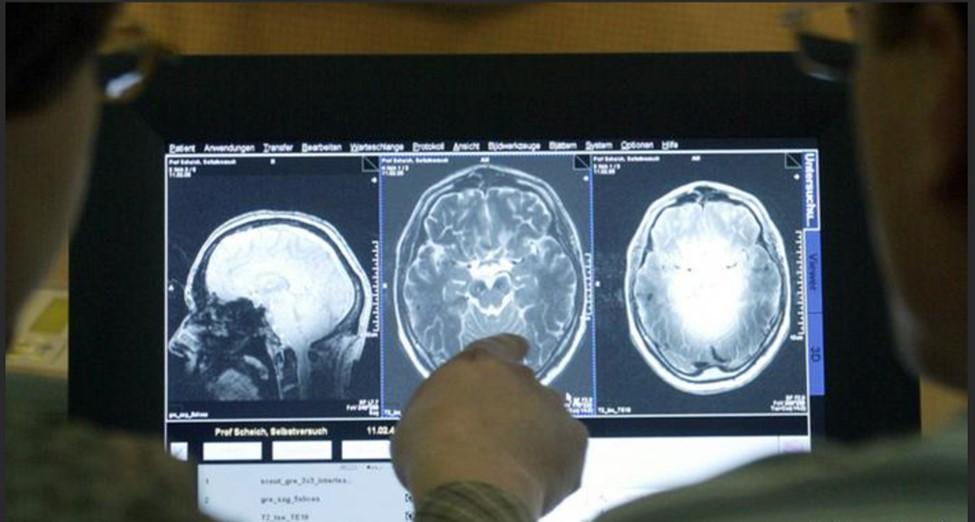
7 minute read
INSOMNIA DECODING
A new area of study called "machine learning decoding of insomnia" seeks to better understand and identify insomnia by using ML algorithms.
A person's health and welfare may be significantly harmed by insomnia, a common sleep disorder marked by difficulties sleeping or staying asleep.
In order to use ML to decode insomnia, it is often necessary to gather and analyze a sizable quantity of data from a variety of sources, including wearable technology, sleep studies, and patient-reported results.
Then, using this data, ML algorithms are trained to spot trends and make predictions about insomnia-related events.
Creating more precise and effective diagnostic tools for insomnia is one use. ML algorithms can find patterns and signals that are suggestive of the condition by examining data from a variety of sources. They then use this knowledge to create more precise diagnostic tools. There are a number of wearable technologies on the market that track sleep patterns and transmit live data to AI applications.
One classy example of a wearable device that analyzes sleep, activity, and other health parameters is the Oura Ring.
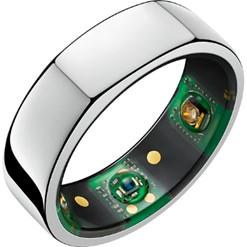
The Oura Ring collects data on body temperature, heart rate variability, and sleep stages using cutting-edge sensors, then uses that information to generate individualized insights and suggestions.
WhohastheaudacitytoarguethatAIisnotaforceforgood?Afterreadingthis example,whowoulddare?Who,who…
MIND READING? NOT YET, BUT SOON.
AI reading brain scans involves using ML algorithms to examine neuroimaging data, such as functional magnetic resonance imaging (fMRI) or positron emission tomography (PET) scans, to learn more about how the brain works and to spot patterns of neural activity linked to conditions.
These algorithms frequently combine supervised and unsupervised learning strategies. A labeled dataset is used for supervised learning, where each scan is tagged with details about the patient's symptoms or condition.
To predict the label for fresh, unlabeled scans, the algorithm learns to identify patterns in the data that are linked to the labels.
On the other hand, unsupervised learning entails training the algorithm on an unlabeled dataset with the program's job being to find underlying patterns or structures in the data.
This can be helpful, for instance, in classifying patient groupings based on similar neuroimaging characteristics.
Dealing with the vast volumes of data produced by neuroimaging techniques is one difficulty in using AI to read brain scans.
These datasets might have millions of data points each scan, making it difficult to handle and interpret the data computationally.
To overcome this, researchers frequently employ methods like dimensionality reduction or feature selection to draw out the most pertinent information from the data and lighten the computing load.
Making sure the algorithms are clear and easy to grasp so that researchers and physicians can comprehend how the algorithm generates its predictions is another crucial factor to consider: DL algorithms, which are frequently referred to as "black boxes" because of their intricate designs, can find this to be especially difficult.
Overall, AI interpreting brain scans has the potential to transform our understanding of brain functions and advance the detection and treatment of neurological illnesses.
However, it is crucial to carefully consider the ethical implications of this technology, especially considering concerns like patient privacy and algorithmic bias. Using advanced image-generating AI and fMRI data, two scientists in Japan recently translated study participants' brain activity into images that uncannily resembled the ones they saw during the scans.
We can develop possible brain-machine interfaces using these kinds of methods, says Yu Takagi, a neurologist at Osaka University in Japan and one of the study's authors.
Future interfaces like this could assist those who are now unable to communicate, like those who may be cognizant but who do not appear to be responding.
Stable Diffusion, a so-called diffusion model from London-based startup Stability AI, was used by the researchers in the most recent study.
These models acquire new skills by introducing noise to their training images. The noise affects the visuals similarly to TV static, but in predictable ways that the model starts to pick up on. The model will eventually be able to create images just from "static."
They were able to produce these images by essentially mapping those to one another. The AI model might then figure out which minute variations in an individual's brain activity relate to certain aspects of the photographs.
When the model had mastered these patterns, the researchers fed it previously unexplored fMRI data and asked it to produce the accompanying image.
To determine how well the model worked, the researchers could finally compare the generated image to the original.
However, this does not mean that scientists have fully understood how the brain interprets the visual world.
Even though it is capable of producing results that are similar to those of the brain, the Stable Diffusion model does not necessarily process images in the same manner.
The comparison between these models and the brain is intended to provide insight into the inner workings of both complicated systems.
Totrytobesomewhatreassuringforthetimebeing,noexistingAImodelwould haveenoughdatatobegininterpretingyourbrainactivityunlessyouagreetolying stillandfocusingonhundredsofimageswithinaclanging,claustrophobicMRItube. That’sarelief.
AImodelsareonlyeffectiveattasksforwhichtheyhavebeenexpresslytaught,even withthatkindofmassivedata.
Itwon'tbepossibletodecodetheconceptsyou'rethinkingaboutusingamodelthat wasonlytrainedonhowyouviewimages.
Forthetimebeinganywaybutbraceyourselfalreadyforthe2040s!
HEALTH TRUTH IN YOUR RETINA?
Retinal scanning is a non-invasive procedure that makes use of high-resolution imaging to evaluate the retina's blood vessels and structural integrity.
A thin layer of tissue called the retina is located at the back of the eye and contains specialized cells that transform light into electrical signals that are sent to the brain. This is coupled with a web of blood arteries that supplies the retina with oxygen and nutrients for the retinal cells.
Retinal scanning is the method for taking precise pictures of the retina using specialist tools like a fundus camera or optical coherence tomography (OCT).
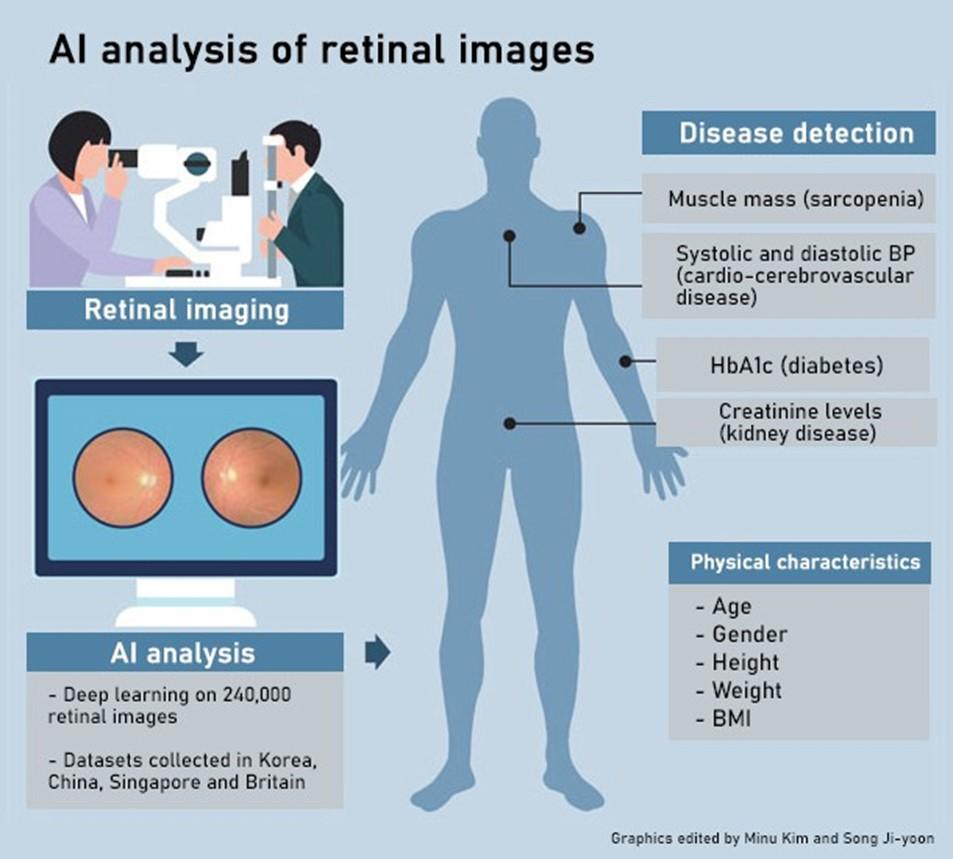
Images show changes in the thickness of the retina or anomalies in the retinal blood vessels, which are signs of several medical disorders: for instance, anomalies in the retinal blood vessels, such as thickening or narrowing, are a sign of cardiovascular disease before symptoms ever appear.
The retinal blood vessels' appearance is also a sign of high blood pressure, which can determine hypertension, while diabetic retinopathy is a condition when there has been damage to the blood vessels in the retina as a result of diabetes. Identification during the early stages of diabetic retinopathy allows early treatment and control of the problem.
According to new studies, alterations in the retina's structure, such the layer of retinal nerve fibers becoming thinner, can be a sign of Alzheimer's disease.
The “Retina Net” program, an AI-powered retinal diagnostic tool, uses a DL architecture to identify diabetic retinopathy from retinal pictures.
The system learns to recognize patterns and features in the images that are suggestive of the disease by training on a sizable dataset of retinal images labeled with various degrees of diabetic retinopathy.
Another illustration is the “Eye Art” algorithm, which analyzes retinal images to identify diabetic retinopathy and macular edema using a combination of computer vision and ML methods.
To find symptoms of the diseases, the algorithm examines several aspects of the images, such as the existence of lesions and changes in the thickness of the retina.
In parallel the “Vascu Net” algorithm uses ML to examine the retinal blood vessels and detect signs of hypertension.
Overall, retinal diagnostic tools powered by AI increase widely the precision and speed of diagnosis for a variety of medical disorders.
Globally, a number of young companies create and provide AI-powered retinal diagnostic devices. The following are a few well-known businesses in this industry:
A USA FDA-approved AI diagnosis system for diabetic retinopathy is offered by Eyenuk and IDx Technologies.
In Europe, a cloud-based AI solution for detecting diabetic retinopathy and maintaining an AI-based retinal camera is available from Optomed (Finland) and Retinalyze (Denmark).
In India, an AI-based retinal screening device called Fundu is offered by Remidio Innovative Solutions for the detection of glaucoma, macular degeneration, and diabetic retinopathy.
Topcon Healthcare Solutions and Nidek, which provide AI-powered diagnostic solutions for various retinal illnesses, are two companies in Japan.
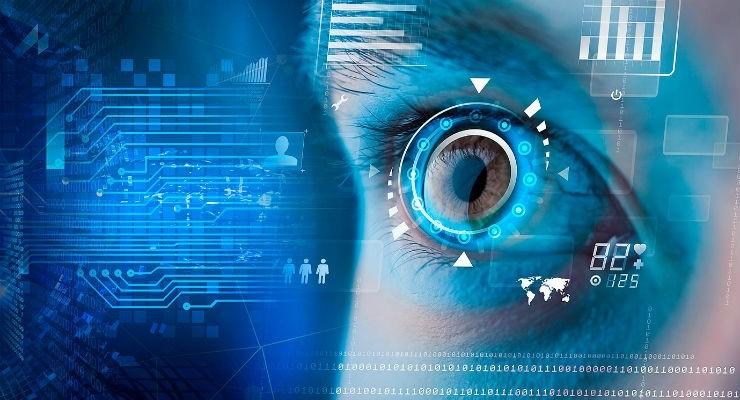
Technology pioneer Mediwhale, based in South Korea, claims that its tool has a sensitivity of 93.8%, a specificity of 96.9%, and can get results in just a few minutes.
Medical diagnosis tools of any kind for any condition are making significant strides thanks to AI algorithms.
Naturally,wemustconsiderthattheaccuracyofthesealgorithmsvariesbasedon thecaliberoftheretinalimagesandthepopulationbeingassessed whichsuggests thatadditionalstudyandvalidationarealwaysrequiredtoguaranteereliabilityand effectivenesstobeproveninclinicalsettings.
Adiscoveryenthusiasmwithprudence.
Ai Crucial In Age Tech Sector
AGE TECH, commonly referred to as "Aging Technology," is the use of technology to enhance the quality of life and wellness of older persons. It consists of a wide range of goods and solutions made to meet the special requirements and difficulties of aging populations, including wearable technology, smart home systems, and telehealth platforms.
AI is a crucial element of many AGE TECH solutions. AI may be used to evaluate vast amounts of data produced by older persons, such as health monitoring data, to spot trends and provide tailored recommendations for enhancing health and wellbeing.
It can also be used to create predictive models that can aid in locating people who run the risk of contracting specific illnesses or suffering specific health outcomes, such hospitalizations or slips and falls.
AI-enhanced AGE TECH solutions can assist older persons to preserve their independence and stay in touch with family and friends, in addition to enhancing health and wellbeing. For instance, social robots with NLP capabilities can offer companionship and support with everyday duties, while virtual assistants powered by AI can assist seniors in managing their meds and making appointments.
A fantastic example is NOBI LIFE, a Belgian business that specialized in the design and production of smart home gadgets for elderly and disabled people. Smart sensors, alarm systems, and home automation tools are among the company's solutions that can assist elderly and disabled people feel safer, more secure, and more independent.
NOBI LIFE seeks to offer cutting-edge solutions that improve the quality of life for seniors and individuals with disabilities while also bringing comfort to their loved ones and caregivers.
The organization, which has its headquarters in Brussels, Belgium, has won praise for its cutting-edge approach to aging and disability technologies.
Their main model, an AI-powered smart lamp with several functions, has received numerous design accolades for its understated elegance.
“To catch the reader's attention, place an interesting sentence or quote from the story here.”
When a fall is detected, it immediately alerts a contact person. It opens the door when assistance comes.
The infrared LEDs detect the fall in complete darkness when it occurs at night and turn on the light.
Additionally, it displays sleep data so that whatever is causing alterations in sleep patterns can be addressed.
ThisnoveldeviceisthistimeaperfectillustrationofanAIessentialapplicationfor society,asseeninthevideo:https://youtu.be/g86cmWf9J6Q
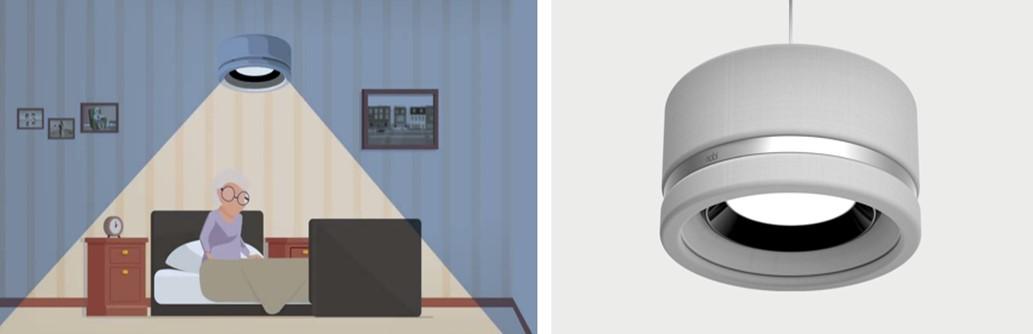