K NEAREST NEIGHBORS ALGORITHM
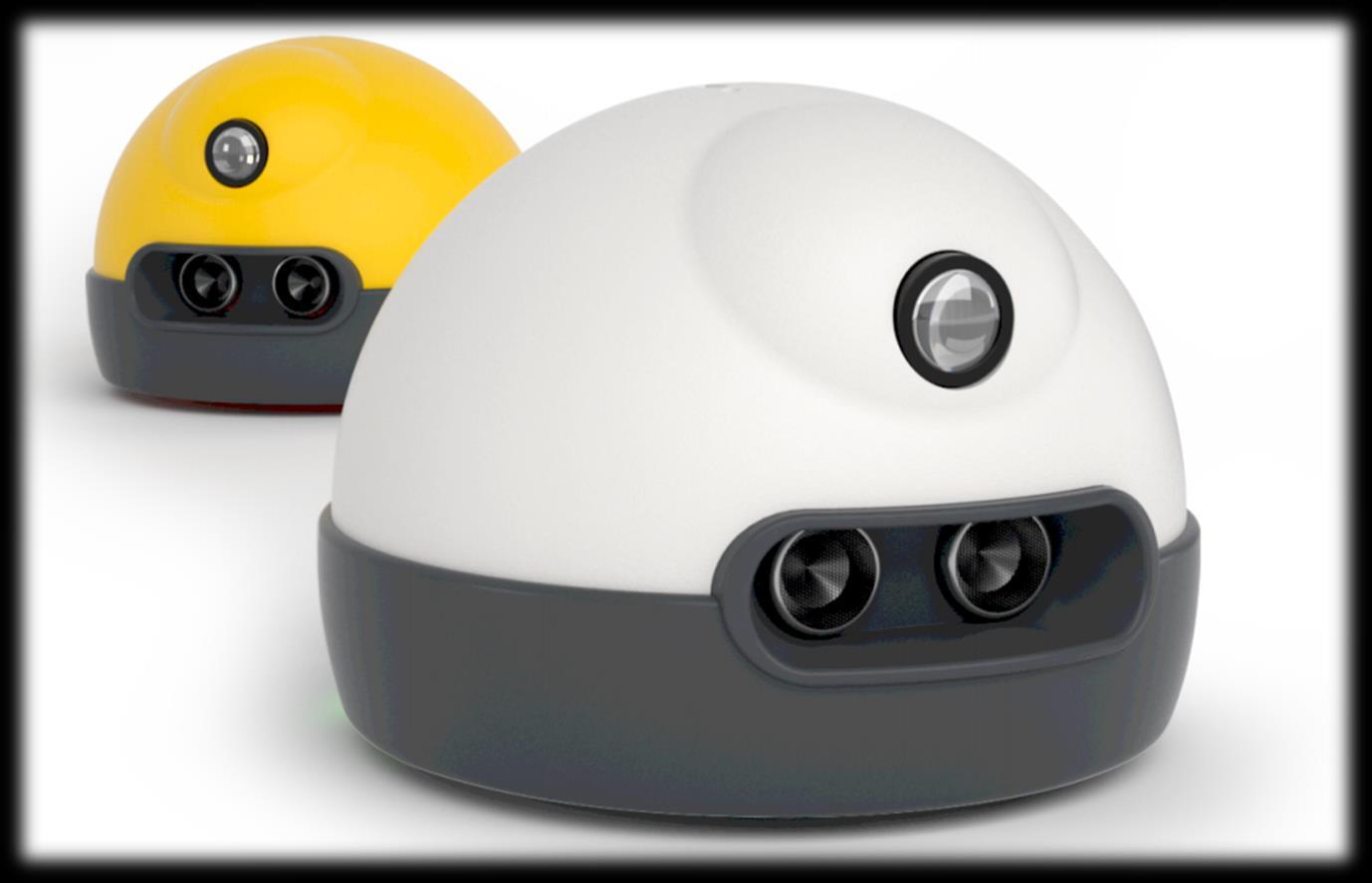
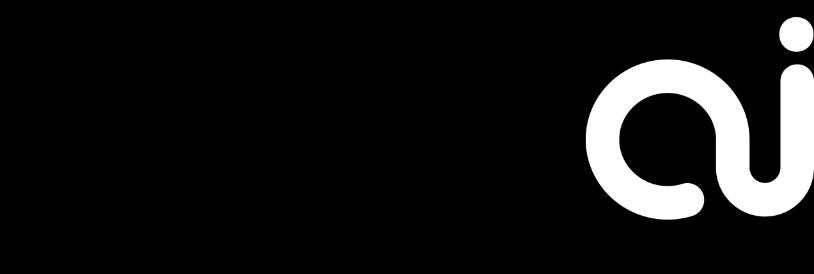
PREREQUISITE KNOWLEDGE PYTHON • • • • • • MATHEMATICS ��(����,����)������(����,����) �� =√(���� ����)2 +(���� ����)2 HARDWARESOFTWARE • • •
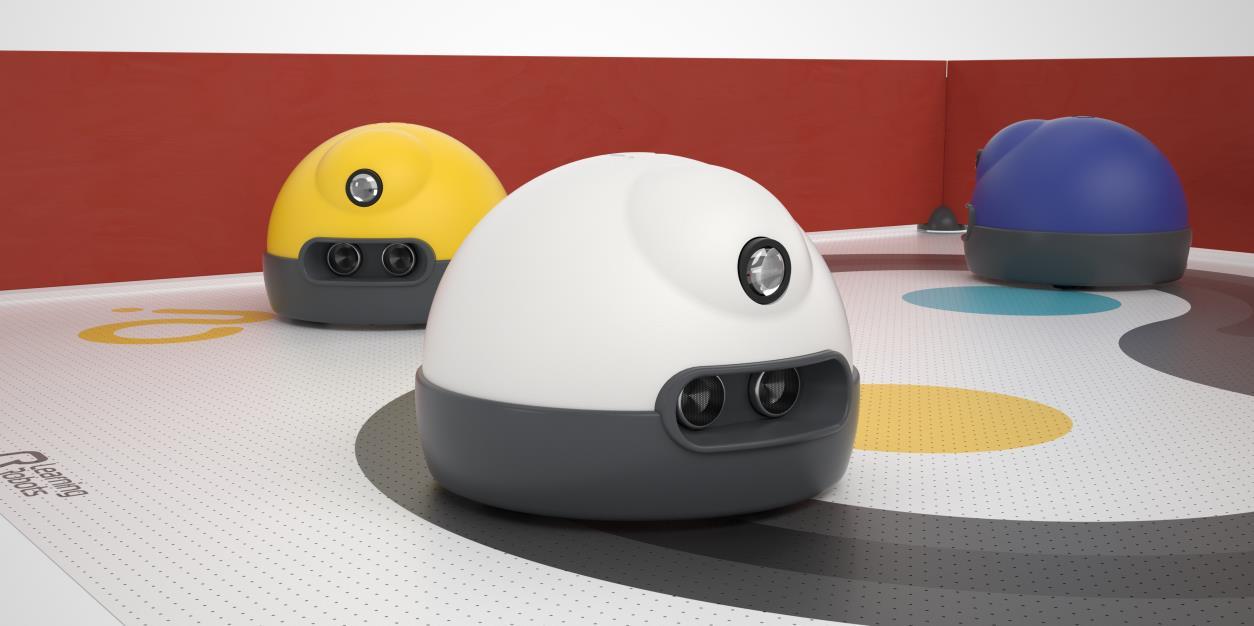
OBJECTIVE
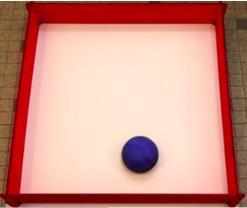
INTRODUCTION MACHINE LEARNING AND SUPERVISED LEARNING • • • �� ≈ ��(��)
STUDY SITUATION ET TRAINING SET OF THE ALPHAI ROBOT • • •
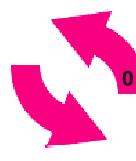
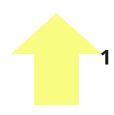
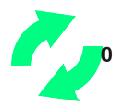
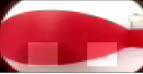
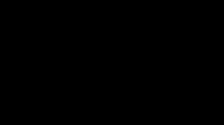


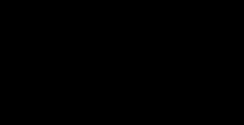


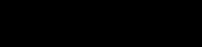
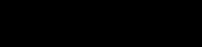
from PIL import Image import numpy as np import matplotlib.pyplot as plt def read_image(name): return np.array(Image.open(name, "r")) img1=read_image("forward_0.jpg ") # give the image path plt.show()plt.imshow(img1)
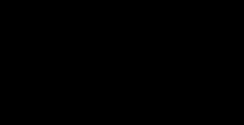


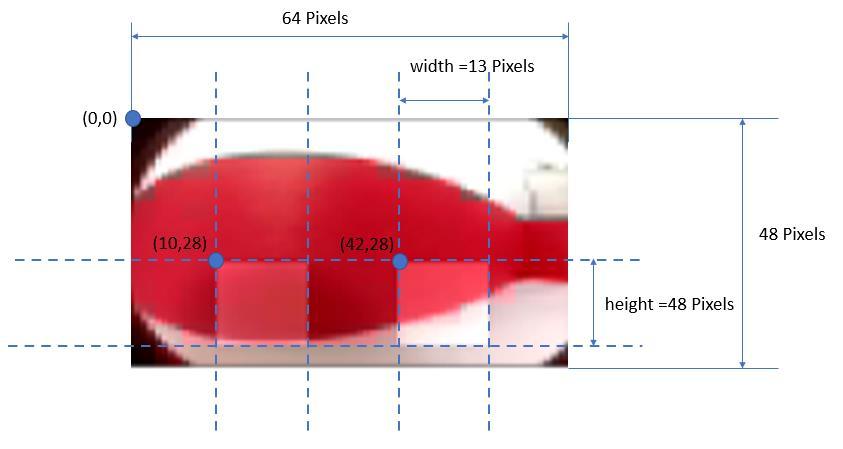
• • • def rgb2gray(rgb): return np.dot(rgb[...,:3], [0.299, 0.587, 0.144]) img2=rgb2gray(img1) plt.imshow(img2, cmap=”gray”) def��������(������,��,��,ℎ������ℎ��,��������ℎ) def get_two_values(img, x1=10, y1=28, x2=42, y2=28, height=16, width=13): value1 = average(img, x1, y1, height, width) value2 = average(img, x2, y2, height, width) return np.array((value1, value2))
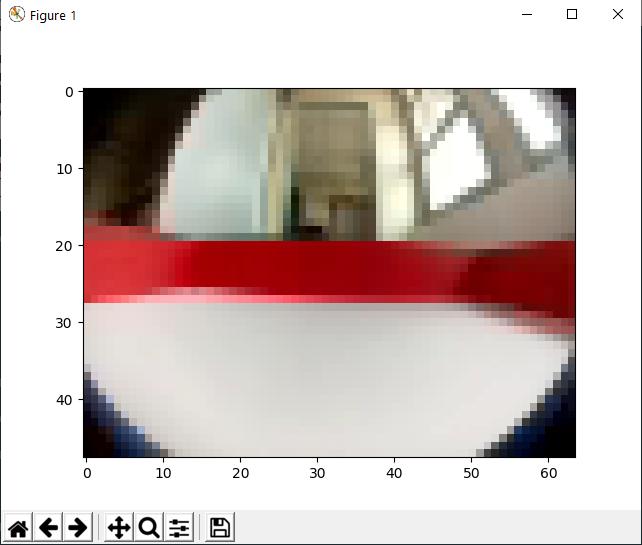
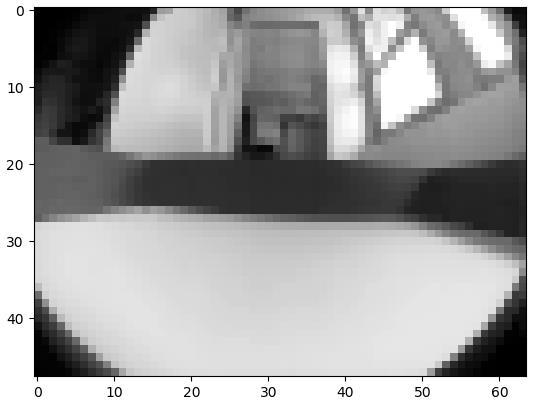
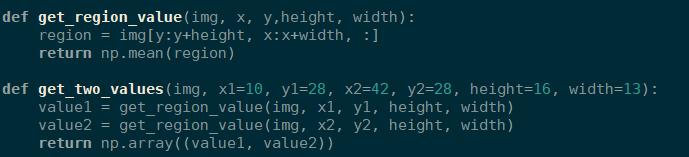
THE K NEAREST NEIGHBORS ALGORITHM, KNN


Start Algorithm Input data: • a dataset D, corresponding to the elaborated training set • a function for calculating the distance between the two considered points of coordinates [x1,y1] and [x2,y2].�� =√(��2 ��1)2 +(��2 ��1)2 • An integer k, representing the number of neighbors to be considered For a new observation X of which we want to predict his decision Y Do: 1. Calculate all distances of this observation X with the other observations in dataset D 2. Retain the K observations of dataset D closest to X using the distance calculation function d 3. Take the values of Y of the k observations retained. Perform a regression and calculate the mean (or median) of Y retained 4. Return the value calculated in step 3 as the value that was predicted by K NN for observation X. End Algorithm def����������������(����������1,����������2)
ROBOT TRAINING PREPARATION PHASE OF THE WORK ENVIRONMENT → → → →
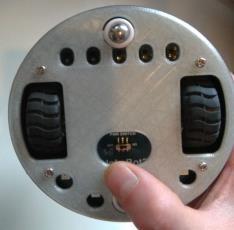
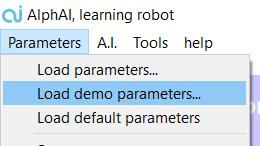
TRAINING PHASE • • • •
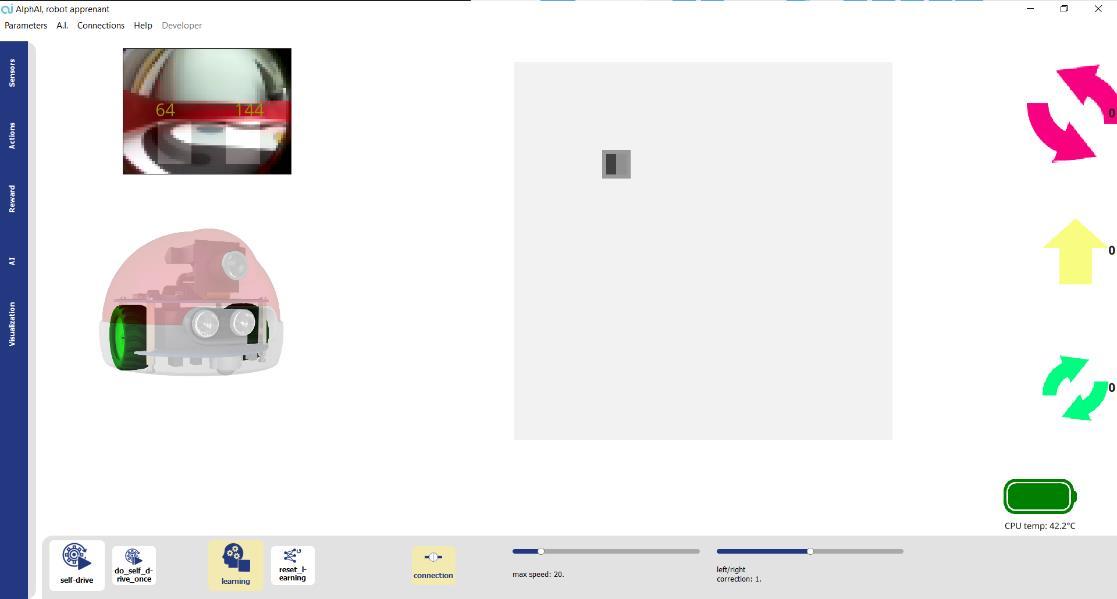



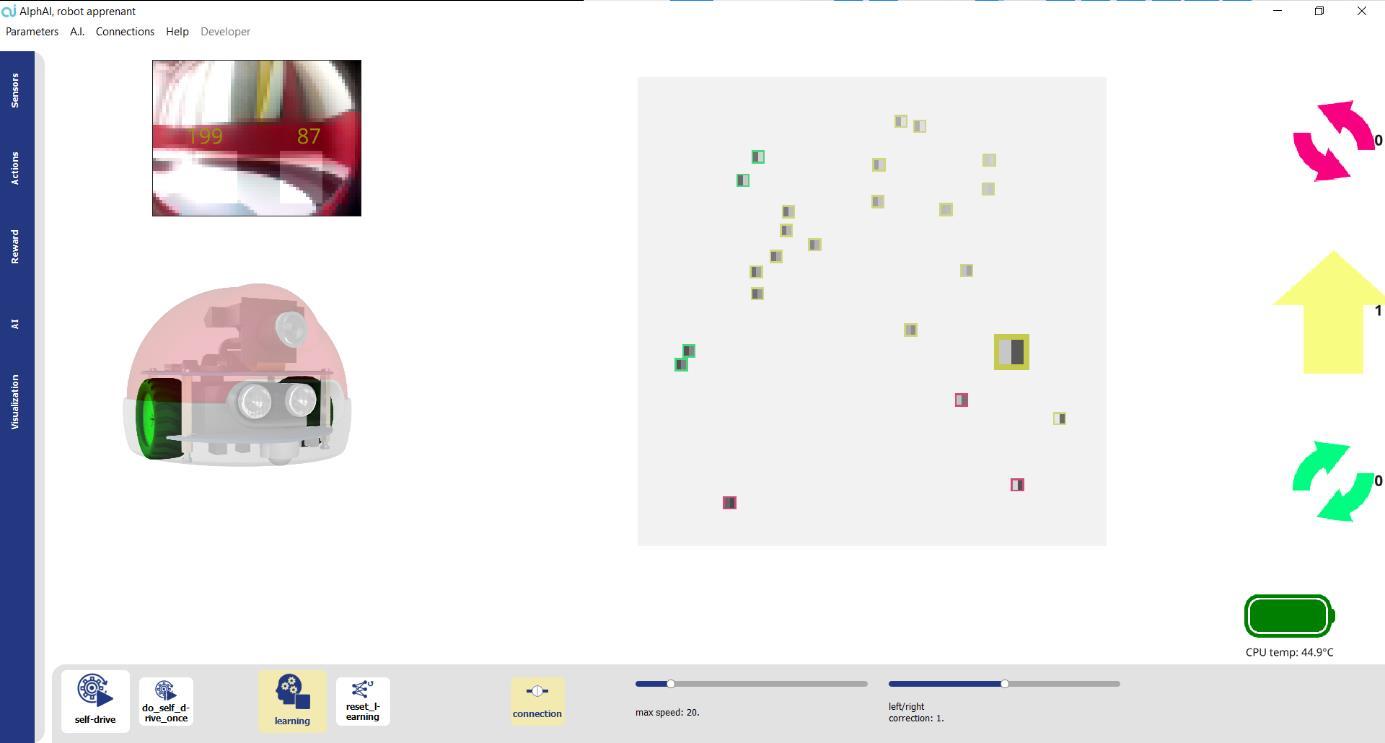
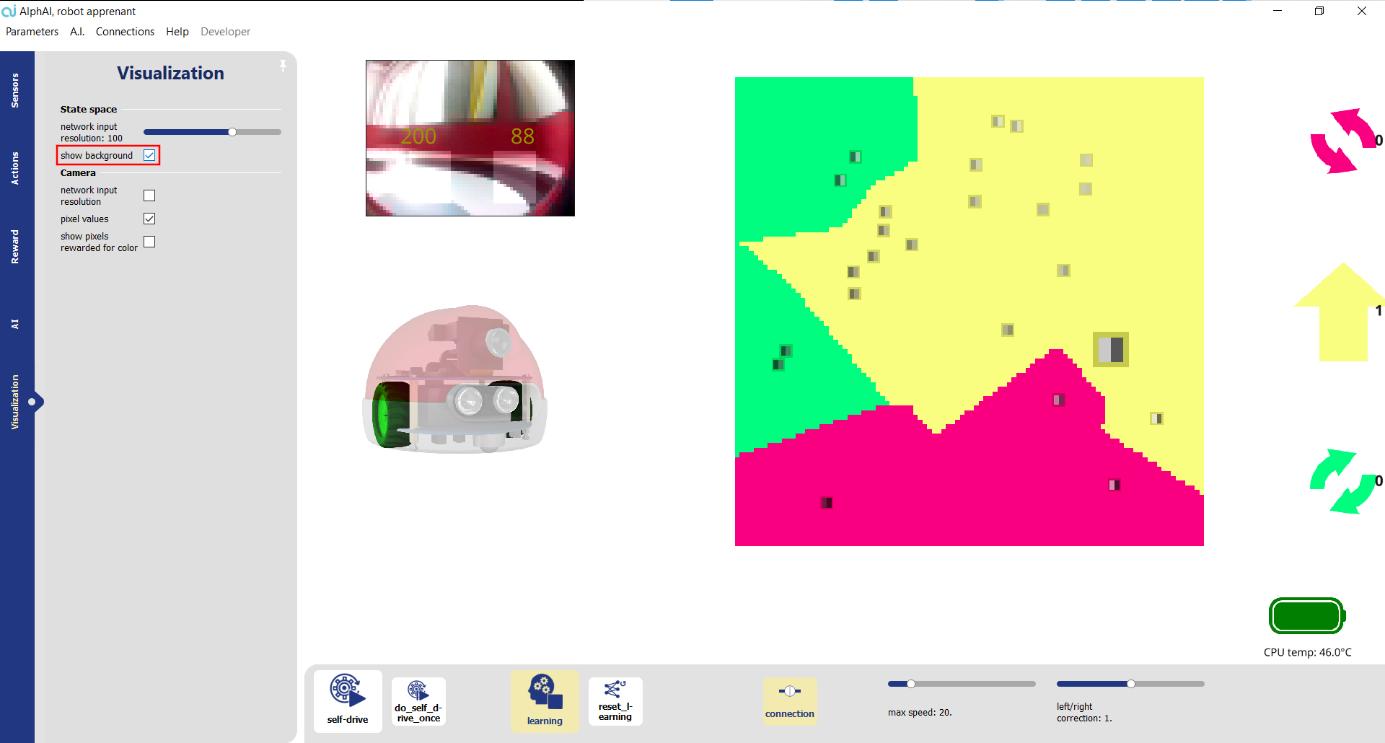
TESTING PHASE GO FURTHER:
TO
PROGRAM THE K'S NEAREST NEIGHBORS ALGORITHM → → → → PROGRAM THE ROBOT DIRECTLY def�������� ����������������(��������������:��������)→������

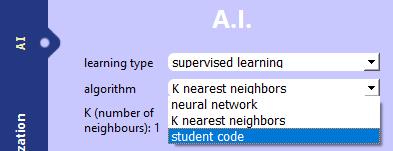
ALGORITHM PROGRAMMING DISTANCE CALCULATION def����������������(��:��������,��:��������) →���������� �� =[����,����]������ =[����,����] �� =√(���� ����)2 +(���� ����)2 import math y = math.sqrt(x) y = x**2
# compute distance a = [0, 0] b = [1, print("distance",2] distance(a, b)) CALCULATION OF ALL DISTANCES def������ ������������������(��:��������[����������],���������� ��������������:��������[��������[����������]])→��������[����������] �� =[��0,��0] ���������� �������������� =[[��1,��1],[��2,��2],…,[����,����]] # compute all distances a = [0.4, train_sensors0.6]= [[0, 0], [0, 1], [1, 0]] distance_list = all_distances(a, train_sensors) print('distances to data', distance_list) FIND THE SMALLEST ELEMENT OF A TABLE def�������� ��������������(����������:��������[����������])→������
# minimum in a list idx_min = print('indexfind_minimum(distance_list)ofminimum',idx_min) NEAREST NEIGHBOR def�������������� ��������ℎ������ ����������������(���������� ��������������:��������[��������[����������]],���������� ������������������:��������[������],��:��������[����������]) →������ �� =[��0,��0]���� ���������� �������������� =[[��1,��1],[��2,��2], ,[����,����]] ���������� ������������������ = [��1,…,����] ���� [����,����] # KNN a = [0.4, train_sensors0.6]= [[0, 0], [0, 1], [1, 0]] train_decisions = [1, 2, 0] decision = nearest_neighbor_decision(train_sensors, train_decisions, a) print('KNN', decision)
USING YOUR ALGORITHM WITH THE ROBOT train_sensors = train_decisions = None def learn(X_train, y_train): global train_sensors, train_decisions train_sensors, train_decisions = X_train, y_train loss = 0 return loss def iftake_decision(sensors):train_sensorsisNone : return 0 return nearest_neigbor_decision(train_sensors, train_decisions, sensors)




TO GO FURTHER K NEAREST NEIGHBORS �� >1,�� ∈ℕ INTRODUCTION TO NEURAL NETWORKS CONCLUSION :
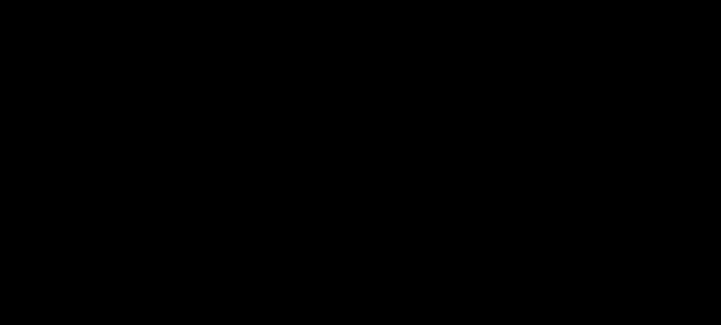
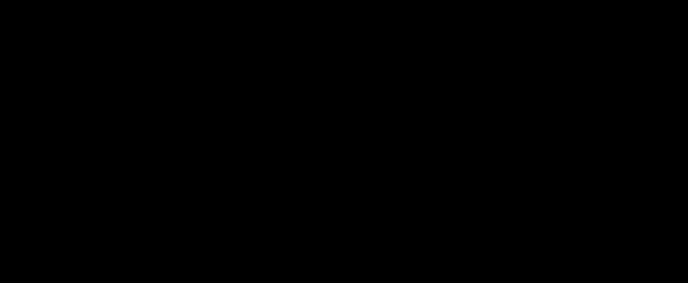
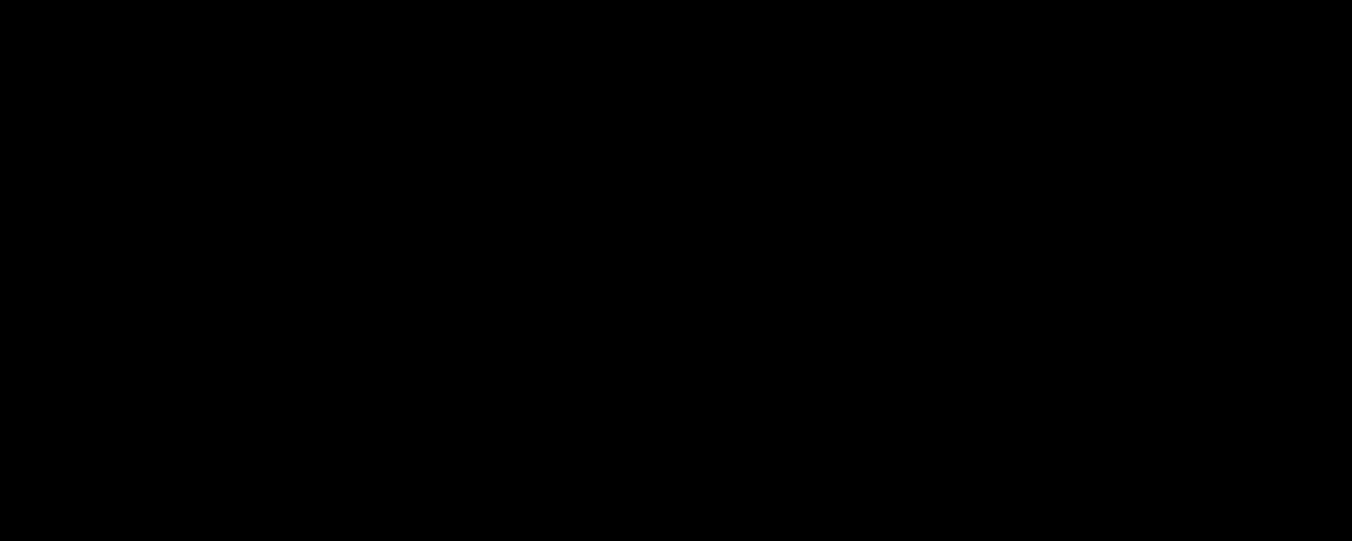