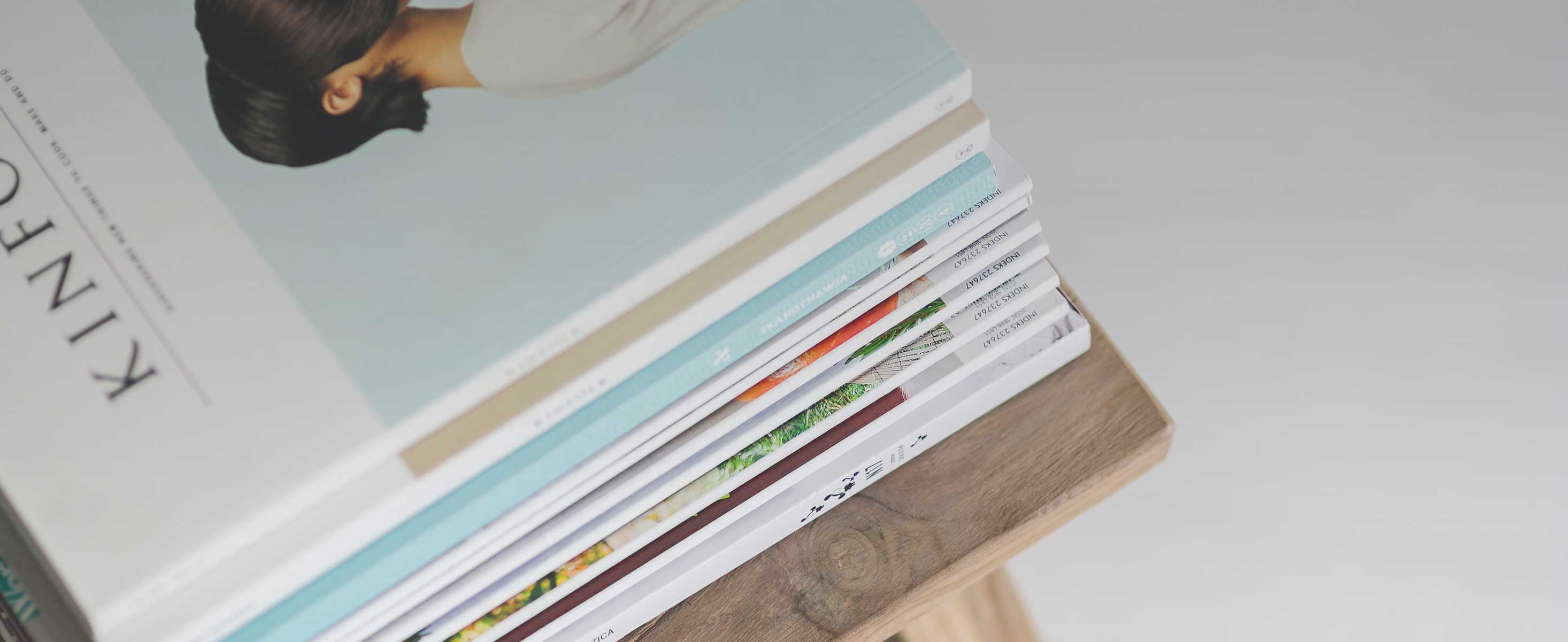
5 minute read
Role of AI Hype in the Economic Ecosystem
an Indo-centric standpoint is to be maintained, the cost of manufacture, research, development, supply as well as labor is bound to increase by some amount which may or may not be significant. The factor that is perhaps the most important is the need for the R&D of resilient infrastructure much faster, in comparison to the other sectors to inhibit & keep at the bay the influence of China as envisaged by the India, U.S., Australia & Japan. The race in perfectly replicating technologies while keeping the costs minimal is something that would cause the cost to go up significantly. Other than these assumptions, no other determinations can be accurately made.
Role of AI Hype in the Economic Ecosystem
Advertisement
Yet another factor to be borne in mind is the Hype with respect to disruptive technologies inclusive of Artificial Intelligence in general. The hype can potentially have an effect on the economy of production, R&D & Supply. Andrew Ng – pioneer in AI & ML applications, founder of Google Brain & Coursera, in a session hosted by DeepLearning.AI & Stanford HAI, said that “Those of us in machine learning are really good at doing well on a test set, but unfortunately deploying a system takes more than doing well on a test set.” Ng brough up the case in which Stanford researchers were able to develop an algorithm to diagnose pneumonia from chest X-rays, which when tested, in fact – performed better than human radiologists (Perry, 2021). It is to be understood that there are challenges in making a research paper into something useful in a clinical setting. He notably remarked that “All of AI, not just healthcare, has a proof-ofconcept-to-production-gap, the full cycle of a machine learning project is not just modeling. It is finding the right data, deploying it, monitoring it, feeding data back [into the model], showing safety – doing all things that need to be done [for a model] to be deployed. [That goes] beyond doing well on the test set,
Regularizing Artificial Intelligence Ethics in the Indo-Pacific, GLA-TR-002
which fortunately or unfortunately is what we in machine learning are great at.” (Perry, 2021) Computer scientist Frederick Hayes-Roth had predicted in 1984 that AI would soon replace experts in law, medicine, & finance among other professions. Soon, the overenthusiasm gave way to a slump knows as an “AI Winter” with disillusionment setting in & funding declining. AI back then, had not lived up to the general expectations. Roth stated that human minds are hard to replicate, because we are “very, very complicated systems that are both evolved and adapted through learning to deal well and differentially with dozens of variables at one time.” (Horgan, 2021) Algorithms that can perform a specialized task, such as playing chess, cannot be easily adapted for other purposes.
“It is an example of what is called nonrecurrent engineering,” said Ross in 1998 (Horgan, 2021).
According to reports all over the world, AI is supposedly & allegedly booming once again. Cell phones, televisions, vehicles & countless other commercial consumer products claim to utilize AI. VC investments in AI have doubled between 2017 & 2018 to a whopping $40 billion, as per WIRED. A Price Waterhouse study estimated that by 2030 AI will boost global economic output by more than $15 trillion, “more than the current output of China and India combined”. A few observers have even expressed fears to AI supposedly moving too fast. New York Times columnist Farhad Manjoo called an AI based reading & writing program –GPT-3 - “amazing, spooky, humbling and more than a little terrifying.” He expressed a concern of himself being replaced by such an application/program/mechanism someday. Neuroscientist Christof Koch suggested that humans might need computer chips implanted into the brains to keep up with intelligent machines. Elon Musk made headlines in 2018 when he warned that “superintelligent” AI represents “the single biggest existential crisis that we face.” In January of 2020 a team from Google Health claimed in Nature that their AI program had outperformed humans in diagnosis of breast cancer while in October, a group led by Benjamin Haibe-Kains – a computational genomics researcher, criticized the Google Health paper,
contending that the “lack of details of the methods and algorithm code undermines its scientific value.” (Horgan, 2021)
Haibe-Kains as well made an allegation to Technology Review that the Google Health report is “more an advertisement for cool technology” than a legitimate, reproducible scientific study. The same perhaps might be true for numerous other advances, said he. It can be assumed that AI like biomedicine & other fields –has become stuck in a replication crisis. Researchers make dramatic claims which cannot be tested, because they while in the industry do not & cannot disclose their algorithms. An interview yielded an opinion that only 15% of AI studies actually share the code (Horgan, 2021).
There are signs that AI investments are not actually paying off. Technology academic Jeffrey Funk examined 40 start-ups developing AI for health care, manufacturing, energy, finance, cybersecurity, & transportation & other industries & concluded that many of them were not “nearly as valuable to society as all the hype would suggest,” Funk went on to say in IEEE Spectrum that Advances in AI “are unlikely to be nearly as disruptive –
for companies, for workers, or for the economy as a whole –
as many observers have been arguing.” (Horgan, 2021)
Science reported that “core progress in AI has stalled in some fields,” such as information retrieval & product recommendation. A study of algorithms used to improve the performance of neural networks found that “no clear evidence of performance improvements over a 10-year period.” (Horgan, 2021) The ages' old goal of AGI still remains elusive. “We have machines that learn in very narrow way”, said Yoshua Bengio, a pioneer in the AI approach termed deep learning, complained in WIRED “They need much more data to learn a task than human examples of intelligence, and they still make stupid mistakes.” In The Gradient, AI entrepreneur & writer Gary Marcus accused AI leaders as well as the media of exaggerating the progresses made in the field. Autonomous cars, deepfake detection, diagnostic programs & chatbots were supposedly oversold. Marcus contended that “if and when the public, governments, and investment, community recognize that they have been sold an