and put guardrails up to ensure data isn’t fed into the model without proper permissions - data may include customer, partner, supplier, or general market knowledge, stories, and operational data, which may be comprised of confidential information, copyrighted material, personal data and other data for which permission to use may be required.
• Give proper attribution to sources used in the generation of the AI’s output - it’s critical that companies acknowledge the origin of work when using AI to generate content, regardless of format or intended use. Make sure Gen AI project leads understand the differences and correlations between “authorship” and “ownership” of content or products created using AI. Providing proper attribution for AIgenerated content promotes the key concept of transparency in responsible AI practices.
Remember, responsible AI practices aren’t just about compliance. They’re about integrity – about one’s character and (more broadly) culture.
AI Showdown: DeepSeek vs. ChatGPT vs. GitHub Copilot
vs. Alibaba Qwen
Here’s an in-depth analysis of four leading AI tools in 2025, focusing on their architectures, performance, use cases, and business implications.
WRITTEN BY:
SEHRISH
TARIQ | ASST EDITOR, GEC
Overview & Core Architectures
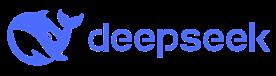
DeepSeek (China)
• Architecture: Uses a Mixture-of-Experts framework with 671B total parameters, activating only 37B per query for efficiency. Optimized for technical tasks like coding and mathematics.
• Training: Trained at 1/10th the cost of ChatGPT using FP8 precision and 2,048 Nvidia H800 GPUs.
• Ethics: Claims emphasize in transparency and bias mitigation, with open-source customization options.
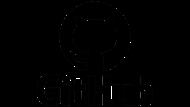
GitHub Copilot (Microsoft/OpenAI)
• Architecture: Built on OpenAI’s Codex (a GPT-3 derivative), fine-tuned for code autocompletion and debugging. Focused on Python, JavaScript, and other programming languages.
• Integration: Seamlessly embedded in GitHub and VS Code, offering real-time coding suggestions.
Security & Compliance
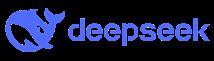
• DeepSeek: Raises data privacy concerns; lacks GDPR/CCPA compliance.
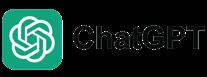
• ChatGPT: Complies with GDPR/CCPA; trusted in regulated industries (finance, healthcare).
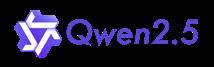
• Alibaba Qwen: Opaque data practices; subject to Chinese censorship laws.
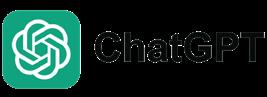
ChatGPT (OpenAI, USA)
• Architecture: Dense transformer model (1.8T parameters) optimized for natural language fluency and versatility. Integrates Reinforcement Learning from Human Feedback for iterative improvements.
• Training: Requires massive computational resources (estimated $100M+ training cost) and cloud-dependent deployment.
• Ethics: Complies with GDPR/CCPA.
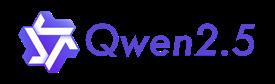
Alibaba Qwen (China)
• Architecture: Transformer-based model optimized for Mandarin-language tasks and e-commerce applications. Limited open-source access due to Chinese regulations.
• Training: Trained on Asian-market data, excelling in product descriptions and basic translations but weaker in complex problem-solving.
Ideal Use Cases
DeepSeek
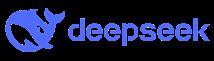
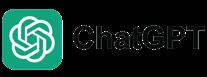
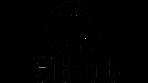
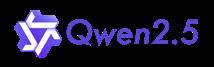
o Technical research, coding tutorials, and cost-sensitive startups.
o Example: Solving advanced math problems or debugging Python code.
ChatGPT
o Creative writing, customer service bots, and enterprise automation.
o Example: Drafting marketing campaigns or summarizing legal documents.
GitHub Copilot
o Software development teams needing real-time coding assistance.
Alibaba Qwen
o E-commerce businesses targeting Mandarin-speaking markets.
Performance & Use Cases
Criteria DeepSeek
Technical Tasks
Superior in coding (97% logic puzzles), math (90% accuracy), and structured problem-solving.
Creative Writing Weak in open-ended creativity; outputs are concise but lack flair.
Efficiency
Processes 14.2 tokens/sec with 0.96s latency; 60% lower energy use.
Multilingual Support Strong in English and Chinese but politically censored.
Pricing Free open-source tier; API at $0.55/million tokens.
Strengths & Weaknesses
DeepSeek
• Strengths:
ChatGPT
Moderate coding skills (89th percentile on Codeforces) but verbose explanations.
Excels in storytelling, marketing copy, and conversational content.
Slower due to monolithic architecture; latency increases with task complexity.
Supports 50+ languages with global context awareness.
Free GPT-3.5 tier; GPT-4 at $20/month.
GitHub Copilot Alibaba Qwen
Best-in-class for code generation, debugging, and autocompletion.
Not applicable.
Optimized for real-time coding assistance.
Primarily Englishfocused.
Paid subscription (varies by plan).
o Cost-effective, open-source, and highly efficient for technical tasks.
o Ethical focus with transparent reasoning (e.g., chain-of-thought explanations).
• Weaknesses:
o Limited conversational fluency and creative capabilities.
o Censorship concerns for politically sensitive topics.
ChatGPT
• Strengths:
o Versatile for creative writing, customer service, and general knowledge.
o Strong integration with enterprise tools (Microsoft Azure, GitHub).
• Weaknesses:
o High operational costs and cloud dependency.
o Limited customization due to proprietary code.
GitHub Copilot
• Strengths:
o Unmatched code generation speed and accuracy for developers.
o Context-aware suggestions within popular IDEs .
• Weaknesses:
o Limited to coding tasks; no broader AI functionalities.
Alibaba Qwen
• Strengths:
o Budget-friendly for Asian markets; excels in Mandarin content and e-commerce.
o Lightweight deployment for small businesses.
• Weaknesses:
o Restricted by Chinese content policies; lacks global adaptability.
o Weak in technical depth and complex problem-solving.
Limited to basic coding and translations 914.
Focused on Mandarin ad scripts and e-commerce content.
Efficient for basic tasks but struggles with highcomplexity queries.
Optimized for Mandarin; limited global adaptability.
Low-cost API; free tier for basic tasks.
Final Verdict
• For Developers: GitHub Copilot > DeepSeek > ChatGPT.
• For Creativity: ChatGPT > Alibaba Qwen > DeepSeek.
• For Budget-Conscious Users: DeepSeek > Alibaba Qwen > ChatGPT.
• For Enterprises: ChatGPT (compliance) > DeepSeek (technical) > Alibaba Qwen (niche).
Each tool excels in specific niches, so the choice depends on your priorities: technical precision (DeepSeek), versatility (ChatGPT), coding efficiency (Copilot), or costeffective Mandarin solutions (Alibaba Qwen).
THE FUTURE OF AI IN THE GCC
BOSTON CONSULTING GROUP (BCG) HAS UNVEILED ITS LATEST REPORT, UNLOCKING POTENTIAL: STRATEGIES DRIVING GCC’S DIGITAL & AI MATURITY. THE STUDY REVEALS HOW ORGANIZATIONS IN QATAR, SAUDI ARABIA, AND THE UNITED ARAB EMIRATES LEVERAGE DIGITAL AND AI STRATEGIES TO ACCELERATE TRANSFORMATION.
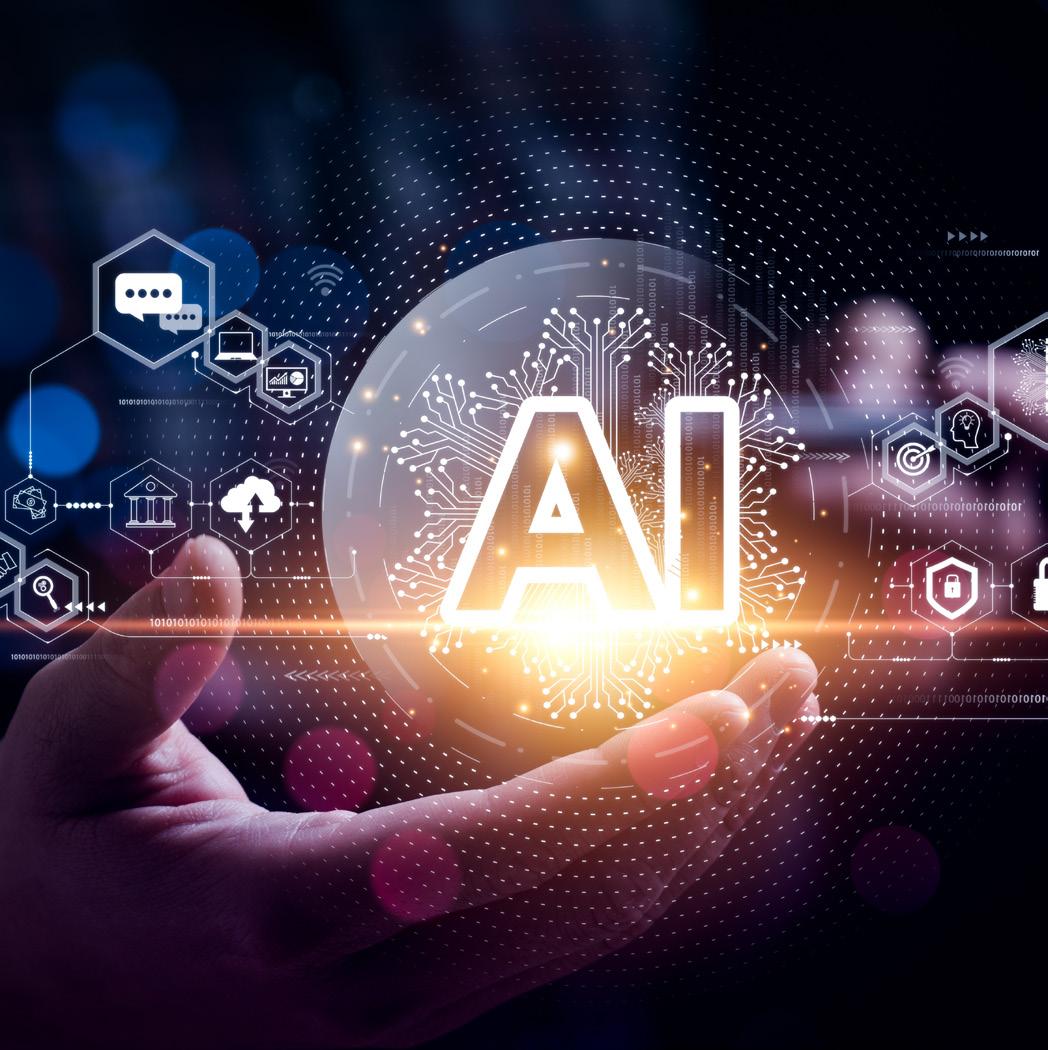
Emerging technologies are reshaping the world at an accelerating pace. As the GCC races ahead with its ambitious economic development plans, the region has already well progressed on its technology infrastructure and has provided many needed legislative, investment, and entrepreneurial environments for digital-first leading organizations to emerge within the region.
To gauge this digital and AI readiness, BCG’s 2024 Build for the Future (BFF) study examined the digital maturity of organizations in the GCC with a special focus on AI as a most transformative emerging technology. The study surveyed C-suite executives and senior leaders from 200+ organizations across eight sectors in Qatar, Saudi Arabia, and the United Arab Emirates.
Upon evaluating organizations across 53 core capabilities pertaining to digital maturity and AI readiness, the BFF study methodology categorizes each surveyed organization into one of four categories representing their stage of digital transformation, from least to most mature: Stagnating, Emerging, Scaling, and Future-Built.
CORE CAPABILITIES: GCC ORGANIZATIONS TO CATCHUP WITH GLOBAL DIGITAL AND AI MATURITY LEVELS
GCC organizations are presented with a unique opportunity and a matching challenge to build on their capabilities to leap into global-level digital and AI maturity. In 2024,
GCC organizations show higher maturity around customer journey and digital operations capabilities, however, have yet to fully possess many of the critical enabler capabilities that would allow them to fully deploy their digital and (Gen)AI strategies, and will need a step-change, particularly in their data and technology capabilities.
Where fast-changing technology landscapes and rapid AI adoption are paramount to future success, GCC organizations have a tangible opportunity to catch up with their global counterparts at the overall digital maturity level. While 25% of GCC organizations fall into the top two scaling or future-built maturity levels the global share is 31%. At a sector level, the Public Sector in the GCC exhibits key areas at world-class digital maturity levels, while Financial Institutions and Tech companies exhibited the highest digital and AI maturity scores across the GCC. Overall, however, digital and AI maturity in the GCC in 2024 fell behind the global average.
CHALLENGES & OPPORTUNITIES: GCC ORGANIZATIONS ON THE ROAD TO AI VALUE DELIVERY
In 2024, 17% of organizations in the GCC scored into the top two AI maturity levels (AI-scaling & AI-Future-Built). In this regard, the Financial Institutions sector had the highest share of top-level AI-maturity organizations1 or “AI leaders” where 29%

of financial institutions scored in the top two levels in 2024, followed by Healthcare sector (23%) and the Public Sector (20%). Additionally, our study found that the highest AI maturity organizations have three times the rate of success extracting value from GenAI (more on this below). Yet, the GCC remains at the early stages of (Gen) AI adoption, with only 9% of organizations surveyed at this level of value delivery. Overall half of all organizations surveyed (53%) are either still experimenting with GenAI with no official policies set in place, or not actively using it at all. The remaining (38%) recognize the value of adoption and are planning to scale up with guardrails. Similar to the overall digital and AI maturity trend in the GCC, sectors with highest share of organizations generating value with (Gen) AI are the Public Sector, as well as the Tech and Telco sectors.
While every AI journey is tailored to each organization, we found common challenges in the region. For instance, 6% of surveyed organizations have expressed not fully understanding GenAI, which lies in the critical need for leadership initiatives to educate and upskill while setting an AIand People, Org, and Process-first strategy. For organizations further down the line of (Gen)AI adoption, several challenges have been marked across BCG’s. “10-20-70” Algorithm- Technology- and People, Org, and Processes2 framework.
In fact, the highest share of GCC organizations observed gaps in the people, processes, and organizational dimension as the biggest barriers to AI maturity. This includes limited specialized talent, a gap in overall AI literacy, and a lack of sufficient incentives for innovation and GenAI adoption in working processes. Difficulty integrating AI within exiting IT systems and lack of access to unified and high-quality data further hinders progress.
On the other hand, compared to stagnating and emerging organizations, AI leaders have been successful in embedding AI for process-level productivity aimed at reshaping critical business and customerfacing functions as well as at integrating innovation in core corporate functions. To do so, AI leaders in the GCC have focused on key enablers including increased investment and focused budget allocation, as well as digital-first resource planning. High-maturity organizations allocated 2.4x
more funding to AI initiatives as well as a 2.3x higher share of FTEs were dedicated to digital & AI transformation, achieving a 1.7x higher share of (Gen)AI products scaled organization-wide and reflecting a long-term commitment to embedding innovation.
VALUE MAKERS: DIGITAL & AIFIRST STRATEGY FOR FUTUREBUILT ORGANIZATIONS
The 2024 BFF study highlights the need for most GCC organizations to progress beyond incremental moves and embrace comprehensive digital and AI strategies to unlock transformative value across sectors. While GCC organizations have made impressive progress in digital and AI capabilities, there remain opportunities to further enhance their maturity levels in critical areas and continue building on their strengths to lead globally in digital transformation and AI adoption.
To bridge the global maturity gap and accelerate impact, GCC organizations must embrace a bold, digital & AI-first strategy, across 5 key recommendations:
The 2024 BFF study highlights the need for most GCC organi zations to progress beyond incremental moves and embrace compre hensive digital and AI strategies to unlock transfor mative value across sectors.
1. Re-align organizational strategy with a digital-first vision to overcome structural barriers like operational agility and talent development.
2. Set a bold strategic ambition for AI adoption focused on clear value pathways and guardrails for responsible AI adoption.
3. Boost viable people and org capabilities and underlying technology platforms to support ambition and invest in parallel to scale up.
4. Maintain a pipeline of continuing innovation pilots to rapidly and effectively adapt to changing landscape of emerging technologies.
5. Prioritize high-profile cross-cutting lighthouse initiatives with high ROI to fund the journey and build momentum for transformational org-wide change. The GCC stands at a crossroads where technological advancements intersect with the region’s aspirations to lead in digital and AI innovation. By addressing these priorities, GCC organizations can unlock transformative potential, enabling them to capitalize on emerging opportunities, catchup to global peers, and earn their position as future-ready pioneers in an increasingly digital world.
DeepSeek marks a potential shift
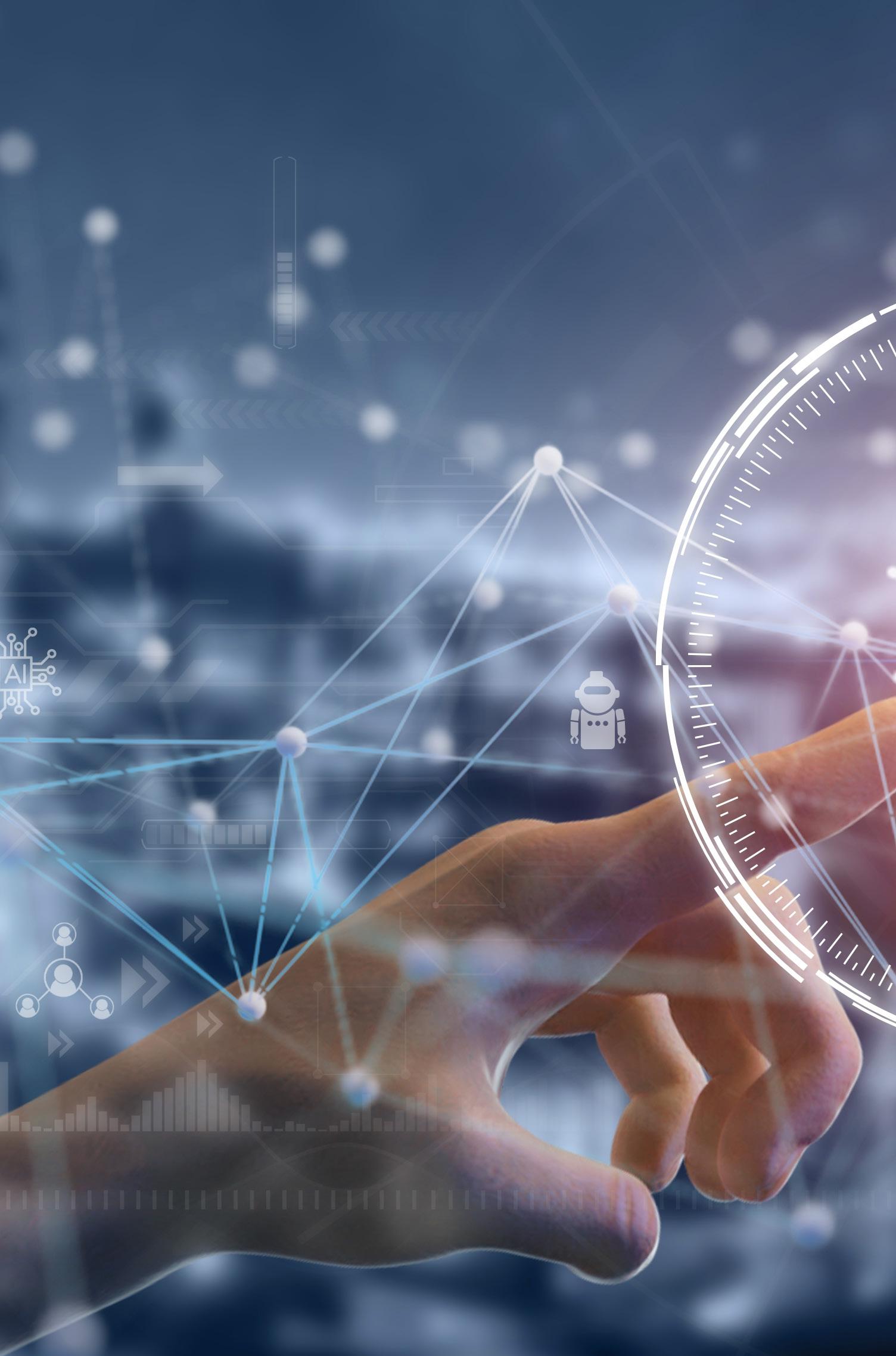
DeepSeek, the Chinese AI startup and developer of open-source large language models (LLMs) launched its third generation V3 LLM in December 2024. DeepSeek-V3, which is a mixture of experts (MoE) model that is benchmarking well against the best developed LLMs in the West and this month DeepSeek-R1, which is a reinforcement learning reasoning model that benchmarks well against OpenAI’s o1 generative pretrained transformer (GPT). V3 uses a MoE model taking several smaller models working together with a total of 671 billion parameters and only 37 billion active parameters at any given moment for each token during inferencing. V3 has further innovations such as multi-head latent attention (MHLA) reducing cache and memory size/usage, mixed precision computation on FP8 and a post-training phase re-architecture. Now MoE always looks more efficient as only a portion of the total parameters are active at any given point during token inferencing so that’s not overly surprising albeit V3 looks even more efficient, about 10x vs peers and 3-7x given other innovations. The DeepSeek-R1 model is claimed uniquely to have done away with supervised fine tuning. So there seems to be some innovation there, even if a lot of the headline improvements come from more standard techniques, while there is a wider debate on how much of the
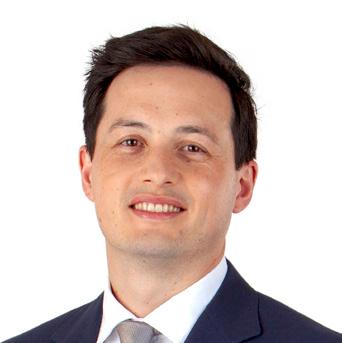
RICHARD CLODE
Janus Henderson Portfolio Manager, Richard Clode discusses the market’s current concerns around DeepSeek’s most recent LLM developments and what impact this may have on investors.
work DeepSeek has done themselves and how much is from leveraging open-source third-party LLMs.
3 KEY REASONS WHY THE MARKETS ARE CONCERNED WITH DEEPSEEK
1. DeepSeek appears to have significantly lower training costs DeepSeek claims to have trained V3 on only 2,048 NVIDIA H800 GPUs for two months, which at US$2 per hour explains the US$5 million total cost headline number announced. That is a fraction of what Western hyperscalers are throwing at their LLM training (eg. it’s 9% of the compute used for Meta’s LLaMA 3.1 405B model).
2. China can still compete despite US restrictions
DeepSeek shows that a Chinese company can compete with the US bestof-breed AI companies, despite the current restrictions on Chinese access to advanced US semiconductor technology. This evokes memories of a generation of Russian coders, who given restrictions on PC time in post-Soviet Russia, invented ingenious ways to code. Has the same thing happened in China where semi restrictions have forced greater LLM architecture innovation vs the US who
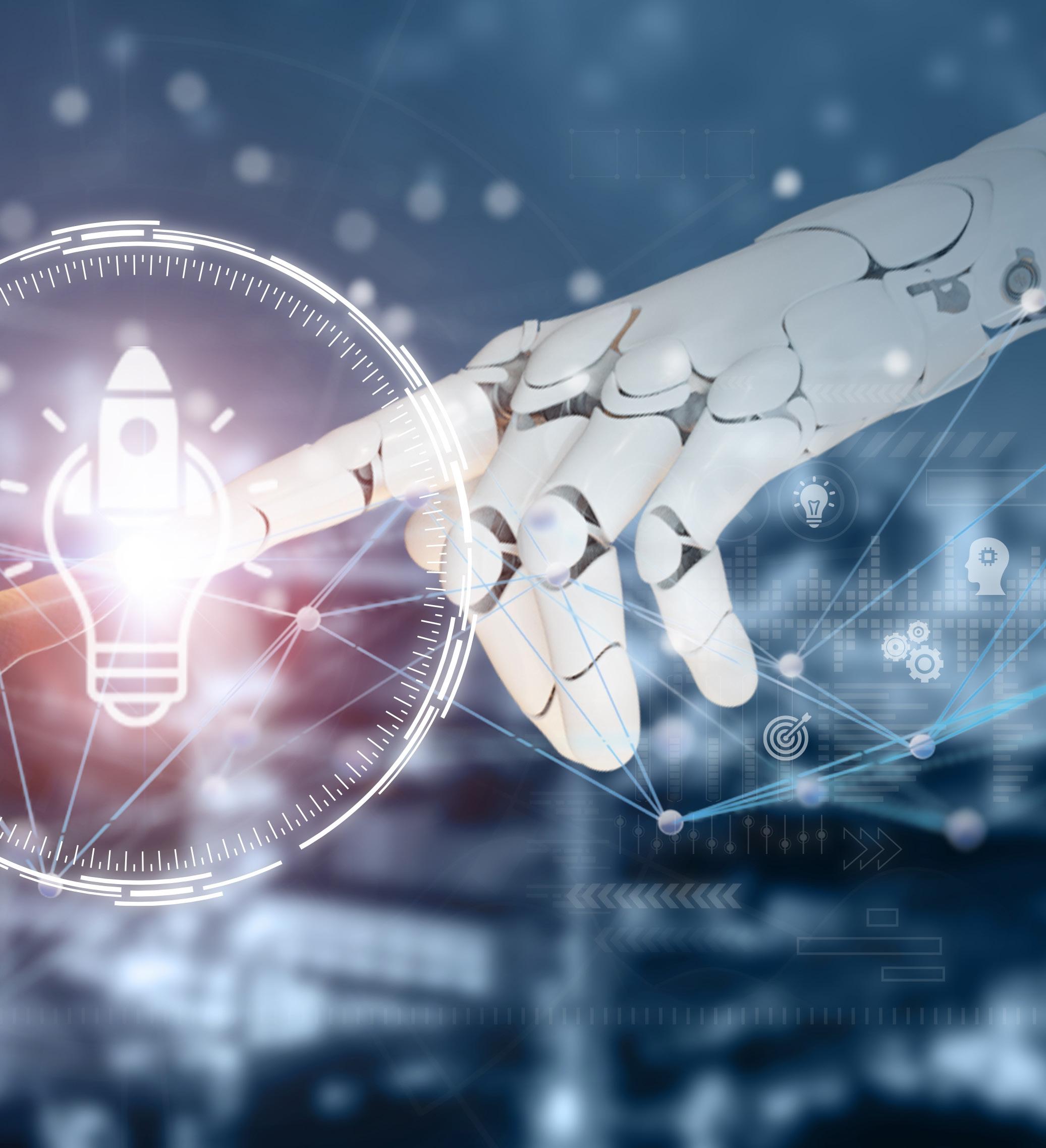
has just relied on throwing the compute kitchen sink at the problem?
3. AI monetisation
DeepSeek is charging significantly less than OpenAI to use its models (about 20-40x lower), which plays into the AI monetisation concern given the extraordinary amounts of capex deployed in the West.
A NOTABLE AI FORCE
The global AI ecosystem is taking note of DeepSeek’s developments. Despite only being launched two years ago (2023), DeepSeek benefits from the pedigree and backing of the team at quantitative fund High-Flyer Capital Management, as well as the success and innovation of its prior generation models. This is why while V3 was launched in December and R1 earlier this month, the market is only reacting now because R1’s reasoning capabilities are now viewed as cutting edge. Plus, over the last weekend DeepSeek became the top free app on Apple’s AppStore, overtaking ChatGPT. Silicon Valley investor Marc Andreessen posted that DeepSeek is “one of the most amazing and impressive breakthroughs I’ve ever seen,” which is high praise from a credible industry veteran. Comments like that have heightened the market’s concerns for the sustainability of AI capex and
associated companies like NVIDIA.
WHAT DO WE MAKE OF ALL THIS?
New technology waves require innovation
Any new technology wave requires innovation to drive down the cost curve over time to enable mass adoption. We are witnessing multiple avenues of AI innovation to address scaling issues with training LLMs as well as more efficient inferencing. DeepSeek appears to bring some genuine innovation to the architecture of general purpose and reasoning models. Innovation and the driving down of costs are key to unlocking AI and enabling mass adoption longer term.
Distillation
We are witnessing multiple avenues of AI innovation to address scaling issues with training LLMs as well as more efficient inferencing.
DeepSeek appears to bring some genuine innovation to the architecture of general purpose and reasoning models.
DeepSeek’s model leverages a technique called distillation, which is being pursued more broadly in the AI industry. Distillation refers to equipping smaller models with the abilities of larger ones, by transferring the learnings of the larger, teacher model into the smaller, student one. However, it is important to note DeepSeek’s distillation techniques are reliant on the work of others. Exactly how reliant is a key question the market is grappling with currently.
Take the capex number with a pinch of salt
Related to the above, the capex numbers referred to are just comparing apples to oranges. The US$5 million cited relates to just one training run, ignoring any prior training runs and the training of the larger teacher models, whether at DeepSeek or the third-party open source LLMs they were built on.
Open source innovation
As AI luminary Yann LeCun has noted, this is a victory for the open source model of driving community innovation with DeepSeek leveraging Meta’s Llama and Alibaba’s Qwen open source models. Again this is positive for the longer-term development of AI, driving and proliferating innovation. However, due to the current state of geopolitics one would probably expect greater US government scrutiny on other countries accessing state of the art AI LLMs from the US.
LLMs commoditising?
It has long been our belief that monetising LLMs in the longer term will be challenging given the volume of competition, including from open source developers and competitors looking to monetise in alternative ways.
Three lenses for AI adoption in a post-hype world
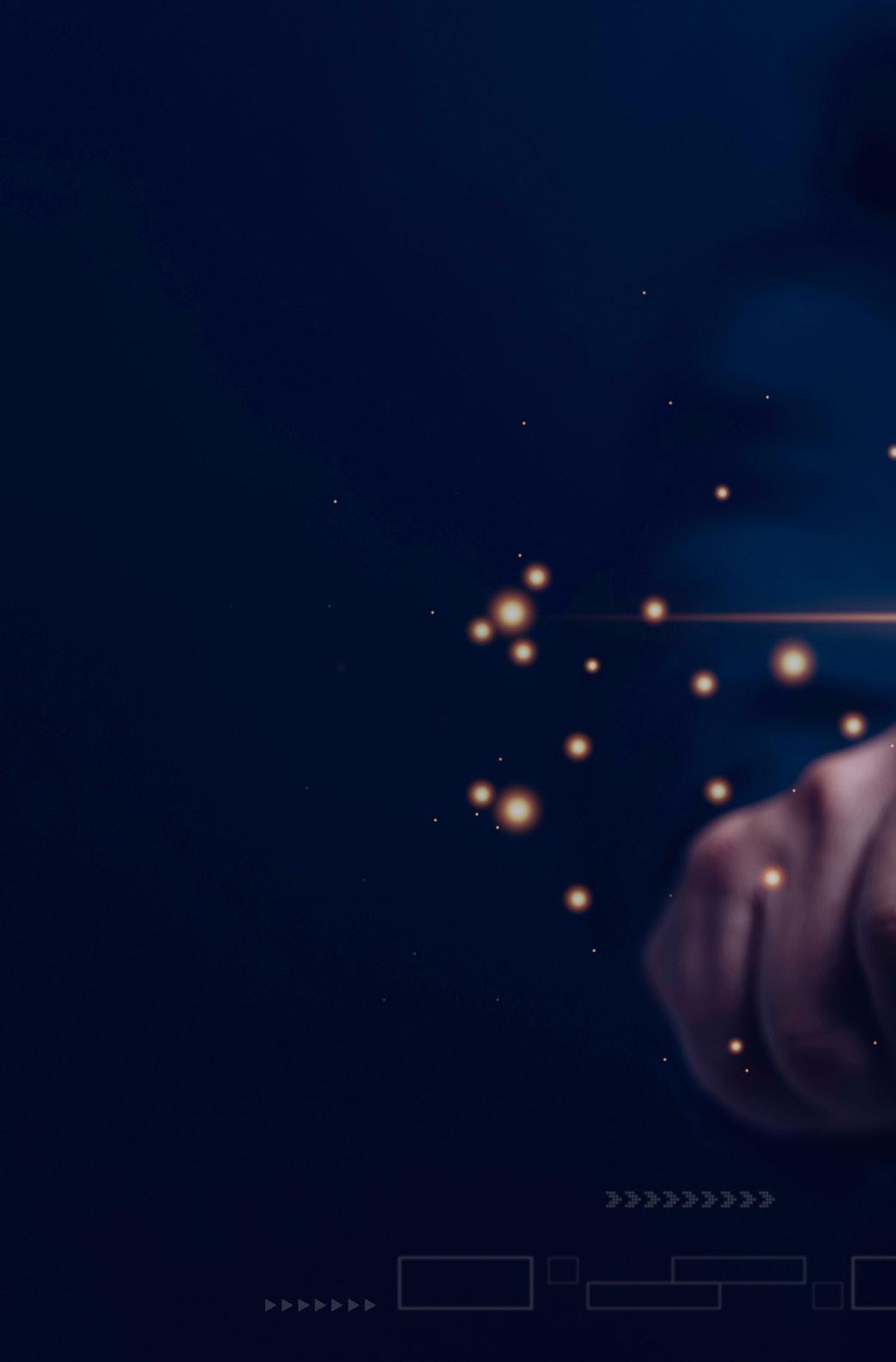
A little over two years on from the launch of ChatGPT and there has been a monumental shift in awareness, activity and desire to implement AI and GenAI projects. Decisions are still driven by FOMO (Fear Of Missing Out) and fear of falling behind competitors, as organizations want to explore the options and business leaders from all areas want to capitalize on the excitement to push through new initiatives.
The potential benefits often seem to outweigh the risks and lack of direction from AI projects: “It’s exploratory! Of course we don’t know what the results will be!” This has led to AI projects starting without deep consideration into what the end result will look like — what success is and how a team can take that project forward and implement it to business benefit.
Because of this profligate approach, we have reached the peak of frenzied, hype driven decision making when many projects have been green-lit without long term thought on strategy and ROI.
HOW TO IMPLEMENT AI WHILE MAINTAINING BUSINESS OVERSIGHT
While AI continues to be a magnet for business budgets, enterprise adoption remains in the early stages of development and implementation. Because we are
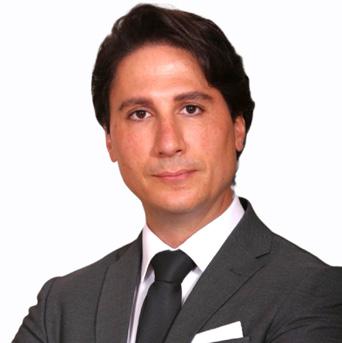
OMAR
AKAR
Omar Akar, Regional VP for META & CEE at Pure Storage, explores how businesses can pare the AI fat
past the point of easy access to funding, organizations need to ensure an AI project delivers real ROI and isn’t driven by FOMO.
In order to implement an AI initiative with business impact, here are three considerations for success:
LENS ONE: CONSIDER THE COST AND DON’T GET TIED IN When the AI boom happened there were many market drivers to start new projects. Budgets were redirected to implement AIdriven initiatives within businesses — cost wasn’t the primary concern. However, this excitement and free spending has recently been reigned in as more organizations want to keep a handle on costs and understand where the ROI is coming from.
When embarking on an AI journey, key considerations include how to experiment without committing funding to a project that may not succeed; being able to scale up or down ensures organizations are not at risk of writing off expenses. Storage and computing resources are central to AI initiatives, ensuring these are fully utilized is key to maximizing the return on investment. If GPUs are bought, but not used for 18 months, that’s an expensive resource to be sitting idle. Better to have the ability to scale up and down to keep costs under control and deliver long term value.
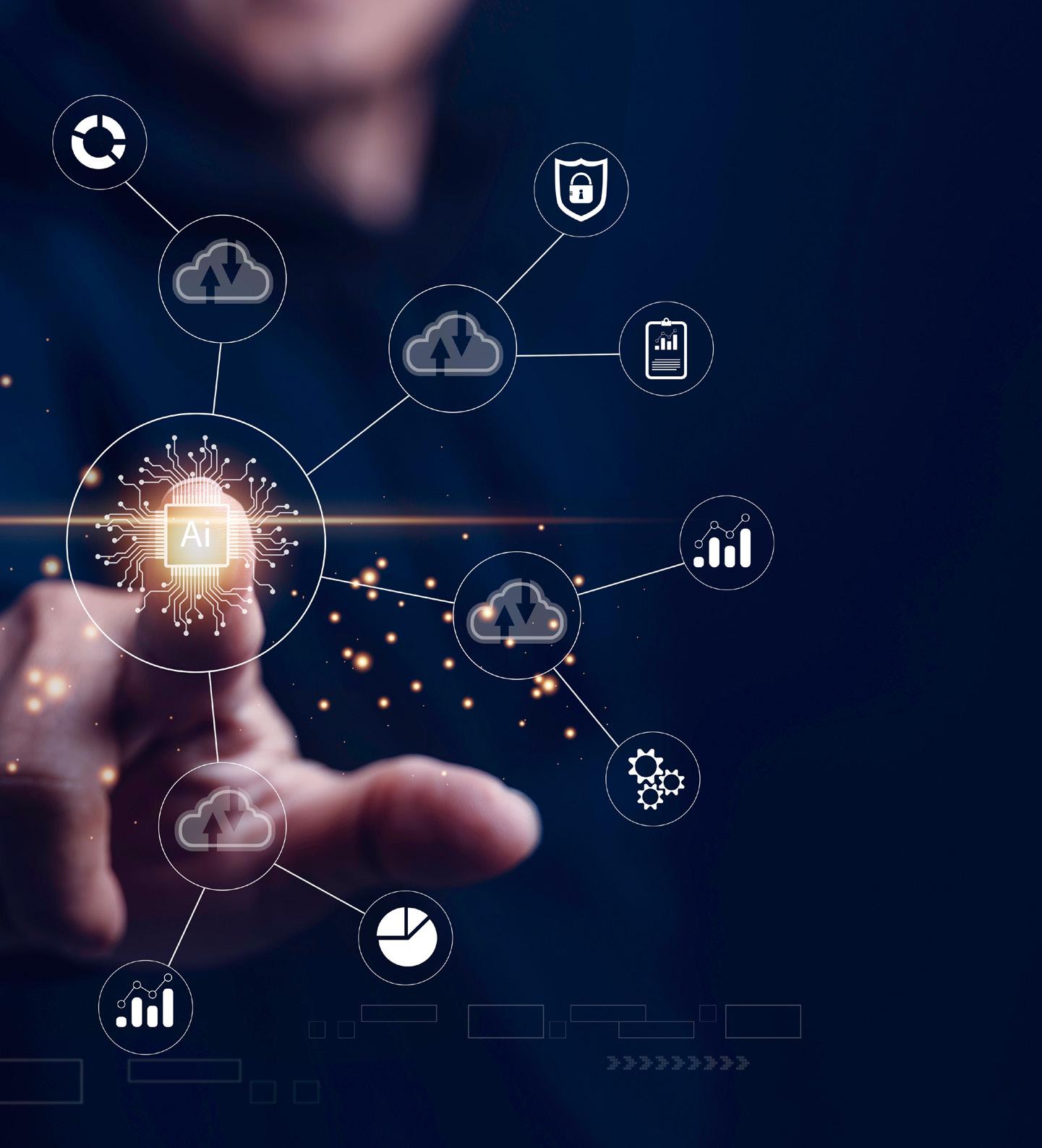
LENS TWO: WHAT’S THE SCOPE AND SCALE, AND IS IT LIKELY TO CHANGE?
If an AI project is an experiment — there’s likely to be uncertainty of how big it could grow. Both at the trial phase and looking further down the line to full scale implementation. Being able to grow from POC, through deployment, and then support a successful project is vital. Organizations need to be able to scale without having to throw away computing resources and start again. In this respect, the cloud is a good place to POC, to experiment and tweak, find out the potential, map the business value and then plan for the future. But, it can be expensive meaning that a successful project may lead to unsustainable costs.
The reality (which more and more are admitting to now) is that organizations don’t know where a project will go in the next 6-12 months. There are many directions and what success looks like varies. Not only is there business uncertainty, there’s the regulatory landscape, security considerations, customer expectations and requirements. While it could be a limitation to not know what the future holds, for organizations who have flexibility — it isn’t going to be an issue.
Consider as-a-service models, which take the guess work out of consumption. Given AI’s dependency on data, one of
the key capabilities that can support this uncertainty is a Storage as-a-Service model underpinned by a physical platform which can be scaled to deliver performance, throughput and capacity non-disruptively with instant impact. It takes the guess work out and allows organizations to react very quickly to changing workloads. This applies not only to scaling up, but also back down. This is really appealing to organizations as they experiment but who don’t want to pay for infrastructure that isn’t being used.
LENS THREE: SYSTEM RELIABILITY
Projects which don’t have a clear path to success or have no proper business case for implementation and success are being halted, or not started. Moving forward, there will be a lot more introspection before people give free reign on budgets for AI projects.
For many organizations, once projects get past the exploratory stage, senior leaders are looking for ways to turn a project into a business critical service. For these situations, many will be looking for reliability and 100% uptime. But considering banks or online retail — customer facing applications are required to be reliable and available 24/7. These cannot be built on weak foundations. For reputational and customer retention reasons, not to mention regulation and cyber resilience, organizations can’t tolerate failure in their systems. It’s unacceptable to not have 100% uptime, so organizations should look to vendors who have the maximum reliability.
CALLING TIME ON PROFLIGATE PROJECT FUNDING
Not all AI implementations are profligate of course, but senior leaders have started calling time on the ones which are. Many wanted to start an AI project and thought it was business critical to do so. But bosses are no longer willing to hand out money in the hope of success.
Projects which don’t have a clear path to success or have no proper business case for implementation and success are being halted, or not started. Moving forward, there will be a lot more introspection before people give free reign on budgets for AI projects.
It’s time organizations have a back to basics approach to funding, rather than decisions being driven by FOMO. Consider what’s the business impact; what’s the chance of success — the likelihood of the project completing and delivering ROI; what’s the strategy behind the project; how will it improve customer interactions or processes; does the team have the necessary data? These are the questions that need to be asked before embarking on and funding a new AI journey.
GUEST ARTICLE
Modernisation without risk
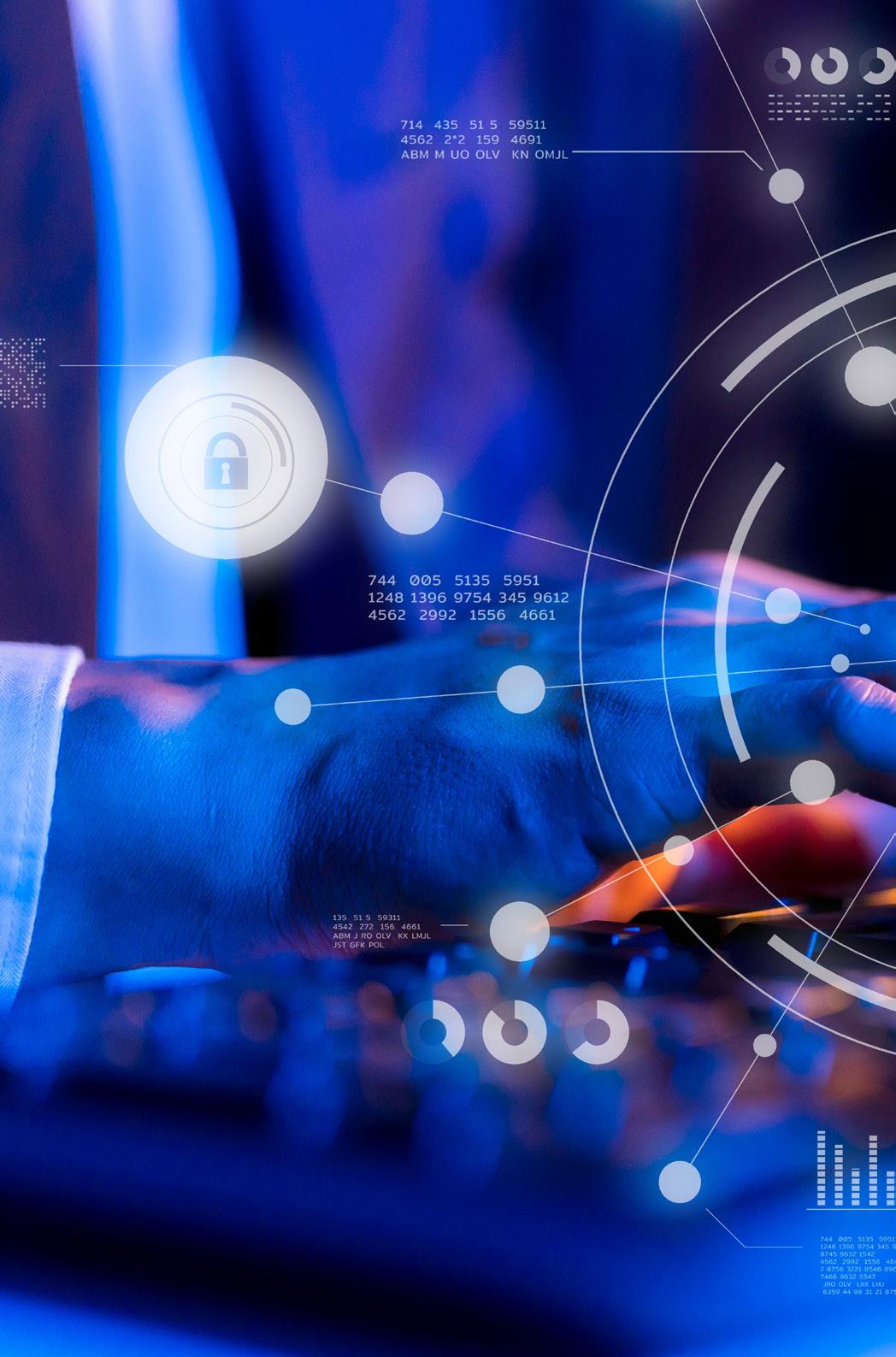
The new year offers the perfect moment to reflect on the past and shape the future. What insights can guide us forward? What strategies will drive success? Which fleeting trends will fade, and which transformative forces will demand our focus? Amongst these, artificial intelligence stands as a pivotal opportunity that businesses can no longer afford to overlook.
Around the world, a wave of quick-fire AI implementations has emerged, driven by the urgency to gain first-mover advantage. Yet, this rush has sparked a new and essential conversation: how can businesses harness AI responsibly, ensuring compliance with data regulations? The reality is that even leveraging first-party data effectively may call for a comprehensive modernisation of core systems. In many ways, this marks a new era of digital transformation — one that balances technological advancement with ethical responsibility.
THE CHANGE BEFORE THE CHANGE
In many cases, AI has been layered onto existing systems as a quick fix. While this approach might work temporarily, it falls short of laying a foundation robust enough to support AI’s full potential. Effective AI
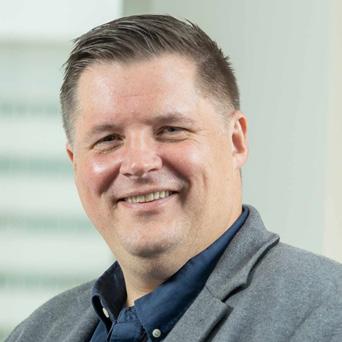
DAVID BOAST
David Boast, General Manager – UAE & KSA, Endava, on how to tread the elusive optimal path to AI adoption
adoption requires more than patchwork solutions; it demands an infrastructure designed for scalability, agility, and governance.
An AI-ready business must be prepped to adapt quickly to shifting market dynamics, customer demands and emerging technologies. This begins with a deep understanding of workflows, business logic and operational nuances that may be obscured by legacy tech sprawl. Leaders must prioritise data governance, invest in modular architectures for rapid deployment and establish systems capable of managing AI’s demands over the long term.
While modernisation is a cornerstone of keynote speeches and whitepapers, CTOs know that it can be fraught with risk. Tacking AI onto legacy systems might seem like a lower-risk option but is often a false economy. Clean data and modular building capabilities must come first. The challenge, therefore, is to find ways of making changes that minimise service disruption.
For incumbent GCC businesses, this urgency is compounded by competitive pressures. The plain fact is that these businesses are prone to disruption by wellfunded startups that do not have to tear down old systems to be AI-ready. But with a
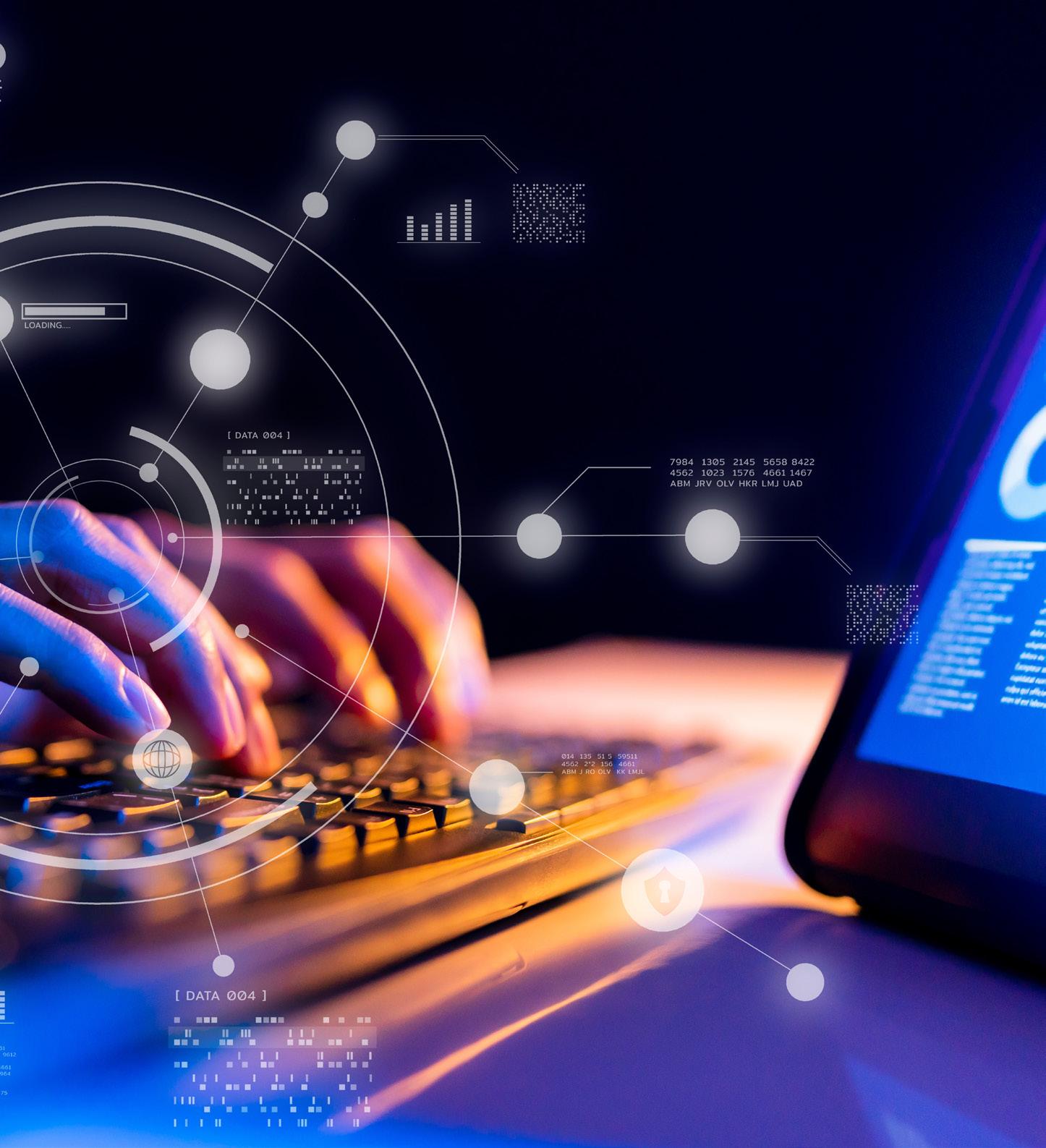
data-based approach and the right expertise, established organisations can rise to the challenge of modernising their core systems without derailing operations, in preparation for AI-driven growth.
BUILDING THE FOUNDATION FOR SUCCESS
Start with the business fundamentals. Understand yourself before trying to understand AI. From this starting point, methods for clearly capturing data will emanate from the way the business operates day to day. Composable architecture comes next; rapid feature deployment is a must in a digital economy. Finally, if the business can dig into its existing systems to understand its workflows and business logic, then the fundamentals are in place. The change before the change is complete. Subsequent modernisation will be more transparent and flexible, leading to reduced risk.
Milestones provide confidence and clarity along this journey. A move to the cloud might come first, or perhaps you will choose to rewrite your application architecture. Whatever foundations you build, AI will sit on a far firmer base than if simply tacked onto a legacy stack. Blending the strengths of technology and people, regional
Essentially, the organisation uses advanced AI in controlled circumstances to pave the way for embedding AI deeper into the fabric of business.
organisations can take deep analytical dives into legacy assets. Tools even exist to offer data-driven automation of this process, backed by the right skillsets, to deliver a derisked, cost-controlled, modernisation process. Essentially, the organisation uses advanced AI in controlled circumstances to pave the way for embedding AI deeper into the fabric of business.
This core modernisation is, remember, only a means to an end. In the GCC, where bold innovation is the norm, businesses already understand the power of AI. Governments and businesses here are practiced early adopters, so they have many lessons to draw on. They know that adoption of a new technology often requires fundamental shifts in infrastructure, business processes and even corporate culture. AI is a manyheaded beast capable of fulfilling a rich menu of use cases. Your organisation has to consider its industry, workforce, operating capital, growth ambitions and more. It must grapple with the eccentricities of its people, shareholders and market. And it must be clear-eyed about where it wants AI to take it and how to undertake the journey with the lowest possible risk.
As is abundantly evident at this point, embracing AI will no longer be optional for GCC businesses. To remain competitive, organisations must modernise their core systems and infrastructure, prioritising clean data and composable architecture. This foundation will enable seamless integration of AI, unlocking its potential while minimising risks. With the region’s history of bold innovation and a readiness to embrace transformative technologies, the GCC is well-positioned to lead in this new digital era. This is a pivotal moment for organisations to align bold technological ambitions with solid execution, paving the way for long-term success.
Tackling agentic AI’s biggest hurdles
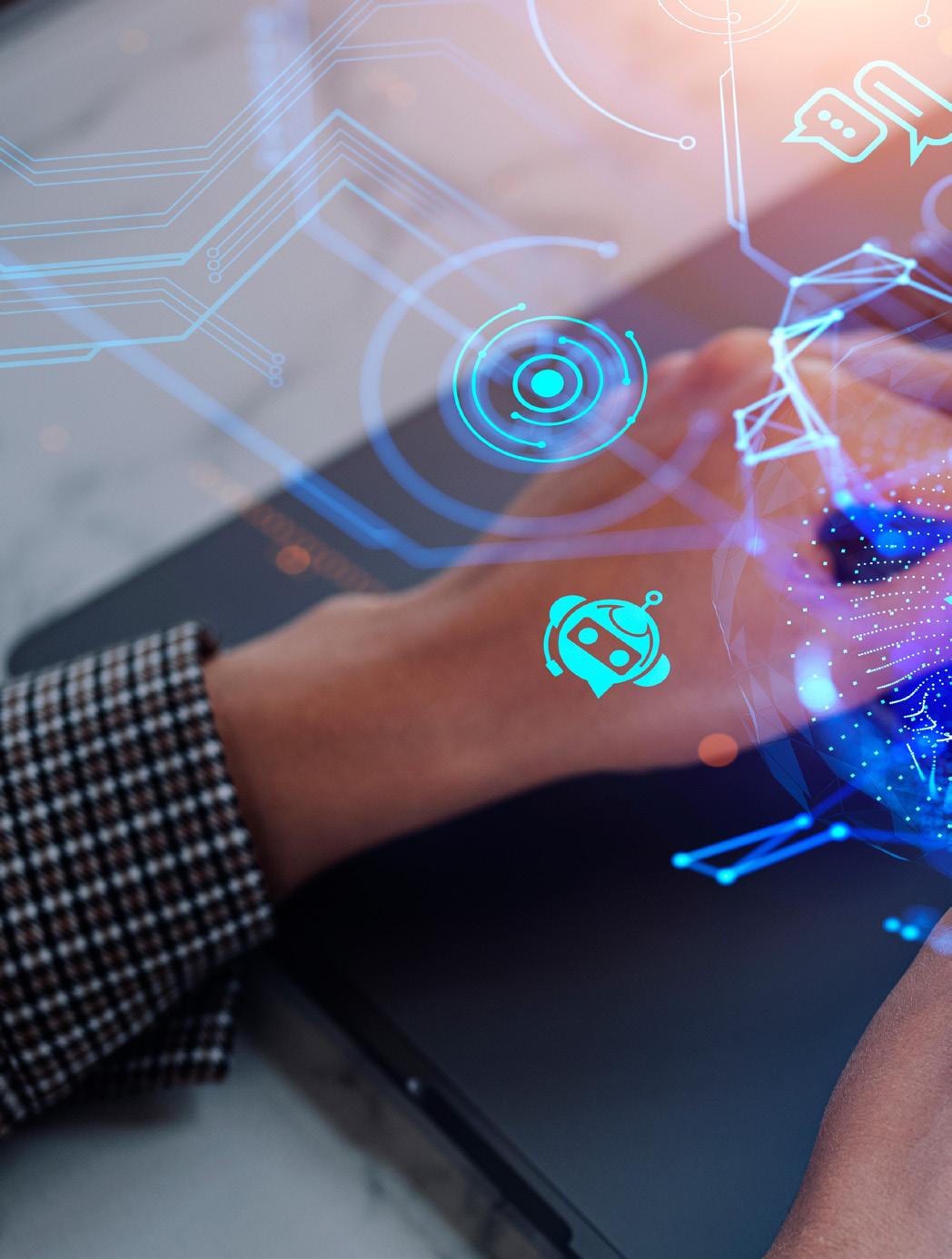
As generative AI continues its unprecedented surge in popularity, we are already seeing it evolve into the next generation of machine-learning-driven technologies: agentic AI. With agentic AI, we are not just prompting models and receiving an answer in a simple one-step process. The AI is engaging in complex multi-step processes, often interacting with different systems to achieve a desired outcome. For example, an organization could have an AIpowered help desk with agents that use natural language processing to understand and process incoming IT support tickets from employees. These agents could autonomously reset passwords, install software updates, and elevate tickets to human staff when necessary.
Agents will be one of the most significant innovations in the AI industry, possibly more than future generations of foundation models. By 2028, Gartner predicts that at least 15% of day-to-day work decisions will be made autonomously by agentic AI, up from 0% in 2024.
However, while AI agents can improve efficiency, save costs and free up IT staff to focus on more critical projects that need human reasoning, they’re not without challenges. Before deploying agentic AI, enterprises should be prepared to address several issues that could otherwise impact the trustworthiness and security of the systems and outputs.
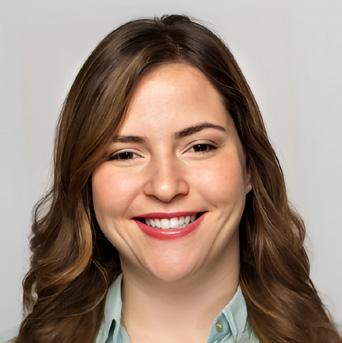
ADI POLAK
Director of Advocacy and Developer Experience Engineering, Confluent, lists out the top 5 agentic AI challenges and how to overcome them.
MODEL LOGIC AND CRITICAL THINKING
With agentic AI, one agent acts as a “planner” and orchestrates the actions of multiple agents. The model provides a “critical thinker” function, offering feedback on the output of the planner and the different agents that are executing on those instructions. The more feedback created, the more insights the model has, and the better the outputs will be.
For this to work well, the critical-thinker model needs to be trained on data that’s as closely grounded in reality as possible. In other words, we need to give it a lot of information on specific goals, plans, actions, and results, and provide a lot of feedback. This can require many iterations — running through hundreds or even thousands of plans and results — before the model has enough data to start acting as a critical thinker.
RELIABILITY AND PREDICTABILITY
The way we interact with computers today is predictable. For instance, when we build software systems, an engineer sits and writes code, telling the computer exactly what to do, step by step. With an agentic AI process, we’re not telling the agent what to do step-by-step. Rather, we lead with the outcome we want to achieve, and the agent determines how to reach this goal. The software agent has a degree of autonomy, which means there can be some randomness in the outputs.
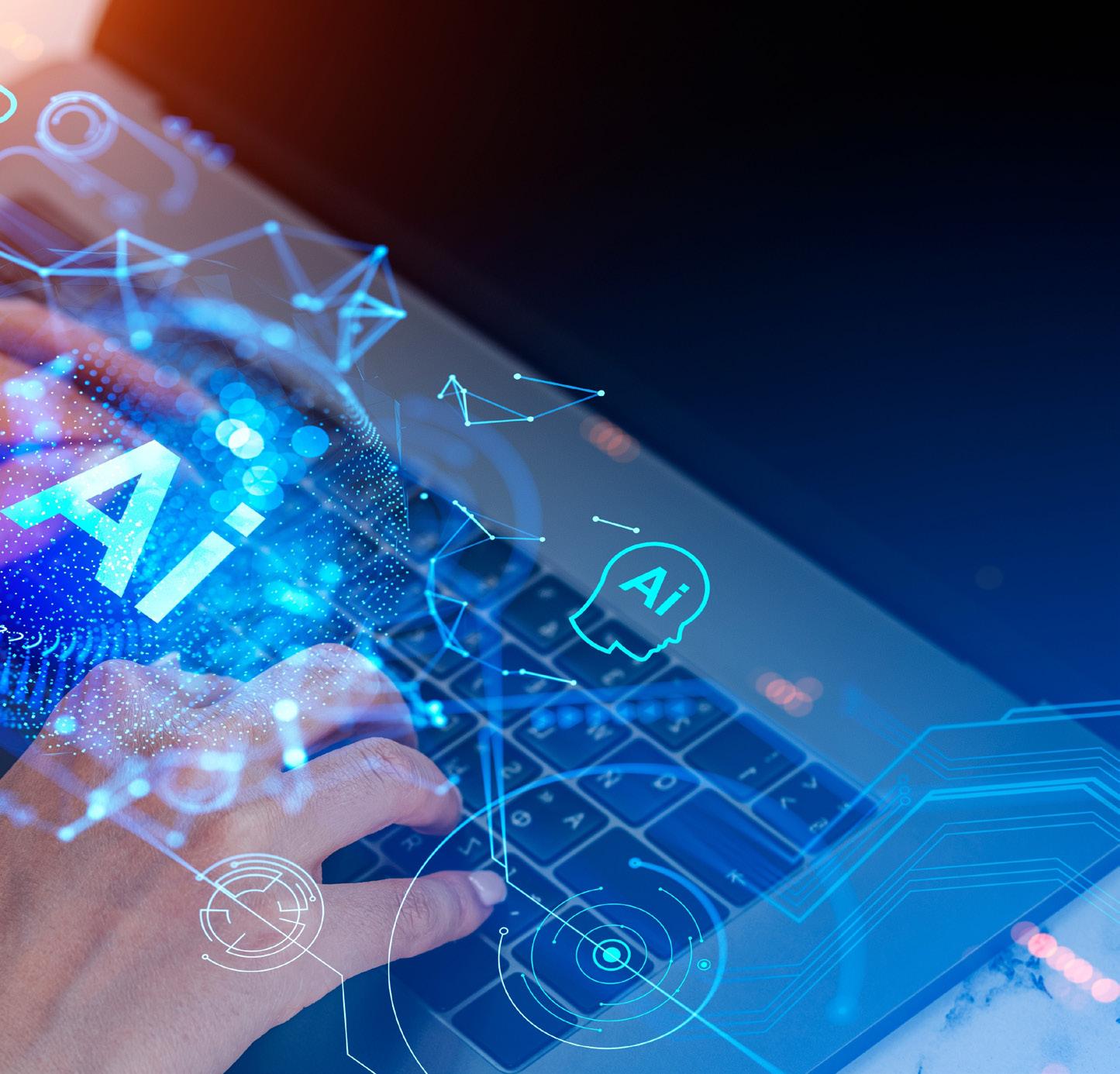
DATA PRIVACY AND SECURITY
Some companies are hesitant to use agentic AI due to privacy and security concerns, which are similar to those with generative AI but can be even more concerning. For example, when a user engages with an LLM, every bit of information given to the model becomes embedded in that model. There’s no way to go back and ask it to “forget” that information. Some types of security attack, such as prompt injection, take advantage of this by trying to get the model to leak proprietary information. Because software agents have access to many different systems with a high level of autonomy, there is an increased risk that it could expose private data from more sources.
To address this problem, companies need to start small, containerizing the data as much as possible, to ensure it is not being exposed beyond the internal domain where it is needed. It’s also critical to anonymize the data, obscuring the user and stripping any personally identifiable information (like social security numbers or addresses) from the prompt, before sending it to the model.
At a high level, we can look at 3 different types of agentic AI systems and their respective security implications for business use:
1 - Consumer agentic AI - Typically an internal user interface with an external AI model. As a company, we have zero control over the AI itself, only over the data and prompts we send.
2 - Employee agentic AI - Built internally
for internal usage. While there is less risk, this still poses a risk of exposing highly private information to non-qualified users in the company. For example, companies can decide to build their own GPT-like experience for internal use only.
3 - Customer-facing agentic-AI - Built by a business to serve its customers. Since there is some risk with interacting and working with customers, the system must have good segmentation to avoid exposing private customer data.
DATA QUALITY AND RELEVANCY
The way we interact with computers today is predictable. For instance, when we build software systems, an engineer sits and writes code, telling the computer exactly what to do, step by step.
Once the data and the user have been anonymized, we want our agentic model to deliver results that are grounded in quality data that is relevant to the user’s prompt. That will be a significant challenge. Too often, generative AI models fail to deliver the expected results because they are disconnected from the most accurate, current data. Agentic AI systems face additional issues because they will need to access data across a wide variety of different platforms and sources.
A data streaming platform (DSP) can be useful here because it provides engineers with tools to enable relevant answers using high-quality data.
ROI AND TALENT
AI is still new territory for many companies, requiring them to purchase new hardware and GPUs, and create a new data infrastructure, particularly with new memory management for caching and for shortterm and long-term storage. It also requires building an inference model in-house. To do this, they’ll need to hire new talent with these specialized skills or train existing workers on AI. Return on investment will take time, especially for early adopters.
Despite these hurdles, agentic AI will spread through enterprises much like generative AI has. We’re already seeing some AI technology vendors move in this direction.
Before companies see the benefits from agentic AI, they will need to be prepared to resolve the issues of reliability, data privacy and quality, and model logic. They will also need to be prepared for significant investments up-front. However, the potential impact on the business may be much greater than what they’re seeing with just generative AI.
CHANGING PRIORITIES
NUTANIX STUDY REVEALS THAT GENAI APPLICATION ADOPTION IS DRIVING A NEW ERA OF APPLICATION AND INFRASTRUCTURE MODERNIZATION.
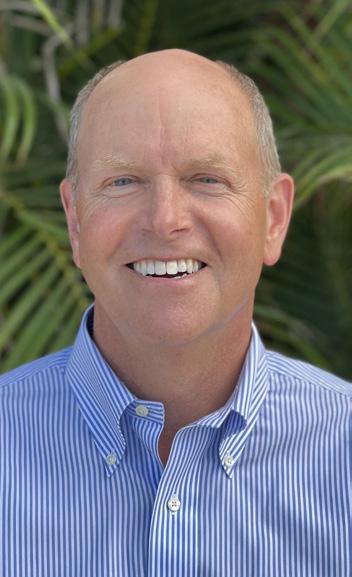
LEE CASWELL
SVP, PRODUCT AND SOLUTIONS MARKETING AT NUTANIX
Nutanix has announced the findings of its seventh annual Enterprise Cloud Index (ECI) survey and research report, which measures global enterprise progress with cloud adoption. This year’s report sheds light on Generative Artificial Intelligence (GenAI) adoption, investment priorities, and benefits along with key challenges organizations face to meet the demands of these emerging workloads.
As GenAI application adoption and implementation move at a blazing pace, the ECI uncovered that while the majority of organizations have already implemented a GenAI strategy, implementation targets vary significantly. Organizations are eager to leverage GenAI for productivity, automation, and innovation, but they also face critical hurdles in the form of data security, compliance, and IT infrastructure modernization. Further, 90% of respondents expect their IT costs to rise due to GenAI and modern application implementation. But promisingly, 70% of organizations expect to make a return on their investment from GenAI projects over the next two to three years.
“Many organizations have reached an inflection point with GenAI implementation and deployment,” said Lee Caswell, SVP, Product and Solutions Marketing at Nutanix. “This year’s ECI revealed key trends that we’re hearing from customers as well, including challenges with scaling GenAI workloads from development to production, new requirements GenAI creates for data governance, privacy, and visibility, and integration with existing IT infrastructure. To successfully unlock ROI with GenAI projects, organizations need to take a holistic approach to modernizing applications and infrastructure and embrace containerization.”
Key findings from this year’s report include:
• Application containerization is the new infrastructure standard. Nearly 90% of organizations report that at least some of their applications are now containerized, and this number is expected to grow with the rapid adoption of new application workloads like GenAI. Simply put, 94% of respondents agree that their organization benefits from adopting cloud native applications/containers. This approach to infrastructure and application development should be considered the gold standard for delivering seamless, secure access to data across hybrid and multicloud environments.
• GenAI application adoption and implementation continue at a rapid pace. Over 80% of organizations have already implemented a GenAI strategy with only 2% of organizations admitting that they have not started planning their GenAI strategy. That said, implementation targets vary significantly. Most organizations believe GenAI solutions will help improve their organization’s levels of productivity, automation, and efficiency. Meanwhile, real-world GenAI use cases gravitate towards customer support and experience solutions today. However, organizations aspire to apply GenAI solutions to cybersecurity and data protection workloads in the near future.
• GenAI adoption will challenge traditional norms for data security and privacy. 95% of respondents agree that GenAI is changing their organization’s priorities, with security and privacy being a primary concern. Over 90% of organizations say data privacy is
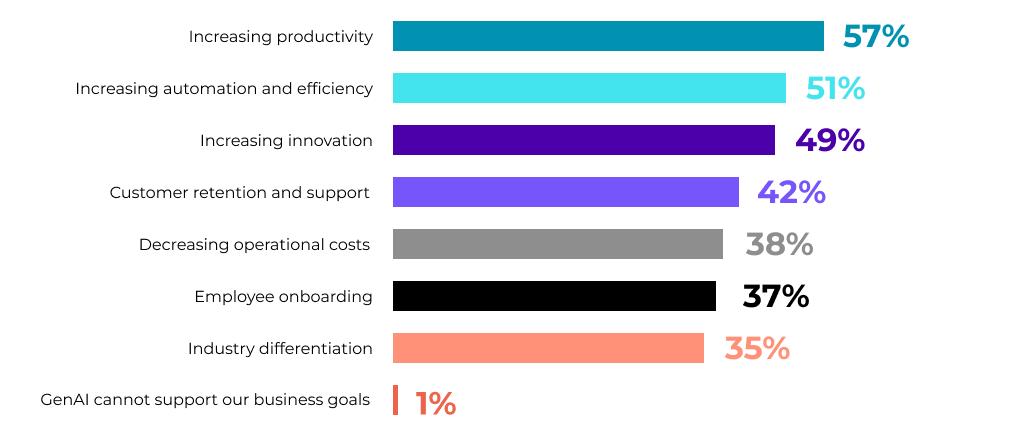
a priority for their organization when implementing GenAI solutions. Clearly, organizations understand that security and privacy are critical components of GenAI success. However, a staggering 95% of respondents still believe their organization could be doing more to secure its GenAI models and applications. Security and privacy will remain a major challenge for organizations as they seek to justify the use of emerging, GenAI-based solutions and ensure that they adhere to traditional security norms, as well as new requirements for data governance, privacy, and visibility.
• Infrastructure modernization to support GenAI at scale. Running cloud native applications at enterprise scale requires an infrastructure that can support the necessary requirements including security, data integrity and resilience. Emerging GenAI applications are no exception to this rule. Almost all respondents (98%) face challenges when it comes to scaling GenAI workloads from development to production. In fact, the #1 challenge organizations face when scaling GenAI workloads from development into production is integration with existing IT infrastructure. As a result, IT Infrastructure was chosen as the #1
area of investment needed to support GenAI.
• GenAI solution adoption requires changes to technology and people 52% of respondents say their organization needs to invest in IT training to support GenAI. Similarly, 48% of respondents believe their organization needs to hire new IT talent to support GenAI. There is no denying organizations face acute skills shortages and competition for GenAIrelated talent. The good news? Many teams will embrace the challenge to adopt AI-related competencies and skills organically, as part of normal work. This year’s survey shows that 53% of respondents believe advancements in GenAI will provide them with an opportunity to become an AI expert.
For the seventh consecutive year, Nutanix commissioned a global research study to learn about the state of global enterprise cloud deployments, application containerization trends, and GenAI application adoption. In the Fall of 2024, U.K. researcher Vanson Bourne surveyed 1,500 IT and DevOps/ Platform Engineering decision-makers around the world. The respondent base spanned multiple industries, business sizes, and geographies, including North and South America; Europe, the Middle East and Africa (EMEA); and Asia-Pacific-Japan (APJ) region.
BALANCING INNOVATION WITH ETHICAL CONSIDERATIONS
AT A RECENT EVENT IN DUBAI, CO-ORGANIZED BY QLIK AND COGNIZANT, CEOS AND DECISION-MAKERS EXPLORED THE TRANSFORMATIVE POTENTIAL OF ARTIFICIAL INTELLIGENCE (AI) AND THE ETHICAL CHALLENGES IT PRESENTS.
MEMBER OF QLIK’S AI COUNCIL AND COFOUNDER OF THE AI ASIA PACIFIC INSTITUTE
Kelly Forbes, a member of Qlik’s AI Council and Co-Founder of the AI Asia Pacific Institute, highlighted the importance of balancing innovation with responsibility as AI continues to reshape industries and
AI’s dual role as a driver of progress and a source of challenges was a central theme. While it promises to revolutionize sectors like healthcare, sustainability, and economic productivity, concerns such as job displacement, ethical biases, and unequal access to technology remain critical. Forbes emphasized the need for proactive strategies to address these challenges while unlocking
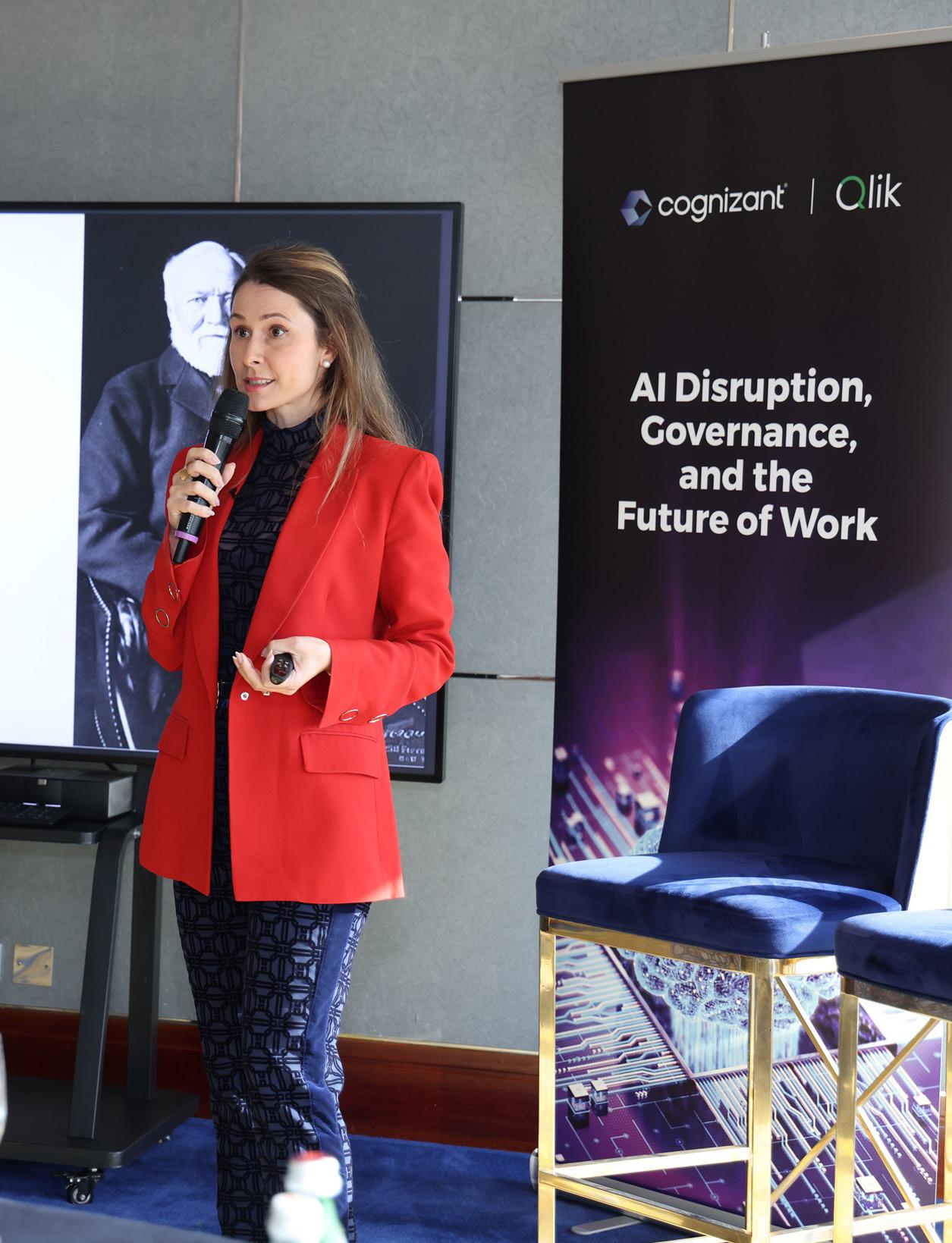
AI is reshaping industries at an unprecedented pace, comparable to the societal shifts of past industrial revolutions but on a much larger scale. Its ability to process and analyze vast amounts of data in real time allows organizations to make faster, more informed decisions. From automating customer service processes to enabling life-saving diagnostics in healthcare, AI’s applications span nearly every sector, offering immense opportunities for innovation.
However, this transformation comes with disruption. AI-driven automation has the potential to boost productivity and free workers from repetitive tasks, fostering opportunities for more complex and creative roles. Yet, CEOs and decision-makers at the event acknowledged the uncertainty
that comes with these advancements. Key considerations include:
• How can businesses prepare their workforce for AI-driven transformations?
• What strategies can address the societal impacts of job displacement?
• How can innovation be accelerated while ensuring ethical implementation? These questions reflect a shared concern: progress must not leave people behind.
LEARNING FROM HISTORY TO TACKLE GLOBAL CHALLENGES
Drawing on lessons from previous industrial revolutions, businesses and policymakers can navigate the current AI revolution with a focus on ethical responsibility. AI presents a unique opportunity to address some of the world’s most pressing challenges, aligning with the United Nations’ Sustainable Development Goals (SDGs).
The UAE serves as a notable example of AI-powered progress. Through smart city initiatives, AI-driven energy optimization, and investments in green technology, the country showcases how AI can contribute to sustainability and resource management. In healthcare, AI enables faster diagnostics, improved treatment plans, and expanded access to medical expertise in underserved regions. From disease prevention to advancements in education and agriculture, AI has the potential to bridge critical gaps.
THE CHALLENGES: ETHICAL CONCERNS, BIAS, AND INEQUALITY

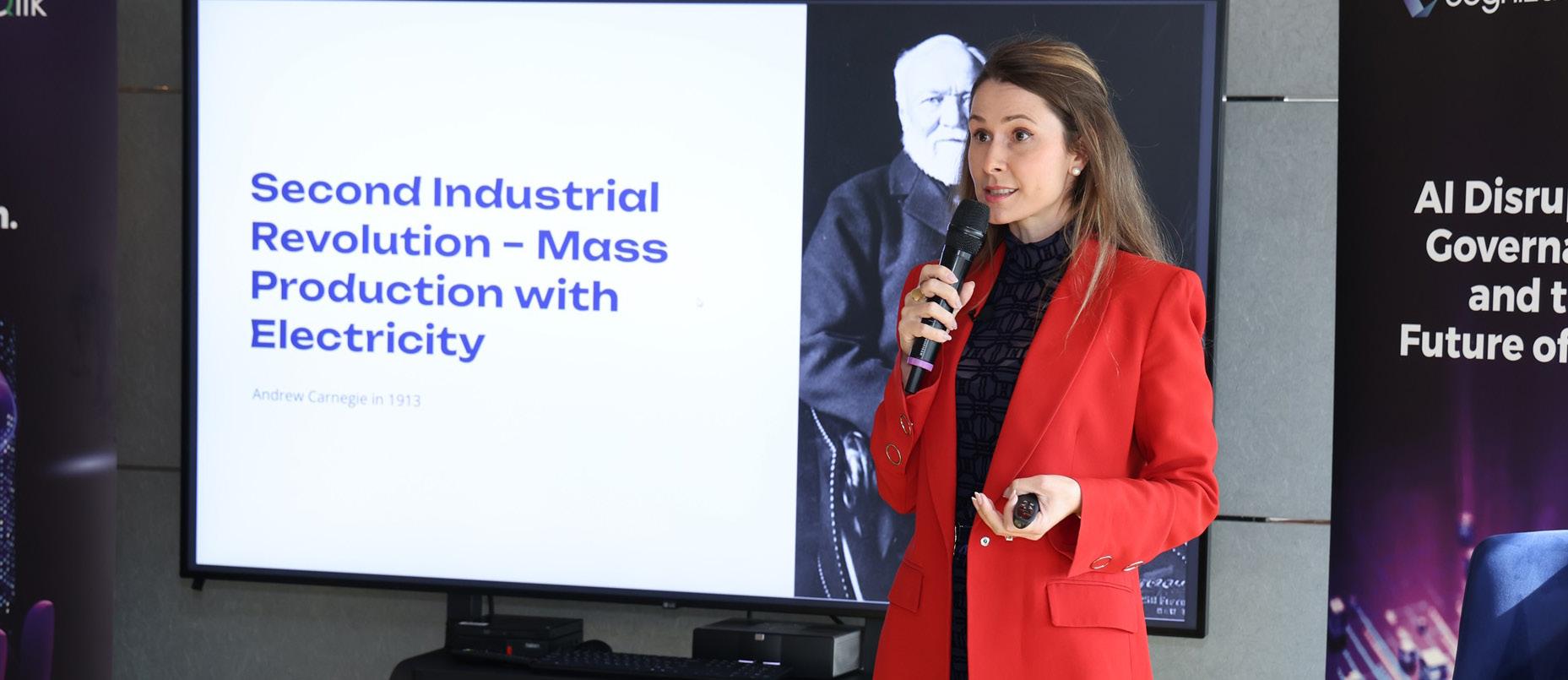
Despite its promise, AI adoption comes with significant challenges that leaders cannot afford to ignore.
1. Bias in AI Systems: AI algorithms are only as fair as the data they are trained on. When datasets contain historical biases, AI can unintentionally perpetuate social inequalities. For example, recruitment tools may favor certain demographics, and facial recognition systems have exhibited racial biases. Addressing these issues requires rigorous data governance and ongoing system audits.
2. Job Displacement and Workforce Readiness: AI-driven automation threatens traditional roles, particularly in manufacturing, retail, and customer service. While businesses can benefit from productivity gains, leaders must prioritize strategies to upskill employees and create pathways for displaced workers to transition into new roles.
3. The Digital Divide: Many developing regions lack the infrastructure to harness AI’s benefits. This growing digital divide risks exacerbating global inequalities. To ensure inclusive progress, investments in technology infrastructure and digital literacy must become a priority. These ethical, economic, and infrastructural concerns formed a critical part of the discussions at the event, with delegates
highlighting the need for collaboration across sectors to address these barriers.
CEOs and decision-makers must approach AI adoption with a clear strategy that prioritizes both innovation and responsibility. Key reflections from the event include:
• Invest in Responsible AI: Companies should focus on building AI systems that are transparent, interpretable, and accountable.
• Prioritize Workforce Development: Investing in reskilling initiatives ensures that employees are equipped for AIdriven roles.
• Foster Collaboration: Policymakers, businesses, and technologists must work together to develop frameworks that encourage ethical AI development and equitable implementation.
• Bridge the Digital Divide: Inclusive infrastructure development and access to AI technologies are critical for ensuring that no communities are left behind.
The event underscored the transformative potential of AI while highlighting the ethical and societal challenges it presents. For AI to become a true force for good, leaders must strike a balance between progress and responsibility. Insights from delegates reflected a shared belief: collaboration across industries, governments, and technology experts is the key to unlocking AI’s benefits while mitigating its risks.
How the UAE is leading the AI revolution
Fifty-five years ago, there was no UAE. Sixty-five years ago, there were very few roads and buildings. Seventy-five years ago, there was absolutely no infrastructure. There are people alive today who remember a UAE where city life resembled small villages across Asia and Africa—without roads, running water, or electricity. Most houses were made of clay if you were wealthy, or of palm tree branches if you weren’t.
This history is crucial for our future. The same people who experienced that simpler time are now witnessing the UAE’s push towards space exploration and investments in artificial intelligence. They’ve seen our evolution from a small fishing village on the Gulf Coast to one of the world’s most advanced countries. These are people who had the audacity to dream—dreams unbounded by resources, geography, or regional limitations.
When our country began, many taboos existed around what an Arab nation could achieve. As a “startup nation,” much seemed audacious or impossible. Yet, our leadership and citizens believed no dream was too big and no path too hard.
What also makes the UAE exceptional is that we have built all of this—not just as Emiratis—but as a community of people from over 200 nationalities. They have come from every corner of the world to achieve dreams that seemed unattainable elsewhere. With their hard work, sweat, and tears, they’ve helped us transform this nation.
Why is this history important in the era of artificial intelligence? Because every opportunity we’ve had came with a limited window for action. About 150 years ago, the Trucial States (now the UAE) were among the world’s leading pearl-diving centers. When the demand for pearls waned, we pivoted to focus on logistics, trade, and globalization— building Jebel Ali, one of the world’s largest ports.
Our next milestone is artificial intelligence. With a population of 10 million, our cities—like Abu Dhabi and Dubai—must leverage AI to compete on the global stage. We believe AI will enable smaller players to have a seat at the table and generate significant benefits for our citizens.
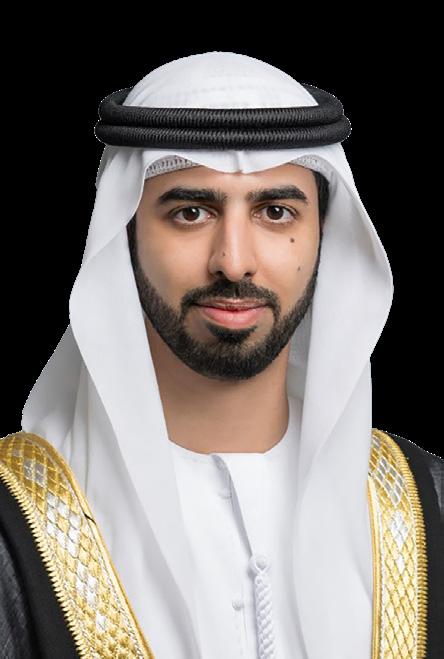
H.E. OMAR SULTAN AL OLAMA
Excerpts from a speech delivered by H.E. Omar Sultan Al Olama, UAE’s Minister of State for Artificial Intelligence, at Oracle CloudWorld.
In the UAE, our focus on AI isn’t solely for economic returns. While many see AI as a tool to generate extra revenue, our leadership is focused on using AI to enhance the quality of life. Effective AI deployment makes technology invisible but impactful— like when you experience seamless airport procedures, where you can disembark, leave the airport, and never interact with another person or even show your passport. This efficiency is the promise of AI we aim to realize.
Most global airports have facial recognition software, but with effective AI, we deliver unmatched services. In deploying AI within our government, our focus remains on improving lives daily.
Furthermore, we aim to be shapers of AI technology rather than mere consumers. When I was appointed in 2017—three years before COVID—the first step was investing in human capital. At that time, discussing AI felt hypothetical. Terms like self-driving cars, computer vision, and large language models were seen as science fiction that might come true in 15 years. Today, we know technology evolves rapidly, and our government must evolve just as swiftly.
Our initial focus in 2017 was on human talent—educating officials and citizens to understand, use, and leverage AI. The next phase involves creating a population of “super users.” An inspirational goal set by the current leadership in Dubai is for 30% of its residents to become expert prompters of AI in the next two years. Training one million people in this timeframe will foster a new generation of architects, doctors, scientists, and entrepreneurs empowered by AI.
We believe that enabling people to wield this technology to enhance their lives and impact is what AI is all about.
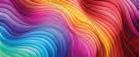