Artificial intelligence in the telecom industry
The telecommunication industry is no longer limited to telephony and internet services, but is at the center of technological advances with an emphasis on artificial intelligence. AI has dominated this industry for more than a decade and has successfully replaced traditional and complex methods with new algorithms, making it easier to analyze and process the vast amounts of data collected from multiple consumer bases, particularly through computer vision technologies Nowadays everyone is looking to implement Ai in their business, Here is the cost to develop Artificial Intelligence
Artificial intelligence (AI) has been advancing remarkably since around 2010 and has been applied to the telecommunications market for more than a decade. In addition to the fact that communications infrastructure is essential to society, more and more applications rely on well-functioning, reliable, and always-available communications services.
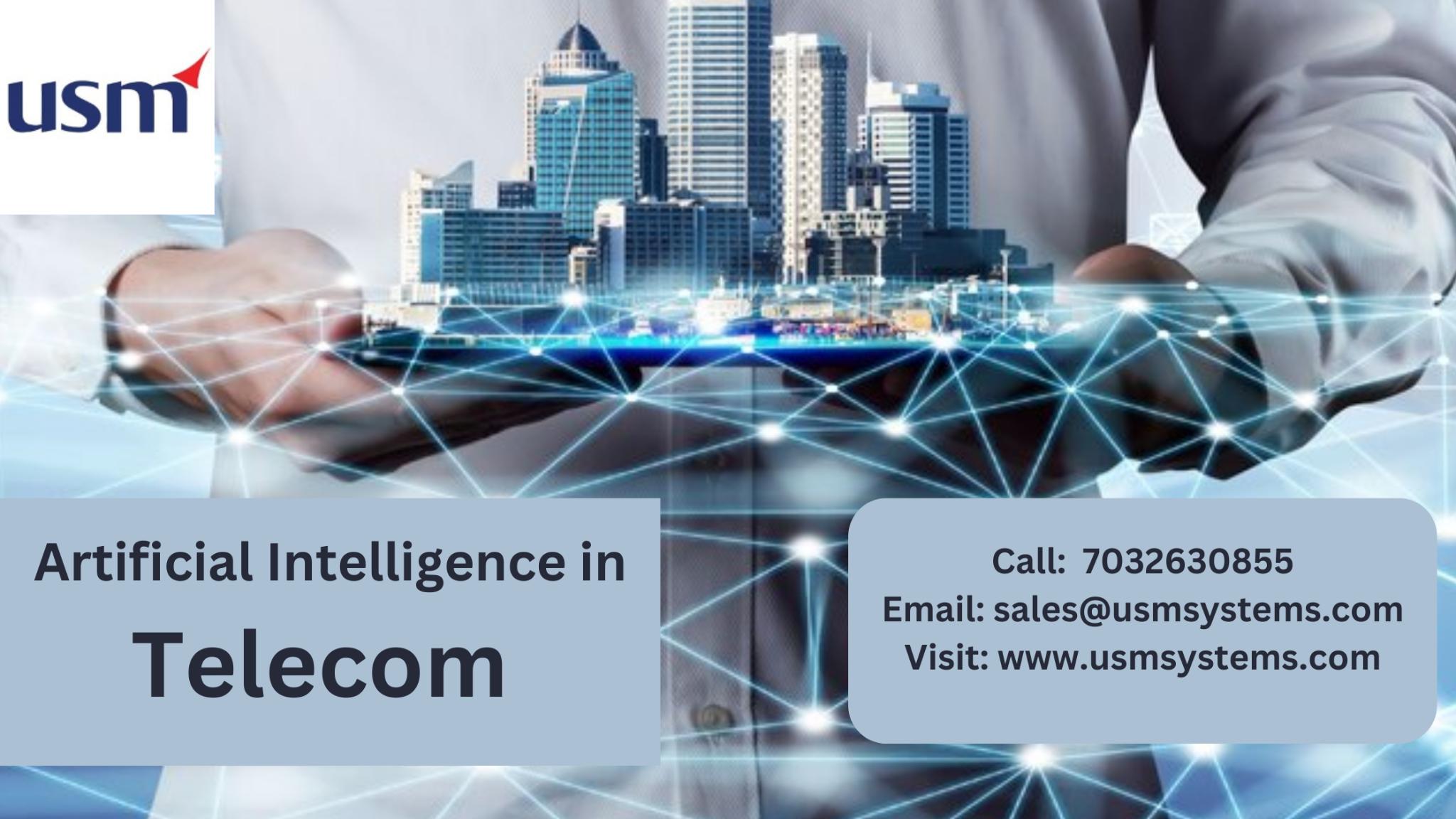
5 Common Applications of AI in Telecommunications:
Forward looking CSPs considered the challenges and focused their AI investments on applications that help solve these challenges. AI in these areas is already beginning to deliver tangible business results
1 Predictive maintenance:
AI powered predictive analytics leverages data, sophisticated algorithms, and machine learning techniques to predict future outcomes based on historical data to help telecom companies better serve them. This means operators can use data-driven insights to monitor equipment health and predict failures based on patterns Implementing AI in communications also enables CSPs to proactively address communications hardware issues such as base stations, power lines, data center servers, and set-top boxes in customer homes.
2.Virtual assistant for customer support:
Another AI in telecom industry use cases is conversational AI platforms Also known as virtual assistants, they have learned how to efficiently automate and scale one on one conversations The adoption of AI in telecoms helps to handle the numerous support requests for installation, setup, troubleshooting and maintenance that often overwhelm customer service centers
Operators can use AI to implement self-service capabilities that show customers how to install and operate their own devices
3.Robotic Process Automation (RPA) for Communications:
CSPs have a vast number of customers that engage in millions of transactions every day, each of which is susceptible to human error Robotic Process Automation (RPA) is a form of business process automation technology based on AI. RPA can increase the efficiency of telecommunications functions by making it easier for telcos to manage back office operations and large volumes of repetitive, rule-based tasks RPA simplifies the execution of complex, labor intensive, and time consuming processes such as billing, data entry, personnel management, and order fulfillment, enabling CSP staff to perform higher value added tasks
4 Fraud Prevention:
Telecommunications is leveraging the powerful analytics capabilities of AI to prevent cases of fraud. The role of artificial intelligence in accounting is AI and machine learning algorithms can detect anomalies in real time, effectively reducing communications related fraudulent activity such as unauthorized network access and fake profiles. As soon as suspicious activity is detected, the system automatically blocks access to scammers to minimize damage.
5.revenue growth:
AI has the powerful ability to integrate and understand a wide range of data such as device, network, mobile applications, geolocation data, detailed customer profiles, service usage and billing data. Telecom can use AI-powered data analytics to increase subscriber growth and average revenue per user (ARPU) through smart upsell and cross sell of services By using real time context to anticipate customer needs, telecom companies can make the right offer at the right time through the right channel.
Most of the current AI Examples focus on improving certain parameters
1. Optimize the parameters of the radio signal:
Currently, machine learning (ML) is used to optimize the flow of data to and from base stations (BTS) in mobile networks The distance to the user, the connected user, and certain environmental factors determine the wireless parameters They in turn determine the maximum amount of data that can be transmitted per amount of spectrum per unit time. Interference also plays a role Radio resources can be coordinated between micro cells and macro cells For maximum efficiency, algorithms are used to dynamically determine which parts of the spectrum should be used for which users and with which parameters. The parameters of these algorithms can be “tuned” by AI
2. Power Management:
In active mobile networks, power savings are achieved via machine learning algorithms Based on weather data, number of users and location, the antenna actively adjusts the radiation pattern, direction and intensity based on demand This results in more efficient use of base stations, for example, by saving energy at night when data demand is relatively low and allowing a larger surface area to be operated at set points where capacity demands are not uniform.
3. Transmission Estimated Quality:
Optical connections can cause interference or interruption of the signal and cause permanent equipment failure Machine learning is applied to predict ahead of time how well a transport will perform over a connection. Calculates the optimal route based on cable length, other signals in the cable, equipment life, and more Traffic is routed based on this assessment Such algorithms might potentially be applied in wireless networks. For example, how much error correction or redundancy (eg retransmission) is used Professional systems and machine learning algorithms are two widely used AI Services in the telecommunication field, and ML and distributed artificial intelligence are the two most promising AI technologies for the future.
AI and Machine Learning:
AI and Machine learning development companies in USA are transforming and transforming businesses These technologies can be used by telecom firms to increase customer satisfaction, enable self-service, enhance equipment maintenance, and cut costs all at once.
Self-Learning and Behavior
AI applications typically take over tasks performed by or supporting humans This means that these systems have some degree of autonomy There are two forms of independence: self-learning and autonomous behavior.
Self study:
Today's AI models are developed based on large amounts of (historical) data. Algorithms 'learn' the desired outcome from this data with specific inputs There are several ways to shape this learning or 'training'.
Offline training:
the model is trained once or very often on a static data set. The model and the data used can be tested and validated before the model is produced
Online Learning:
The model is trained like offline training and then periodically retrained based on new data
Continuous Learning:
Continuously updates the model using incoming data Unlike online learning, there is no longer another 'version' of the model. Each inference request potentially directly affects the next AI decision
Challenges facing telecom companies today
Today's telecommunications service providers (CSPs) face internal and external challenges
Poor network management:
The rapid increase in global traffic and the need for more network equipment makes network management more complex and expensive
Lack of data analysis:
Telecom companies are struggling to leverage the vast amounts of data they have gathered from their vast customer bases over the years Data may be fragmented or stored across multiple systems, unstructured and unclassified, or simply incomplete and not very useful
High cost:
Following massive investments in infrastructure and digitization, industry analysts expect telecommunications companies' global operational spending to grow by billions of dollars. Many telecommunications companies are facing a financial crisis and need to find ways to improve their bottom line.
Crowded market:
Telecom customers are demanding higher quality of service and better customer experience (CX) and are known to be particularly prone to churn when their needs are not met
The Future of telecommunications with AI:
AI will become an integral part of the future digital market Growing adoption of AI in the telecom industry enables CSPs to manage, maintain and optimize their infrastructure and support operations The use cases mentioned in this article demonstrate the impact of AI on the telecom industry. This enables businesses to deliver an improved customer experience and increase business value The use of AI in the telecommunications industry is booming And it's still growing, and experts expect it to grow at least 42% this year
Conclusion: Leveraging AI in telecommunications offers many benefits to telecommunications service providers AI and automation can reduce operating costs while keeping networks healthy and secure This is why telecom companies value the best Artificial intelligence companies in USA and look forward to adopting AI for better business opportunities. AI's unparalleled ability to analyze massive amounts of data and prevent downtime and service outages is likely to have even greater scope in the future.
If advances continue, AI, combined with other innovative technologies such as machine learning and computer vision, could greatly help the telecommunications industry address its current and future challenges
If you are interested read here to know more about : Computer Vision Applications in 2022