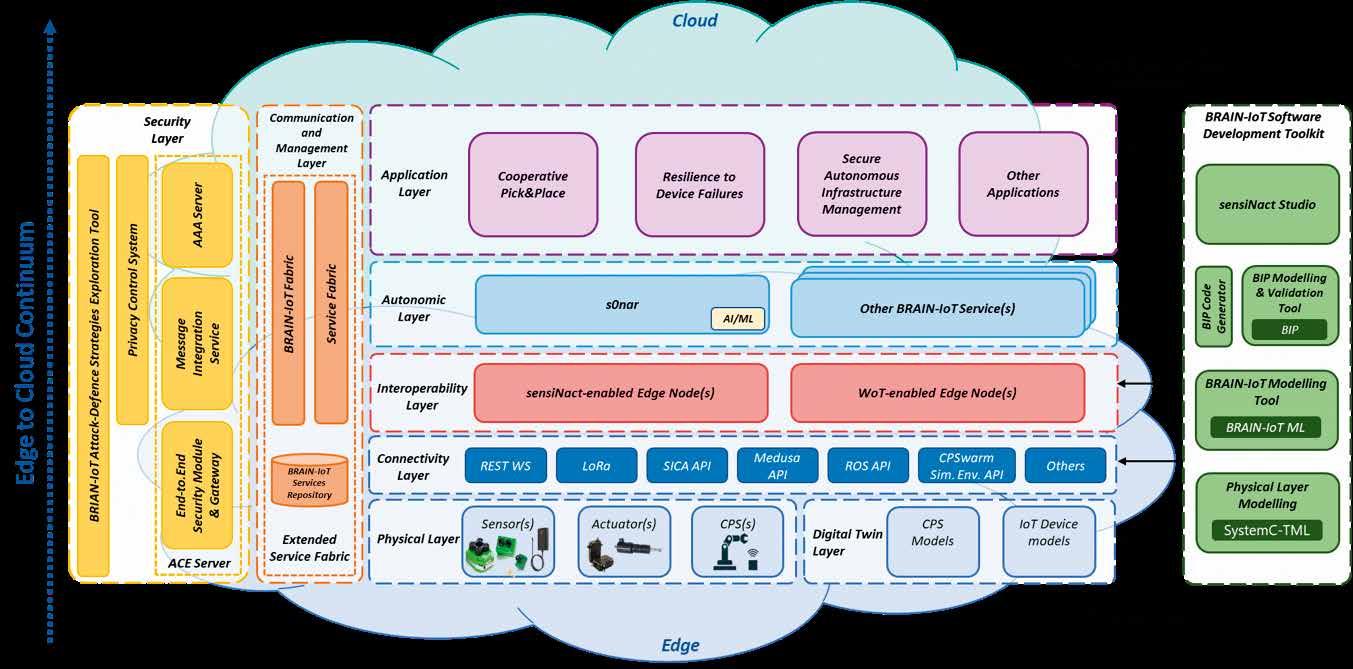
8 minute read
BRAIN-IoT
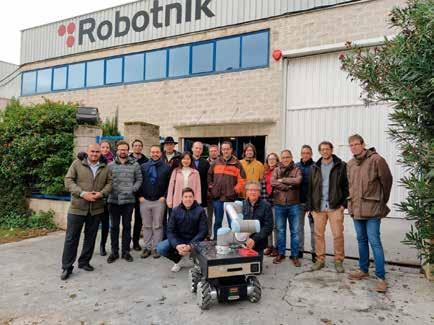
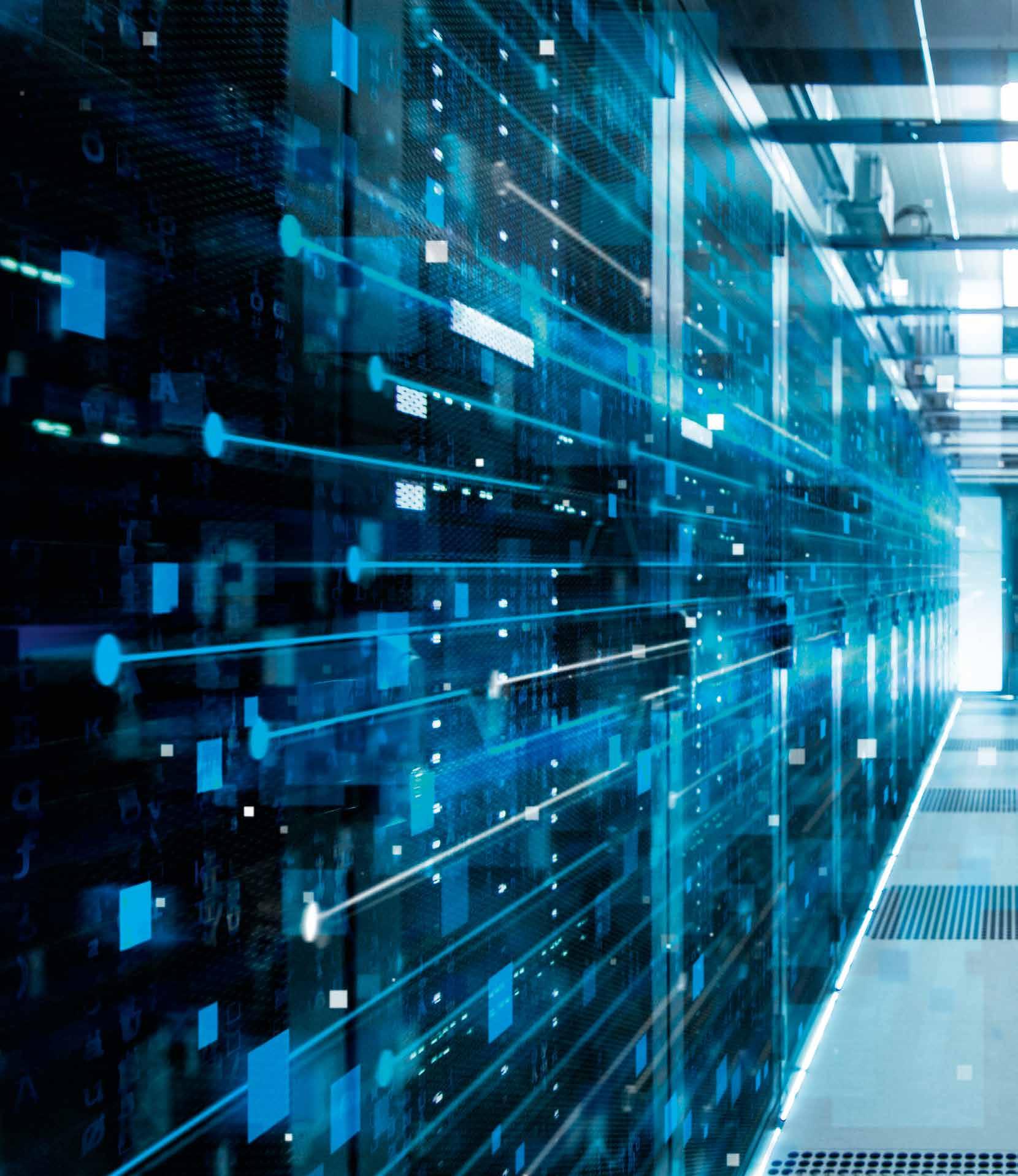
Enrico Ferrera of the BRAIN-IoT project explains how a new approach for the Internet of Things around distributed artificial intelligence creates a powerful tool for industrial use, reducing resources, the strains on operators and increasing accuracy in autonomous systems, able to self-manage.
The Internet of Things is getting widely
adopted in different domains to monitor and control systems of heterogeneous devices and platforms, to ensure effective and efficient functioning. Project coordinator of BRAIN IoT, Enrico Ferrera is leading a consortium pioneering a new AI driven IoT management system, to realise the tremendous advantages when you scale IoT for critical industrial purposes.
Thinking big with IoT
“IoT is a reality. Many different IoT platforms already exists, facing different challenges in several application domains, as whilst these are very similar to each other, they are not compliant to each other, so this remains a problem. For instance, IoT is already widely used for smart homes, and you can control many different things for home automation. What we are trying to do is to extend these concepts to cope with specific requirements in the industrial domains. The near real time reaction, the resiliency, making critical decisions. A business must have continuity and carry on operating effectively.”
The project is developing a smart, flexible IoT based system which can drive critical decision making for businesses, collecting, managing and using real time IoT generated data. Applying this to large scale industrial operations would reap great benefits such as having greater accuracy for making good autonomous decisions in the moment and diminishing human resources mistakes and disconnected operations. BRAIN-IoT proposes a solution based on the architecture in Figure 1.
The interoperability layer is implemented through the integration of the Eclipse sensiNact IoT platform as edge node for the semantic adaptation of the data coming from the Physical Layer. Edge nodes based on the W3C Web of Things standard have also been implemented to support the interoperability with ROS (Robot Operating System) based robotic platforms. The real beauty of the BRAIN IoT approach is in its use of learning AI. BRAIN-IoT is pushing to automate the management of IoT-based systems, implementing cognitive capabilities to learn and make decisions at the right time, as well as to support the autonomous healing in case
Figure 1: BRAIN-IoT Architecture.
of failures. “We would like to develop and apply these artificial intelligence strategies, in order to identify situations, and to react in the same system,” said Enrico.
These functionalities are supported by the s0nar server, which implements AI algorithms for data analysis, critical events detection, and prediction. Along with the BRAINIoT Fabric, s0nar supports the dynamic
reconfigurability of the overall behaviour of the system, to react properly and promptly to critical situations that may happen (e.g. cyber attacks, network and device failures).
“We want to show that this platform can be applied in different domains, that appear unrelated but in fact we’d like to illustrate that the challenges are the same, and the technologies that you can use in order to mitigate the issues in these domains are the same. This project has two different main use cases,” divulges Enrico. “In order to cover the widest range of applications we wanted to identify two scenarios that are quite different from each other. One is a robotics scenario, the other one is the management of critical infrastructure, specifically the water distribution management infrastructure situated in A Coruña Spain.”
Robots that know what matters
The factory scenario sought to make teams of ‘pick and place’ robots autonomous in solving their own problems and challenges, without human intervention. Usually, these IoT platforms have a structure of data management where they can integrate some local devices and this data is sent to one or more central points into the cloud, but in a more de-centralised system as proposed by the project, it was imperative to make the system more distributed and peer-to-peer.
“In the service robotics use case, robots select items and bring them to the right place in the warehouse. They are communicating with the backend system because the backend system is the part of the warehouse system that knows where the specific box should be stored. In these fleets of robots, each have their own objective paths and they cooperate with each other to make the activity efficient.
In this case, imagine the battery of the robot runs out. In this situation the system can redeploy the behaviour of one the robots to compensate for the non-functioning robot. For example, to fix a problem with one fleet operating the pick and place task, one other robot that was originally working another task can be re-allocated to compensate for the missing robot on the other fleet. This system can deploy the new behaviour to one robot of the fleet, in such a way that different fleet robots can work with other fleet robots, in order to accomplish the pick and place task. There is also criticality in the system, so it’s okay if one robot in fleet B can leave its task and join fleet A, because it is considered that its task for fleet B is less critical than A.”
Awareness of all the data
The other case study focused on Critical Infrastructure Management, which is a suitable use case to show the advantages of an advanced IoT system to monitor and manage a water distribution system, made of valves, water levels, dams and flows to be properly coordinated. One requirement was to make the system adapt to the environmental changes, supporting the IT manager who can focus on different tasks rather than staying there to check that everything is still running.
“A manager would usually check the components of these systems, one at a time, which is time consuming. We would like to go beyond that, adding AI that would allow the system to autonomously react, to recognise critical situations.”
BRAIN IoT has a specific focus for the security domain and one of the objectives in terms of maintenance of the system, is to make the system more robust and resilient to critical situations.
“The system can recognise a cyber-attack and set up counter measures. It can also identify anomalies on devices. For instance, one of the meters in the water management infrastructure could generate data that is not in line with the normal behaviour and so the system can identify that and instead of keeping the same control strategy for opening specific valves, to maintain the level of the water, it can react in a different way, to implement a different strategy controlling other valves of the system. It would be relying on the meters that are more reliable and not on the measurements that are coming in from just one part of the system, which is not as reliable. “This means it can change the strategy of the control of the system, in order to implement the same objective, if in this example, the objective is to maintain an overall level of the water below a certain pressure.”
A key advantage of the approach is that when there are failures in a system it can redeploy the new node on the network to substitute one in this critical infrastructure. This is a step beyond a backup system for coping, as the system can reconfigure itself to allocate resources. It means less potential for interruption and less reliance on human intervention for critical decisions.
As Ferrera puts it: “One of the main objectives of this platform, that we are analysing in the implementation, is to make the life of the operators easier, so we are simplifying people’s work.” He continues: “For the operational phase, this will be an easier management platform. The developer can write the code and it is compiled, deployed and managed automatically.”
BRAIN-IoT’s stack is being released as an open source toolset, to make the benefits it promotes widely available for various industrial sectors.
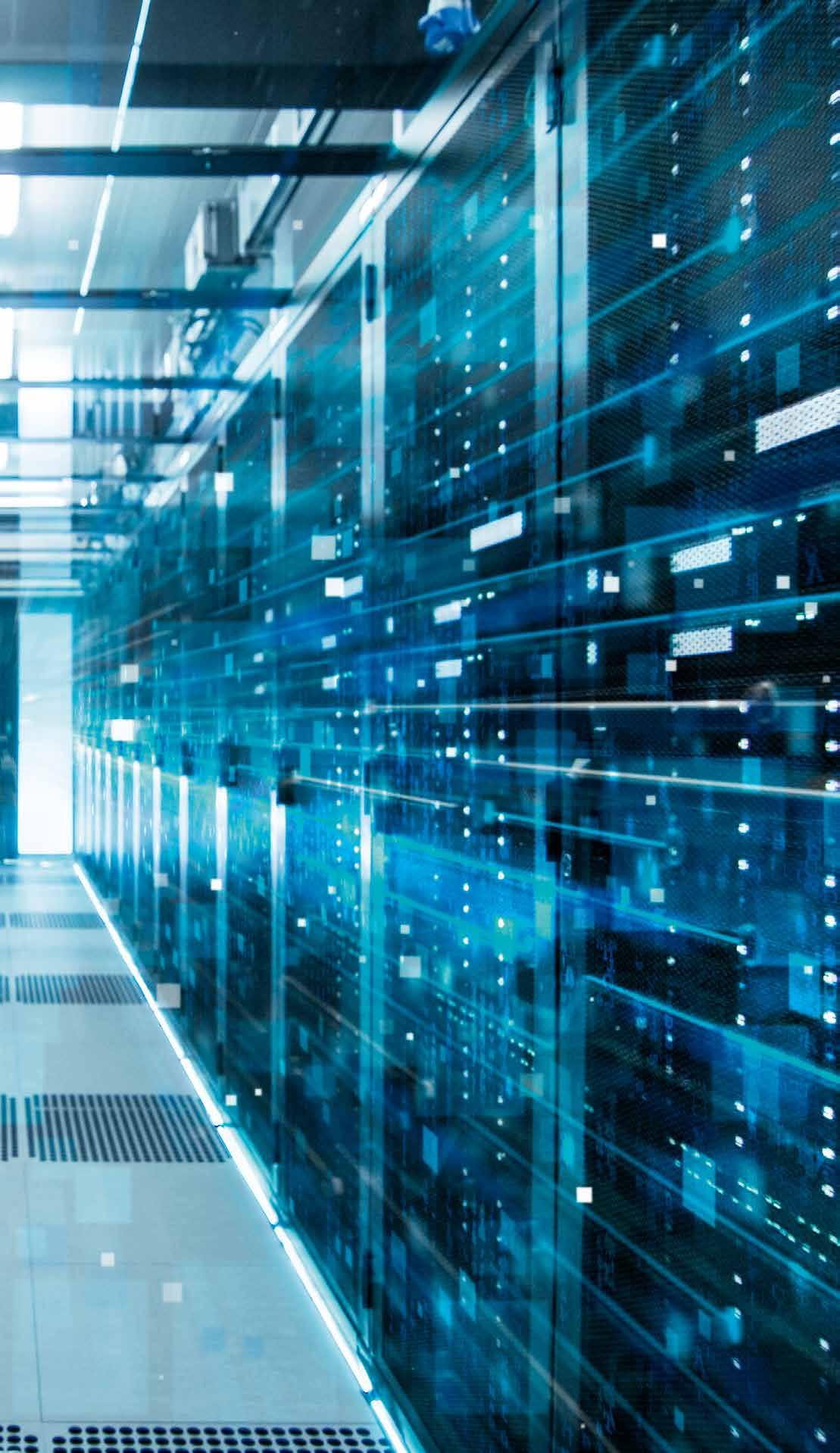

Service robotics use-case.
model-Based fRamework for dependable sensing and Actuation in INtelligent decentralized IoT systems Project Objectives
BRAIN-IoT provides a secure platform which adopts AI-enabled self-managing functionalities to implement resilient autonomous distributed systems for Smart Cities and Industry 4.0. Along with its modelbased Software Development Toolkit, the BRAIN-IoT platform aims at reducing the effort for developing and maintaining systems composed by heterogeneous IoT and robotic resources as well as external legacy services, operating in dynamic critical environments.
Project Funding
This project has received funding from the European Union’s Horizon 2020 research and innovation programme under grant agreement no. 780089. Overall budget: € 4 992 581. https://cordis.europa.eu/project/id/780089
Project Partners
• Fondazione LINKS, Italy– Project Coordinator • CEA List, France • Universite grenoble alpes, France • Paremus, UK • Improving Metrics, Spain • ST Microelectronics, France • Siemens, Germany • Eclipse Foundation, Germany • IDATE, France • Airbus Cybersecurity, France • Robotnik Automation, Spain • Empresa Municipal de Aguas de La Coruna, Spain • Kentyou, France
Contact Details
Project Coordinator, Enrico Ferrera Fondazione LINKS Via Pier Carlo Boggio 61 10138 Torino Italy T: +39 011 227 6710 E: enrico.ferrera@linksfoundation.com W: www.brain-iot.eu W: https://github.com/eclipse-researchlabs/brain-iot
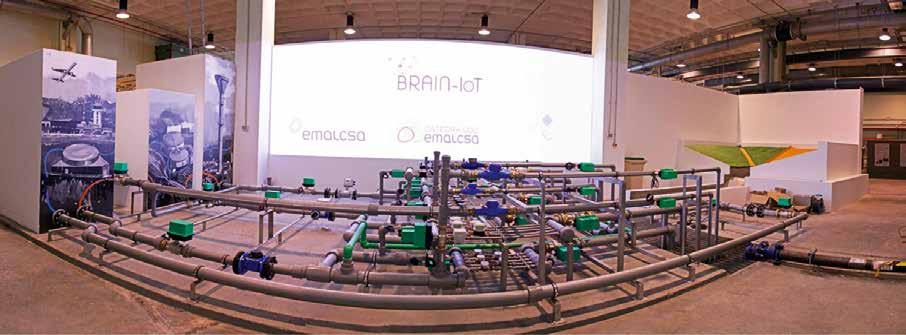
Enrico Ferrera
Enrico Ferrera leads the Distributed Intelligence and Secure Services research team of LINKS Foundation, Turin, Italy. He actively participates in national and international/H2020 EU research and large-scale pilot projects in IoT/ Robotics, distributed autonomous systems, process optimization domains. As coordinator of BRAIN-IoT, he is involved in the European Cluster of security and privacy projects.
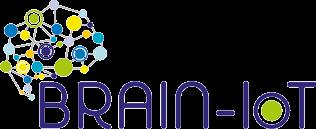
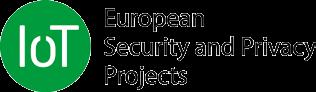
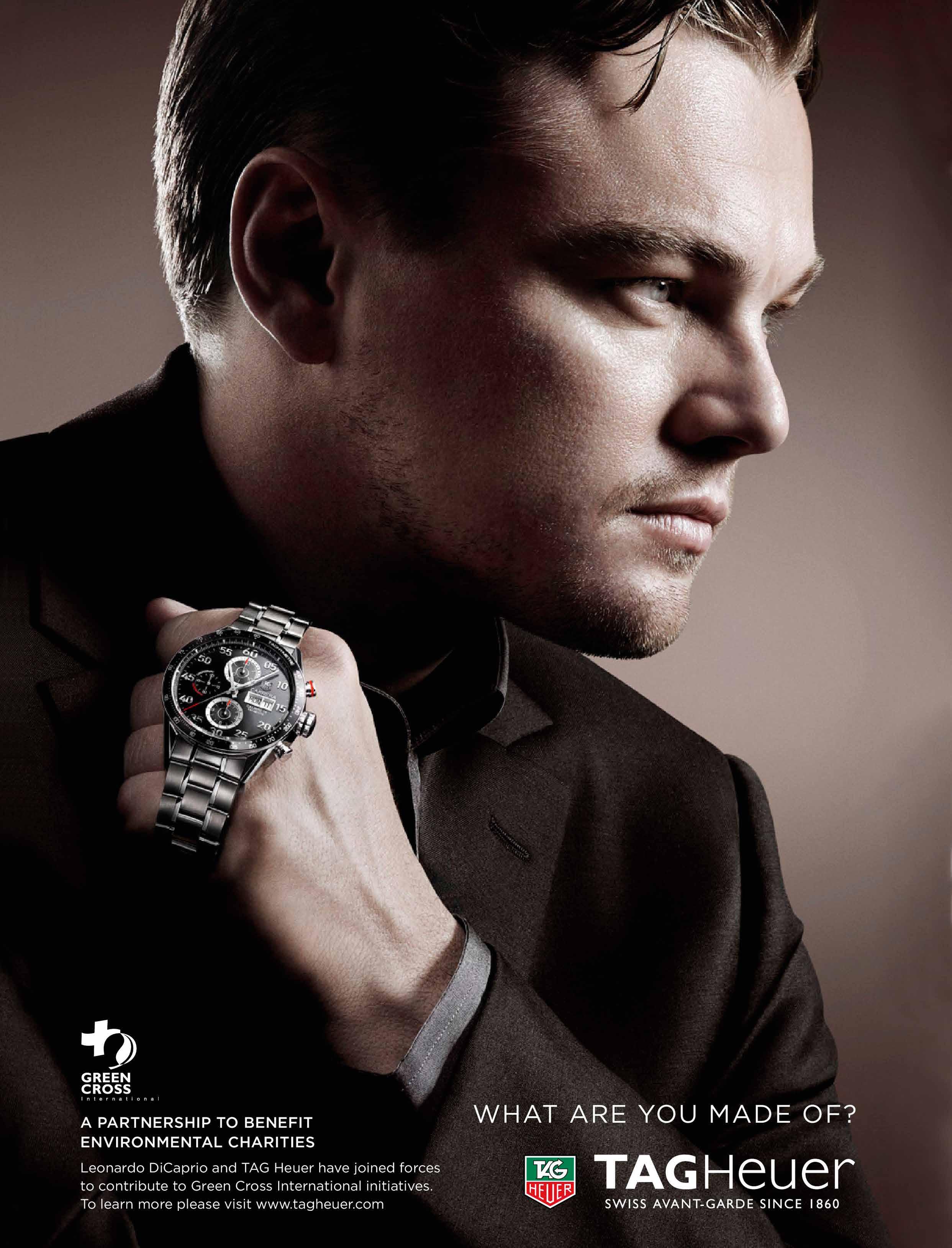