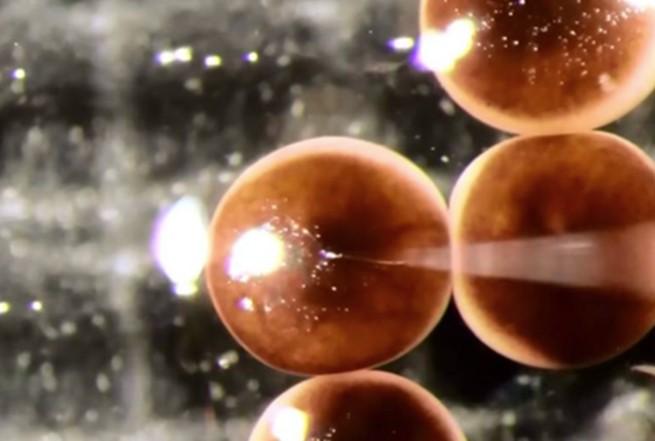
5 minute read
Part 3—THE SCIENCE SPECTRUM
Speeding Cancer Research With Xenobots
In the field of cancer research, AI is becoming more and more significant, especially for diagnosis, prognosis, and individualized therapy. To find trends and generate predictions about a patient's cancer diagnosis, development, and response to treatment, ML algorithms can be trained on enormous databases of clinical and genetic data.
In order to accurately identify breast cancer and lung cancer, for instance, researchers have employed DL algorithms to evaluate medical imagery such as mammograms and CT scans. Additionally, by analyzing genomic data, AI can find mutations and patterns of gene expression that may be connected to certain cancer types.AI can be utilized to create tailored treatment regimens for cancer patients in addition to diagnosis and prognosis.
AI algorithms can determine the most effective treatments for a patient's specific form of cancer and forecast how the cancer will likely respond to various therapies by examining the patient's genetic and clinical data.Oncologists may be able to make better treatment choices and patient outcomes as a result.
AI is also beginning to be used in the search for new cancer treatments. Using chemical characteristics and interactions with biological systems, for instance, AI algorithms can be used to forecast the efficacy and toxicity of possible medication candidates.
This may speed up the process of identifying prospective drug candidates and may shorten the time and expense involved in bringing new cancer treatments to market. The TRACERx project, a cancer research initiative in the UK, uses AI to better understand how tumors change over time and become resistant to therapy. In order to find patterns in the data and forecast how the tumors would develop, the study entails examining genomic data from individuals with lung, esophagus, pancreatic, and kidney cancer.
For instance, scientists are using AI to examine how genetic changes in tumors affect cancer cells' activity and to determine which genetic changes are most likely to result in medication resistance.
The initiative also uses AI to examine medical pictures like CT scans and MRI scans in order to find patterns that could be connected to certain cancer kinds.
Thanks to AI, after two years of intensive research on monitoring lung cancer growth, the findings—which were released on April 13, 2023—showed that malignancies alter and evolve over time; they are not a permanent and unchanging entity.They can develop into more aggressive forms that are better able to spread throughout the body and elude the immune system.
A tumor begins as a single damaged cell and develops into a mass of millions of cells that have each undergone a slightly different type of mutation.The results of TracerX's study, which followed the diversity and its alterations over time in lung cancer patients, are said to be generalizable to other cancer types.
Given the nearly infinite possibilities for how a tumor can develop and the enormous number of cells in a late-stage tumor, which may be several hundred billion cells, the research team came to the conclusion that it would be difficult to cure all patients with late-stage disease.
The science publication Nature published the results: The evolution of non-small cell lung cancer metastases in TRACERx | NatureProf Swanton concluded: “I don't think we're going to be able to come up with universal cures. If we want to make the biggest impact we need to focus on prevention, early detection and early detection of relapse.”
Obviously a politically correct conclusion. He slightly forgot that the development of AI in the field of cancer research will enable early cancer diagnosis from patient history, which is a thousand times faster and more accurate than practicing physicians.
It is frustrating to have studied for so long and be unable to respond as precisely as AI. He neglected to mention that we require human empathy to communicate to the patient what AI concludes
To assist, guess what is next? "Xenobots."
Xenobots are a new type of biological machine created by fusing AI with living cells. They were developed by a group of researchers from Tufts University and the University of Vermont in 2020, and they are manufactured from frog embryo cells that are joined together according to predetermined forms by a computer program. Video https://youtu.be/ M18nPjLZrMA
The aim behind Xenobots is to build biological machines that are capable of carrying out particular duties, including delivering medications or clearing up environmental toxins. Xenobots are live organisms that can self-heal after being wounded since they are formed of living cells.
It is important to keep in mind that Xenobots’ use in medicine is still in its infancy and that numerous technological and moral issues must be resolved before it can be applied to people. Making certain, for instance, that the Xenobots are reliable and safe, and that they do not hurt the patient's own cells or any other living things around them.
Overall, the development of Xenobots is a fascinating new frontier in synthetic biology that has the potential to change numerous fields of biotechnology and medicine. To fully comprehend their powers and limitations, as well as to guarantee their ethical and safe use, much more research is necessary.
Targeted medicine distribution is one possible use for Xenobots. The Xenobots could be used to transport medications right to the site of the sickness by being programmed to recognize and target particular types of cells, such as cancer cells. This might lessen the negative effects of medications and increase their potency.
DearReaders,thisisnotasciencefictionstory.
TheFuturologyChronicleeditioninDecemberon “Biohacking”
Willhavemoreinformationonthisfascinatingsubject.
Proteins In 3d A Huge Step Forward
DeepMind (Google owned) created an AI system called Alpha Fold (AF21) that can forecast the 3D shapes of proteins. Proteins are intricate molecules that are essential to numerous biological processes, and their structure and function are intimately intertwined.
Therefore, understanding protein structure including enzymes, receptors, antibodies, and many more is crucial for comprehending many biological concepts, such as disease mechanisms and therapeutic development.
Based on a protein's amino acid sequence, Alpha Fold uses DL algorithms to predict the 3D structure of the protein. The system can predict outcomes quickly and accurately since it has been trained on a vast dataset of known protein structures.
The AF21 DL algorithm for protein structure prediction uses multiple sequence alignments (MSA) and the Evoformer module to extract information about interrelationships between protein sequences and template structures.
It then iterates to improve the local structural details analogous to traditional refinement. The key to AF21's success is the fact that the library of single domain protein structures is essentially complete.
It used uncommon DESTI-NI convolutional neural network (CNN) and SAdLSA algorithms to demonstrate that DL can learn long-range correlations among residues. AF2 has also been used to predict the structures of the human protein exome.
Combining AF2 with a more traditional approach was a winning strategy to increase exome structure coverage. AF21 is now a knowledge-based scoring function that can predict alternative conformations. It can also predict the structural effects of missense mutations and splice variants, as well as residues in “Chameleon” sequences which change the global fold.
However, much work still needs to be done to establish the strengths and limitations of AF2. To improve the ability to predict drug side effects, efficacy, and the role of missense variations, accurate characterizing the interplay of many molecular players is needed.
Researchers now better understand how proteins operate and can create medications and other therapeutic agents that target certain proteins with greater accuracy and efficacy by properly predicting the 3D structures of proteins.
Proteins involved in conditions like Alzheimer's and Parkinson’s, as well as protein targets for medications like ion channels and G protein-coupled receptors (GPCRs), are some of the proteins whose structures Alpha Fold has been able to predict.
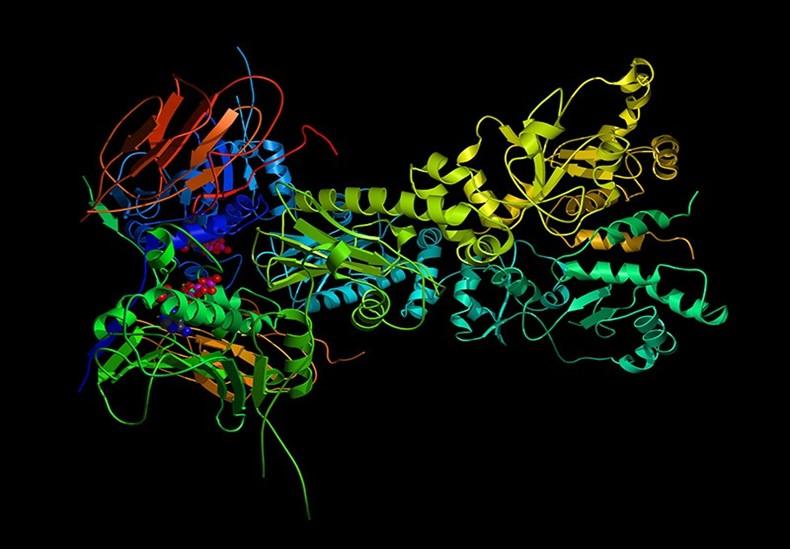
Researchers are going to be able to identify and develop medications more quickly when they can accurately predict the structures of these proteins since they will be able to target them more precisely and effectively.
Overall, Alpha Fold has the potential to speed up research in a wide range of fields, from fundamental science to drug discovery and beyond, due to its capacity to reliably predict the structures of numerous different types of proteins.
AlarmistAIdetractorscannotignorethiskindofscientificadvancement. Ifpeoplerejectthecurrentfourthindustrialrevolution,theyshallreturntolifeasit wasbeforethefirst!
Therearestillafewroomsavailableinthe"Supai"Arizonacommunity(200people) neartheGrandCanyon,whichisprimarilyaccessibleviaamuleride.
I’ msureyou’dbewelcome!