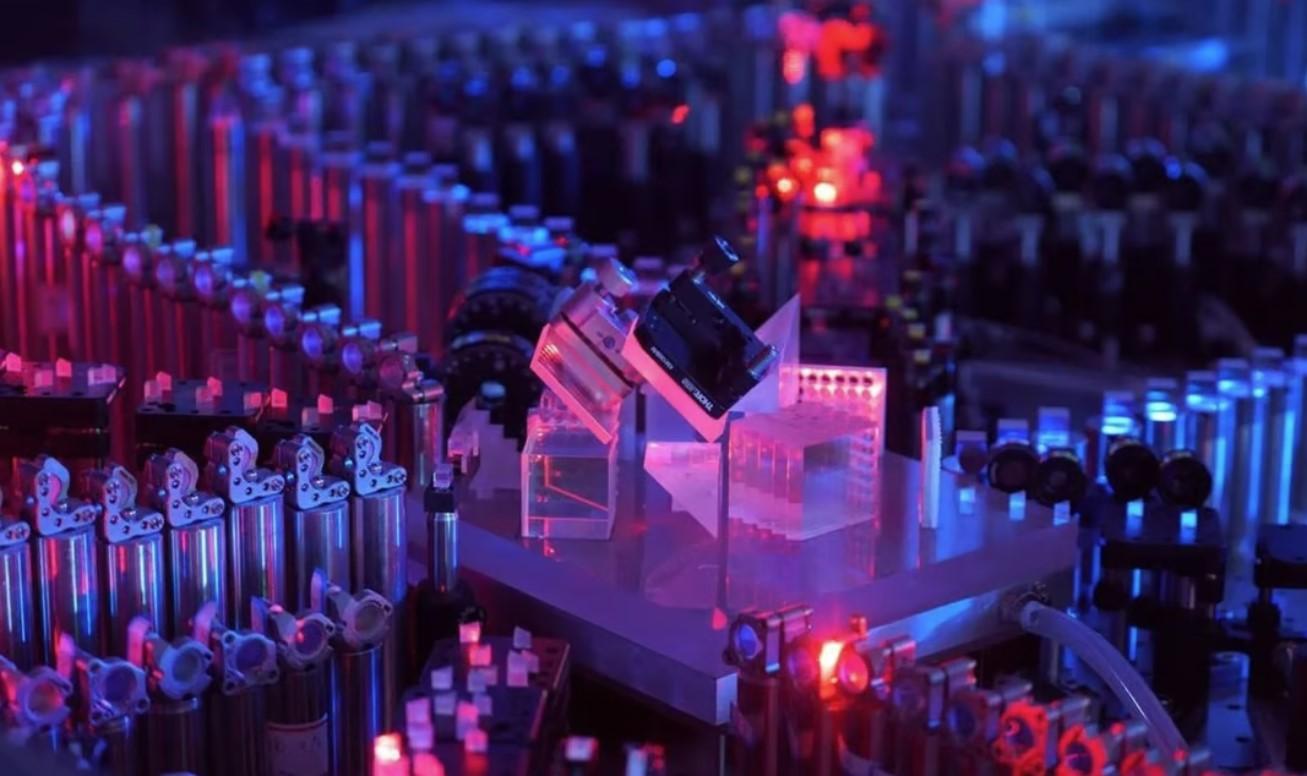
1 minute read
PHOTONIC QUANTUM SAVING ENERGY FOR AI TRAINING
A speedier and more energy-efficient way to deploy AI is through processors that run on photonics light rather than electricity.
As AI models grow larger, there is increasing concern about how much energy they need due to rising expenses and potential environmental effects. With photonic processors emerging as a top contender, this is igniting interest in novel strategies that can lower AI's energy costs.
These chips build computational circuits by using optical elements including waveguides, filters, and light detectors in place of the electrons found in conventional processors. Because they perform matrix multiplications a crucial calculation at the core of all deep-learning models very effectively, they are very promising for running AI.
They have had trouble implementing backpropagation, a critical process required to train neural networks, up until now.
In backpropagation, training examples are repeatedly fed to a neural network, which is then asked to predict outcomes based on the data. The system gauges how inaccurate the predictions are each time, and the error signal is then transmitted backward through the network.
To enhance prediction performance, this is utilized to modify the strength of connections, or weights, between neurons. This procedure is performed numerous times until the network completes the task that has been assigned to it.
Sunil Pail of PSI Quantum made a breakthrough in photonic quantum computing by using a design known as a "photonic mesh," which includes a variety of programmable optical components that regulate how light signals are spread across the chip.
The chip can do matrix multiplications and, as a result, create a photonic neural network by forcing light beams to mix and interfere with one another.
It was demonstrated by the researchers that a straightforward neural network could be trained to name points on a graph based on their location with up to 98 percent accuracy, which is equivalent to methods used in the past.
But they acknowledge that in order to get decent performance, they must utilize rather high optical power, which raises the possibility of a compromise between precision and energy utilization.
The development of practical machine-learning algorithms using photonic chips is advanced significantly by this research.
This paves the path for entirely photonic computing on-chip for applications in AI when combined with effective on-chip nonlinear operations that are currently being developed.
As someone who has long been interested in quantum computing, I frequently wonder when AI and quantum computing will converge.
ItappearsthatmypredictionthatquantumcomputingwillboostAIcomputing capacitywasalittleoffbaseandnotthemostimpressiveangle.
Thestunningsurpriseisthatthequantumphotonicanglewillbeabigenergysaver. Definitelyaneye-opener!