EDUCATION IN ARTIFICIAL INTELLIGENCE K NEAREST NEIGHBORS ALGORITHM
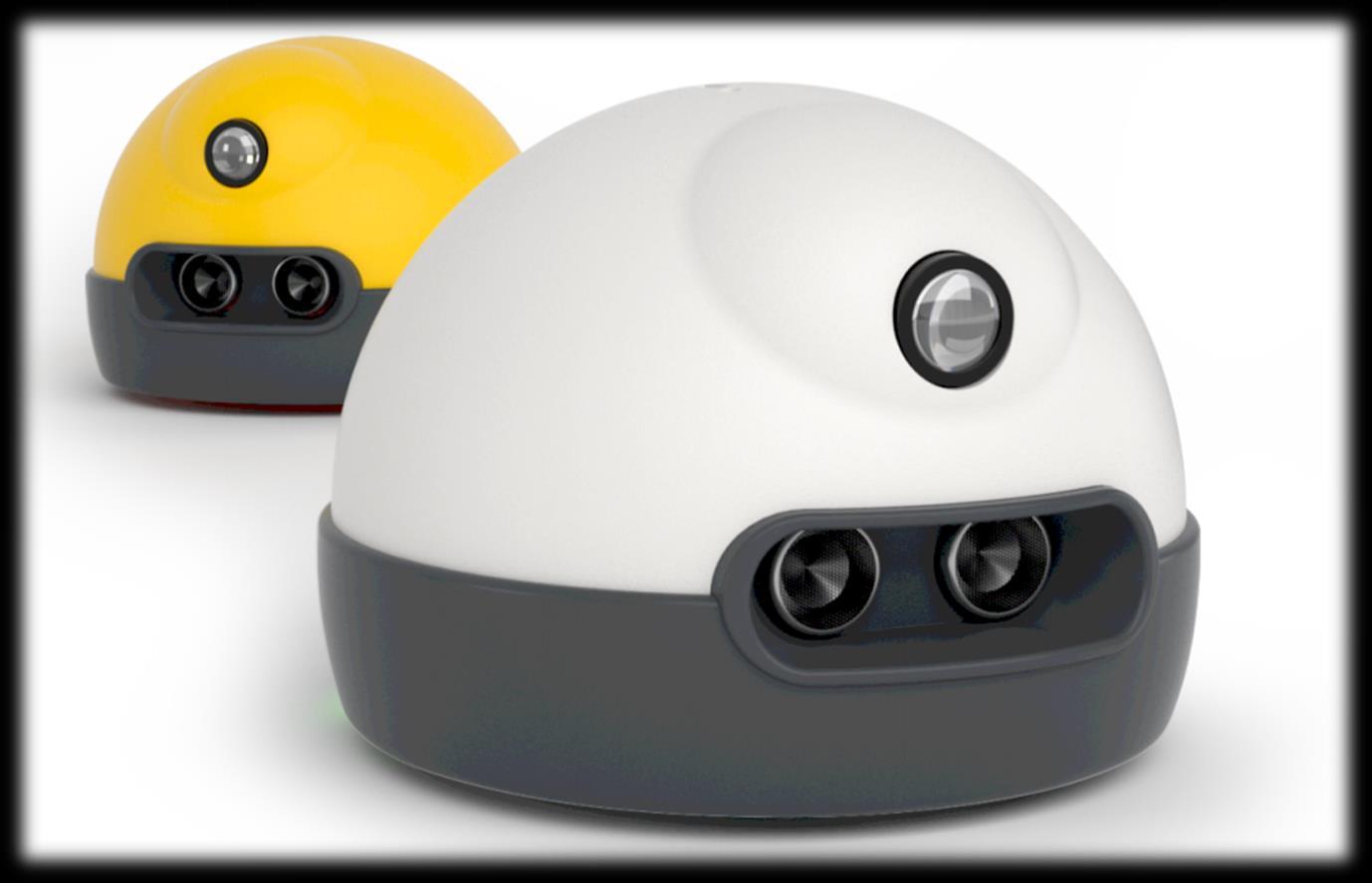
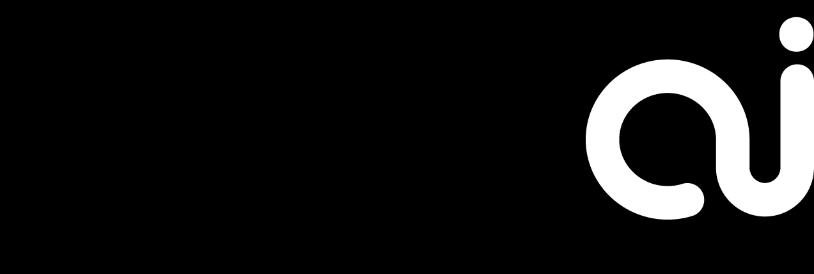
PREREQUISITES KNOWLEDGE PYTHON • • • • • • MATHEMATICS ��(��1,��1)������(��2,��2) �� =√(��2 ��1)2 +(��2 ��1)2 MATERISOFTWAREALS
INTRODUCTIONACTIVITYSTEPS
DISCOVERY OF THE K NEAREST NEIGHBORS IN THE SOFTWARE → → → → →

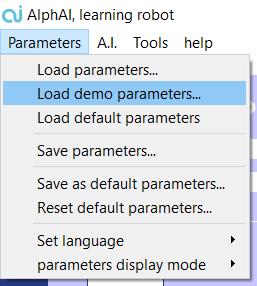
TRAINING PHASE • Connect the robot • • •



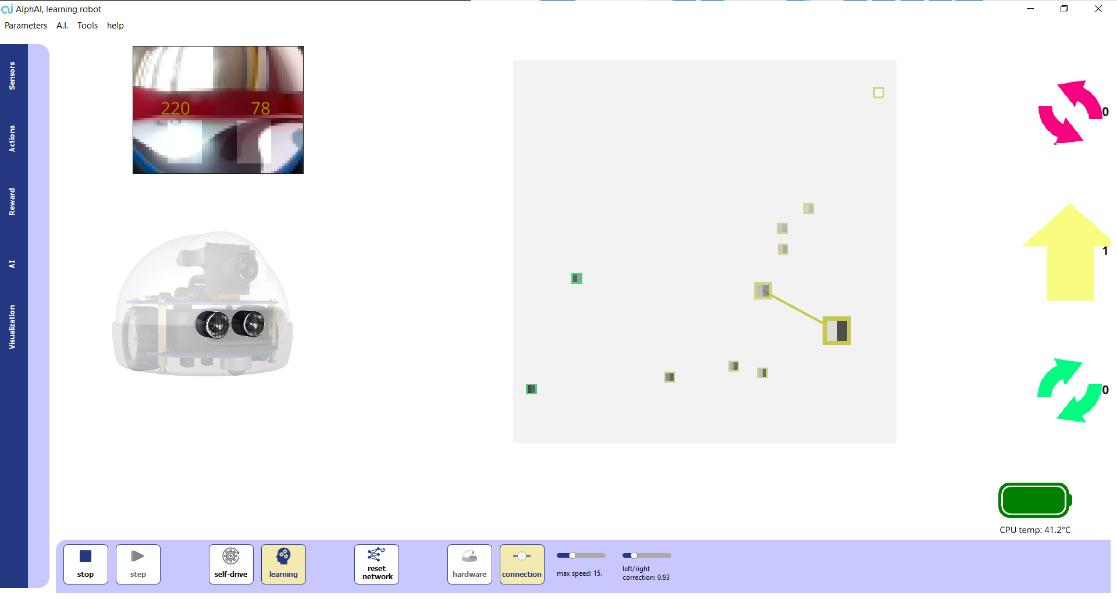
TEST PHASE • PROGRAM THE K NEAREST NEIGHBORS ALGORITHM → → → → →
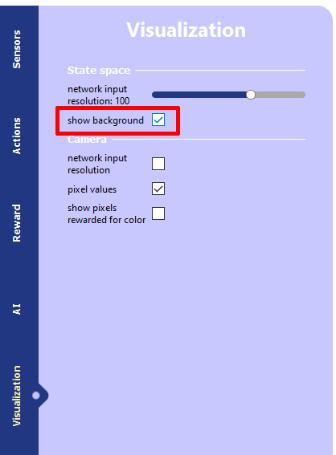
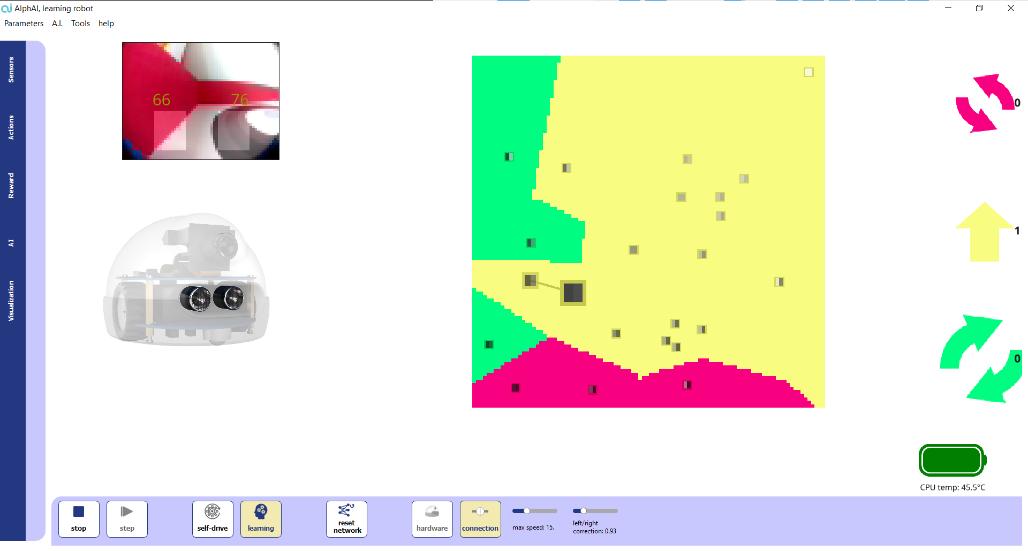


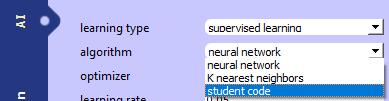
def�������� ����������������(��������������:��������)→������


PROGRAMMING THE ALGORITHM DISTANCE CALCULATION def����������������(��:��������,��:��������) →���������� �� =[��1,��1]������ =[��2,��2] �� =√(��2 ��1)2 +(��2 ��1)2 import math y = math.sqrt(x) y = x**2 # compute distance a = [0, 0] b = [1, print("distance",2] distance(a, b)) COMPUTATION OF ALL DISTANCES def������ ������������������(��:��������[����������],���������� ��������������:��������[��������[����������]])→��������[����������] �� =[��0,��0] ���������� �������������� =[[��1,��1],[��2,��2], ,[����,����]]
# compute all distances a = [0.4, train_sensors0.6]= [[0, 0], [0, 1], [1, 0]] distance_list = all_distances(a, train_sensors) print('distances to data', distance_list) FIND THE SMALLEST ELEMENT OF AN ARRAY def�������� ��������������(����������:��������[����������])→������ # minimum in a list idx_min = print('indexfind_minimum(distance_list)ofminimum',idx_min) NEAREST NEIGHBOR def�������������� ��������ℎ������ ����������������(���������� ��������������:��������[��������[����������]],���������� ������������������:��������[������],��:��������[����������]) →������ �� =[��0,��0]������ ���������� �������������� =[[��1,��1],[��2,��2],…,[����,����]] ���������� ������������������ = [��1, ,����] ���� [����,����]
# KNN a = [0.4, train_sensors0.6]= [[0, 0], [0, 1], [1, 0]] train_decisions = [1, 2, 0] decision = nearest_neighbor_decision(train_sensors, train_decisions, a) print('KNN', decision) USE OF YOUR ALGORITHM WITH THE ROBOT train_sensors = train_decisions = None def learn(X_train, y_train): global train_sensors, train_decisions train_sensors, train_decisions = X_train, y_train loss = 0 return loss def returniftake_decision(sensors):train_sensorsisNone:return0nearest_neigbor_decision(train_sensors, train_decisions, sensors)



Supervised learning: By giving the robot a consistent dataset and an algorithm, it was able to generalise its decision making to situations it did not know before by approximating what was done Nearestbefore.Neighbour
Algorithm: By implementing classical algorithms such as calculating a distance or finding a minimum in a list, we discover that it is possible to program a machine learning program ourselves
TO GO FURTHER K NEAREST NEIGHBOURS �� > 1,�� ∈ℕ INTRODUCTION TO NEURAL NETWORKS CONCLUSION
Notions learned
:


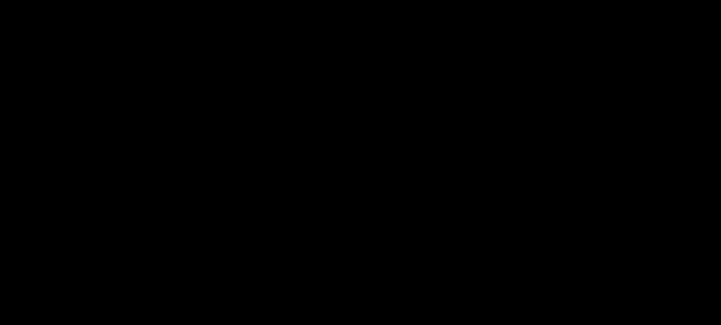
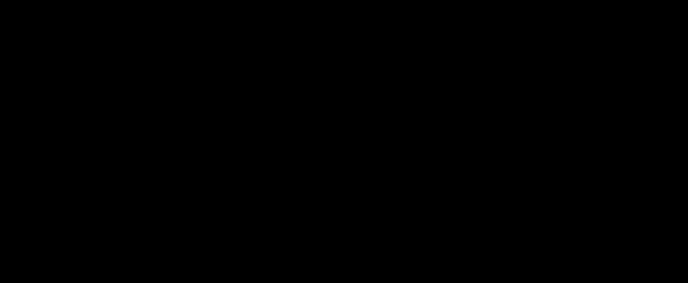
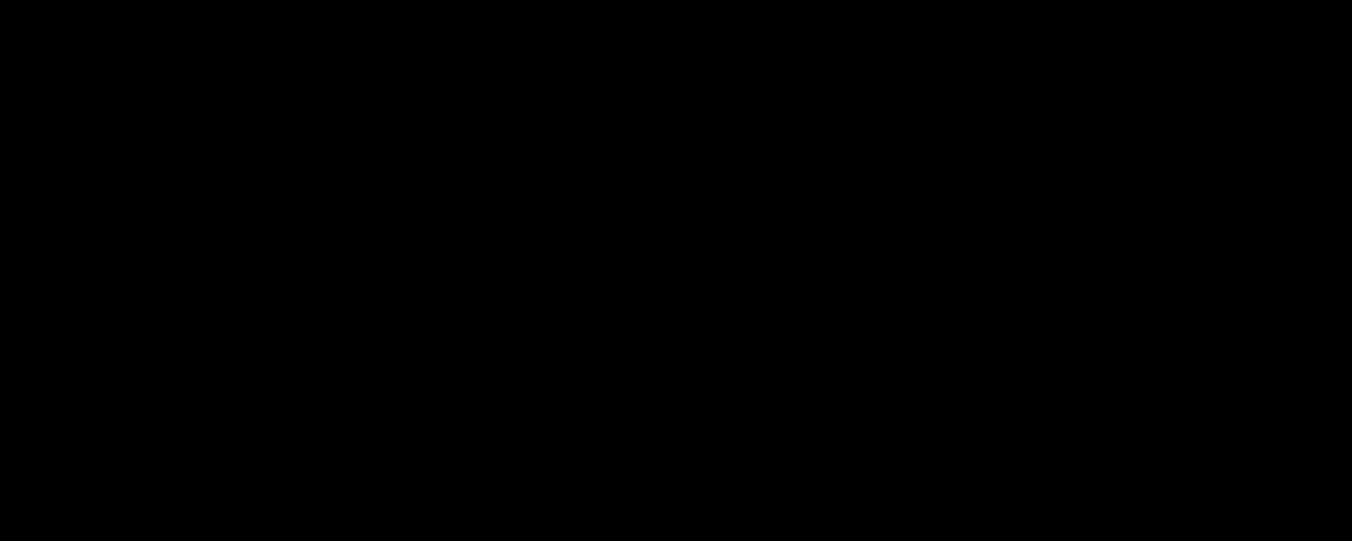