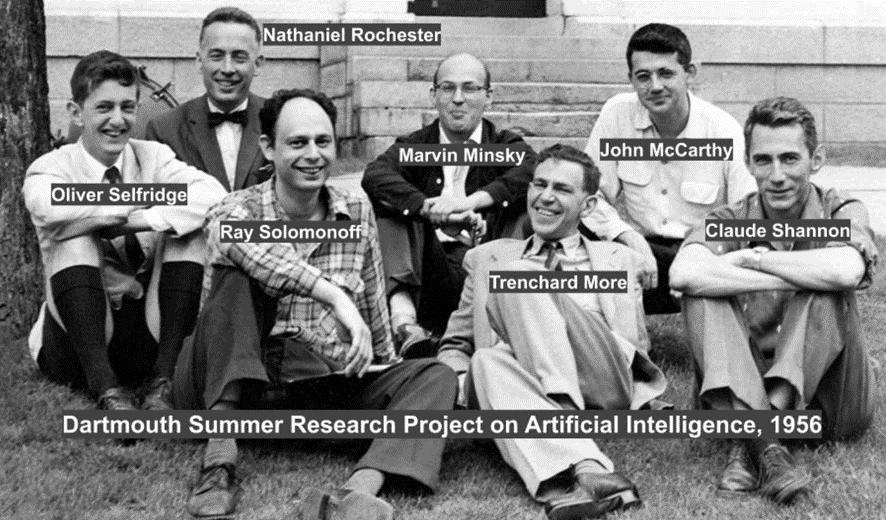
22 minute read
AI PIONEERS TIMELINE
The phrase ‘Artificial Intelligence’ was first coined by John McCarthy in 1955.
While working as a research scientist at Dartmouth College, McCarthy was composing a proposal for a workshop on "the study of automata," which he believed would bring researchers from many disciplines together to work on the challenge of building intelligent machines. He tried to find something more striking than “Automata” and nailed down the intriguing and catchy term of AI.
In 1956, he was one of the main organizers of “The Dartmouth Summer Research Project on Artificial Intelligence,” a six-week program gathering a group of scholars from diverse fields who were interested in building robots that could accomplish activities that would ordinarily need human intellect.
Besides McCarthy, the contributors were Marvin Minsky, Nathaniel Rochester, Claude Shannon,. Oliver Selfridge,Ray Solomonoff and Trenchard More.
The major objective of the workshop was to create a "thinking machine," computer software that could simulate human cognitive processes and find solutions to be issued logically. Natural language processing (NLP), problem-solving, pattern recognition, and learning were some of the subjects covered throughout the workshop by the attendees.
The 1956 Dartmouth Conference established AI as a separate field of study and is therefore regarded as a pivotal moment in the history of the discipline.
The first AI research labs and the first AI programs were developed as a result of the conference. The foundation was created for many of the advancements that would come in the following decades.
The next pioneers were Tom Mitchell, who developed the first machine learning (ML) algorithm, and Arthur Samuel, who in 1959 created the first self-learning program, dubbed the Samuel Checkers-playing Program (photo).

By assessing its own gameplay and changing its strategy as necessary, the program employed ML techniques to enhance its performance over time. Samuel's work paved the way for the creation of algorithms, decision trees and neural networks, which have since grown to be crucial resources in the field of AI.This was followed by the creation of the first neural network in the 1980s.
The backpropagation technique, created in the early 1980s by David Rumelhart enabled neural networks to be taught to spot patterns in data. This innovation made it possible to train multi-layer deep neural networks, which are currently widely utilized in functions like speech and picture recognition.
Still In the 1980s, Geoffrey Hinton is recognized as one of the forerunners of deep learning (DL). The very first neural network—called a “Boltzmann” machine—which could recognize and understand complicated correlations in data, was created in the 1980s by Hinton and his colleagues.
By creating a method known as deep belief networks, which are deep neural networks that can learn to represent complex data distributions, Hinton made important strides in DL in the following years.
Research into DL by Hinton has significantly influenced the field of AI, and his work has aided in the creation of systems for speech and picture recognition, NLP, and autonomous cars.
The development of the next discipline of reinforcement learning (RL) has been significantly aided by a number of pioneers over the years. Richard Sutton, who is recognized as one of the pioneers of the subject, is among the most significant individuals in the history of RL. He created the ‘temporal-difference learning’ framework for RL in the 1980s, which allowed agents to learn from experience more effectively than earlier techniques. Sutton is also credited for co-authoring the renowned book Reinforcement Learning: An Introduction, which has gained ubiquity as the main source of information in the area.
Richard Bellman, who is credited with first inventing the term ‘dynamic programming’ and set the framework for RL, is another well-known pioneer in the subject. Andrew Ng, who co-founded Google Brain and later launched the AI education company, Deeplearning.ai, is another.

All this seems a smooth ride but historically AI research has swung wildly in and out of favor. In the 1970s and the late 1980s to early 1990s, respectively, there were two AI winters hype cycles of inflated expectations that caused AI research to fail to live up to the over-anticipated reality.
Bell Labs was known as the epicenter of innovation for many years, and several of its scientists, including Yann Le Cun, Yoshua Bengio, and Geoffrey Hinton, were honored with Nobel Prizes and Turing Awards on AI related matters.
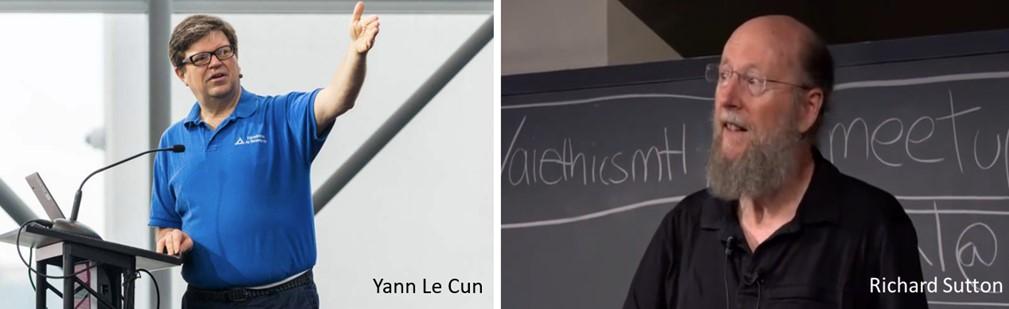
But the lab's resources were reduced as management began pushing for more immediate returns based on incremental technological changes, and it ultimately failed spectacularly to capitalize on the internet revolution of the early 2000s.
The downturns occurred when the hottest AI techniques of the time failed to demonstrate advancement and were unstable and challenging to operate.
Government organizations in the US and the UK that had supported AI research quickly concluded that this strategy was fruitless and stopped funding not least due to the drop off in defense-related spending and research after the end of the Cold War and the increasing demand for ‘immediate’ and ‘commercially-focused’ results.
However, the ‘main character’ moment in AI research is just currently taking place – more precisely, since very recently in the fall of 2022.
AI research is now finally fascinating, and we are witnessing weekly spectacular rollouts of systems that are pushing back the frontiers of what AI can do.
But it was not always ‘fashionable.’ In the 1990s, the reputation of the field was regarded as a strange, fringe activity by the larger computer industry, much how homeopathy is regarded by conventional medicine!
Neural networks, which made a significant advancement in the 1980s and mimic the patterns of the human brain, were the driving force behind the current surge in AI research.
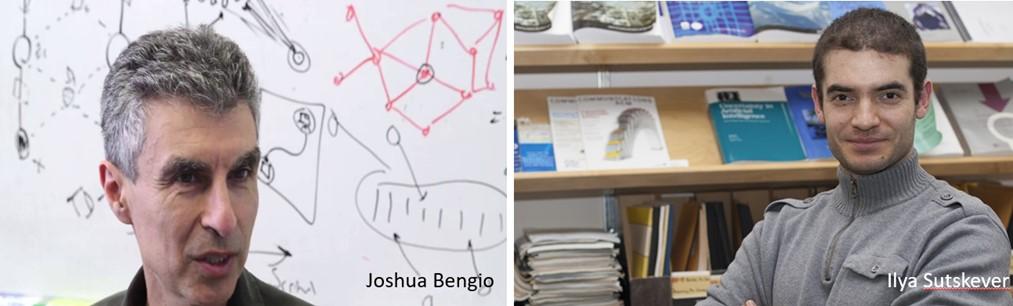
Since computers at the time could not run the software properly, technology hit a brick wall. This is the opposite of today where we have a lot of data available and incredibly powerful computers.
The AI moment is now! Google has declared a "code red" threat status for its key search product in light of the popularity of Open Ai’s Chat GPT; it is attempting to aggressively redesign its search engine with its own AI R&D.
The AI industry has a once-in-a-generation chance to experiment with the potential of new technology at this time. Despite the negativity, anxieties and fear, it's a fascinating moment to witness.
This is even more so with the coming out of large language models (LLMs), the talk of the town since November 2022. LLMs have been developed as a result of the contributions of numerous researchers but three stand out: Tomas Mikolow (Czech), Joshua Bengio (Canada), and Ilya Sutskever (Canada).
In the AI community today, it is Ilya whose brilliant mind is seen as being slightly ahead of the others.
Lately, his name has been in the press as a result of his work at Open Ai, while Sutskever, as one of Open Ai's co-founders, was instrumental in the creation of the company's most illustrious accomplishments, including the GPT-2 and GPT-3 language models.
Sutskever has made important contributions to the field of DL outside of his work at Open Ai, including the creation of the Sequence to Sequence (Seq2Seq) model for machine translation, which has grown to be a popular method for translating text between different languages.
He has also contributed to significant AI research in other areas like generative modeling and RL.
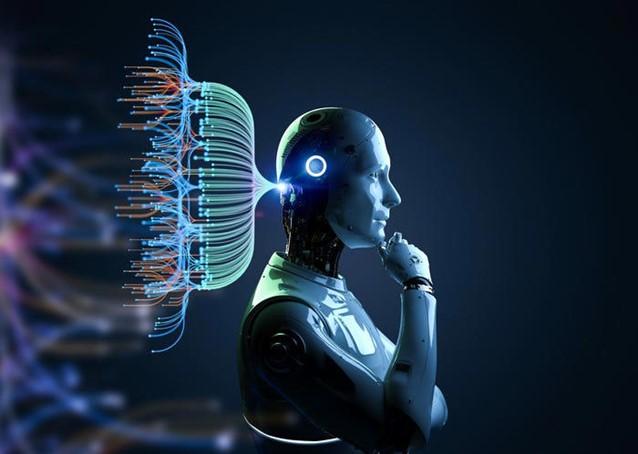
Wehavevisitedallthesignificantpioneers,butthousandsoflesser-known researchersbravethetwo “Winters”ofresearchandmadesignificantcontributions tothenextandrecentrapiddevelopmentofAI.
Anewgenerationofcreativemindsandexcitingtechnologicalandscientific advancementswillundoubtedlyresultfromthenewAItoolsthatarecurrentlyopen toallacademicsatlowornocost .
AIisnotanymore,ascientistfantasyland. Withtheenergyofthenewgenerationshiningbrains
Weshallbereadyforaradiantfuture
Ihearyoucoughingandgrumbling!
Waittoreadmore, Youarejustatthestart
Beforemakingyourownconclusion
Asking Ai To Explain Himself
This chapter will offer you a brief overview of AI and how it functions, whether you are a total beginner or just seeking to brush up on the fundamentals.
What exactly is AI?
AI describes computer programs that are capable of learning, solving problems, and making decisions tasks that would typically need human intellect of any required level. The two basic classifications of AI can be considered with broad brushes as follows. Strong AI is capable of performing any intellectual work that a human is capable of, while narrow AI is created to execute a specific task like in robotics or such as playing chess
How does AI function?
Algorithms, which are collections of guidelines that direct computers to what to do, are used by AI systems. These algorithms are made to learn from data and form hypotheses or judgments based on it.
Now after this extremely basic introduction let’s move to the next step. Being a solid partisan of creativity, as you know it’s now three years since publishing my first Futurology Chronicle – where I am writing all of my text, A to Z.
As the opportunity presented itself this time, I asked Generative AI, to work at my place, and express itself on this vast domain. Accordingly, I used with Chat GPT 3.5 (No 4 is still in final testing phase) to present in intelligible terms the AI basics for beginners.
You will read below my prompt or question and the ultra-fast immediate replies. I have underlined certain important wording and added some minor comments in hard brackets, but the replies are untouched and totally ‘in texto’. **BEGINS**
Q:Algorithmaretrainedunderthreemodels.Canyoudescribethemin300words? [Foursecondslater….]
A: Certainly! Here is a concise explanation of the differences between machine learning, deep learning, and reinforcement learning:
Machine learning is a branch of artificial intelligence that involves training algorithms to recognize patterns in data. These algorithms can be used to make predictions, recognize images or speech, or perform other tasks that would be difficult or impossible for humans to do manually. Machine learning algorithms learn from data without being explicitly programmed, and can improve their performance over time as they are exposed to more data.
Deep learning is a type of machine learning that involves training artificial neural networks with multiple layers to recognize patterns in data. These networks are modeled after the structure of the human brain, and can learn to recognize complex patterns in data such as images, speech, and text. Deep learning has been used to achieve breakthroughs in areas such as computer vision, NLP, and speech recognition.
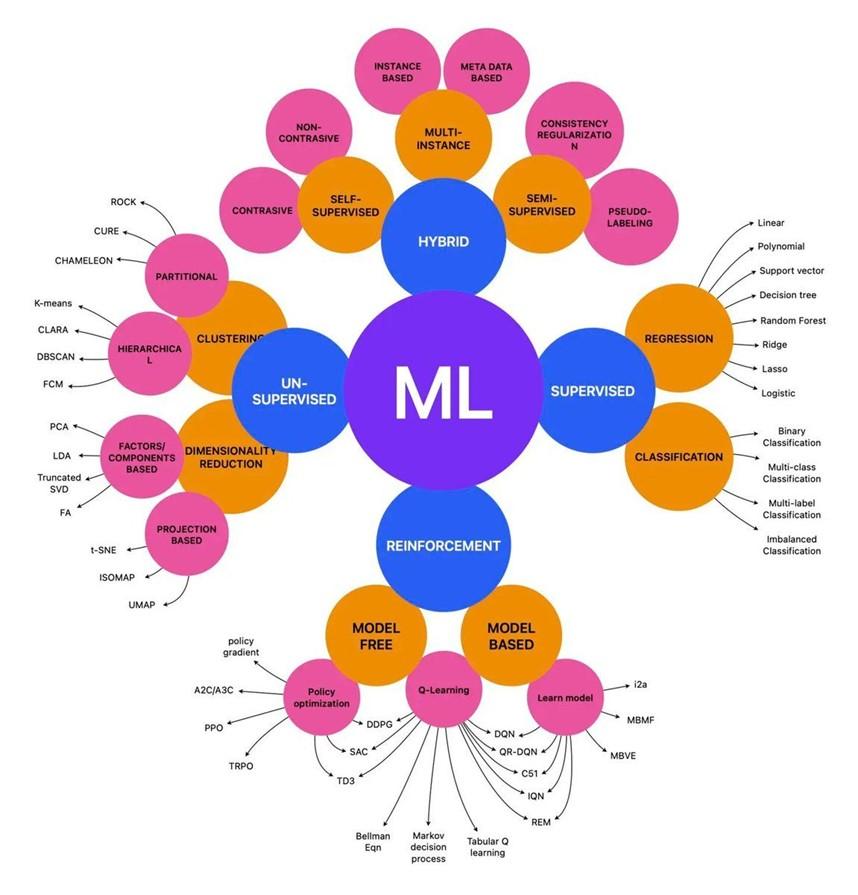
Reinforcement learning is another type of machine learning that involves training agents to make decisions based on the rewards or punishments they receive from their environment. This type of learning is often used in robotics, gaming, and other applications where an agent needs to learn how to navigate a complex environment and make decisions in real-time. Reinforcement learning algorithms learn by trial and error, and can improve their performance over time as they receive feedback from their environment.
In summary, machine learning is a general term for algorithms that learn from data, while deep learning is a specific type of machine learning that uses neural networks with multiple layers to recognize complex patterns. Reinforcement learning is a type of machine learning that involves training agents to make decisions based on feedback from their environment.
Q:WhenAlgorithmarenotusingneuralnetworkswhatelsedotheyuse?? [Fasterandfasterreply…1.5seconds.GPTrecognizedthatweareonthesame patternofrelatedquestions.]
A: In addition to neural networks, various methods can be used by machine learning algorithms to learn from data. Non-neural network machine learning methods come in a variety of forms, such as:
Decision Trees: A decision tree is a flowchart-like structure where each internal node represents a “test” on an attribute, each branch represents the outcome of the test, and each leaf node represents a class label. Decision trees are often used for classification tasks, such as predicting whether a customer will churn or not.
Support Vector Machines: A support vector machine (SVM) is a type of algorithm that tries to find a hyperplane that separates different classes of data. SVMs can be used for both classification and regression tasks.
K-Nearest Neighbors: The k-nearest neighbors (KNN) algorithm is a type of instance-based learning where the algorithm finds the k data points that are closest to a new data point, and predicts the class label of the new data point based on the labels of the k-nearest neighbors.
Random Forest: A random forest is an ensemble learning algorithm that constructs a multitude of decision trees and combines their predictions to improve the accuracy and reduce overfitting.
Naive Bayes: A naive Bayes algorithm is a probabilistic classifier that uses Bayes' theorem to predict the probability of each class label given a set of features. Naive Bayes assumes that each feature is independent of the others, which is often not the case in real-world applications.
These are just a few examples of machine learning algorithms that do not use neural networks. Each algorithm has its strengths and weaknesses and is suited for different types of problems.
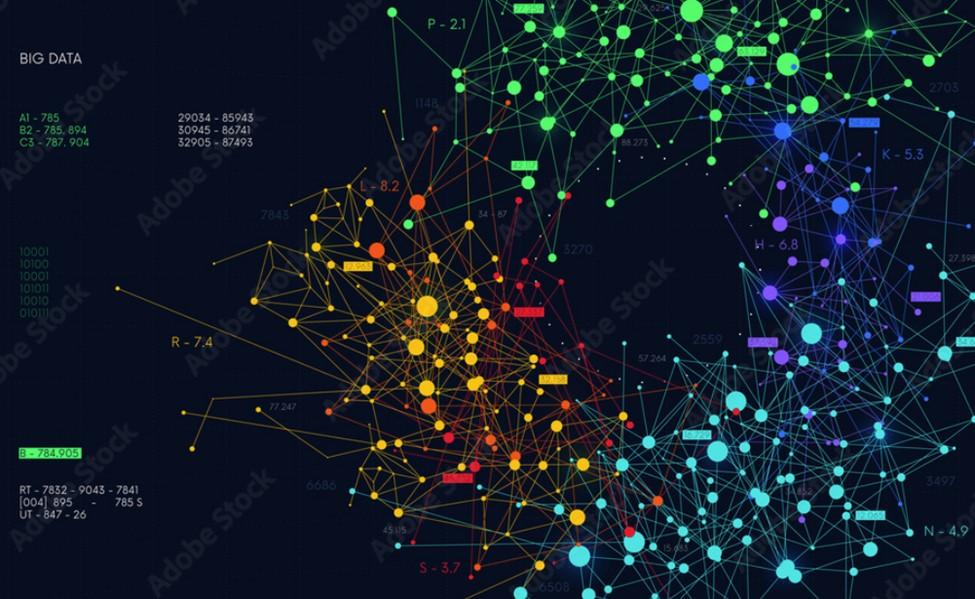
Q:Whatisthemostusedneuralnetworksbetweenthesefivetechniques. [2.8secondsonmymobiletimer.Slowingdown… sortof… duetotheintricaciesof thequestionwiththeprecedentone.]
A: Within the category of deep neural networks, some of the most commonly used architectures include:
Convolutional Neural Networks (CNNs): CNNs are often used for image and video recognition tasks. They apply a set of learnable filters to the input image to extract relevant features and classify the image.
Recurrent Neural Networks (RNNs): RNNs are used for sequential data processing tasks, such as speech recognition and natural language processing. They have a "memory" component that allows them to keep track of previous inputs and use this information to predict the next output.
Long Short-Term Memory Networks (LSTMs): LSTMs are a type of RNN that are designed to avoid the "vanishing gradient" problem, which can occur when training traditional RNNs on long sequences of data.
Generative Adversarial Networks (GANs): GANs are used for generative modeling tasks, such as generating realistic images or videos. They consist of two networks, a generator network that produces the output and a discriminator network that tries to distinguish the generated output from real data.
Transformer Networks: Transformer networks are a type of neural network architecture that have been used to achieve state-of-the-art performance on NLP tasks, such as language translation and question answering.
While deep neural networks are currently one of the most popular techniques in machine learning, the choice of algorithm ultimately depends on the specific problem being addressed and the characteristics of the data.
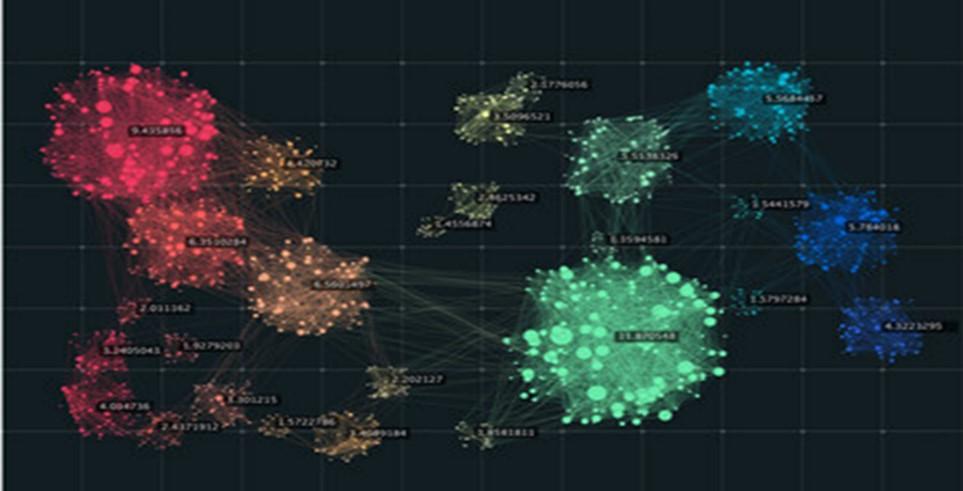
Q:IamreadingalotaboutANNpotential.Pleasedescribeinsimpleterms?
[Twosecondslater…]
A: ANN stands for Artificial Neural Network, which is a type of machine learning algorithm inspired by the structure and function of the human brain.
ANNs consist of multiple layers of interconnected nodes, called "neurons," that can learn to recognize patterns in data through a process called training.
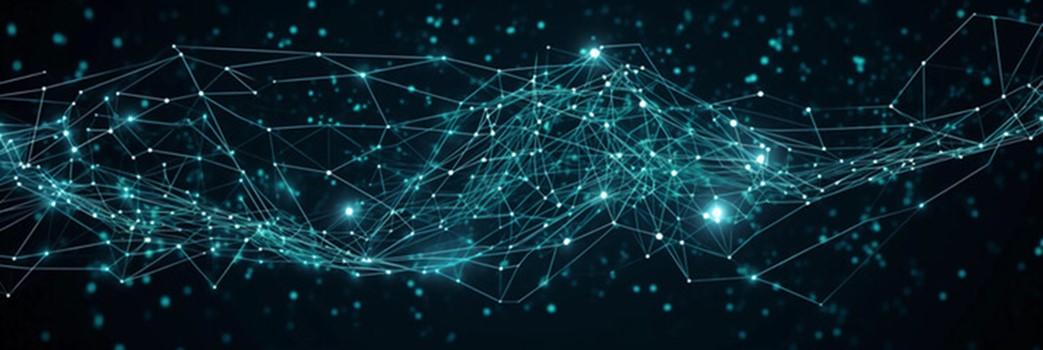
In an ANN, each neuron receives input from other neurons and processes that input using a set of learnable parameters, known as weights.
The output of the neuron is then passed to other neurons in the network, and the process is repeated until the final output is produced.
ANNs are used for a variety of tasks, including image and speech recognition, NLP, and control systems.
They can learn from large amounts of data without being explicitly programmed, and can be used to make predictions, classify data, or perform other tasks. There are different types of ANNs:
Feedforward neural networks are the simplest type of ANN, with inputs that are processed through one or more hidden layers before producing an output.
Recurrent neural networks are designed to process sequential data, such as speech or time-series data.
CNNs are designed to process spatially structured data, such as images or videos. Overall, ANNs are a powerful tool in machine learning, with applications in a wide range of fields including computer vision, NLP, and robotics.
Q:Allisratherclear,butallthesearestandardAItechnologyoftheday.Whatare themodelsoftomorrow?
[Ifeltsomehesitationhere,andittookfivesecondsthistime…foraprudentreply!]
A: As an AI language model, I cannot predict the future or anticipate new technologies that may emerge.
However, I can provide some insights into current research and developments in machine learning that may point towards future directions.
One area of research that is receiving increasing attention is called ”meta-learning," or learning to learn. Meta-learning involves training models that can learn how to learn from a small amount of data, which could lead to more efficient and adaptive learning models in the future.
Another area of interest is "Explainable AI," which involves developing machine learning algorithms that can provide explanations for their decisions or recommendations. This could help to increase the trust and transparency of AI systems and could be especially important in applications such as healthcare or finance.
In addition, there is growing interest in "unsupervised learning," which involves training models without the use of labeled data. This could be particularly useful in situations where labeled data is scarce or expensive to obtain.
Finally, "reinforcement learning" is an area of machine learning that is still evolving and could see new developments in the future.
Reinforcement learning involves training agents to make decisions based on the rewards or punishments they receive from their environment and has been used in applications such as robotics and gaming.
These are just a few examples of areas of research that could lead to new learning models in the future. As technology continues to evolve, we can expect to see new developments in machine learning that will shape the future of AI. **ENDS**
So,inconclusion
BeingabletowatchtheAI'sspeedandhesitancyinresponsetothequeriesin secondsgivesmeasenseofintellectualsatisfaction.
EventhoughI'vebeenfamiliarwithalltheAIjargonanditsvariationsforawhile— Chronicleno3ofMarch2021wasanintroductiontotheAIsector–itwasobviously efficientforAItorespondaboutitsownfield.
IthinkeasilythatIwillneverhavethespeedorabilitytoexpressalltheseconcepts insimpleterms,farfromit.,
1527wordsofthiscaliberwouldrequiremetowriteit,revise,andcleanitupover twohoursasopposedto15.3secondsoverallforChatGPT.
GenerativeAIisourgo-toassistantforeveryresearchproject,workingfastto increaseouroutputacrossallindustries.
Weareallbetteroffquicklybecomingaccustomedtothisnew,soon-to-beessential working ‘assistant’thanthecryingwolfinthedarkforitsbiasesandotherirrational anxieties.
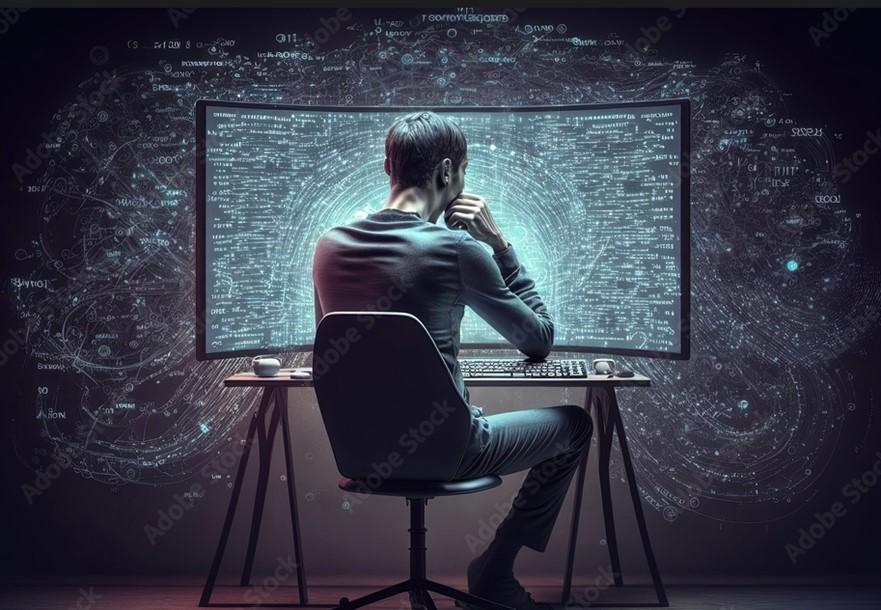
THE LAMP GENIE: TO RUB OR NOT?
Science fiction authors, ethicists, and scientists have long been fascinated about AI that develops abilities against the will of its programmers.
An arresting illustration from mid-April (on 16th): the Google data scientist team found that despite not having been educated to know Bengali, its system was able to teach itself the language. They found that it can now translate all of Bengali with only a small amount of prompting in that language.
The team responded with excitement, enthusiasm, and anxiety. How can we now control an AI that learns on its own, with very few components to begin with, that goes against the million pieces of information in DL we believed it needed to be properly trained?
When a journalist (CBS) pressed Sundar Pichai, the CEO, for an explanation, he responded that some aspects of how AI systems both learn and act continue to astound both us and our top experts. Humm really!
He continues saying that internally when we do not fully comprehend and are unable to determine why AI said something in a certain way, we refer to this internally as the "black box mystery."
He makes then the easy official claim that additional research is necessary before we can fully understand how it operates and came up with a non-acceptable off-ramp dumb citation:
A low level of diplomatic sheer escapism for someone in this position!
The problem is now evident to everyone that the Big Tech Big Boys have been caught off guard by their own fast, billion-dollar AI R&D success.
In a well-known open letter signed by big wigs like Musk, Thiel, and thousands of other tech titans, data science professors and luminaries, they demanded a "pause" in the creation of more potent models.
A pause. It is an utterly cynical easy term to describe a budget halt in the race to create the next generation.
The letter to read is here: Pause Giant AI Experiments: An Open Letter - Future of Life Institute
Open Ai CEO, Sam Altman, received criticism for declining to sign the letter with his colleagues (including his former associate Elon Musk) but then went on his own, on 18th April, that he would not advance further than GPT-5. Exactly following his colleagues “pause” signal! If Sam is also very concerned, we should all be alongside him.
So, now they all have their feet on the brake – but for how long until corporate competition comes back? The letter asks for a six-months pause. Frankly, do you see all these data scientists’ top brains going for a long holiday in Cancun?
It is pure fantasy, but on the other hand that letter did not miss the point that they have been moving too fast without any strings attached, and that it is time to calm down and go back to the AI ethics founding term, called the “Asilomar Accord.”
Asilomar is a small conference town near Monterey, California, which had its illustrious debut with a group of scientists who met in February 1975 to talk about the advantages and disadvantages of genetic engineering. There, they signed an international agreement on ethics.
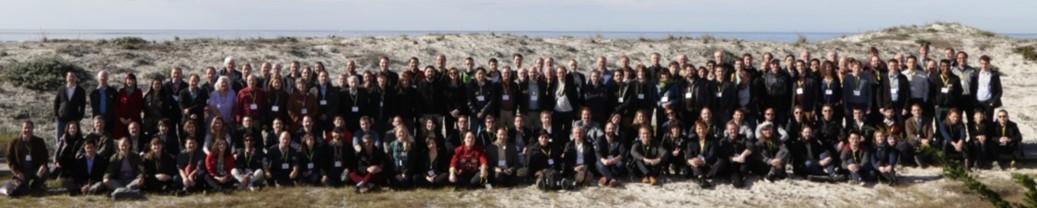
On the same ethics philosophy debate, top AI researchers (800+) convened in 2017 at the same place on the potential drawbacks and advantages of AI technology. Theycan’ thide.TheywereallpresentonAugust11th,2017,
The Asilomar AI principles (5720 signatories) set forward several safety precautions and moral principles for the creation and application of AI systems in the same pattern as the Asilomar Accords for genetic engineering. The guidelines take transparency, justice, privacy, and the potential social effects of AI into account.
The official documents with the participants’ list: AI Principles - Future of Life Institute
On purpose, as it was a bit of a long search to find them properly, I asked Chat GPT for assistance in summarizing the Asilomar AI principles in bullet points. It came back in less than three seconds (!) and is below with no alterations:
Research Goal: The goal of AI research should be to create safe and beneficial AI.
Safety: AI systems should be designed and operated to avoid unintended negative consequences.
Transparency: The operation of AI systems should be transparent to allow for scrutiny and accountability.
Fairness: AI systems should be designed and operated fairly, without bias or discrimination.
Privacy: People should have the right to privacy regarding the data collected by AI systems.
Controllability: People should be able to control and override the actions of AI systems that directly affect them.
Lawfulness: AI systems should be developed and operated in accordance with the rule of law.
Ethical Considerations: AI systems should take ethical considerations into account, such as avoiding harm, respecting human dignity, and promoting human well-being.
Societal and Environmental Well-Being: AI systems should be developed and deployed to promote societal and environmental well-being.
Back to ‘human’ writing …
These beautiful minds are returning to the Asilomar Accords that they have neglected in their haste to advance, having moved too fast without a clear understanding of what they were doing especially now that that they are being watched by everyone on Earth who are catching up all the daily AI news on “anxiety” meds (!).
The entire community of data scientists should take the principles they all defined back in 2017 very seriously and make genuine commitments. To align with the values and interests of society, it has to be hoped that the next phase of AI research will be conducted responsibly for all humanity and not only for Big Tech’s classic greed.
Wishfulthinkingorotherwise?
HastheGenielampalreadybeenrubbed,ornotyetcompletely….?
COUNTRIES ON THEIR HIGH REGULATORY HEELS Vs AI
The necessity for regulations regarding the use of artificial intelligence (AI) is growing as this technology does.
The area of law known as AI law is focused on figuring out the subtleties of AI and how it will affect society and the future.
From the ethical ramifications of AI to the economic and legal ramifications of the technology, the legal field of AI law addresses a broad variety of concerns.
In 2022, 37 related AI laws addressing global artificial intelligence were enacted by legislative bodies in 127 nations.
The United States took the top spot on the list, passing nine laws, followed by Spain with five laws and the Philippines with four laws. These laws address education changes to address the difficulties brought on by new technologies, including AI.
Worth noting, the Spanish legislation emphasized nondiscrimination and accountability for AI algorithms.
123 AI-related laws have been enacted across 123 nations since 2016, with a rapid increase starting from 2021.
This looks new for the public but very early in 2018 United Kingdom created a Centre for data ethics and Innovation to provide guidance and advice on the ethical use of date and AI.
In 2019 Canada release a directive on automated decision making that requires federal government departments to provide explanations to individuals when automated decisions are made that affect them.
On the same wavelength Singapore created an AI Model for governance framework to provide guidance on the responsible use of AI in various industries.
EU have the solid base of the general data protection regulation (GDPR ) that regulates the collection , processing and storage of personal data of EU citizens ,but this was clearly not enough and don’t include properly all the new elements coming from AI
A white paper followed by a draft legislation by the EU Commission was published in April 2021. It suggests a legal framework for AI in the EU with provisions for openness, responsibility, and the moral application of AI included in the suggested framework. A European approach to artificial intelligence | Shaping Europe’s digital future (europa.eu)
The AI Act, the mother of all artificial intelligence regulations, is what the European Union believes to be the answer. It is the first law that tries to reduce these effects by regulating the entire industry. It was released on 28 March 2023 and shall be voted in October after the summer recess.
Veryambitiousatscale
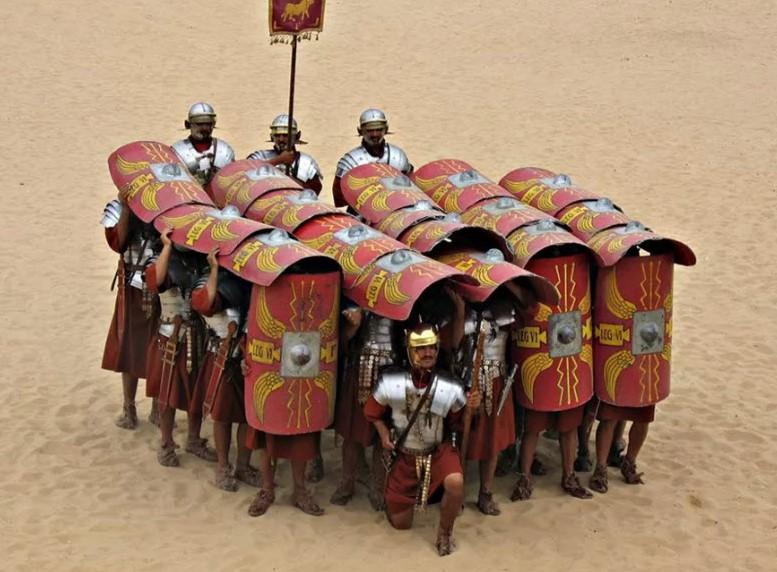
The AI Act has a very lofty goal. Additional oversight would be necessary for "high risk" applications of AI that pose the greatest risk to human safety. This could apply to tools used in hiring staff, grading tests, or assisting judges with legal and judicial decisions.
The bill's initial draft also prohibits the use of AI for "unacceptable" purposes, such as assigning people a trustworthiness score.
The bill would also limit the use of facial recognition technology by law enforcement agencies in public areas.
There is a vocal group of influential people who demand a complete ban or moratorium on its usage in public by both law enforcement and private corporations, claiming that the technology allows for mass surveillance. This group includes members of the EU Parliament and nations like Germany.
Howwillitimpactpeople?
By making sure that applications are subject to at least some amount of inspection and accountability, it should, in principle, shield humans from the worst effects of AI. According to Brando Benifei, an Italian member of the European Parliament and a significant contributor to the team revising the legislation, people can have faith that they will be shielded from the most dangerous types of AI.
The legislation mandates that people be informed when they come across deepfakes, biometric identification tools, or AI programs that assert to be able to read their emotions. Legislators are also debating whether the law needs to establish a process for those who have been harmed by an AI system to file complaints and seek remedies. Predictive policing technologies are also being pushed for a ban by the European Parliament, one of the EU institutions working on changing the law.
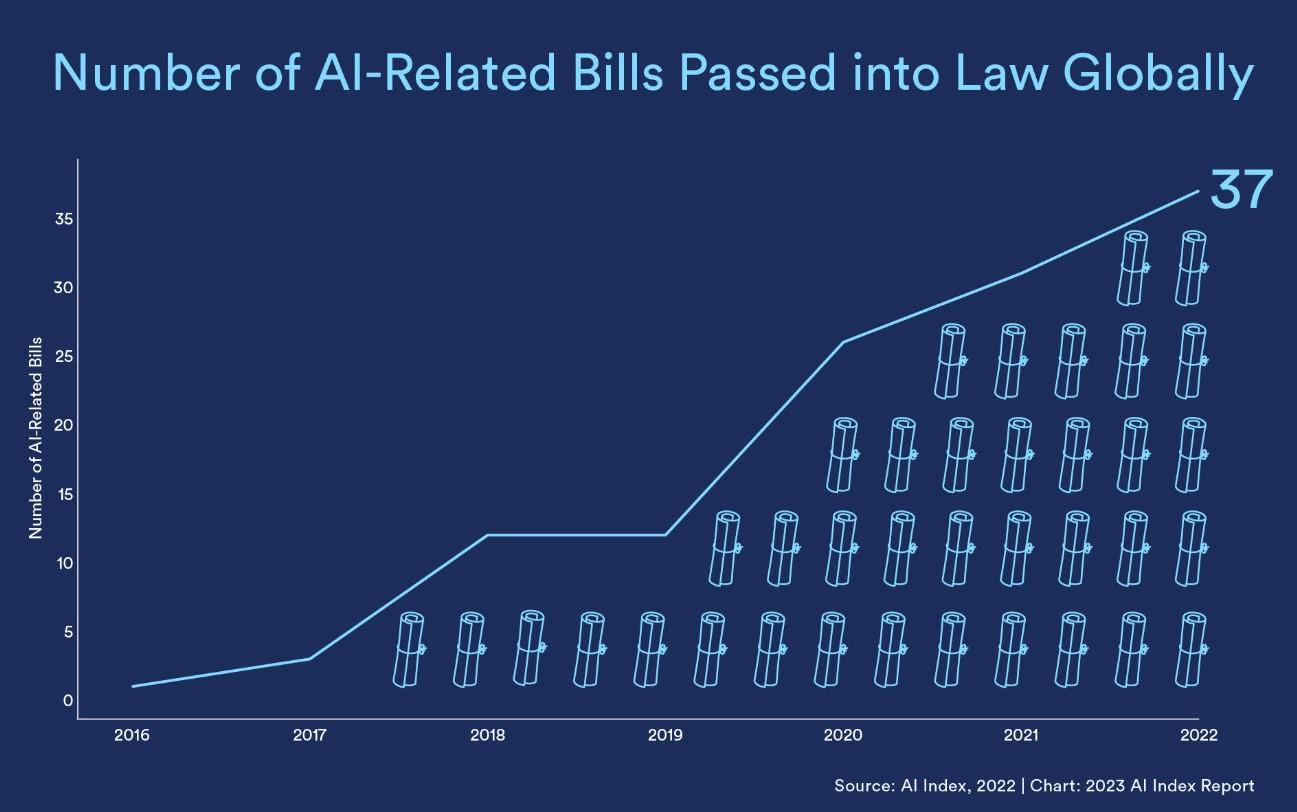
These systems employ AI to evaluate massive data sets in an effort to either forecast a person's prospective criminality or to dispatch police in advance to crime-prone locations. These systems are quite contentious, and their detractors claim that they frequently display racism and lack transparency.
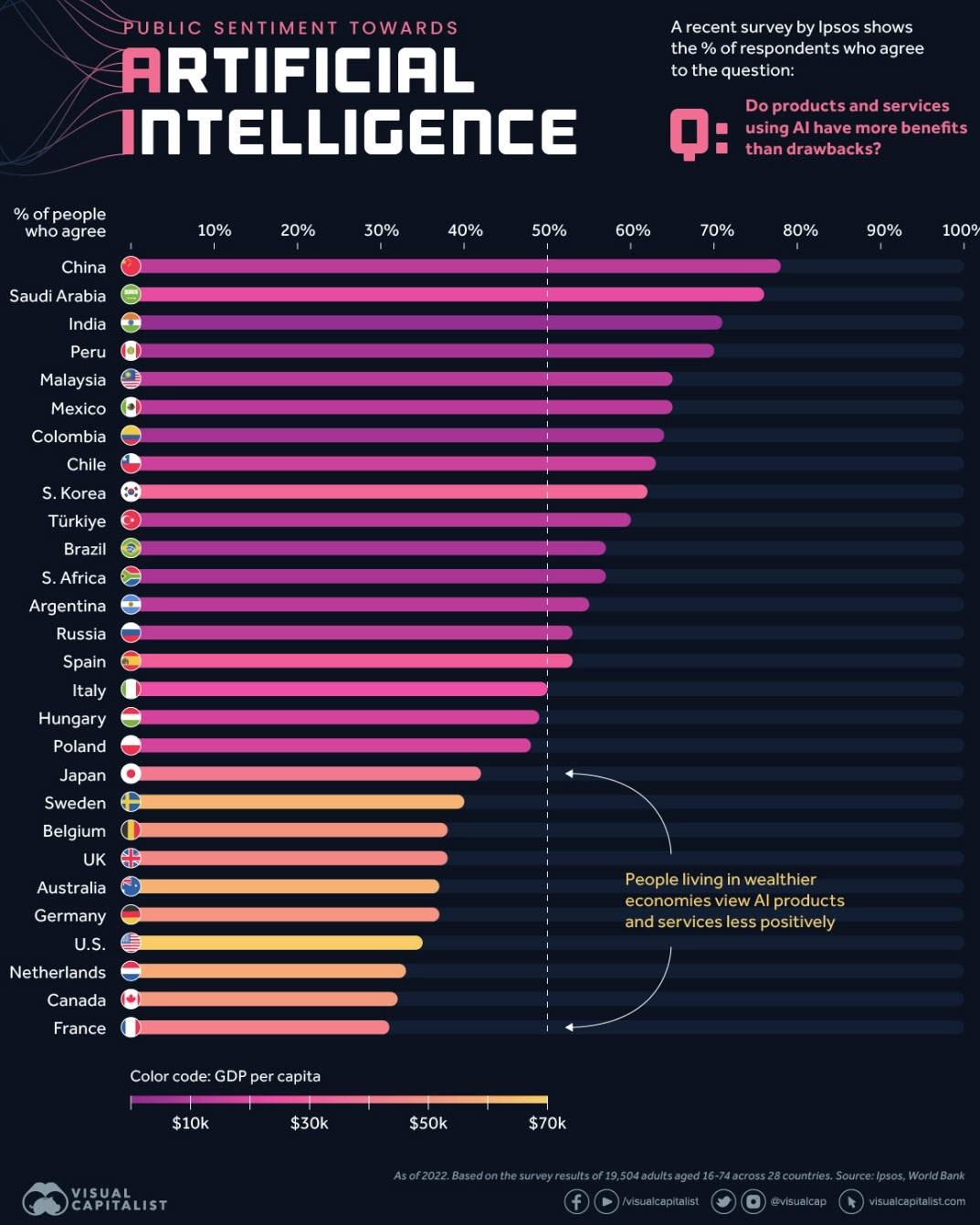
Whatdifficultiesarethegreatest?
Some of the provisions of the bill are currently technically impractical to implement. The bill's initial version stipulates that data sets must be error-free and that people must be able to "fully understand" how AI systems operate.
The size of the data sets required to train AI systems makes it impossible for a person to verify that they are truly error-free without investing countless hours of labor.
Furthermore, modern neural networks are so complex that even their designers are unsure of how they come to their conclusions.
Tech firms are also quite uneasy about needs to grant access to their source code and a lgorithms to external auditors or regulators in order to uphold the law.
"The current drafting is creating a lot of discomfort because people feel that they actually can't comply with the regulations as currently drafted," says Miriam Vogel, president and chief executive officer of Equal AI, a nonprofit organization whose goal is to lessen implicit prejudice in AI systems. She also serves as the chair of the recently established National AI Advisory Committee, which provides the White House with AI policy advice.
There is also a heated debate over whether the AI Act should outright forbid the use of facial recognition. It's controversial because EU nations despise when Brussels tries to tell them how to handle concerns of law enforcement or national security.
Many nations, including France, want to grant exceptions for using facial recognition technology to safeguard national security. In contrast, the newly elected government of Germany, a sizable European nation with a significant say in EU policymaking, has stated that it is in favor of a complete ban on the use of face recognition technology in public spaces.
What types of AI are labeled as "high risk" will be the subject of yet another heated debate. The list of items covered by the AI Act includes everything from lie detectors to welfare payment distribution systems.
There are two competing political camps: one worries that the extensive regulation would stifle innovation, while the other claims that the bill's current form won't adequately safeguard citizens from grave harm.
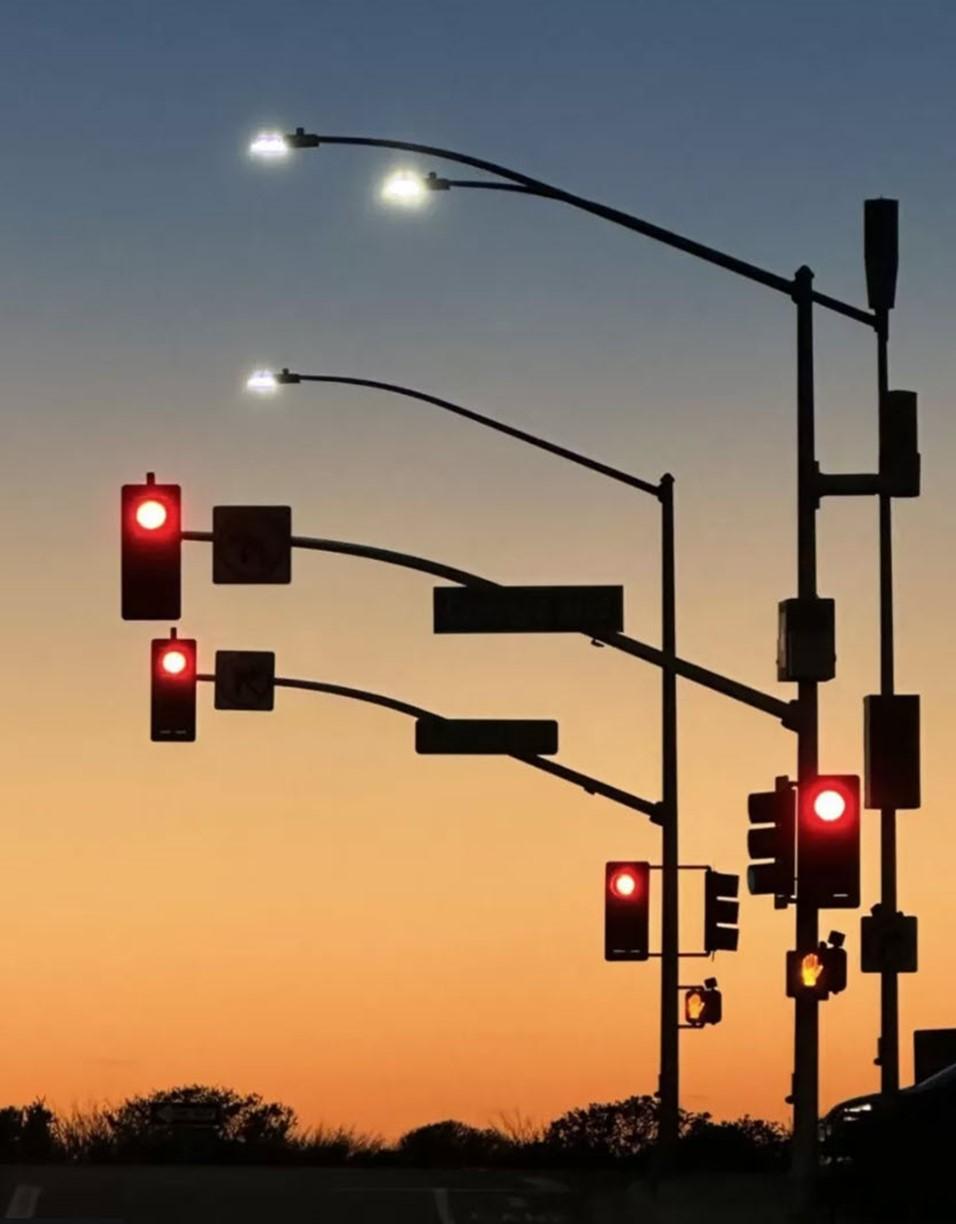
Willthispreventinnovation?
Silicon Valley lobbyists frequently complain that the regulation will burden AI businesses with additional red tape. Europe is in disagreement.
The EU responds by claiming that the AI Act will only be applicable to the riskiest types of AI applications, which the European Commission, the EU's executive body, predicts will only account for 5 to 15% of all AI applications.
Tech firms "should be reassured that we want to give them a stable, clear, legally sound set of rules so that they can develop most of AI with very limited regulation," says Benifei.
Failure to comply can result in fines of up to €30 million ($31 million) or, in the case of corporations, up to 6% of annual global revenue. And history demonstrates that Europe is not averse to fining tech corporations.
For violating the GDPR, Amazon was fined €746 million ($775 million) in 2021, while Google was fined €4.3 billion ($4.5 billion) for breaking the EU's antitrust laws in 2018.
Whenwillitbecomeactive?
Before a final text is enshrined in law, at least another year will pass, and it will take a few more years before businesses must abide by it. It's possible that working out the specifics of a complete measure with so many difficult issues would take much longer.
The GDPR was negotiated for more than four years, and it took another six years for it to go into effect. Anything is possible in the world of European Union lawmaking.
One of the greatest technical restrictions to date would be achieved if the EU is successful in doing this. Facial recognition is subject to limitations in some US states and cities, such as San Francisco and Virginia, but the EU's ban would cover 27 nations with a combined population of over 447 million people.
If the EU is successful, it might establish a new international standard for AI oversight. The EU's Artificial Intelligence Act, explained | World Economic Forum (weforum.org)
They need to act fast to avoid a wind of excessive fear like the one expressed by one of his members: Italy
The Italian government's, via the Italian data protection authority, block and ban the use of Chat GPT 3 on 31st march 2023 with immediate effect,
The Italian data-protection authority said Open AI had one month to say how it would address the watchdog's concerns, under penalty of a fine of €20 million or up to 4% of annual revenues.
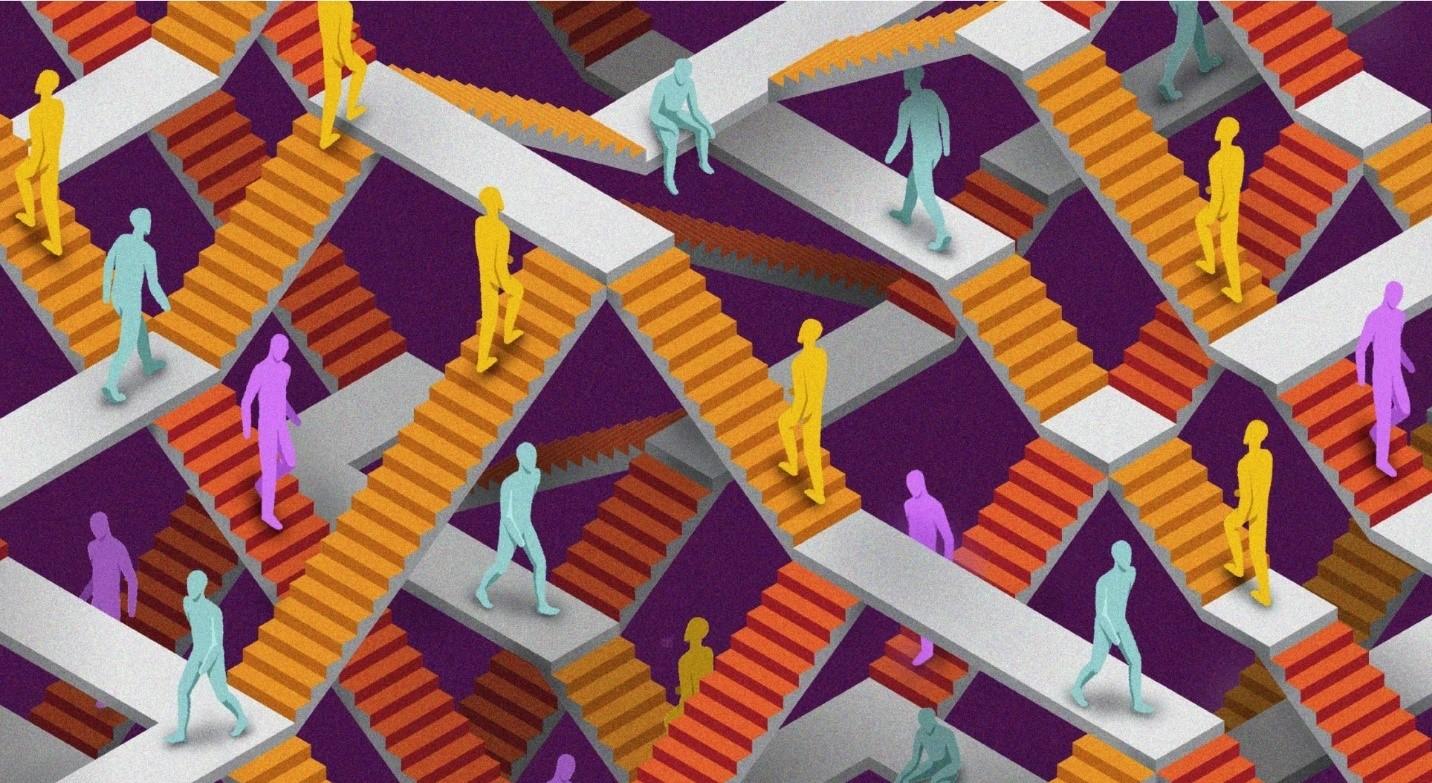
Sudden backtrack on 29 th April the Italian regulator conceded that Open Ai complied -with no specific details- but seems satisfied by the new safety measures!
It did not resolve the anxiety ,but on the opposite create a feeling that GPT is certainly evil somewhere ?Australia, South Korea and Japan are working on with more calm on AI related legislation.
Weanticipatethat2023willbeapivotalyearforstabilizingglobal “angst”overthis newadditiontoourdigitalenvironmentthatisoperatingtoowell,ortoobadly dependingonwhichsideofthefenceyouplaceyourself!