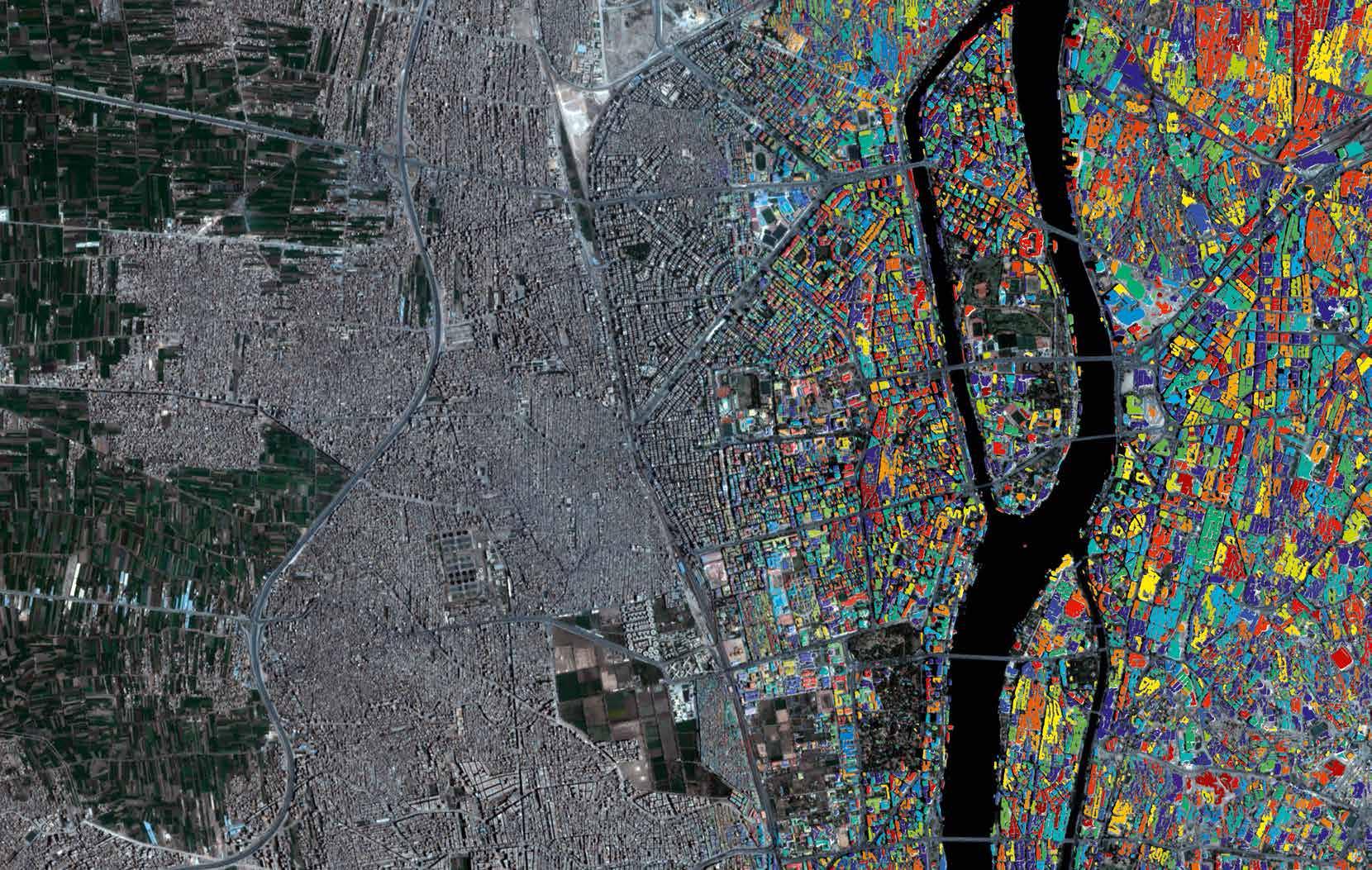
6 minute read
So2Sat
Mapping global urbanisation with satellite and social media in a revolutionary approach
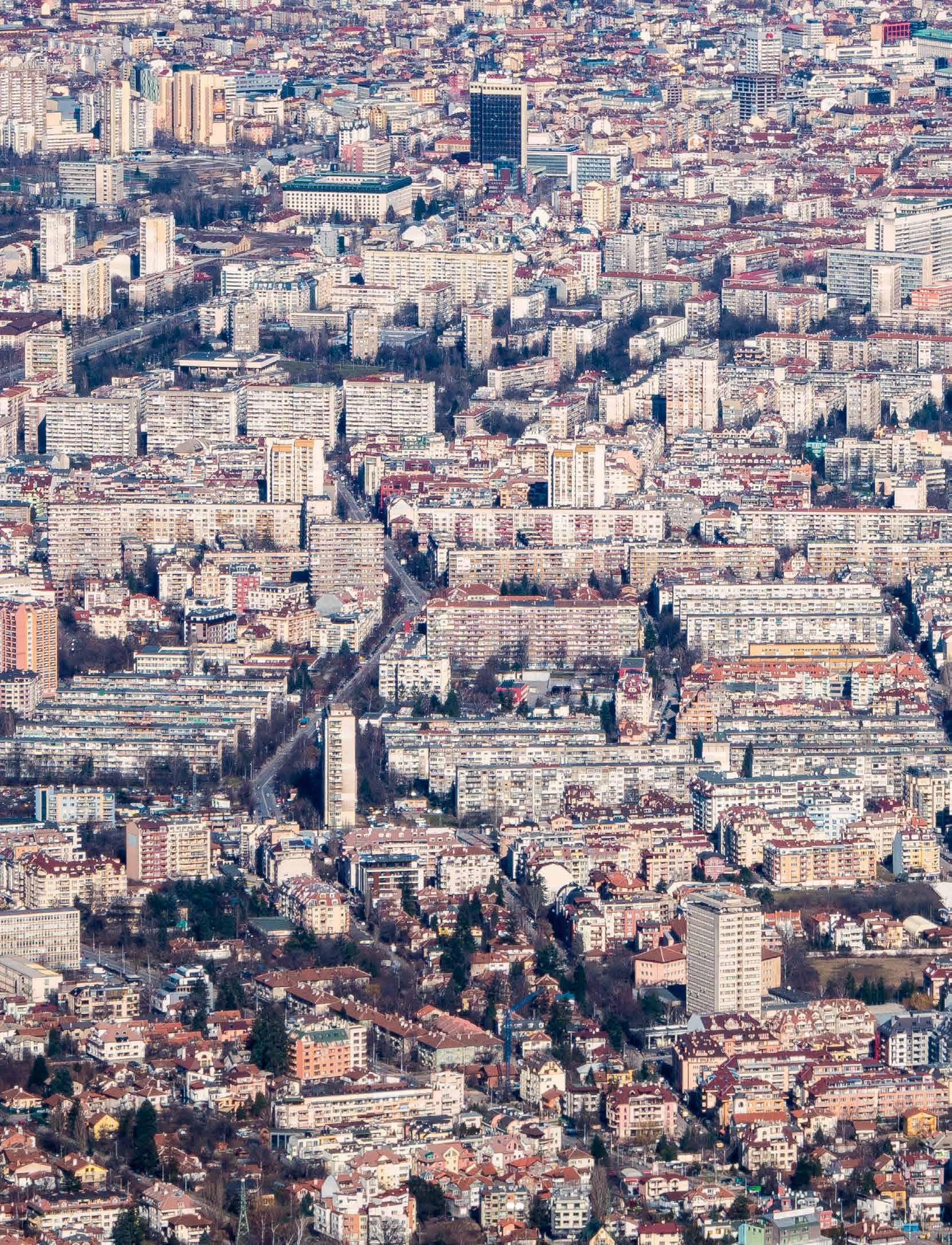
So2Sat is a pioneering European Research Council (ERC) project, led by Professor Xiaoxiang Zhu, using petabytes of data from Earth observational satellites and social media to create 3D/4D models of individual buildings on a global scale, in order to better understand the global urbanisation process.
In the 20th Century alone, the global population has expanded from 1.65 billion to 6 billion and 50 years ago there were approximately half as many people in the world as there are today. A symptom of this exponential growth is worldwide urbanisation and spontaneously growing human settlements. Effectively tracking such amorphous developments globally was widely considered to be near impossible. City expansions, especially, are happening at pace, with migrations of people moving to urban areas.
“Urbanisation is the second biggest important mega trend of global changes after climate change,” explains Professor Zhu. “If you look at the evolution of rural and urban population over time, there is actually a secret change of human history in 2008, when there were more people in the cities than in rural areas and if you look at the prediction for 2050, there are much more people who will eventually live in cities.”
In 2050 we can expect three fourths of the population to be living in metropolises.
The challenges of urban expansion
The evolution of very large cities can be chaotic, and how populations are distributed is not always known. For example, mega cities invariably include substantial informal settlements such as slum areas with unknown numbers of people residing in them. These kinds of gaps in knowledge mean that sustainable planning for city planners and urban developers can be highly problematic.
“Urban growth is mostly occurring in the developing areas: Africa, South America and Asia. Urbanisation, if not well controlled, will lead to informal settlements and slums. Without proper management this could endanger the lives of people who are living there. An example is in Mumbai, where in the past ten years more than fifty thousand fires happened in this city and about sixty seven percent of these fires are actually caused by faulty wiring. We need Geo-information.”
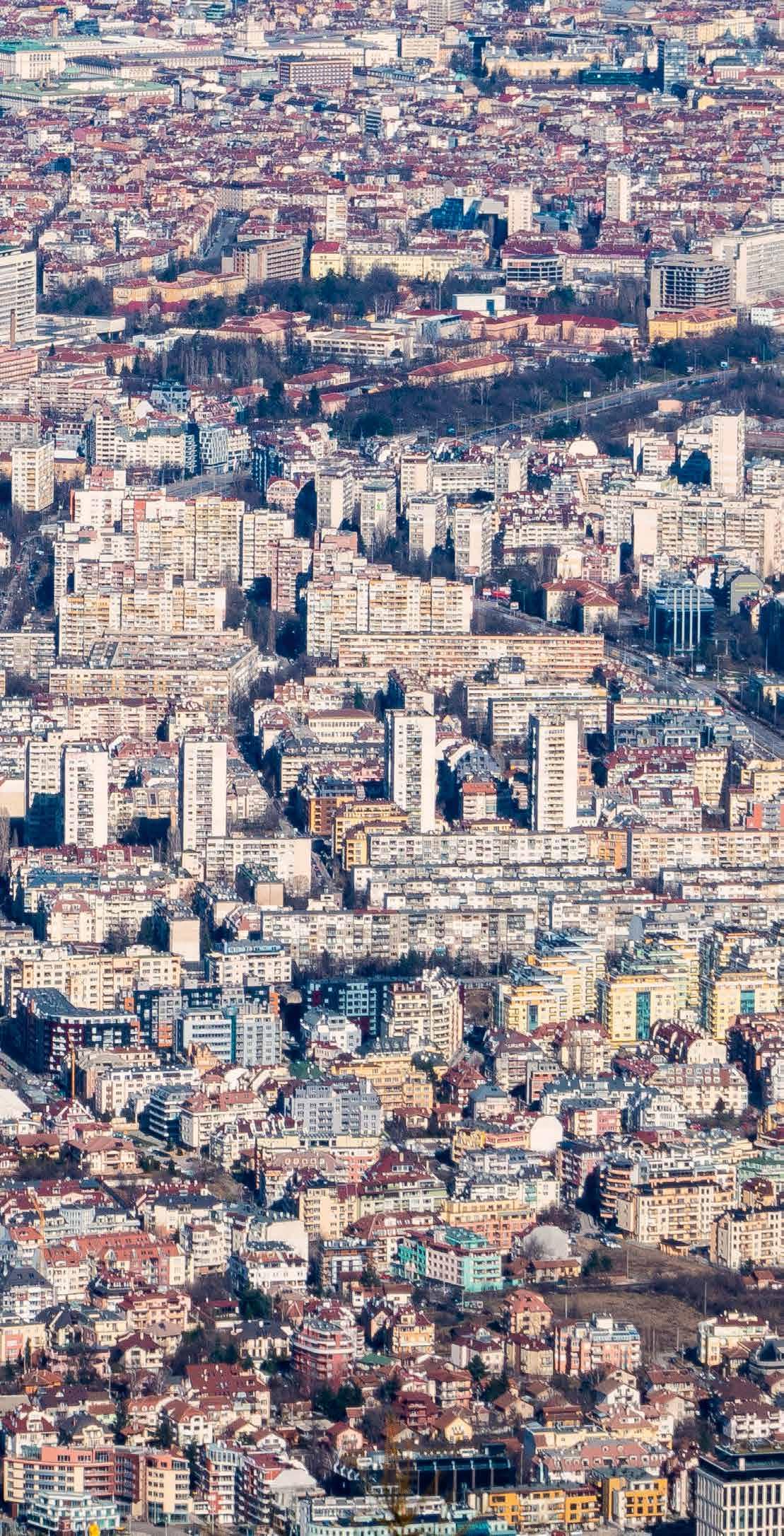
Unregulated, mismanaged and misunderstood city areas can cause significant problems like this, so good, realistic data is needed for competent and safe city development. However, the relevant data is just not available. In reality, there are many large urban areas on Earth that have little information on them, for example, the African city of Lagos, with 21 million people living there, has no open 3D model available of it. With an accurate census and an idea of the developing environment, urban conditions could be better managed and improved by authorities.
Data can change the map
The aim of So2Sat is to reveal accurate details of human settlements, on a global scale, using 10 petabytes of Earth observation and social media data. We are said to be in the Golden Era of Big Earth Observational Data, with several satellite missions mapping the Earth’s surface, such as the Sentinel series and TerraSAR-X/ TanDEM-X, so it is now possible to think big for mapping projects. However, getting insights from such a huge amount of data is a challenge. Therefore, So2Sat also develops state-of-the-art machine learning algorithms and big data analytics solutions to tackle this big data challenge.
“Together with my team we are exploiting different types of satellite data, we combine them even with social media data and what we want to do on a global scale is to reconstruct the 3D shapes of buildings, the evolution over time, and we want to derive the functions of the buildings so you can find residential and non-residential areas, and if we combine the 3D information with the functions, we can also get a transparent calculation of the population density capacity. In slums, the population density in the census data is extremely underestimated,” said Professor Zhu. platforms like Flickr and Twitter, which mean there is access to ground-level measurements of buildings.
In a nutshell, the So2Sat sophisticated mapmaking strategy is as follows: radar satellite imagery first deciphers the height of a building, high-resolution RGB images delineate the 2D building shapes, then hyperspectral satellite senses the roof material and finally, street level imagery and tweets are found from social media platforms and assigned to individual buildings. This already gives a rich 3D map of urban areas. But data associated with individual buildings will then be further used to understand the functionality of the building, such as residential or nonresidential. AI algorithm, to be specific, various types of deep neural networks, plays important roles in various steps in the pipeline, for example, using graph neural network to segment the building boundaries from satellite images, and using natural language deep learning models to predict the building type from tweets.
The banner image shows the buildings in Cairo, Egypt predicted by the So2Sat graph neural network from a PlanetScope imagery of Planet Labs. The algorithm is not only able to classify the built up areas from the relative low cost satellite imagery, but also able to segment individual buildings which
Painting in the building’s details
The innovation really comes to the fore when the project combines the satellite imagery and data with images and information mined from social media, on are marked by different colours in the figure.
Relying on the aforementioned series of big EO data and cutting-edge AI algorithms, the research team will attain the first global and consistent 3D/4D spatial data set on urban morphology of settlements up to the detail of individual buildings as well as their evolution over time (4D), whereby it will be possible to derive population density more accurately than ever. Those data will be made available to the community. This is powerful information that can aid decision making on urban development.
The project demonstrates a significant step change in how we can perceive cities, with more accuracy, to make better planning decisions in urban areas and for the first time, we will possess a complete, accurate, 4D model of every urban environment on Earth.
Big Data for 4D Global Urban Mapping – 1016 Bytes from Social Media to EO Satellites Project Objectives
By the fusion of petabytes of Earth observation data from satellite to social media, fermented with tailored and sophisticated machine learning algorithms and big data analytics solutions, So2Sat reveals for the first time, detailed 3D geometry, morphology and composition of global urban agglomeration on an individual building level, providing reliable dataset for monitoring the global urbanisation, especially in developing countries.
Project Funding
A total funding of about €1.5 million was granted for So2Sat in 2017. The success of So2Sat also attracted a follow-on ERC proof of concept grant AI4SmartCities, which has started in 2021.
Project Partners
The So2Sat project consortium consists of the Professorship of Data Science in Earth Observation, Technical University of Munich, and the Remote Sensing Technology Institute of German Aerospace Center. We also have the German Remote Sensing Data Center, and the Leibniz Supercomputing Centre in Garching, Germany, as partners.
Contact Details
Professor Xiaoxiang Zhu Principal Investigator Technical University of Munich (TUM) Data Science in Earth Observation, Arcisstr. 21, 80333 Munich, Germany German Aerospace Center (DLR), Remote Sensing Technology Institute 82234 Wessling, Germany T: +49 8153283531 E: Xiaoxiang.Zhu@dlr.de W: so2sat.eu W: ai4smartcities.eu W: https://www.professoren.tum.de/en/zhu-xiaoxiang
Professor Xiaoxiang Zhu
Xiaoxiang Zhu is the Professor for Data Science in Earth Observation at the Technical University of Munich (TUM) and heads the Earth Observation Data Science department at the German Aerospace Center (DLR). She serves as the Director of the international AI future Lab AI4EO, co-director of the Munich Data Science Institute of TUM, the co-spokeswoman of the Munich Data Science Research School, as well as the head of the Helmholtz AI – Research Field: Aeronautics, Space and Transport.