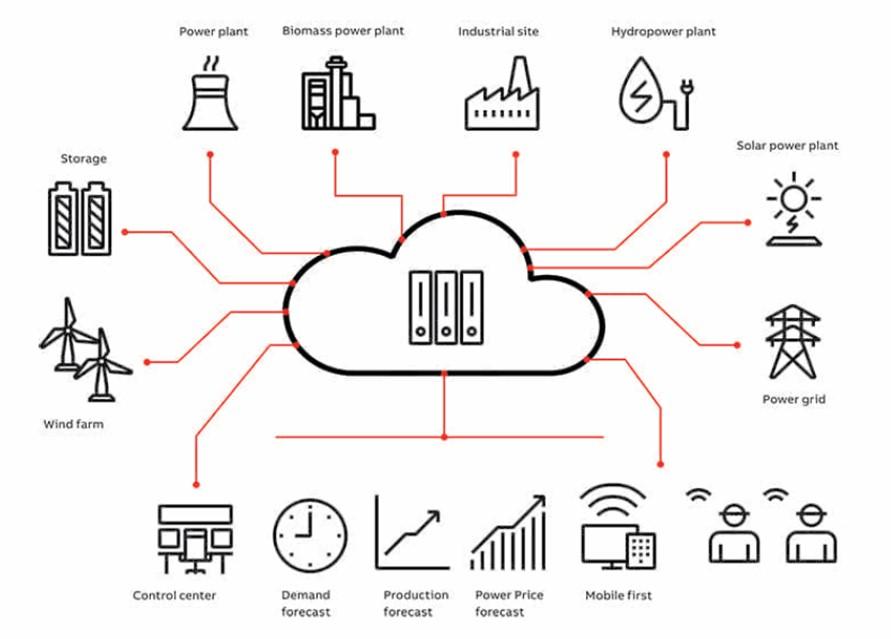
12 minute read
DECENTRALISED AI
Large data collections are a major problem for centralized AI systems, which leads to several other problems. Determining whether data and intelligence become more accessible to the general public or continue to be under the hands of a select few large corporations is one of the key issues of AI in the coming decade.
AI that is centralized favors certain individuals. It grants access to individuals who have access to vast amounts of data and cutting-edge technology, giving them control over smart technology. Due to this, there is a digital divide and certain people are left behind. AI systems that are decentralized or built on blockchain technology can be used to overcome this problem.
Decentralized AI (DAI) initiatives that use blockchain technology profit from the shared ledger's transparency and traceability. This is so that anyone may openly verify open blockchain networks. Startups now have more equitable access to the market because to DAI marketplaces like Singularity Net that allow smaller businesses to provide their AI apps as a service.
Decentralization can also result in more innovation. In a centralized system, only one organization is in charge of system development and decision-making. But under a decentralized system, numerous different organizations can work together to develop and enhance the AI system, opening a larger range of viewpoints and stimulating greater creativity.
Compared to a closed AI system, blockchain-based AI technology is more open. Blockchains encrypt data to restrict access to only authorized users. This stops anyone who is not allowed from seeing anything. As a result, it is also simpler to identify and stop assaults because several nodes can immediately identify and fix any anomalies.
DAI has many wonderful advantages, but there are also a number of difficulties that must be overcome.
Ensuring data on decentralized networks is accurate and reliable: DAI algorithms require access to a large amount of data to make precise predictions and choices. However, in order to protect sensitive information, this data must be kept secret. Thus, decentralized, and encrypted data storage are features of DAI systems. By addressing the difficulty of protecting sensitive information, this contributes to the privacy and security of the data.
Making it available to everyone, DAI needs to be simple to use for it to be adopted by the public. DAI initiatives must have intuitive designs, user-friendly interfaces, and clear explanations.
This will facilitate widespread adoption and application of blockchain-based AI by making DAI easy to understand and utilize for everyone.
The AI industry for marketing was valued at $15.84 billion USD in 2021. By 2028, the value is anticipated to surpass $107.5 billion. Notably, federated and decentralized learning methodologies could rank among the most significant fields of applied AI.
AI and blockchain technology integration is still in its infancy. The potential for unique data uses from combining the two technologies to develop DAI is huge. The trend toward DAI seems more feasible than ever thanks to the interaction of economic and technological variables.
A prime example: a virtual power plant with energy grid management
A virtual power plant (VPP) is a collection of distributed energy resources (DERs) including solar cells, wind turbines, and battery storage devices that are linked together and work together to simulate a single power plant.
DERs can also be referred to as decentralized energy systems (DES). By enabling local, on-site generation and storage, DES can help to improve energy resilience and lower energy costs.
They are situated nearer the site of energy consumption and are frequently owned and operated by individual consumers or communities rather than centralized energy providers,.
By real-time balancing the supply and demand of electricity, VPPs can aid in enhancing the efficiency and dependability of energy networks.
To manage DER–DESs more effectively and increase grid stability, VPPs are utilizing AI. AI algorithms are used to forecast energy demand and supply and modify the functioning of DERs accordingly.
By doing this, energy waste is lessened, and it is made sure that it is distributed safely and effectively.
The conventional power grid, which uses energy sources based on coal, oil, gas, nuclear, hydropower, and other fossil fuels, are coupled with VPPs to complement the efficiency and dependability of the current energy infrastructure.
By balancing the fluctuation of these sources with the more reliable output of conventional power plants, VPPs support the grid integration of renewable energy sources.
For instance, a VPP can use energy storage devices or other DER–DESs to balance the supply and demand of electricity in the grid when a wind or solar farm's output varies due to changes in the weather.
This can assist in ensuring that the grid maintains its dependability and stability as more renewable energy sources are added to it.
VPPs have the potential to balance out the fluctuation of renewable energy sources as well as improve the efficiency of the utilization of conventional power plants.
The use of AI in VPPs also has the important advantage of predicting and minimizing possible grid issues.
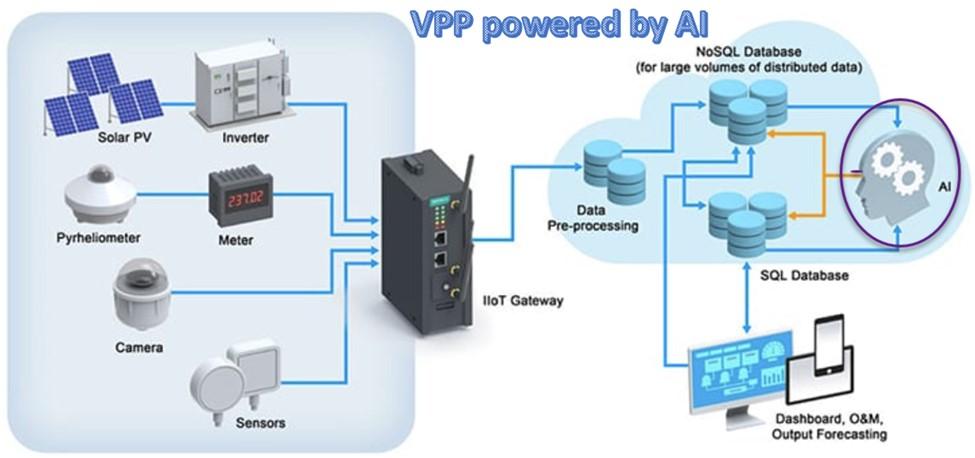
For instance, AI systems can spot DER irregularities or failures and notify operators so they can take corrective action before a bigger problem arises.
The overall stability and dependability of the electricity grid may be enhanced as a result. Overall,theuseofAIinVPPsisassistinginthetransformationoftheenergysector byenhancingtheeffectiveness,dependability,andsustainabilityofenergynetworks.
Wecananticipateseeingevenmorecutting-edgeusesofAIinVPPsandother energy-relatedindustriesfromnewtechcompaniesinthatsector,asAItechnology continuestoprogressinthesefundamentaldirections.
NOT… GONE WITH THE WIND!
The system analyzes the movement of water vapor in the atmosphere using satellite-based sensors. These sensors are used to measure the amount of water vapor present at various elevations and to identify its existence in the atmosphere.
The sensors can identify patterns of water vapor produced by the wind as it moves across the Earth's surface. The wind's direction and speed at different elevations can be calculated by analyzing these patterns.
The technique uses a mathematical model that accounts for the atmospheric conditions, such as temperature and humidity, and the movement of the water vapor over time in order to derive wind information from the water vapor data. The model employs a method known as "motion tracking" to trace the movement of the water vapor patterns over time and determine the wind's speed and direction.
Even in locations where conventional approaches are not practicable or possible, such as over oceans or difficult terrain, the algorithm is able to measure wind speeds and directions with a high degree of precision.
Numerous applications, such as weather forecasting, aviation safety, and renewable energy, can make use of the data produced by the algorithm.
Overall, the method that measures wind using water vapor is a sophisticated and intricate procedure that depends on cutting-edge mathematical models and satellite-based sensors to precisely assess wind patterns.
It is conceivable that this algorithm and comparable techniques will become increasingly more precise and beneficial in the years to come as technology advances.
Already in that niche are three companies—Radiance (USA), Valsala (Finland) and Earth Networks (USA)—that offer a range of solutions for measuring weather and environmental conditions including satellite-based sensors that use water vapor data to measure wind speed patterns.
Goodnewsforprojectsinvolvingonshoreandoffshorewindfarms.
Nomoreguessworkorholdinga “fingerinthebreeze.” Nomorefancifulfiguresinthebusinessplan.
AIprovidesengineersastraightforwardrealitycheck!
REAL-TIME INDUSTRY MANUFACTURING
At every step of the manufacturing process, manufacturers of all sizes gather data based on computer vision.
The initiative to advance is led by vision sensing. Pressure, heat, location, and other sensors, however, provide data streams that are digitalized and stored in enormous amounts.
Manual analysis is thus no longer practical for single lines, much less for whole factories or networks of companies.
The manufacturing industry is evolving steadily, and AI for the production line will have a significant impact on procedures, operations, and efficiency.
The extent of the change is undeniable. According to Precedence Research, the worldwide internet of things (IoT) manufacturing industry will be worth $220 billion in 2023.
It is anticipated to expand at a CAGR of 24.9% from 2023 to 2030, reaching over USD $1.5 trillion.
AI is a game-changer in manufacturing and associated supply chain systems. By 2026, more than 75% of providers of commercial supply chain management applications will include embedded advanced analytics (AA), AI, and/or data science.
Real-time significance
Making well-informed decisions has always been essential in the manufacturing industry to ensure quality, managing deadlines, and avoiding unanticipated outages, flaws, or safety concerns.
A few years ago, when factories and associated supply chain systems started using the IoT to convert analog to digital operations, approaches underwent a substantial change. It was an industry-wide initiative to assist the prompt action and response necessary to locate and address issues before they worsened.
Industry 4.0, also known as the industrial internet of things or IIoT, is a new era marked by the usage of AI-based solutions in manufacturing, supply chains, and logistics.
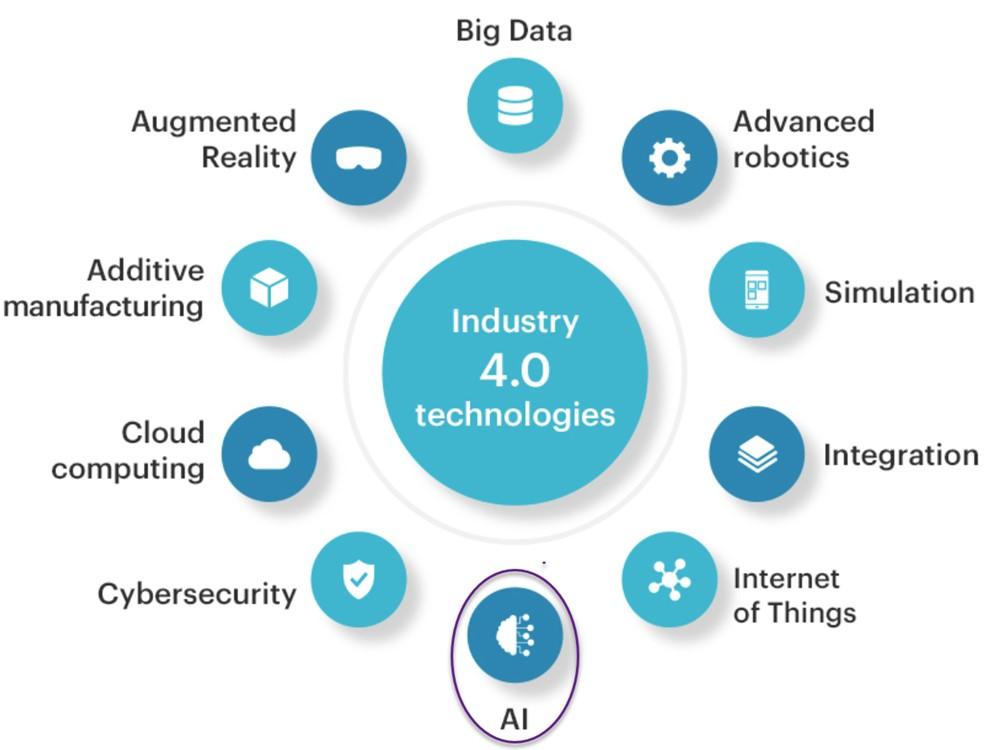
The entire supply chain must be maintained without any manual involvement. Additionally, AI-powered intelligent factories can operate more effectively, experience less downtime, and improve the overall customer experience.
For instance, AI-based technologies like intelligent document processing (IDP) are gaining traction and helping manufacturers reduce the amount of time spent processing documents by instantly converting unstructured and semi-structured data into usable data.
The traditional paperwork bottleneck that industrial organizations encounter on a regular basis is also completely eliminated, thereby revolutionizing the data collecting process. To provide their clients with differentiated mission-critical applications, proactive field service delivery, and more individualized customer outcomes, AI and data analytics are essential, and Rolls-Royce is one illustration.
In order to reduce downtime and emissions from Rolls-Royce aviation engines used by airlines all over the world, AI evaluates hundreds of data points each second. With the help of meta data, manufacturers now run their own in-house tech-enabled services at scale.
In the past, it was possible to foresee when a defect would be produced by a machine, but this prediction typically occurred too late in the manufacturing process.
When the first signs of flaws appeared, the damage was already severe enough to call for the shutdown of an expensive piece of equipment. Poor quality could cost as much as 20% of revenues.
In the manufacturing process, prompt detection of nonconforming materials can significantly lower waste, raise product quality, and enhance product traceability.
AI algorithms can find even the smallest production flaws, including misaligned parts or damaged components, by using computer vision.
This will aid producers in delivering goods of consistently excellent quality, lowering the likelihood of pricey product recalls, and enhancing brand recognition.
Real-time data and AI in manufacturing also have the potential to enhance supply chain management, including procedures that formerly used paper.
Manufacturers can estimate demand, manage delivery, and check inventory levels with real -time data, which enables them to make more informed decisions about when and how much to produce.
As a result, there is less chance of stockouts and excess production, which lowers costs and boosts customer satisfaction.AI and real-time data are accelerating output in manufacturing by detecting, contextualizing, and processing the increasing volume of invoices and documents, predicting failures, and planning maintenance.
AI algorithms will find even the smallest production flaws, including misaligned parts or damaged components, by using computer vision.
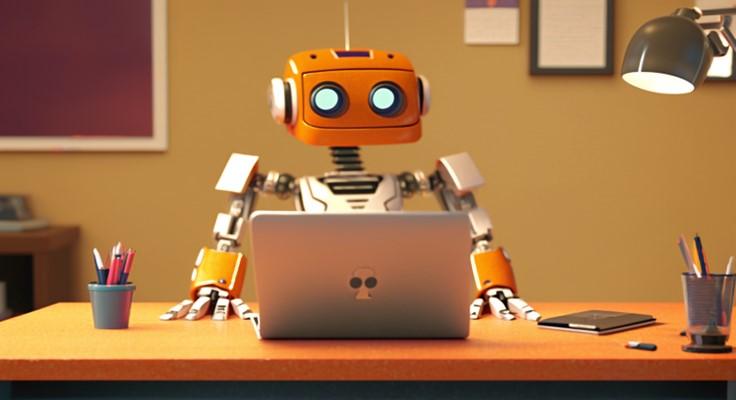
Workplace safety
The application of AI and real-time data in manufacturing has also improved workplace safety. AI algorithms can identify potentially dangerous circumstances, such as equipment failures or human error, and can notify staff to take the necessary action.
These algorithms are fed by visual sensor systems. The necessity for human workers to do physically demanding activities has also decreased because of the use of AI-powered robots, lowering the danger of harm.
AI maintains workplace safety by quickly detecting data irregularities. Manufacturers are able to consistently monitor and troubleshoot live, resolving any production issues before a disruption or hazard occurs, thanks to real-time data and AI.
Real-timedataandAIwillsoonreplacethenotionthattheyare"nicetohaves"inthe manufacturingsectorandbecomethenorm.
Real-timevisibilitymakesshifts,days,andweeksmoreproductive.Managersof warehouseswon'tbeginwithadeficitanymore.
TherapiduptakeofDL-basedcomputervisionmodelsforautomatedfaultdetection onproductionlineswillmarkanewnormalinthemanufacturingsector.
Tsunami And Earthquake Early Warning
An early warning system for undersea earthquakes and tsunamis has been researched utilizing AI. By alerting individuals ahead of time to impending natural disasters and allowing them to leave before the tsunami or earthquake, such technology will save more lives compared to the present early warning system.
Using sensors positioned on the ocean floor, the system monitors changes in water pressure that may be caused by an earthquake or tsunami. An AI system then receives the data from these sensors and instantly evaluates it to look for patterns that point to an impending earthquake or tsunami.
To forecast the possibility and severity of a tsunami, the AI system can also include additional elements, such as the depth and position of the earthquake.
To provide residents of coastal areas enough time to flee to higher ground, this information can subsequently be utilized to send alarms to them.
Cardiff University (Wales-UK) made the breakthrough by developing their own early warning system for undersea earthquakes and tsunamis powered by AI. Using technology like underwater microphones and AI, the team has been able to track the movement of tectonic plates in real-time.
Experts have now identified 200 earthquakes in the Pacific and Indian oceans using sound recordings.
As a senior lecturer in Applied Mathematics at Cardiff University and a study co-author, Dr. Usama Kadri said: "Our study illustrates how to gather quick and accurate information about the size and extent of tsunamis by monitoring acoustic-gravity waves.
They move through the water considerably more quickly than tsunami waves, giving places more time to evacuate before landing. Sound waves that travel across the deep ocean at the speed of sound are called acoustic-gravity waves.
They can be caused by landslides, explosions, and underwater earthquakes, all of which can result in tsunamis.”
The new method uses recordings from an underwater microphone called a hydrophone along with an AI computational model to triangulate the source of the tectonic event, even thousands of kilometers offshore.
Tsunami alerts are triggered by waves hitting seismic sensors and sea buoys. A few extra minutes mean the difference between life and death, with little time for evacuation.
However, they do not always correctly foresee the threat that the ensuing tsunamis will pose. There are 11 hydrophones currently deployed in the oceans around the world by Dr. Kadri and his collaborator, Dr. Bernabe Gomez Perez. They stated that that they will be able to watch the entire planet with 24.
To put it in real terms, Kadri looked back: "Theoretically, we could have given [the inhabitants] 65 minutes to flee in the 2004 tsunami in Sri Lanka [Indian Ocean]. That represents the chance to save almost every life.”
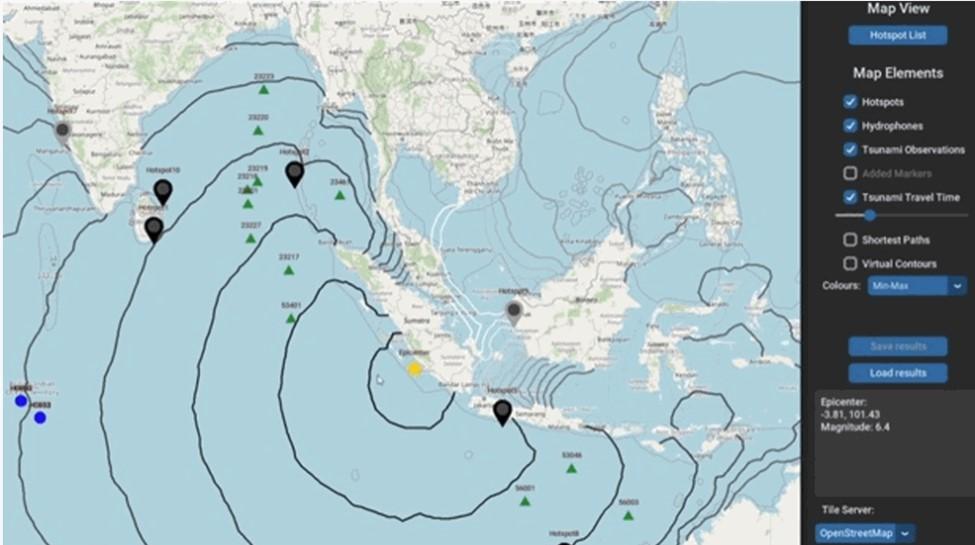
Due to the proximity of the buoys to the shore and the lack of real-time calculations and equipment to measure the tsunami, the existing system frequently arrives too late.
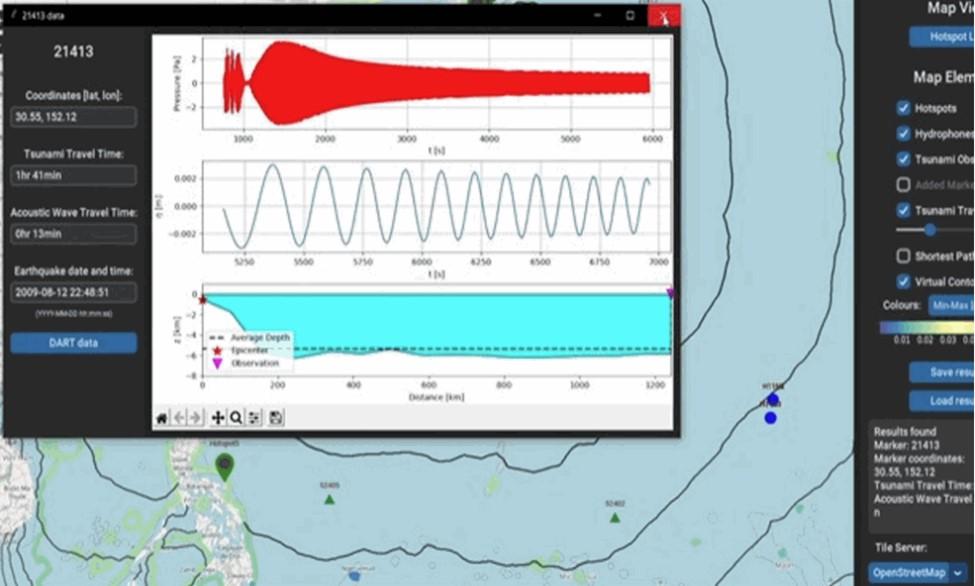
AI calculations take around 17 seconds after the wave hits the hydrophones which is extremely fast compared to today’s “not early enough warning system.”It is clarified that their system is intended to operate in concert with current systems, with each serving as a check on the other.
Prior to analyzing the earthquake's physical characteristics, such as its length and width, uplift speed, and duration, the new AI program can categorize the earthquake's type and magnitude. This will be used to determine the tsunami's exact size.
Ofcourse,cryingwolfwithnoproofunderminestrustinthesystemamongthe public—thesameproblemthatthecurrentsystemfaces.Peoplewon'tleavethen whenit'sreal.ThatistheeternalriskfacingteamsuptonowwithoutAIextra support.
Hopefully,fewerseconds-longrushtopack:peoplewillhavesomemoreminutes nexttime!
SCARING THE FRENCH, AI COMING TO THE WINE INDUSTRY!
The management of vineyards, winemaking, and marketing are three areas where AI is reshaping the wine industry.
Today, AI algorithms are applied to vineyard management to assess data on soil quality, weather patterns, and grape quality and provide advice on when to harvest, how much water to use, and other crucial aspects, all to aid farms in increasing grape quality and optimizing yields.
There are various new ways where AI is applied in winemaking, including:
Quality control: AI algorithms are used to examine information from fermentation tanks, including temperature, sugar levels, and other variables, to find any anomalies or fluctuations that might affect the wine's quality. To produce the highest-quality wine, this can enable winemakers in making real-time modifications to the process.
Predictive analytics: it is possible to forecast how the wine will turn out by examining data from prior vintages, weather patterns, soil conditions, and other factors. To make sure the wine has the right taste and fragrance profiles, winemakers can use this information to assist them decide when to harvest, how long to ferment, and other crucial criteria.
New flavor profiling generates or enhances current ones by analyzing data on the chemical elements that contribute to wine's flavor and aroma.
Barrel management to analyze data on the effects of various types of barrels on the flavor and aroma of wine and provide recommendations for the best barrels to utilize for various types of wine. Winemakers can use this to experiment with new flavors and wine styles while producing more reliable, high-quality wines.
Wine making process monitoring to spot check any potential quality problems or variations from the expected flavor and aroma characteristics. To ensure that the wine reaches their requirements for quality, this can assist winemakers identify any issues early on and take the necessary remedial action.
Overall, using AI to winemaking has the ability to raise the caliber and consistency of wines while assisting vintners in experimenting with new tastes and styles.
It's important to remember, though, that AI is merely a tool that can support winemakers in making better educated and data-driven decisions rather than taking the place of their skill and creativity.
AI will also be used in marketing to assess data on customer preferences and trends and provide advice on how to target particular demographics with various wine varieties. This can assist wineries in understanding their clients' preferences and developing more successful marketing efforts.
Overall, the application of AI to the wine industry is a fascinating new frontier with the potential to raise wine quality and boost the productivity and profitability of wineries and vineyards.
Don’ tcry “Argentina”…wines… GoheadsontouseAItocompetewithefficiency, ButkeepyourrespectfortheFrench “sommelier” WhosescentskillsarestillunmatchedbyAI… Hopefullyuptonow!
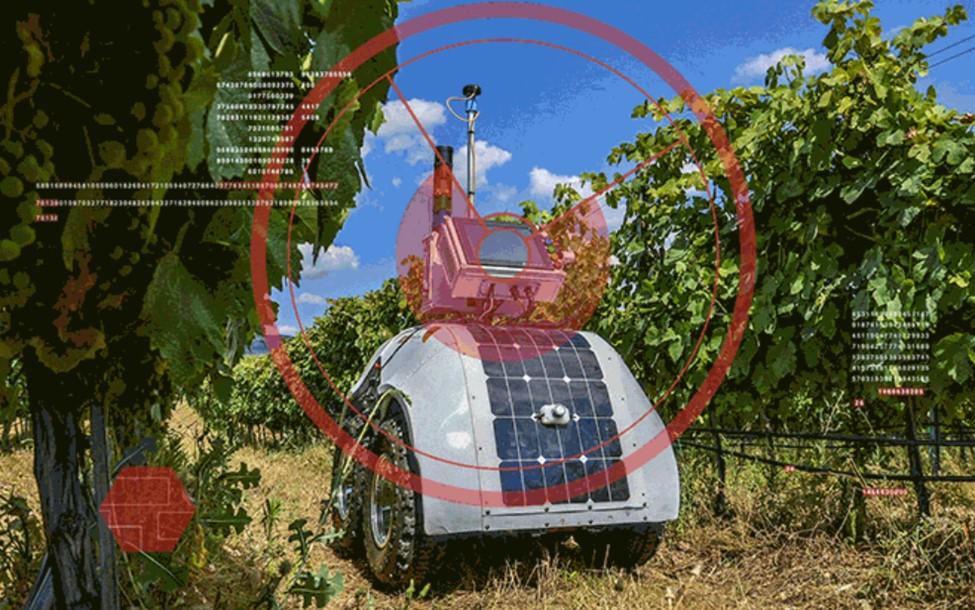
“To catch the reader's attention, place an interesting sentence or quote from the story here.”