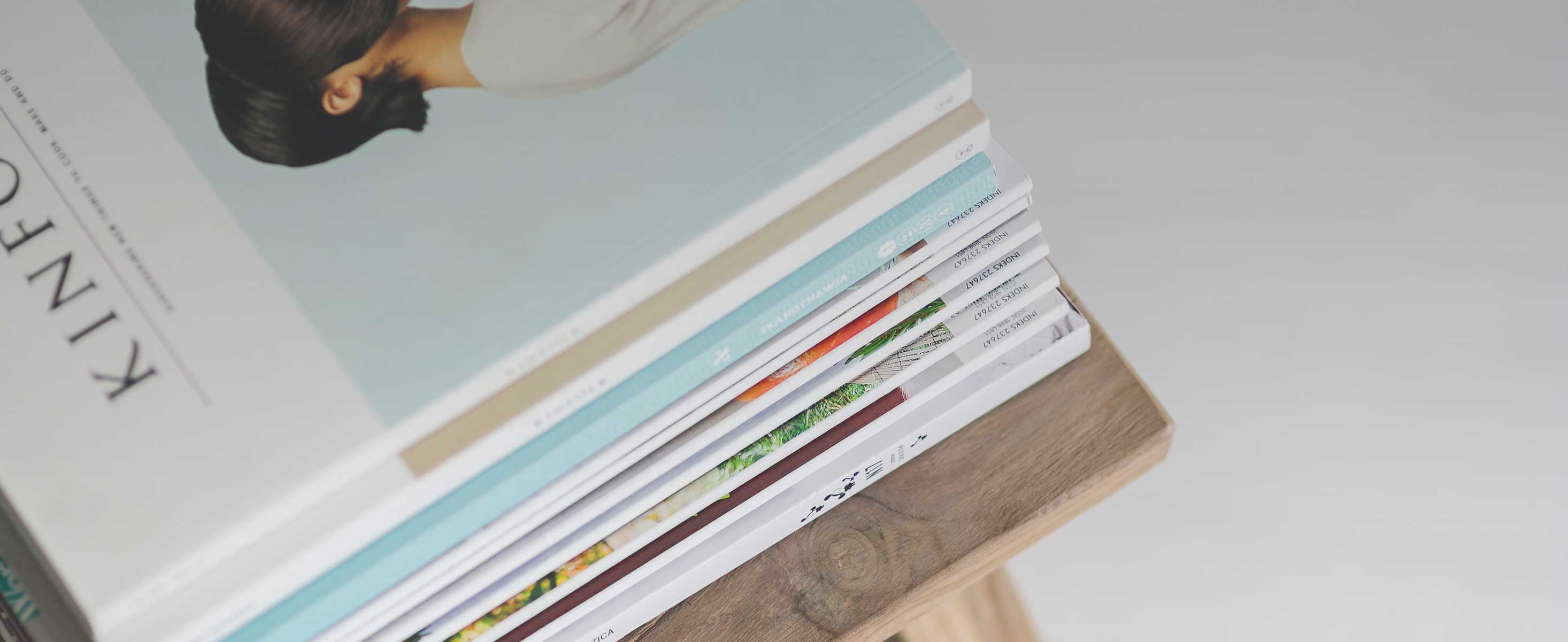
3 minute read
Tracking Keratoconus Progression with AI
Dermot McGrath reports from the 39th Congress of the ESCRS in Amsterdam
Artificial intelligence (AI) has the potential to improve on current methods of quantifying keratoconus detection and could help track eventual disease progression, reports Riccardo Vinciguerra MD.
Speaking at a Clinical Research Symposium, Dr Vinciguerra presented an overview of the current state of play in terms of AI applications in keratoconus diagnosis and progression.
The ideal preoperative assessment for patients with keratoconus should be reliable, easy, fast, and validated.
“It should have high sensitivity, which means you detect all cases at risk, but on the other hand, it should also have high specificity so we avoid excluding patients that would be fit for surgery,” he added.
Artificial intelligence—particularly statistical AI techniques such as logistic regression, random forest, neural network, and support vector machine—can assist in detecting and tracking keratoconus progression.
“The basic idea is we can use the data we derive from our devices to feed AIs to detect keratoconus and predict its progression. The ‘food’ we can provide to AI tools include topography, tomography, epithelial maps, aberrometry, corneal biomechanics, and biomarkers—basically everything that could help in the detection of the disease,” he said.
A common problem of many AI algorithms is while the methodology may have been published, they are still not widely used in clinical practice and their use has not been validated in external databases, Dr Vinciguerra explained.
Focusing on devices that currently use AI algorithms for keratoconus detection, Dr Vinciguerra said the OCULUS platform can use biomechanical data from the Corvis ST device and tomographical data from Pentacam®.
More specifically, the first AI tool on the Pentacam was the Belin Ambrósio Enhanced Ectasia Display (BAD-D) which uses logistic regression, has a cut-off range of 1.4 to 1.6 Standard Deviations (D values) from normal, uses multiple tomographic parameters, and has been widely validated.
Another algorithm, the Pentacam Random Forest Index (PRFI) uses more complex AI and has a cut-off range of 0.125.
“It has been shown to be better than BAD-D, particularly to improve the detection of early cases of keratoconus,” Dr Vinciguerra noted.
Another tool, the Corneal Biomechanical Index (CBI) has also been created with logistic regression, has a cut-off of 0.5, and its use has been validated in multiple papers, he added.
Promising results have also been obtained in published studies using a new combined tomographic and biomechanical index (TBI).
“It uses a random forest methodology, has a cut-off of 0.35, and is a significant improvement over CBI and BAD-D— particularly to detect early cases of keratoconus,” he said.
In terms of the future of detection methods, Dr Vinciguerra said while there have been many papers published on various AI algorithms, very few have been validated in external databases.
“Until we have a clear validation of the AI applications, we cannot be sure they are better than the current standard of care,” he said.
For detecting keratoconus progression, the commercially available platforms on the market do not use AI—rather, they depend on progression over noise level methods to flag it. However, a lot of interesting research is ongoing in this field, and new algorithms are sure to find their way into clinical practice in the near future, he concluded.
Riccardo Vinciguerra MD currently works as an Ophthalmologist and Scientific researcher at the Humanitas San Pio X Hospital, Milan, as well as a research collaborator at Biomechanical Engineering Group, University of Liverpool, UK. vinciguerra.riccardo@gmail.com