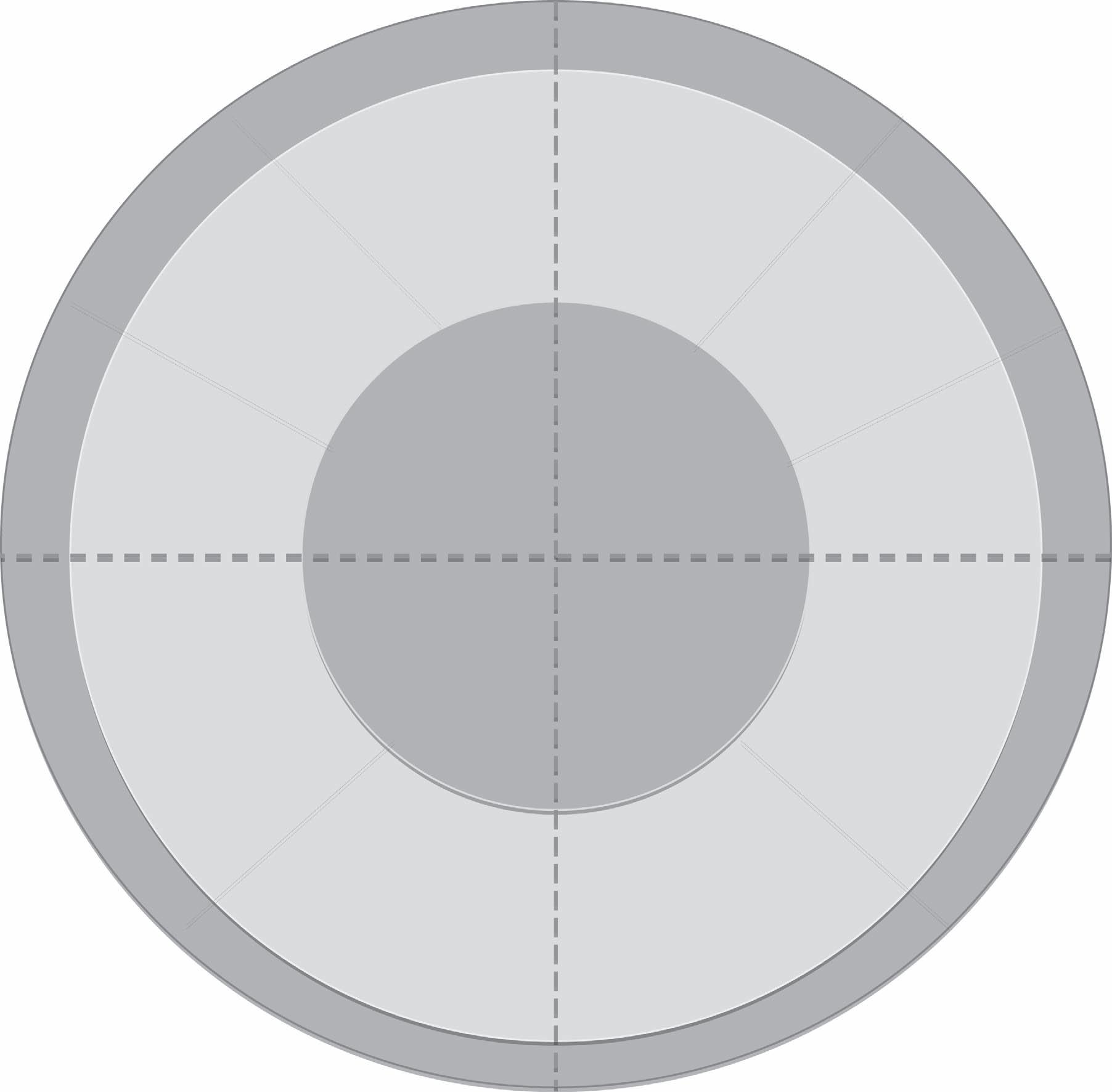
70 minute read
Best Practices and Recommendations for Digital Interventions to Improve Engagement and Adherence in Chronic Illness Sufferers Maria Karekla, Orestis Kasinopoulos, David Dias Neto, David Daniel Ebert, Tom Van Daele, Tine Nordgreen, Stefan Hofer, Svein Oeverland, and Kit Lisbeth Jensen
by Hogrefe
Special Issue: Adjustment to Chronic Illness Original Articles and Reviews
Best Practices and Recommendations for Digital Interventions to Improve Engagement and Adherence in Chronic Illness Sufferers
Advertisement
Maria Karekla, 1,2,3
Orestis Kasinopoulos, 1
David Dias Neto, 2,4
David Daniel Ebert, 3,5 Tom Van Daele, 3,6
Tine Nordgreen, 3,7
Stefan Höfer, 2,8
Svein Oeverland, 3,9 and Kit Lisbeth Jensen 3,10
1
Department of Psychology, University of Cyprus, Nikosia, Cyprus 2
Psychology and Health Standing Committee of the European Federation of Psychology Associations, Brussels, Belgium 3
e-Health Task Force of the European Federation of Psychology Associations, Brussels, Belgium 4
APPsyCI –Applied Psychology Research Center Capabilities & Inclusion, ISPA –Instituto Universitário, Lisbon, Portugal 5
Friedrich Alexander University, Erlangen-Nürnberg, Germany 6
Thomas More University of Applied Sciences, Belgium 7
Division of Psychiatry, Haukeland University Hospital, Bergen, Norway 8
Medical University Innsbruck, Austria 9
SuperEgo AS, Norway 10
Clinical Psychology, Private Practice, Denmark
Abstract: Chronic illnesses cause considerable burden in quality of life, often leading to physical, psychological, and social dysfunctioning of the sufferers and their family. There is a growing need for flexible provision of home-based psychological services to increase reach even for traditionally underserved chronic illness sufferer populations. Digital interventions can fulfill this role and provide a range of psychological services to improve functioning. Despite the potential of digital interventions, concerns remain regarding users’ engagement, as low engagement is associated with low adherence rates, high attrition, and suboptimal exposure to the intervention. Human–computer interaction (e.g., theoretical models of persuasive system design, gamification, tailoring, and supportive accountability) and user characteristics (e.g., gender, age, computer literacy) are the main identified culprits contributing to engagement and adherence difficulties. To date, there have not been any clear and concise recommendations for improved utilization and engagement in digital interventions. This paper provides an overview of user engagement factors and proposes research informed recommendations for engagement and adherence planning in digital intervention development. The recommendations were derived from the literature and consensualized by expert members of the European Federation of Psychology Associations, Psychology and Health Standing Committee, and e-Health Task Force. These recommendations serve as a starting point for researchers and clinicians interested in the digitalized health field and promote effective planning for engagement when developing digital interventions with the potential to maximize adherence and optimal exposure in the treatment of chronic health conditions.
Keywords: digital interventions, e-health, recommendations, adherence, engagement
Chronic health illnesses cause considerable burden to individuals, families, and society, with significant impact on physical, emotional, and social functioning (Breivik, Collett, Ventafridda, Cohen, & Gallacher, 2006; Sprangers et al., 2000). Although there is a growing body of evidence that pharmacological and psychosocial interventions can effectively treat illness-related interference in daily life, numerous chronic illness sufferers remain untreated or inadequately treated. This is partly a result of access, mobility, and transportation problems (Jerant, von FriederichsFitzwater, & Moore, 2005); financial barriers; reluctance to seek treatment; and paucity of clinicians trained in evidence-based multidisciplinary treatments (Breivik et al.,
2006; Jamison, Gintner, Rogers, & Fairchild, 2002; Jensen, Nielson, Romano, Hill, & Turner, 2000; Shapiro, Cavanagh, & Lomas, 2003). In an attempt to overcome such barriers to treatment, an interest in home-based selfmanagement support has emerged (Jerant et al., 2005). Of particular interest are digital interventions (used here as an umbrella term for e-health, m-health, Internet-based, text message, self-management interventions, etc.) aiming to improve health care for persons with chronic conditions in the convenience of their own space and time (Bender, Radhakrishnan, Diorio, Englesakis, & Jadad, 2011; Bennett & Glasgow, 2009; Keogh, 2013; Long & Palermo, 2009; McGeary, McGeary, & Gatchel, 2012). A digital health intervention is often based on a selfguided approach, executed through the use of a platform (a Website if computer-based or application if smartphone or tablet-based) by consumers who seek help for health or mental health-related issues (Barak, Klein, & Proudfoot, 2009). Different digital intervention delivery vehicles each come with their own unique challenges, as well as opportunities. For example, a smartphone health application allows for Ecological Momentary Assessment (i.e., capturing the user’s experience in the moment and less prone to recall bias) and portability, yet it also comes with a smaller screen size, possibility of battery drain, or a sense of intrusiveness by the user (Yardley et al., 2016). For purposes of this review, a digital intervention is defined broadly as a selfguided Internet- or computer-based health intervention and will not provide an in-depth consideration of each digital platform specifically.
The purpose of digital health interventions is to create behavior change, enhance knowledge, awareness, and understanding for health difficulties, through the use of interactive Web-based components (Barak et al., 2009). Digital health interventions present with numerous benefits over traditional face-to-face ones, including wide accessibility, cost-effectiveness, access to treatment without waitingtime, overcoming stigma-induced barriers through anonymity, and providing quality treatment to the sufferer at their own individual pace (Brouwer et al., 2010; Brug, Oenema, & Campbell, 2003; Brug, Oenema, Kroeze, & Raat, 2005; Cuijpers, Van Straten, & Andersson, 2008; De Nooijer et al., 2005; Savvides & Karekla, 2015). There is amounting support for the efficacy of digital cognitive behavioral interventions in improving management of patients’ health conditions, reducing pain intensity and catastrophizing, and improving physical and psychosocial functioning (Eccleston et al., 2014; Keogh, Rosser, & Eccleston, 2010; Macea, Gajos, Calil, Fregni, 2010; Velleman, Stallard, & Richardson, 2010). However, enthusiasm about the effects of digital-based interventions is tempered by findings presenting users’ failure to engage with the digital media (Macea et al., 2010). Lack of
engagement is associated with low motivation and adherence to the intervention, resulting in high dropout rates affecting treatment outcomes and effectiveness (Carlbring, Westling, Ljungstrand, Ekselius, & Andersson, 2001; C hristensen, Griffiths, & Korten 2002). Understanding the reasons for low engagement and adherence in digital interventions may enable their improved evaluation and possibly lead to increased effectiveness and utility. It is paramount that practical strategies and recommendations to tackle this problem need to be developed.
The Challenge of Digital Health Interventions: (1) Definition and Conceptualization of Adherence and Engagement
Adherence is a critical topic in the rapidly expanding area of digital interventions. Greater adherence to digital interventions leads to healthy lifestyle changes and consequently improved health outcomes (e.g., improved quality of life, reduced mortality rates; Donkin et al., 2011; Manwaring et al., 2008). Conversely, low adherence significantly limits treatment effectiveness, increases discontinuation and dropout (Glasgow, 2007; Hilvert-Bruce, Rossouw, Wong, Sunderland, & Andrews, 2012; Leslie, Marshall, Owen, & Bauman, 2005; Trompetter, Bohlmeijer, Veehof, & Schreurs, 2015). Within the medication literature, adherence is the extent to which a person’s behavior (e.g., taking medication) corresponds with agreed upon recommendations from a healthcare provider (Sabaté, 2003). In digital interventions, adherence is often regarded as the degree to which users engage with the content of an intervention as intended by the developer. Engagement is thus another crucial variable that impacts the effectiveness of digital health interventions.
Unfortunately, there is no consensus definition of engagement and different fields (e.g., computer science, psychology, and behavioral health) conceptualize it differently. The behavioral science perspective of engagement defines it as a behavior in terms of intervention “usage” assessed via duration of contact with an intervention and frequency of visits, thus may be interchangeable with the term “adherence” (Couper et al., 2010a; Kelders, Kok, Ossebaard, & Van Gemert-Pijnen, 2012; Voils et al., 2014; Wang et al., 2012). Given substantial differences in definitions of engagement, a synthesis of factors affecting digital interventions engagement (intra-individual to contextual to designrelated factors) is difficult, hindering the formation of recommendations to guide digital intervention development. Perski, Blandford, West, and Michie (2017) in a recent review attempted to consolidate the literature definitions of engagement. They concluded that engagement is a
multidimensional construct and a dynamic process expected to vary over time both between and within users and is comprised of two parts: “(1) the extent (e.g. amount, frequency, duration, depth) of usage and (2) a subjective experience characterized by attention, interest and affect.” An advantage of this conceptual framework is that it highlights both direct (e.g., content of intervention) and indirect (e.g., beliefs about intervention) influences on engagement; thus, this definition is adopted in this paper.
A related problem facing researchers and digital intervention developers is the challenge of measuring adherence and engagement. Having a clearer consensus definition of these terms can be a first step in establishing means via which to evaluate levels of adherence and engagement, as it is yet unclear what levels of adherence or engagement are desired for interventions to have an effect. Methodologies of evaluation, assessment of reliability, and related statistical issues need to emerge. Also, metrics to assess them (e.g., time spent within an intervention or components of an intervention, logins, intensity, and frequency of engagement with the content) need to be decided.
The Challenge of Digital Health Interventions: (2) Low Adherence and High Dropouts
Almost twice as many chronic illness users drop out from self-guided digital interventions compared to traditional interventions (Macea et al., 2010), whereas guided interventions (i.e., either an actual therapist through e-mail, or a digital character through animation, guides the user through the content) have shown to result in similar adherence rates as face-to-face ones, for conditions such as depression (van Ballegooijen et al., 2014). Even among users who complete a digital intervention as intended, few adhere to the intervention in the desired way envisioned by the intervention creators (Kelders, Van GemertPijnen, Werkman, Nijland, & Seydel, 2011; Wangberg, Bergmo, & Johnsen, 2008). Nonadherence suggests that users are not optimally exposed to the intervention, a dose-response relationship problem, which consequently lessens the effectiveness of digital interventions (Donkin et al., 2011; Manwaring et al., 2008). Proposed reasons for poor adherence include: sociodemographic or user-related characteristics, such as poor digital health literacy (O’Connor et al., 2016); being male (Lorig et al., 2002); lower education level (Karyotaki et al., 2015); and severity and longer chronicity of condition (Lorig, Ritter, Laurent, & Plant, 2006). Eysenbach (2005) proposed that participants in traditional randomized controlled trials (RCTs) go through several levels of clinical filtering prior to entering treatment, an indication of
commitment level. In contrast, the broad, easy, and unfiltered participant enrollment accompanied by the highly accessible digital interventions, may come with the cost of lower motivation or commitment of users to the intervention. This is further supported by a recent systematic review concluding that one of four overarching themes found to affect user engagement and enrollment in digital interventions relates to the engagement and recruitment approach (O’Connor et al., 2016). For example, difficulty understanding the recruitment message or lack of advice from the researcher/developer, as well as lack of clinical endorsement, is linked to poor engagement. Another contributor to low adherence is related to the developers’ approach to technology, envisioning digital means as only mere vehicles of delivering intervention content, without recognition of the dynamic nature of technology and its potential in engaging a user.
Apart from researchers and healthcare professionals, nonacademic or nonmedical, technical developers (e.g., programmers) are involved in digital interventions. Teams of IT technical developers and business specialists (developers, designers, user experience specialists, marketing specialists) as well as researchers or healthcare professionals need to efficiently collaborate among them, for successful implementation, continued development and evaluation of the digital intervention (Michie, Yardley, West, Patrick, & Greaves, 2017). In this paper, the term “researcher” will be used to account for the person who is often in direct contact with the user whereas the term “developer” will be used for the person commissioned to develop and program an information technology (IT) product.
This paper aims to propose research informed recommendations for engagement and adherence planning in digital interventions for chronic health conditions. It does not constitute an exhaustive set of recommendations based on all relevant theory, nor does it suggest that the theories and recommendations included are superior to those that are not been discussed. The purpose of this paper was twofold: (1) to serve as a starting point for researchers and developers interested in developing or utilizing digital health interventions and (2) to propose research informed recommendations for increased engagement and adherence planning in digital interventions for chronic health conditions.
Methods
The first two authors used Google Scholar and PubMed as the main search engines, and conducted an electronic search of the relevant literature dating back to 1965. To identify key papers, search terms included: “digital health interventions,” “e-health, m-health, chronic illness,”
Recommendation 3
Recommendation 1
The planning of digital interventions should take into account relevant ethical considerations Digital interventions should consist of theory driven evidencebased psychological intervention content Recommendation 2
The design of digital interventions should include a-priori considerations of adherence utilizing a digital theory-driven approach
Dimension I: A-priori theoretical planning Recommendation 4
Apply theory-driven and empirically supported technological characteristics in digital interventions Digital interventions should, if suitable, include human or a sense of human contact to promote user accountability Recommendation 5
Dimension II: Human –Computer Interaction
Digital interventions benefit from frequent content update
Recomme n dation 6
Digital interventions may utilize web-metrics to assess and monitor adherence of disengaged users Recommendation 10 Dimension IV: Active assessment of usage
Instructions for use should be simple and direct
Recommendation 9 Dimension III: Tailoring and targeting to user groups
Digital interventions should include an assessment of computer knowledge and experience and provide easy tutorials and technical assistance Recommendation 7 Recommendation 8 Digital interventions should be designed taking into account known user characteristics that improve adherence
Figure 1. Diagram summarizing dimensions and recommendations for improving engagement and adherence of chronic illness sufferers in digital interventions.
“recommendations,” “best practices,” “adherence,” “user engagement,” “system design,” “dropouts,” and “psychological theory.” An emphasis was placed in identifying systematic reviews and meta-analyses, and article references were also checked to identify additional relevant papers. All types of articles were included except articles that were not peer-reviewed or not in English. The authors independently reviewed identified papers, coding for challenges presented to user adherence and engagement and recommendations provided.
A list of generated areas of importance was created by each of the two authors, and then these were discussed and grouped into categories/dimensions to formulate the proposed recommendations. These were then presented to expert members of two European Federation of Psychology
Associations’ (EFPA) committees: Psychology and Health Standing Committee and e-Health Task Force. Feedback was received from the experts of the two committees, and adjustments were made to the final consolidated list of recommendations.
Results
The final consolidated list consists of 10 recommendations organized around four dimensions: (I) A-priori Theoretical Planning, (II) Human–Computer Interaction, (III) Tailoring and Targeting to User Groups, and (IV) Active Assessment of Usage (see Figure 1). The format of each
recommendation consists of: (1) a Rationale section and (2) a practical Recommendation section to facilitate the readers in conceptualizing the theory and the application of each recommendation. The dimensions and their associated recommendations are interdependent, and while some overlap exists among them, it is suggested that they are considered in their entirety. Challenges faced by digital interventions in engagement and adherence as well as their respective recommendations and practical examples can be found in the Electronic Supplementary Material, ESM 1.
Recommendations
Dimension I: A-Priori Theoretical Planning 1. The Design of Digital Interventions Should Include A-Priori Considerations of Adherence Utilizing a Digital Theory-Driven Approach Rationale. Psychologist creators of digital interventions tend to focus exclusively on their psychological content and may merely transfer face-to-face treatment content into a digital medium (e.g., transport the written content onto a Website; van Gemert-Pijnen et al., 2011). Though this approach intuitively appears beneficial, what tends to be lacking is the consideration for the unique technological abilities of digitalization that can potentially provide new ways of interacting with chronic illness patients. Utilization of a theoretical design framework in digital intervention planning cultivates and maintains user engagement and motivation to adhere to the intervention throughout its intended duration.
Examining the literature on digital interventions suggests that most digital programs evaluated are not rooted in specific theoretical frameworks (Christensen, Griffiths, & Farrer, 2009). A systematic review of 83 digital interventions reported that only a third (33 studies) had planned a-priori to include design principles to address adherence (Kelders, Kok, Ossebaard, & Van Gemert-Pijnen, 2012). In addition, half of the studies (N = 18) that addressed adherence stated that encouraging adherence is a task for a counselor, thereby ignoring the potential of technology. Of the remaining 15 studies that mentioned planning to increase adherence, 8 did so without any theoretically driven design basis (i.e., digital intervention design theory). Adapting digital interventions to promote adherence is often done in an ad hoc manner without relying on a theoretical framework for the design of such interventions, missing in the process capabilities that would make the digital interventions more appealing and potentially improve adherence.
Recommendation. Creators of digital health interventions are encouraged to investigate and plan a-priori for the design of digital interventions using a theoretical framework or approach, which aims to engage or motivate users
to adhere to the intervention. It is recommended that researchers keep abreast of the latest research regarding theory-driven digital intervention design. Frequently used search terms to obtain access to theory-driven design frameworks may include: design, adherence, user engagement, theory-driven and/or framework, e-health, digital, motivation. Furthermore, it is important that researchers include and clearly describe the use of a-priori design planning in their methodology.
To our knowledge, there is a scarcity in digital design theories reported in the literature. The two most widely researched theories, which will be emphasized in this paper are: (1) gamification and (2) persuasive technology. Gamification is the use of game design features in nongame contexts such as healthcare applications or digital interventions (Deterding, Dixon, Khaled, & Nacke, 2011). Persuasive technology is a theory-driven digital intervention design framework, which suggests that technology itself has the capacity of being persuasive (or engaging) through its role as a tool, a medium, and a creator of experiences (Fogg, 2002). The rationale and application of the features comprising these two conceptual approaches are discussed in more detail in the recommendations that follow.
2. Digital Interventions Should Consist of Theory-Driven Evidence-Based Psychological Intervention Content Rationale. The Web is filled with information, however, the credibility of the material and sources producing the material are often put to question and may even confuse users. Indeed, a systematic overview of the impact of e-health on the quality and safety of health care, reports that numerous digital interventions make claims, which are not substantiated by empirical evidence (Black et al., 2011). Globally, there is a challenge to implement and disseminate empirically supported psychological treatments. Fairburn and Patel (2017) report that the coverage of such treatments does not exceed 50% in any country. Morrison (2015) argues that in order to enhance the impact and usage of digital interventions, it is essential that psychological theories (such as theories of social support and motivation) guide and inform the designing and delivering of these interventions. Therefore, best practices of digital interventions need to be implemented, in order for empirically supported treatments to be disseminated widely.
According to persuasive technology theory, a system must convey to the user a sense of system credibility (Fogg, 2002), through the following principles: (1) trustworthiness (i.e., system providing truthful, fair, and unbiased information); (2) expertise (i.e., system providing information demonstrating knowledge, experience, and competence); (3) surface credibility (i.e., similar to face validity, a system should provide a sense of credibility to the users upon first inspection); (4) real-world feel (i.e., system providing
information or means to communicate with the people behind its content); (5) authority (i.e., system quoting that the material originates or has been evaluated by an acknowledged authority); (6) third-party endorsements (i. e., system providing endorsements from respected and renowned sources, such as a university); and (7) verifiability (i.e., system providing means to verify the accuracy of the site content via outside sources such as peer-reviewed research articles). A system that uses content from theory-driven evidence-based psychological approaches may provide a sense of trustworthiness, expertise, and credibility to the user. Previous research indicated that perceived credibility (as indicated by trust/expertise and depth) was significantly related to the intention of the user revisiting a Website (Hong, 2006). Recommendation. It is encouraged that digital interventions for health-related problems, be based on theory-driven empirically supported psychological interventions. For example, a digital intervention for pain management may base its contents on an evidence-based psychological intervention, such as Acceptance and Commitment Therapy (Hayes, Strosahl, & Wilson, 1999), classified as an empirically supported treatment for general chronic pain conditions (Society of Clinical Psychology, Division 12 of the APA, 2011). Such an example is the recently developed “AlgeApp” chronic pain digital intervention (Karekla et al., 2017). To provide a sense of trustworthiness and credibility, the seven persuasive technology theory principles can be utilized. If the content is provided in written formats, citations, and empirical references supporting the approach can be used; whereas if the content is provided in an audiovisual dynamic format, links or preferably nonintrusive pop-up information should be accessible to the user who wants to check the credibility of the source.
3. The Planning of Digital Interventions Should Take Into Account Relevant Ethical Considerations Rationale. Many users seek help through digital interventions because they perceive this medium to offer numerous benefits (e.g., convenience of time and space, anonymity, lack of stigma). However, the absence of a physical presence may present with several challenges regarding ethical considerations, which may act as a barrier to the trustworthiness and safe use of the intervention (Hsiung, 2001). Perhaps one of the most significant concerns is privacy of sensitive information (Arora, Yttri, & Nilsen, 2014). Confidentiality is generally recognized to constitute the basis for a helping relationship and building of trust (Haas & Malouf, 2002; Koocher & Keith-Spiegel, 1998). However, therapists need to be able to maintain practices of emergency and to act in the best interest of their clients. The ease of accessibility and relative anonymity within digital
interventions may paradoxically make it more challenging to identify participants who might be in crisis (e.g., having suicidal or homicidal thoughts) or suffer from additional mental health problems and may need additional care (e.g., active psychosis, manic episodes, or substance abuse disorders). Ethical issues and especially ones of privacy, confidentiality, and emergency should be considered when planning a digital intervention, including consideration for the rights of non-research participant users. Recommendation. Ethical considerations and solutions (particularly informed consent, anonymity and confidentiality, promoting welfare and avoidance of harm, and competency) should be considered during the developing stages of a project. Operating procedures need to be established so as to deal with ethical issues that may arise (Arora et al., 2014). Developing a-priori practices and principles of conduct for digital health projects is a crucial step in enhancing data collection and ensuring participant safety. According to the International Society for Mental Health Online (ISMHO, 2000) and the European Group of Ethics (2012), researchers should provide all users with an informed consent consisting of a detailed description of: (1) the purpose and process, (2) contact details of researchers, (3) any potential benefits (e.g., convenience of time and space, low cost, anonymity), (4) any risks that may arise from the use of the digital intervention (e.g., likelihood of technical difficulties, breach of confidentiality if the digital means are used in public areas or by others), and (5) safeguards taken to ensure confidentiality and privacy (e.g., use of encrypted platform of communication). Additionally, providing the users with transparency (clearly informing users) and the right to withdraw from the study or have their data deleted should be fundamental for all digital interventions (European Group of Ethics, 2012). The inclusion of participants with certain mental health problems (e.g., active suicidal ideation, psychosis, manic episodes) or at-risk populations (e.g., drug users) entails risks and especially ethical concerns related to mandated reporting of suicidal, homicidal, or abusive situations. Creators of digital interventions need to assess from the beginning for these situations and establish action plans on how to deal with them, if they arise. Further, inclusion of comorbid active mental health problems may interfere with an intervention, contribute to nonadherence, or may render a program not suitable for the needs of these participants. It is paramount that users are informed about the parameters of the program and are provided with alternative treatment options or a stepped care model of treatment. Overall, digital programs should provide users with relevant and accurate information regarding the content and goals of the specific program, including expected outcomes following program completion. The absence of such information leads to false or unrealistic expectations,
disappointment or lack of motivation to engage with the program, and consequently non-completion. For example, if a digital intervention aims to aid individuals with chronic pain to live a more valued life, users whose primary goal is to get rid of their pain or reduce its intensity are likely to feel unmotivated or even tricked by the researchers. This in turn will affect their engagement, adherence, and future involvement with digitalized interventions.
Dimension II: Human–Computer Interaction 4. Apply Theory-Driven and Empirically Supported Technological Characteristics in Digital Interventions Rationale. As stated in recommendation 1, digital intervention developers and researchers are encouraged to plan a-priori for adherence using theory-driven approaches to digitalization. This recommendation presents details regarding the most prominent theory-driven approaches and provides examples of how they can best be applied in practice.
Gamification, utilizes behavioral principles of, for example, positive reinforcement, along with mechanisms found to make games addictive and implements these principles in nongame digital situations (Cugelman, 2013). Reeves and Read (2009) present the following gamification principles as important: self-representation with avatars, threedimensional environments, narrative context, feedback, competition among users via ranks and levels achieved within a game, rewards, teaming up and communicating in parallel with others to achieve a goal, and time pressure. Examples of gamified positive reinforcement include ingame rewards, badges, and challenges. These are found to be highly effective resulting in high usage and adherence (Cobb & Poirier, 2014). Similar support has been provided for gamification components of, visualization of progress and automated goal setting activities (Irvine, Gelatt, Seeley, Macfarlane, & Gau, 2013), and inclusion of a story line (Imamura et al., 2014). A recent systematic review of 61 RCTs evaluating 47 different intervention programs and their effects on adherence (Brown et al., 2016) found active gamified ingredients to explain only 9.4% of variance, suggesting that gamification features may not impact adherence to the level expected. Future studies are needed to compare interventions with versus without the inclusion of gamified elements (and different types of elements) for improving adherence, utilizing rigorous randomized trial methodologies. Until more research becomes available, researchers should be cautious when designing digital interventions to improve adherence based on gamified models alone.
Persuasive technology is another dominant theory-driven digital intervention design framework. A persuasive system may be defined as an information system (e.g., computerized software, Web-based intervention, smartphone
application), which by its nature can reinforce, change, or shape attitudes and/or behaviors (Oinas-Kukkonen & Harjumaa, 2009). Although it has been argued that all information systems may be regarded as capable of influencing users in some way (Oinas-Kukkonen, 2013), for a technology to be called persuasive, the persuasion has to be intentional or designed purposefully to guide the user toward behavior change (Fogg, 1997). Fogg (2002) proposed the “Persuasive System Design” (PSD) framework comprised of 28 technological characteristics in order to guide the design of interventions with an eye toward engagement and user adherence (Oinas-Kukkonen, 2013). These principles are distributed into the following 4 categories: (1) primary task support: principles supporting the core activity or behavior; (2) dialogue support: principles related to the user receiving feedback from the system; (3) system credibility support: principles responsible for making the system seem credible and trustworthy; and (4) social support: principles inducing motivation through social influence. A review and meta-analysis of 83 digital interventions examining whether persuasive design influences adherence (Kelders et al., 2012) found 55% of variance in adherence being explained by human–computer interaction variables, such as PSD. Lacking from analysis was the category of “system credibility support,” as included studies did not report on this feature. Multiple regression analysis suggested that only the “dialogue support” category significantly contributed to adherence. Dialogue support features aim to engage the user through human–computer interaction via providing a significant degree of system feedback in a way that helps users keep moving toward their stated goal or target behaviors. An example of a dialogue support feature would be the use of text or e-mail reminders between intervention sessions to remind a user of a pre-reported goal (e.g., fitting into a dress in the case of a weight-loss program, or of improving breathing capability in a smoking cessation trial). Dialogue support features consist of: praise, rewards, reminders, suggestion, similarity, liking, and social role. Despite the significant contribution of dialogue support features in user adherence, Kelders and colleagues (2012) found that they are less commonly employed in digital interventions, with a mean use of 1.5 out of 7 possible features used in the interventions examined. Reminders and suggestions were the two most frequently employed features, while positive reinforcement (e.g., praise and rewards) was seldom used. This is interesting when considering that positive reinforcement is among the best means of establishing new behaviors and is one of the most empirically supported techniques available (Abraham & Michie, 2008; Michie et al., 2013). Yet, it appears that when transporting behavior change programs into digital means, the creators neglect the basic psychological theories of change (see also Recommendation 2).
Recommendation. It is recommended that interventions be developed based on persuasive technology and particularly by incorporating the 7 dialogue support system features. Specific suggestions include: Praise: A key operant conditioning component is providing positive verbal reinforcement when a desired behavior is observed. Fogg (1997) found that a single dialog box praising the user was enough to engage, motivate, persuade, and empower the user without any actual available social presence (i.e., of a therapist). Praise can include words, images, symbols, or sounds as a way to provide feedback information based on exhibited behavior. An example can be the use of automated text messages for reaching individual exercise goals each day. Rewards: Providing rewards works via a similar positive reinforcement mechanism as praise. This feature is common to both persuasive and gamified interventions as a means to signal achievement to the user. Examples of virtual rewards are budges, virtual trophies, allowance to alter media items (e.g., change sounds, backgrounds, avatar gender), or points that can be cashed in for an external reward (e.g., airline miles bonus; Liu, Hodgson, Zbib, Payne, & Nolan, 2014). Successful application of rewards follows traditional behavioral schedules of reinforcement and involves systematic and successive rewarding of desired behaviors while switching to intermittent rewards midway (Reynolds, 1968). Reminders: The use of reminders to prompt target behaviors is again rooted in behavior theory and particularly the concept of shaping desired behavior. Digital intervention trials present the importance of reminders in increasing adherence, intervention effectiveness, and the likelihood that the user will achieve their goals (Neff & Fry, 2009; Webb, Joseph, Yardley, & Michie, 2010). Reminders should be frequent; however, the exact frequency is not yet determined; thus, this is stated with caution as many reminders may lead to notification fatigue (Dennison, Morrison, Conway, & Yardley, 2013; Proudfoot et al., 2010). Additionally, the provision of automatic reminders may lower the cost of an intervention while increasing completion rates (Titov et al., 2013). Suggestion: Suggestions, similar to reminders, tend to be more direct. Suggestions often imply that those behind the system are experts providing their opinion about a particular behavior and its change. Suggestions may include recommendations based on empirical findings and offered in response to a user’s comment, data input, or reactions to questions posed within the intervention, etc. In order for suggestions to be effective, they have to provide the right content at the right time. An example illustrating the use of suggestions is in cases of promoting sleep hygiene in patients with chronic pain, and an automated message is sent to the user in the afternoon, proposing that they focus on activities and remain awake until the evening. Similarity: System components should be designed to appear familiar to users in some meaningful way. According to the theory of self-efficacy (Bandura, 1982), people complete a task if they perceive themselves as capable of executing it, especially after observing someone they consider similar to themselves performing the behavior. An example of utilizing the principle of similarity includes, in a digital intervention for chronic pain management, avatars (in the role of psycho-educators) are designed to visually resemble an average chronic pain user in terms of age, gender, language spoken, and health condition (e.g., exhibit movement difficulties; Karekla, 2017). Liking: Involves the development of a system that is aesthetically attractive to its users. Geissler, Zinkhan, and Watson (2006) state that aesthetically appealing designs encourage users to continue browsing. Sonderegger and Sauer (2010) manipulated the visual appearance (highly appealing vs. non-appealing) of two functionally identical mobile phones and found that participants with highly appealing phones rated their appliance as more usable than participants with the unappealing model. Designs that use a variety of well-designed images, videos, and animations are more likely to capture users’ interest and engage them in the intervention. Social role: Social role presents the medium through which the 7 system credibility principles (trustworthiness, expertise, surface credibility, real-world feel, authority, third-party endorsements, and verifiability; Fogg, 2002) can be transferred to the user. This medium might be in the form of a virtual character who also has a real-world feel and might have the role of a healthcare specialist, a “co-traveler,” a psycho-educator, a co-patient, and so forth (Karekla, 2017).
5. Digital Interventions Should, if Suitable, Include Human or a Sense of Human Contact to Promote User Accountability Rationale. Human or a sense of human contact has several layers and involves: building a therapeutic alliance, feeling accountable, and opportunity to contact a human if needed. Poor therapeutic alliance is a significant predictor of premature termination (Mohl, Martinez, Ticknor, Huang, & Cordell, 1991). In face-to-face interventions, the therapist can coordinate his/her actions while simultaneously delivering intervention content, so as to establish and maintain a therapeutic relationship that will benefit and keep the client interested to continue the treatment. In digital interventions, however, content is provided through a
medium (e.g., mobile device) in the form of texts, e-mails, and phone calls, which may create an obstacle for a therapeutic bond to be effectively built. Jasper and colleagues (2014) found that more time was needed to build a strong alliance in a digital intervention compared to a face-to-face intervention. Obstacles to fostering alliance in digital interventions included having too little information on the therapist behind the intervention.
The model of supportive accountability (Mohr, Cuijpers, & Lehman, 2011) proposes that effective researcher–user interaction can be achieved when mediated by a sense of accountability. Accountability refers to implicit or explicit expectations that an individual may be called upon to justify their actions or inactions (Lerner & Tetlock, 1999). Accountability may be created when clear adherence goals are stated, and there are monitored and scheduled contacts to review the user’s progress. Yet, for accountability, it is necessary that researchers must be perceived (through their electronic interaction) by the users as trustworthy, benevolent, and expert (Mohr et al., 2011). There is evidence to suggest that when the users are accountable for their active participation in the intervention in a broken-down, processoriented (step-by-step) expectation (e.g., see following recommendation section), they are more likely to complete the target behaviors and produce better outcomes than when the user is accountable in an outcome-oriented expectation (Adams, 1965; Konow, 2000). Additionally, the Efficiency Model of Support (Schueller, Tomasino, & Mohr, 2016) suggests that providing support resources should be based on assessing areas that the user may be lacking in (e.g., motivation, meeting needs of user, having sufficient knowledge to use an online tool). Human contact in this model is used to address these areas, which may further contribute to the therapeutic alliance (Schueller et al., 2016). Digital interventions with some form of human support have a significantly greater success in engaging users than those without therapist guidance (Palmqvist, Carlbring, & Andersson, 2007). Baumeister, Reichler, Munzinger, and Lin (2014), in a systematic review, found that digital interventions with accompanying therapeutic support had a significantly lower dropout rate (OR = 2.67), more implemented modules per intervention (g = 0.52), and achieved great reduction of symptoms (g = 0.27) than interventions without such support. Reviews examining the dose-efficacy relation support that the number of completed modules rises with increased human support time. However, an increase of therapist time beyond 100 min per patient within a 10-week digital intervention was found to have no incremental additional effect on efficacy (Andersson, Carlbring, Berger, Almlöv, & Cuijpers, 2009; Johansson & Andersson, 2012; Palmqvist et al., 2007). Recommendation. In order to establish a therapist-client relationship as in traditional therapeutic modalities, enhance accountability, and provide the sense of human contact, digital programs are encouraged to ask the users a-priori for permission to electronically interact with them. It may be explained to users that permission to interact is sought, so as to provide useful and tailored feedback for improving their experience and enhancing possible benefits from the digital intervention. This interaction should be frequent (but not excessively so, though the exact helpful amount has not yet been determined), by providing encouragement and tailored feedback according to responses (utilizing previous recommendations). A way to foster therapeutic alliance could involve the therapist or animated virtual characters purposely revealing information about them, especially if offered details present a shared perspective with the user.
Utilization of broken-down, process-oriented expectations of usage, where the users’ active involvement is sought, is more likely to achieve accountability. Examples of process-oriented expectations include instructions to complete one module per week, to complete the specific activity within a specific time frame, to keep a weekly record of thoughts, and to log in x times per week. Active involvement may come in the form of users being provided with the option of what time during the day they may log in or be prompted (similar to making an appointment). Generic outcome-oriented expectations (e.g., instructions to complete all modules and activities) should be avoided.
Information about the development of the intervention and the human entities (e.g., researchers) behind an intervention should be made available to the user. Also a way to contact the researchers if need arises should be made available. Finally, as proposed by the Efficiency Model of Support, assessing and conceptualizing the needs of the user prior to providing human contact (e.g., if a user appears unable to complete a weight diary as intended probably due to low computer literacy as assessed prior to the intervention may be contacted by a human via telephone to guide them through its completion) may be more beneficial (Schueller et al., 2016).
6. Digital Interventions Benefit From Frequent Content Update Rationale. Digital intervention visits tend to decrease sharply after the initial weeks of use. Regular and frequent intervention content updates, that are made known to users through e-mail or text prompts, are related to repeated visits and higher frequency of user logins (Brouwer et al., 2011). Kelders and colleagues (2012) found that frequent updates of digital interventions are associated with user adherence.
Recommendation. It is recommended that digital interventions are frequently updated with new content, addition of new modules, or with changes in the layout. Updates are more likely to attract the user’s attention if followed by e-mail or text prompts informing about updates.
Dimension III: Tailoring and Targeting to User Groups 7. Digital Interventions Should Be Designed to Take Into Account Known User Characteristics That Improve Adherence and Engagement Exploration of user-related sociodemographic variables provides useful information in understanding and predicting which individuals are at dropout risk. Numerous user characteristics and sociodemographic variables (e.g., gender, age, duration and severity of illness, quality of relationships, and computer literacy) are associated with lower adherence and/or higher dropout rates (Carlbring et al., 2001; Lange, Van De Ven, & Schrieken, 2003; Macea et al., 2010; Melville, Casey, & Kavanagh, 2010; Zarski et al., 2016). Knowledge of characteristics that place individuals at risk for dropping out enables the early identification of disengaged users and the implementation of strategies to prevent dropout.
To date, there are only two systematic reviews that investigated the impact of sociodemographic predictors of dropout within digital interventions, however, one focused on psychological disorders (Melville et al., 2010), whereas the other focused on chronic pain (Macea et al., 2010). We were unable to find specific reviews or meta-analysis of predictors of dropout from digital interventions for various chronic health conditions. Thus, the rationale and recommendations for sociodemographic predictors of dropout (e.g., youth, males) below are based on these available reviews and studies.
7.1. Digital Interventions Should Be Particularly Careful When the Target Population Includes Youth Rationale. Studies of digital interventions for a variety of health-related behaviors (e.g., improving healthy diet and physical activity, diabetes self-management, smoking cessation) consistently report that younger users present with higher dropout rates whereas increasing age is associated with more adherence and usage as illustrated by number of logins (Kelders et al., 2011; Wangberg et al., 2008). Interestingly, however, youth utilizes digital technologies at greater rates (Buckingham, 2008). Possible explanations for lower youth adherence include that older participants may experience more health problems compared to youth (Japuntich et al., 2006). Additionally, youth who are more technologically savvy may find the quality or technological features of digital health interventions less interesting or challenging. However, numerous prevention and intervention programs (e.g., for smoking or healthy diet) particularly
aim to target youth at an early age, so it is important to consider how digital means may be utilized to attract and engage youth.
Recommendation. Researchers are encouraged to be vigilant (e.g., oversample) when recruiting youth in digital interventions. Prior to designing a digital intervention, researchers are also encouraged to perform focus groups with youth in order to obtain feedback or suggestions of design features and intervention structure. Personalization and tailored interventions to youths’ needs, including specific youth communication features, language, music, videos, and unique interests, may increase engagement and motivation. In addition, the inclusion of gaming elements within digital interventions might be of particular importance for young participants.
7.2. Digital Interventions Should Be Particularly Careful When the Target Population Includes Males Rationale. Similar to traditional interventions, female participants are more likely to adhere to Internet-based programs and less likely to drop out (Lange et al., 2003; Macea et al., 2010). Lange and colleagues (2003), for example, reported 71% of males compared to 19% of females dropped out of their digital intervention, a pattern repeatedly observed by others and for a variety of health-related conditions (Carpenter, Stoner, Mundt, & Stoelb, 2012). Explanations of this phenomenon may be that as in traditional interventions, more females as opposed to males seek help and psychological interventions may be more appealing to women than men.
Recommendation. During the design of a digital intervention, it is proposed that attempts are made to personalize and tailor the content to be applicable and engaging for male users as well as females. Suggestions include the provision of choices (e.g., choice of a male or female avatar within a digital program; see Savvides & Karekla, 2015), material that may fall within the particular interests of males, and inclusion of motivational interviewing elements or values clarification prior to the initiation of an intervention.
7.3. Digital Interventions Should Be Particularly Careful When the Target Population Includes Long-Standing and Severe Chronic Health Conditions and Particularly When Chronic Pain or Psychological Problems Are Involved Rationale. Many chronic health conditions involve chronic pain (e.g., fibromyalgia, rheumatoid arthritis, chronic low back pain, chronic migraines, peripheral neuropathy, cancer pain, diabetic pain). Macea and colleagues (2010) in their meta-analysis showed that longer duration of chronic pain might significantly increase the likelihood of users dropping out of digital intervention. DiMatteo, Haskard, and
Williams (2007) in their meta-analysis reported similar associations between chronicity of health conditions and nonadherence and both objective and subjective severity of health condition and dropout (DiMatteo et al., 2007). Users with longer duration and greater severity of chronic health condition may have an array of unsuccessful condition management or treatment attempts, which may result in lowered motivation to engage in new approaches (Horne & Weinman, 1999). Further, as severity of condition increases, expectations and interactions with health providers reduce in quality, making the more severely ill more prone to drop out (Hall, Roter, Milburn, & Daltroy, 1996; Kravitz et al., 2002). Additionally, usually individuals with long-standing chronic health conditions especially those involving pain may become overly dependent on substance use (e.g., analgesics, opioids, alcohol) to manage their pain (Fishbain, Rosomoff, & Rosomoff, 1992; Hoffmann, Olofsson, Salen, & Wickstrom, 1995). Users with comorbid conditions are more likely to drop out and may also be more hesitant to utilize psychologically based methods (Corsonello et al., 2010). Moreover, poor adherence in patients with chronic health conditions may be mediated by feelings of pessimism or depression, social withdrawal, and hopelessness about survival (Carney et al., 1995; Christensen, Benotsch, & Smith, 1997). Recommendation. Any digital intervention should include a thorough assessment of previous experiences with psychological interventions, previous unsuccessful attempts, motivation to engage in the digital intervention, and screen for substance use disorders or overmedication. Given that chronic users may have extensive experiences with traditional therapy modalities, any benefits of digital interventions (e.g., convenience of time and space, anonymity, minimization of dependency on others to drive them to treatment, self-management solutions) should be highlighted. Limitations of digital interventions should also be explicated from the beginning so as to reduce the likelihood of disappointment and de-motivation.
7.4. Digital Interventions Should Examine and Involve Where Possible the Social Environment of the User Rationale. The quality of an individual’s social environment (e.g., quality of the relationship with a significant other) may influence adherence in a digital intervention (Melville et al., 2010). Supportive partners who motivate the continued involvement of the users may lead to a lower risk of dropout. However, partners in addition to being a source of social support may actually be a potential source of stress (e.g., communication problems may result; Reid, Eccleston, & Pillemer, 2015) or even treatment saboteurs (most of the time without consciously realizing this) in a patient’s attempts for change (Kavanagh, 1992). For example, when
spouses of patients with diabetes conveyed disregard with the diabetics’ diet, the result was worse dietary adherence (Henry, Rook, Stephens, & Franks, 2013). Recommendation. Digital interventions may include an assessment of perceived social support and of the relationship of the user with significant others or caretakers. Significant others may also be asked to become involved and have their attitudes toward the intervention assessed prior to commencing the intervention. Though matching and tailoring partner responses may be tricky for digital interventions and may increase chances of nonadherence when two people are involved, it can be accomplished via a variety of ways: (1) in the form of developing a dual platform where caregivers or significant others receive in parallel support, psychoeducation or online counseling about the impact that their support is having on the user, and management of their attitudes toward treatment; (2) providing for example additional relationship and communication with significant other modules for individuals who present with deficiencies in their social interactions; (3) positively reinforcing successful interactions and reduced negative expressed emotion.
8. Digital Interventions Should Include an Assessment of Computer Knowledge and Experience and Provide Easy Tutorials and Technical Assistance Rationale. One of the most common reasons for users dropping out of digital interventions involves computer illiteracy or lack of sufficient computer knowledge or experience (Carlbring et al., 2001; Kenwright, Marks, Gega, & Mataix-Cols, 2004; Lange et al., 2003). Common technical problems may occur when interacting with digital means, such as with Internet connection, slow loading of a Website, security concerns (Kuijpers, Groen, Aaronson, & Harten, 2013), or incompatibility issues (e.g., pre or post a software update). If technical difficulties occur and the user lacks the knowledge to respond to them, it is more likely that they will feel disengaged and discontinue. Recommendation. Digital interventions should first assess their users’ levels of computing literacy and experience. Second, developers should aim for use simplicity. This will increase the possibility for noncomputer literate individuals to be able to interact with the delivery medium (especially in cases where the target population is expected to have minimal interaction with digital means). Technical assistance should be provided as quickly and efficiently as possible. The ideal would be to have a 24-hour online technical support; however, we acknowledge that this may be impossible for most programs. Digital interventions should be designed for usage in a broad array of operating systems (e.g., Microsoft Windows, Mac OS) and platforms (e.g., computers, tablets, smartphones). Short video tutorials on ways to use the intervention, seek support, or solve
common technical issues should be readily available and presented to the users before the intervention.
Dimension IV: Active Assessment of Usage 9. Instructions for Use Should Be Simple and Direct Rationale. According to Kelders and colleagues (2012), one of the most significant contributors to adherence in digital interventions is frequent intended usage. Frequent intended usage implies that users are provided with clear instructions and expectations about their active interaction with the intervention.
Recommendation. Users should be informed about intended usage prior to the intervention and be provided with clear expectations and simple instructions for use. According to the model of supportive accountability, the user should be involved in the definitions of goals and expectations. Adequate justification of expectations (e.g., explanation about why the user is asked to interact with a digital intervention daily) should be followed by a mutual agreement, framed in terms of the benefits to the user (Mohr et al., 2011).
10. Digital Interventions may Utilize Web-Metrics to Assess and Monitor Adherence of Disengaged Users Rationale. Digital interventions need reliable and objective means of assessing adherence and engagement, while being sensitive to maintain user anonymity. A problem of assessing adherence across different digital intervention studies involves that variable means of assessment are used without uniformity across studies. Yardley and colleagues (2016) describe the value and considerations for using different methods of assessing engagement in digital interventions (e.g., self-report questionnaires, ecological momentary assessment, qualitative analysis of self-report data, smartphone sensors, logs of system usage data, and physiological measures); however, it is also important to establish specific assessment means so as to enable comparisons between studies.
Digital interventions have an advantage over face-to-face interventions for assessing adherence, in that Web-metrics, which are objective measures of usage are readily available via the digital medium (Donkin et al., 2011). The availability of Web-metrics (e.g., number of logins, activities and modules completed, time spent online, and pages opened) is a particular advantage of digital interventions, as traditional interventions usually have to rely on subjective means of retrospective self-report amenable to recall bias for information about adherence. However, despite the relative availability of Web-metrics, very few studies actually report Web-metric statistics, while some even continue to use selfreport for assessing adherence (Brouwer et al., 2011). In addition, Web-metrics can be used as an additional tool to other modes of assessment such as questionnaires
and qualitative analysis of self-report, ecological momentary assessment, physiological measures, for accurate monitoring and tracking of users and thus efficiently and immediately intervene to reengage a user if needed. Christensen, Griffiths, Korten, Brittliffe, and Groves (2004) found that weekly tracking followed by reminders significantly reduced attrition in a cognitive behavioral therapy (CBT) intervention for depression. In addition, awareness of having ones performance or adherence monitored is found to increase accountability toward the intervention (Mohr et al., 2011), which leads to increased engagement. However, if adherence monitoring is perceived as surveillance or as controlling without explicit rational, it may reduce compliance and contribute to demoralization (Lerner & Tetlock, 1999) and damage user adherence (Checkland, Marshall, & Harrison, 2004; Enzle & Anderson, 1993). It is important to consider that disengagement from an intervention may mean a number of things (Yardley et al., 2016). For example, discontinued engagement may be due to successful behavior change or positive health outcomes mirroring sufficient mastery to the extent that the digital intervention is no longer needed. On the other hand, it may result from unsuccessful change/poor outcome, the user being bored with the specific intervention or facing technical issues with the device. High engagement and improved outcomes may be both due to individual characteristics (e.g., higher commitment to the procedures/greater motivation), or they may be observed in a study setting and not real life (because someone is monitored and expected to engage), or it may mean that someone struggles and requires more support. Given the range of reasons that may affect user engagement, it is important to utilize various methods of assessment and especially the easily available Web-metrics to be able to better clarify and understand the phenomenon of engagement in its different contexts.
Recommendation. Firstly, we encourage the use of all metrics available when assessing for adherence. Additionally as proposed by Donkin and colleagues (2011), measures of inactivity or idle time may also be useful. A time-out m essage in the form of a positive prompt can be included within the digital intervention for users who appear to be unresponsive for some time. Reporting of adherence (e.g., via Web-metrics) should be included in publications of findings.
Secondly, use of Web-metrics may be a useful way to identify the stage where a user begins to feel disengaged. Computer software programs (e.g., MySQL or Oracle Database 12c) detect a variety of metrics such as users’ registration, frequency of visits, time spent online or at specific modules, and material downloaded. Users, who are observed through metrics to not adhere to the intervention
as intended, could be personally contacted (via text, e-mail, or phone) with tailored feedback according to their needs, aiming to motivate reengagement. For instance, if a user appears disengaged, yet has expressed as part of the intervention that spending time with family is important to him/ her, a message reminding the user of their stated value and goals may motivate and reengage the user. Monitoring of engagement may also be helpful in cases where users are found to be at higher risk for dropout (e.g., present with sociodemographic parameters that place them at risk as discussed previously).
Prior to monitoring and contacting a disengaged user, researchers are encouraged to provide an adequate explanation to the user (e.g., in the consent form) about when they may be contacted and how this will be done. Clarifying to the user that the aim of monitoring is to provide feedback and indications of disengagement so as to motivate and mobilize the user to reengage and achieve stated goals. It is important to ensure that all this is done in junction with the ethical considerations presented previously and clarifying to the individual that there will be no negative consequences, implications, or penalties if they choose to discontinue the intervention.
Discussion
Digital interventions for chronic health conditions have bloomed over the past few years, with the advent of technology and the widespread accessibility of digital means. Yet research on digital interventions and particularly on issues related to engagement and adherence of users remains in its infancy. This paper provided an overview of user engagement factors and proposed research informed and consensual recommendations (from two EFPA committees: Psychology and Health Standing Committee and e-Health Task Force) for engagement and adherence planning in digital intervention development. A four-dimensional set of 10 recommendations consisting of: (1) a-priori theoretical planning, (2) human–computer interaction factors, (3) tailoring and targeting to use groups, and (4) active assessment of usage were proposed, with supporting evidence and suggested recommendations. A combination of a-priori theoretical planning in terms of psychological approaches and designing of the intervention while maintaining ethical principles in the field of health is of paramount importance. This paper further argued that user characteristics (such as age and gender) in addition to computer literacy of users should be considered when planning a digital intervention and ways to do this were proposed. Finally, active monitoring using Web-metrics and intervening to reengage users early was suggested so as to significantly boost adherence rates.
Researchers and developers are strongly encouraged to be mindful of best practices and recommendations in respect to digital interventions and work as a team of professionals to achieve these. Specialists working in groups could put forward and be responsible for recommendations mostly tied to their specialty field. For example, the clinician or researcher could bring forth knowledge regarding theory-driven evidence-based psychological intervention content whereas the developer could propose empirically supported technological characteristics. However, recommendations such as maintaining ethical standards should be overarching across the whole digital intervention development and application process.
Future systematic reviews of adherence in digital interventions for chronic health conditions should include an examination of a combination of user characteristics and human–computer interaction factors, including mediators and moderators of change, so as to more clearly elucidate parameters affecting adherence to digital interventions. We hope that the proposed recommendations will be adapted and examined by future research so as to investigate their impact on user engagement and adherence for chronic health conditions, but also for other chronic mental health difficulties and even acute conditions and health behavior change. This paper adopted a general definition of digital interventions to include a range of approaches from self-monitoring apps to Internet-based interventions to simple drug intake reminders. Again future research should examine whether the proposed recommendations are equally relevant and significant for all interventions modalities included under the digital intervention umbrella.
Overall, we strongly urge researchers to use a holistic apriori theoretical approach and planning and to consider the recommendations from all four proposed dimensions when designing a digital intervention. We hope that these recommendations will serve as a reference point to inform researchers and developers on how to maximize user engagement, which in turn will lead to greater adherence, lower attrition rates, optimized exposure to the intervention, and improve effectiveness in the treatment of chronic health conditions via digital interventions.
Electronic Supplementary Materials
The electronic supplementary material is available with the online version of the article at https://doi.org/10.1027/ 1016-9040/a000349
ESM 1. Table (.pdf) Engagement and adherence to digital interventions: challenges, recommendations, and examples.
References
Abraham, C., & Michie, S. (2008). A taxonomy of behavior change techniques used in interventions. Health Psychology, 27, 379–387. https://doi.org/10.1037/0278-6133.27.3.379 Adams, J. S. (1965). Inequity in social exchange. Advances in Experimental Social Psychology, 2, 267–299. https://doi.org/ 10.1016/S0065-2601(08)60108-2 Andersson, G., Carlbring, P., Berger, T., Almlöv, J., & Cuijpers, P. (2009). What makes Internet therapy work? Cognitive Behaviour Therapy, 38(S1), 55–60. https://doi.org/10.1080/ 16506070902916400 Arora, S., Yttri, J., & Nilsen, W. (2014). Privacy and security in Mobile Health (mHealth) Research. Alcohol Research: Current Reviews, 36, 143–151. Bandura, A. (1982). Self-efficacy mechanism in human agency. American Psychologist, 37, 122–147. https://doi.org/10.1037/ 0003-066X.37.2.122 Barak, A., Klein, B., & Proudfoot, J. G. (2009). Defining Internetsupported therapeutic interventions. Annals of Behavioral Medicine, 38, 4–17. https://doi.org/10.1007/s12160-009- 9130-7 Baumeister, H., Reichler, L., Munzinger, M., & Lin, J. (2014). The impact of guidance on Internet-based mental health interventions –A systematic review. Internet Interventions, 1 , 205–215. https://doi.org/10.1016/j.invent.2014.08.003 Bender, J. L., Radhakrishnan, A., Diorio, C., Englesakis, M., & Jadad, A. R. (2011). Can pain be managed through the Internet? A systematic review of randomized controlled trials. Pain, 152, 1740–1750. https://doi.org/10.1016/j.pain.2011.02.012 Bennett, G. G., & Glasgow, R. E. (2009). The delivery of public health interventions via the Internet: actualizing their potential. Annual Review of Public Health, 30, 273–292. https://doi.org/ 10.1146/annurev.publhealth.031308.100235 Black, A. D., Car, J., Pagliari, C., Anandan, C., Cresswell, K., Bokun, T., ... Sheikh, A. (2011). The impact of eHealth on the quality and safety of health care: A systematic overview. PLoS Medicine, 8, e1000387. https://doi.org/10.1371/journal. pmed.1000387 Breivik, H., Collett, B., Ventafridda, V., Cohen, R., & Gallacher, D. (2006). Survey of chronic pain in Europe: Prevalence, impact on daily life, and treatment. European Journal of Pain, 10, 287–333. https://doi.org/10.1016/j.ejpain.2005.06.009 Brouwer, W., Kroeze, W., Crutzen, R., de Nooijer, J., de Vries, N. K., Brug, J., & Oenema, A. (2011). Which intervention characteristics are related to more exposure to Internet-delivered healthy lifestyle promotion interventions? A systematic review. Journal of Medical Internet Research, 13, e2. https://doi.org/10.2196/ jmir.1639 Brouwer, W., Oenema, A., Raat, H., Crutzen, R., de Nooijer, J., de Vries, N. K., & Brug, J. (2010). Characteristics of visitors and revisitors to an Internet-delivered computer-tailored lifestyle intervention implemented for use by the general public. Health Education Research, 25, 585–595. https://doi.org/10.1093/ her/cyp063 Brown, M., O’Neill, N., van Woerden, H., Eslambolchilar, P., Jones, M., & John, A. (2016). Gamification and adherence to Webbased mental health interventions: A systematic review. JMIR Mental Health, 3, e39. https://doi.org/10.2196/mental.5710 Brug, J., Oenema, A., & Campbell, M. (2003). Past, present, and future of computer-tailored nutrition education. The American Journal of Clinical Nutrition, 77, 1028S–1034S. https://doi.org/ 10.1093/ajcn/77.4.1028S Brug, J., Oenema, A., Kroeze, W., & Raat, H. (2005). The Internet and nutrition education: Challenges and opportunities.
European Psychologist (2019), 24(1), 49–67 European Journal of Clinical Nutrition, 59, S130–S139. https:// doi.org/10.1038/sj.ejcn.1602186 Buckingham D. (Ed.). (2008). Youth, identity, and digital media. Cambridge, MA: MIT Press. Carlbring, P., Westling, B. E., Ljungstrand, P., Ekselius, L., & Andersson, G. (2001). Treatment of panic disorder via the Internet: A randomized trial of a self-help program. Behavior Therapy, 32, 751 –764. https://doi.org/10.1016/S0005-7894(01) 80019-8 Carney, R. M., Freedland, K. E., Kenneth, E., Eisen, S. A., Rich, M. W., & Jaffe, A. S. (1995). Major depression and medication adherence in elderly patients with coronary artery disease. Health Psychology, 14, 88–90. https://doi.org/10.1037/0278- 6133.14.1.88 Carpenter, K. M., Stoner, S. A., Mundt, J. M., & Stoelb, B. (2012). An online self-help CBT intervention for chronic lower back pain. The Clinical Journal of Pain, 28, 14–22. https://doi.org/ 10.1097%2FAJP.0b013e31822363db Checkland, K., Marshall, M., & Harrison, S. (2004). Re-thinking accountability: Trust versus confidence in medical practice. Quality and Safety in Health Care, 13, 130–135. https://doi.org/ 10.1136/qshc.2003.009720 Christensen, A. J., Benotsch, E. G., & Smith, T. W. (1997). Determinants of regimen adherence in renal dialysis. In D. S. Gochman (Ed.), Handbook of health behavior research II (pp. 231 –244). Boston, MA: Springer. https://doi.org/10.1007/978- 1-4899-1760-7_12 Christensen, H., Griffiths, K. M., & Farrer, L. (2009). Adherence in Internet interventions for anxiety and depression: Systematic review. Journal of Medical Internet Research, 11 , e13. https:// doi.org/10.2196/jmir.1194 Christensen, H., Griffiths, K. M., & Korten, A. (2002). Web-based cognitive behavior therapy: Analysis of site usage and changes in depression and anxiety scores. Journal of Medical Internet Research, 4, e3. https://doi.org/10.2196/jmir.4.1.e3 Christensen, H., Griffiths, K. M., Korten, A. E., Brittliffe, K., & Groves, C. (2004). A comparison of changes in anxiety and depression symptoms of spontaneous users and trial participants of a cognitive behavior therapy website. Journal of Medical Internet Research, 6, e46. https://doi.org/10.2196/ jmir.6.4.e46 Cobb, N. K., & Poirier, J. (2014). Effectiveness of a multimodal online well-being intervention: A randomized controlled trial. American Journal of Preventive Medicine, 46, 41 –48. https:// doi.org/10.1016/j.amepre.2013.08.018 Corsonello, A., Pedone, C., Garasto, S., Maiuri, G., Carelli, A., Zottola, C., & Lattanzio, F. (2010). The impact of comorbidity on medication adherence and therapeutic goals. BMC Geriatrics, 10(Suppl 1), L21. https://doi.org/10.1186/1471-2318-10-S1-L21 Couper, M. P., Alexander, G. L., Zhang, N., Little, R. J. A., Maddy, N., Nowak, M. A., ... Johnson, C. C. (2010). Engagement and retention: Measuring breadth and depth of participant use of an online intervention. Journal of Medical Internet Research, 12 , e52. https://doi.org/10.2196/jmir.1430 Cugelman, B. (2013). Gamification: What it is and why it matters to digital health behavior change developers. JMIR Serious Games, 1 , e3. https://doi.org/10.2196/games.3139 Cuijpers, P., Van Straten, A., & Andersson, G. (2008). Internetadministered cognitive behavior therapy for health problems: A systematic review. Journal of Behavioral Medicine, 31 , 169–177. https://doi.org/10.1007/s10865-007-9144-1 De Nooijer, J., Oenema, A., Kloek, G., Brug, H., De Vries, H., & De Vries, N. (2005). Bevordering van gezond gedrag via het Internet: nu en in de toekomst [Promotion of healthy behavior through the Internet: Now and in the future] Retrieved from https:// maastrichtuniversity.nl/portal/en/publications/bevordering
van-gezond-gedrag-via-internet-nu-en-in-de-toekomst (1d99b191 –a6b8–4895-8e08–7eefefbcb9b3).html. Maastricht, The Netherlands: Universiteit Maastricht. Dennison, L., Morrison, L., Conway, G., & Yardley, L. (2013). Opportunities and challenges for smartphone applications in supporting health behavior change: Qualitative study. Journal of medical Internet research, 15, e86. https://doi.org/10.2196/ jmir.2583 Deterding, S., Dixon, D., Khaled, R., & Nacke, L. (2011). From game design elements to gamefulness: Defining gamification. In ACM. (Eds.), Proceedings of the 15th International Academic MindTrek Conference: Envisioning future media environments (pp. 9–15) https://doi.org/10.1145/2181037.2181040. New York, NY: ACM DiMatteo, M. R., Haskard, K. B., & Williams, S. L. (2007). Health beliefs, disease severity, and patient adherence: A metaanalysis. Medical Care, 45, 521 –528. https://doi.org/10.1097/ MLR.0b013e318032937e Donkin, L., Christensen, H., Naismith, S. L., Neal, B., Hickie, I. B., & Glozier, N. (2011). A systematic review of the impact of adherence on the effectiveness of e-therapies. Journal of medical Internet research, 13, e52. https://doi.org/10.2196/ jmir.1772 Eccleston, C., Fisher, E., Craig, L., Duggan, G. B., Rosser, B. A., & Keogh, E. (2014). Psychological therapies (Internet-delivered) for the management of chronic pain in adults. The Cochrane Library, 2, CD010152. https://doi.org/10.1002/14651858. CD010152.pub2 Enzle, M. E., & Anderson, S. C. (1993). Surveillant intentions and intrinsic motivation. Journal of Personality and Social Psychology, 64, 257–266. https://doi.org/10.1037/0022-3514. 64.2.257 European Group on Ethics in Science and New Technologies to the European Commission, & European Commission. (2012). Ethics of information and communication technologies. Publications Office of the European Union. Eysenbach, G. (2005). The law of attrition. Journal of Medical Internet Research, 7, e11. https://doi.org/10.2196/jmir.7.1.e11 Fairburn, C. G., & Patel, V. (2017). The impact of digital technology on psychological treatments and their dissemination. Behaviour Research and Therapy, 88, 19–25. https://doi.org/ 10.1016/j.brat.2016.08.012 Fishbain, D. A., Rosomoff, H. L., & Rosomoff, R. S. (1992). Drug abuse, dependence, and addiction in chronic pain patients. The Clinical Journal of Pain, 8, 77–85. https://doi.org/10.1097/ 00002508-199206000-00003 Fogg, B. J. (1997). Charismatic computers: Creating more likable and persuasive interactive technologies by leveraging principles from social psychology PhD Thesis. Stanford, CA: Stanford University Fogg, B. J. (1998). Persuasive computers: Perspectives and research directions. In ACM. (Eds.), Proceedings of the SIGCHI conference on Human factors in computing systems (pp. 225–232). New York, NY: ACM Press/Addison-Wesley. https:// doi.org/10.1145/274644.274677 Fogg, B. J. (2002). Persuasive technology: Using computers to change what we think and do. Ubiquity, 2002, 5. https://doi.org/ 10.1145/764008.763957 Geissler, G. L., Zinkhan, G. M., & Watson, R. T. (2006). The influence of home page complexity on consumer attention, attitudes, and purchase intent. Journal of Advertising, 35, 69–80. https://doi.org/10.1080/00913367.2006.10639232 Glasgow, R. E. (2007). eHealth evaluation and dissemination research. American Journal of Preventive Medicine, 32, S119–S126. https://doi.org/10.1016/j.amepre.2007.01.023 63
Haas, L. J., & Malouf, J. L. (2002). Keeping up the good work: A practitioner’s guide to mental health ethics (2nd ed.). Sarasota, FL: Professional Resource Press. https://doi.org/10.1207/ s15327019eb0201_5 Hall, J. A., Roter, D. L., Milburn, M. A., & Daltroy, L. H. (1996). Patients’ health as a predictor of physician and patient behavior in medical visits: A synthesis of four studies. Medical Care, 34, 1205–1218. Hayes, S. C., Strosahl, K. D., & Wilson, K. G. (1999). Acceptance and commitment therapy: An experiential approach to behavior change. New York, NY: Guilford Press. Henry, S. L., Rook, K. S., Stephens, M. A., & Franks, M. M. (2013). Spousal undermining of older diabetic patients’ disease management. Journal of Health Psychology, 18, 1550–1561. https:// doi.org/10.1177/1359105312465913 Hilvert-Bruce, Z., Rossouw, P. J., Wong, N., Sunderland, M., & Andrews, G. (2012). Adherence as a determinant of effectiveness of Internet cognitive behavioural therapy for anxiety and depressive disorders. Behaviour Research and Therapy, 50, 463–468. https://doi.org/10.1016/j.brat.2012.04.001 Hoffmann, N. G., Olofsson, O., Salen, B., & Wickstrom, L. (1995). Prevalence of abuse and dependency in chronic pain patients. International Journal of the Addictions, 30, 919–927. https:// doi.org/10.3109/10826089509055820 Hong, T. (2006). Contributing factors to the use of health-related websites. Journal of Health Communication, 11 , 149–165. https://doi.org/10.1080/10810730500526679 Horne, R., & Weinman, J. (1999). Patients’ beliefs about prescribed medicines and their role in adherence to treatment in chronic physical illness. Journal of Psychosomatic Research, 47, 555–567. https://doi.org/10.1016/S0022-3999(99)00057-4 Hsiung, R. (2001). PSI Suggested principles of professional ethics for the online provision of mental health services. Telemedicine Journal and E-health, 7, 39–45. https://doi.org/10.1089/ 153056201300093895 Imamura, K., Kawakami, N., Furukawa, T. A., Matsuyama, Y., Shimazu, A., Umanodan, R., ... Kasai, K. (2014). Effects of an Internet-based Cognitive Behavioral Therapy (iCBT) program in Manga format on improving subthreshold depressive symptoms among healthy workers: A randomized controlled trial. PLoS One, 9, e97167. https://doi.org/10.1371/journal. pone.0097167 International Society for Mental Health Online. (2000). ISMHO/PSI suggested principles for the online provision of mental health services. Retrieved from http://www.ismho.org/suggestions. html Irvine, A. B., Gelatt, V. A., Seeley, J. R., Macfarlane, P., & Gau, J. M. (2013). Web-based intervention to promote physical activity by sedentary older adults: Randomized controlled trial. Journal of Medical Internet Research, 15, e19. https://doi.org/10.2196/ jmir.2158 Jamison, R. N., Gintner, L., Rogers, J. F., & Fairchild, D. G. (2002). Disease management for chronic pain: Barriers of program implementation with primary care physicians. Pain Medicine, 3, 92–101. https://doi.org/10.1046/j.1526-4637.2002.02022.x Japuntich, S. J., Zehner, M. E., Smith, S. S., Jorenby, D. E., Valdez, J. A., Fiore, M. C., ... Gustafson, D. H. (2006). Smoking cessation via the Internet: A randomized clinical trial of an Internet intervention as adjuvant treatment in a smoking cessation intervention. Nicotine & Tobacco Research, 8(Suppl 1), S59–S67. https://doi.org/10.1080/14622200601047900 Jasper, K., Weise, C., Conrad, I., Andersson, G., Hiller, W., & Kleinstäuber, M. (2014). The working alliance in a randomized controlled trial comparing Internet-based self-help and faceto-face cognitive behavior therapy for chronic tinnitus. Internet
Interventions, 1 , 49–57. https://doi.org/10.1016/j.invent.2014. 04.002 Jensen, M. P., Nielson, W. R., Romano, J. M., Hill, M. L., & Turner, J. A. (2000). Further evaluation of the pain stages of change questionnaire: Is the transtheoretical model of change useful for patients with chronic pain? Pain, 86, 255–264. https://doi. org/10.1016/S0304-3959(00)00257-8 Jerant, A. F., von Friederichs-Fitzwater, M. M., & Moore, M. (2005). Patients’ perceived barriers to active self-management of chronic conditions. Patient Education and Counseling, 57, 300–307. https://doi.org/10.1016/j.pec.2004.08.004 Johansson, R., & Andersson, G. (2012). Internet-based psychological treatments for depression. Expert Review of Neurotherapeutics, 12, 861. https://doi.org/10.1586/ern.12.63 Karekla, M. (2017). ACT-based digital applications for health behavior change: #FlexiQuit #AcceptMe#AlgeaApp. Invited address at the Association of Contextual Behavior Science Annual World Conference, Seville, Spain. Karekla, M., Karademas, E., Vasiliou, V., Kasinopoulos, O., Flouri, M., Christou, G., & Papacostas, S. (2017). In search for novel and more effective psychological treatments for chronic pain: The “Algea” Research Project. European Health Psychologist, 19, 297–301. Karyotaki, E., Kleiboer, A., Smit, F., Turner, D. T., Pastor, A. M., Andersson, G., ... Christensen, H. (2015). Predictors of treatment dropout in self-guided web-based interventions for depression: An “individual patient data” meta-analysis. Psychological Medicine, 45, 2717–2726. https://doi.org/10.1017/ S0033291715000665 Kavanagh, D. J. (1992). Recent developments in expressed emotion and schizophrenia. The British Journal of Psychiatry, 160, 601 –620. https://doi.org/10.1192/bjp.160.5.601 Kelders, S. M., Kok, R. N., Ossebaard, H. C., & Van Gemert-Pijnen, J. E. (2012). Persuasive system design does matter: A systematic review of adherence to web-based interventions. Journal of Medical Internet Research, 14, e152. https://doi.org/10.2196/ jmir.2104 Kelders, S. M., Van Gemert-Pijnen, J. E., Werkman, A., Nijland, N., & Seydel, E. R. (2011). Effectiveness of a web-based intervention aimed at healthy dietary and physical activity behavior: A randomized controlled trial about users and usage. Journal of Medical Internet Research, 13, e32. https://doi.org/10.2196/ jmir.1624 Kenwright, M., Marks, I. M., Gega, L., & Mataix-Cols, D. (2004). Computer-aided self-help for phobia/panic via Internet at home: A pilot study. The British Journal of Psychiatry, 184, 448–449. https://doi.org/10.1192/bjp.184.5.448 Keogh, E. (2013). Developments in the use of e-health for chronic pain management. Pain Management, 3, 27–33. https://doi. org/10.2217/pmt.12.70 Keogh, E., Rosser, B. A., & Eccleston, C. (2010). eHealth and chronic pain management: Current status and developments. Pain, 151 , 18–21. https://doi.org/10.2217/pmt.12.70 Konow, J. (2000). Fair shares: Accountability and cognitive dissonance in allocation decisions. The American Economic Review, 90, 1072–1091. https://doi.org/10.1257/aer.90.4.1072 Koocher, G. P., & Keith-Spiegel, P. (1998). Ethics in psychology: Professional standards and cases (2nd ed.). New York, NY: Oxford University Press. Kravitz, R. L., Bell, R. A., Azari, R., Krupat, E., Kelly-Reif, S., & Thom, D. (2002). Request fulfillment in office practice: Antecedents and relationship to outcomes. Medical Care, 40, 38–51. Kuijpers, W., Groen, W. G., Aaronson, N. K., & Harten, W. V. (2013). A systematic review of web-based interventions for patient empowerment and physical activity in chronic diseases: Relevance for cancer survivors. Journal of Medical Internet Research, 15, e37. https://doi.org/10.2196/jmir.2281 Lange, A., Van De Ven, J. P., & Schrieken, B. (2003). Interapy: Treatment of post-traumatic stress via the Internet. Cognitive Behaviour Therapy, 32, 110–124. https://doi.org/10.1080/ 16506070302317 Lerner, J. S., & Tetlock, P. E. (1999). Accounting for the effects of accountability. Psychological Bulletin, 125, 255–275. https:// doi.org/10.1037/0033-2909.125.2.255 Leslie, E., Marshall, A. L., Owen, N., & Bauman, A. (2005). Engagement and retention of participants in a physical activity website. Preventive Medicine, 40, 54–59. https://doi.org/ 10.1016/j.ypmed.2004.05.002 Long, A. C., & Palermo, T. M. (2009). Brief report: Web-based management of adolescent chronic pain: Development and usability testing of an online family cognitive behavioral therapy program. Journal of Pediatric Psychology, 34, 511 –516. https:// doi.org/10.1093/jpepsy/jsn082 Liu, S., Hodgson, C., Zbib, A. M., Payne, A. Y., & Nolan, R. P. (2014). The effectiveness of loyalty rewards to promote the use of an Internet-based heart health program. Journal of Medical Internet Research, 16, e163. https://doi.org/10.2196/jmir.3458 Lorig, K. R., Laurent, D. D., Deyo, R. A., Marnell, M. E., Minor, M. A., & Ritter, P. L. (2002). Can a Back Pain E-mail Discussion Group improve health status and lower health care costs? A randomized study. Archives of Internal Medicine, 162, 792–796. https://doi.org/10.1001/archinte.162.7.792 Lorig, K. R., Ritter, P. L., Laurent, D. D., & Plant, K. (2006). Internet-based chronic disease self-management: A randomized trial. Medical Care, 44, 964–971. https://doi.org/10.1097/ 01.mlr.0000233678.80203.c1 Macea, D. D., Gajos, K., Calil, Y. A. D., & Fregni, F. (2010). The efficacy of web-based cognitive behavioral interventions for chronic pain: A systematic review and meta-analysis. The Journal of Pain, 11 , 917–929. https://doi.org/10.1016/j. jpain.2010.06.005 Manwaring, J. L., Bryson, S. W., Goldschmidt, A. B., Winzelberg, A. J., Luce, K. H., Cunning, D., ... Taylor, C. B. (2008). Do adherence variables predict outcome in an online program for the prevention of eating disorders? Journal of Consulting and Clinical Psychology, 76, 341 –346. https://doi.org/10.1037/ 0022-006X.76.2.341 McGeary, D. D., McGeary, C. A., & Gatchel, R. J. (2012). A comprehensive review of telehealth for pain management: Where we are and the way ahead. Pain Practice, 12, 570–577. https://doi. org/10.1111/j.1533-2500.2012.00534.x Melville, K. M., Casey, L. M., & Kavanagh, D. J. (2010). Dropout from Internet-based treatment for psychological disorders. British Journal of Clinical Psychology, 49, 455–471. https://doi. org/10.1348/014466509X472138 Michie, S., Richardson, M., Johnston, M., Abraham, C., Francis, J., Hardeman, W., ... Wood, C. E. (2013). The behavior change technique taxonomy (v1) of 93 hierarchically clustered techniques: Building an international consensus for the reporting of behavior change interventions. Annals of Behavioral Medicine, 46, 81 –95. https://doi.org/10.1007/s12160-013-9486-6 Michie, S., Yardley, L., West, R., Patrick, K., & Greaves, F. (2017). Developing and evaluating digital interventions to promote behavior change in health and health care: Recommendations resulting from an international workshop. Journal of Medical Internet Research, 19, e232. https://doi.org/10.2196/jmir.7126 Mohl, P. C., Martinez, D., Ticknor, C., Huang, M., & Cordell, L. (1991). Early dropouts from psychotherapy. The Journal of Nervous and Mental Disease, 179, 478–481. https://doi.org/ 10.1097/00005053-199108000-00005
Mohr, D., Cuijpers, P., & Lehman, K. (2011). Supportive accountability: A model for providing human support to enhance adherence to eHealth interventions. Journal of Medical Internet Research, 13, e30. https://doi.org/10.2196/jmir.1602 Morrison, L. G. (2015). Theory-based strategies for enhancing the impact and usage of digital health behaviour change interventions: A review. Digital Health, 1 , 2055207615595335. https:// doi.org/10.1177/2055207615595335 Neff, R., & Fry, J. (2009). Periodic prompts and reminders in health promotion and health behavior interventions: Systematic review. Journal of Medical Internet Research, 11 , e16. https:// doi.org/10.2196/jmir.1138 O’Connor, S., Hanlon, P., O’Donnell, C. A., Garcia, S., Glanville, J., & Mair, F. S. (2016). Understanding factors affecting patient and public engagement and recruitment to digital health interventions: A systematic review of qualitative studies. BMC Medical Informatics and Decision Making, 16, 120. https://doi.org/ 10.1186/s12911-016-0359-3 Oinas-Kukkonen, H. (2013). A foundation for the study of behavior change support systems. Personal and Ubiquitous Computing, 17, 1223–1235. https://doi.org/10.1007/s00779-012-0591-5 Oinas-Kukkonen, H., & Harjumaa, M. (2009). Persuasive systems design: Key issues, process model, and system features. Communications of the Association for Information Systems, 24, 28. Retrieved from http://aisel.aisnet.org/cais/vol24/iss1/28 Palmqvist, B., Carlbring, P., & Andersson, G. (2007). Internetdelivered treatments with or without therapist input: Does the therapist factor have implications for efficacy and cost? Expert Review of Pharmacoeconomics & Outcomes Research, 7, 291 –297. https://doi.org/10.1586/14737167.7.3.291 Perski, O., Blandford, A., West, R., & Michie, S. (2017). Conceptualising engagement with digital behaviour change interventions: A systematic review using principles from critical interpretive synthesis. Translational Behavioral Medicine, 7, 254–267. https://doi.org/10.1007/s1314 Proudfoot, J. G., Parker, G. B., Pavlovic, D. H., Manicavasagar, V., Adler, E., & Whitton, A. E. (2010). Community attitudes to the appropriation of mobile phones for monitoring and managing depression, anxiety, and stress. Journal of Medical Internet Research, 12, e64. https://doi.org/10.2196/jmir.1475 Reeves, B., & Read, J. L. (2009). Total engagement. Using Games and Virtual Worlds to change the way people work and businesses compete. Computers in Human Behavior, 26, 1276–1277. Reid, M. C., Eccleston, C., & Pillemer, K. (2015). Management of chronic pain in older adults. British Medical Journal, 350, 1 –10. https://doi.org/10.1136/bmj.h532 Reynolds, G. S. (1968). A primer of operant conditioning. Glenview, IL: Scott Foresman. Sabaté, E. (2003). Adherence to long-term therapies: Evidence for action. Geneva, Switzerland: World Health Organization. https://doi.org/10.1016/S1474-5151(03)00091-4 Savvides, S. N., & Karekla, M. (2015). Evaluating an acceptance and commitment therapy Internet-based intervention for smoking cessation in young adults. European Health Psychologist, 17(S), 415. Schueller, S. M., Tomasino, K. N., & Mohr, D. C. (2016). Integrating human support into behavioral intervention technologies: The efficiency model of support. Clinical Psychology: Science and Practice, 24, 27–45. https://doi.org/10.1111/cpsp.12173 Shapiro, D. A., Cavanagh, K., & Lomas, H. (2003). Geographic inequity in the availability of cognitive behavioural therapy in England and Wales. Behaviour al and Cognitive Psychotherapy, 31 , 185–192. https://doi.org/10.1017/S1352465803002066 Society of Clinical Psychology, Division 12 of the American Psychological Association. (2011, March 21). Research-supported
2019 Hogrefe Publishing psychological therapies. Retrieved from http://www.div12. org/PsychologicalTreatments/treatments/chronicpain_act.html Sonderegger, A., & Sauer, J. (2010). The influence of design aesthetics in usability testing: Effects on user performance and perceived usability. Applied Ergonomics, 41 , 403–410. https:// doi.org/10.1016/j.apergo.2009.09.002 Sprangers, M. A., de Regt, E. B., Andries, F., van Agt, H. M., Bijl, R. V., de Boer, J. B., ... Miedema, H. S. (2000). Which chronic conditions are associated with better or poorer quality of life? Journal of Clinical Epidemiology, 53, 895–907. https://doi.org/ 10.1016/S0895-4356(00)00204-3 Titov, N., Dear, B. F., Johnston, L., Lorian, C., Zou, J., Wootton, B., ... Rapee, R. M. (2013). Improving adherence and clinical outcomes in self-guided Internet treatment for anxiety and depression: Randomised controlled trial. PLoS One, 8, e62873. https://doi. org/10.1371/journal.pone.0062873 Trompetter, H. R., Bohlmeijer, E. T., Veehof, M. M., & Schreurs, K. M. (2015). Internet-based guided self-help intervention for chronic pain based on Acceptance and Commitment Therapy: A randomized controlled trial. Journal of Behavioral Medicine, 38, 66–80. https://doi.org/10.1007/s10865-014-9579-0 van Ballegooijen, W., Cuijpers, P., van Straten, A., Karyotaki, E., Andersson, G., Smit, J. H., & Riper, H. (2014). Adherence to Internet-based and face-to-face cognitive behavioural therapy for depression: A meta-analysis. PLoS One, 9, e100674. https:// doi.org/10.1371/journal.pone.0100674 van Gemert-Pijnen, J. E., Nijland, N., van Limburg, M., Ossebaard, H. C., Kelders, S. M., Eysenbach, G., & Seydel, E. R. (2011). A holistic framework to improve the uptake and impact of eHealth technologies. Journal of Medical Internet Research, 13, e111. https://doi.org/10.2196/jmir.1672 Velleman, S., Stallard, P., & Richardson, T. (2010). A review and meta-analysis of computerized cognitive behaviour therapy for the treatment of pain in children and adolescents. Child: Care, Health and Development, 36, 465–472. https://doi.org/ 10.1111/j.1365-2214.2010.01088.x Voils, C. I., King, H. A., Maciejewski, M. L., Allen, K. D., Yancy, W. S., & Shaffer, J. A. (2014). Approaches for informing optimal dose of behavioral interventions. Annals of Behavioral Medicine, 48, 392–401. https://doi.org/10.1007/s12160-014-9618-7 Wang, J., Sereika, S. M., Chasens, E. R., Ewing, L. J., Matthews, J. T., & Burke, L. E. (2012). Effect of adherence to selfmonitoring of diet and physical activity on weight loss in a technology-supported behavioral intervention. Patient Preference and Adherence, 6, 221 –226. https://doi.org/10.2147/PPA. S28889 Wangberg, S. C., Bergmo, T. S., & Johnsen, J. A. (2008). Adherence in Internet-based interventions. Patient Preference and Adherence, 2, 57–65. Webb, T., Joseph, J., Yardley, L., & Michie, S. (2010). Using the Internet to promote health behavior change: A systematic review and meta-analysis of the impact of theoretical basis, use of behavior change techniques, and mode of delivery on efficacy. Journal of Medical Internet Research, 12, e4. https:// doi.org/10.2196/jmir.1376 Yardley, L., Spring, B. J., Riper, H., Morrison, L. G., Crane, D. H., Curtis, K., ... Blandford, A. (2016). Understanding and promoting effective engagement with digital behavior change interventions. America n Journal of Preventive Medicine, 51 , 833–842. https://doi.org/10.1016/j.amepre.2016.06.015 Zarski, A. C., Lehr, D., Berking, M., Riper, H., Cuijpers, P., & Ebert, D. D. (2016). Adherence to Internet-based mobile-supported stress management: A pooled analysis of individual participant data from three randomized controlled trials. Journal of Medical Internet Research, 18, e146. https://doi.org/10.2196/ jmir.4493
History Received May 2, 2017 Revision received February 22, 2018 Accepted April 19, 2018 Published online February 11, 2019
Acknowledgments This review has been prepared in association with the European Federation of Psychology Associations, Psychology and Health Standing Committee, and e-Health Task Force. The authors wish to acknowledge and thank the expert members of the two European Federation of Psychology Associations’ (EFPA) committees: Psychology and Health Standing Committee and e-Health Task Force. The authors state that each member association appoints the individual members in these committees as their local experts in the respective field.
Maria Karekla Department of Psychology University of Cyprus 2108 Nicosia Republic of Cyprus mkarekla@ucy.ac.cy
Maria Karekla (PhD) currently holds the position of Assistant Professor of Clinical Psychology at the University of Cyprus and is the chair of the Clinical Psychology Doctorate committee. Her research focuses on areas of health promotion and the investigation of individual difference factors (especially experiential avoidance) as they relate to the development and maintenance of various behavioral difficulties (especially anxiety and health-related problems). She is a member of the Cyprus Psychologist Licensing Board, the EFPA Psychology and Health and e-Health Task Forces.
Orestis Kasinopoulos (MSc) is a PhD student in the Clinical Psychology Doctorate program at the University of Cyprus, Department of Psychology. He is an external associate to the Spinal Cord Injury Rehabilitation Unit, Nicosia General Hospital, providing counseling and support services to physically disabled patients. In his PhD thesis, he has developed a digital ACT-based application, which aims to investigate the effectiveness in improving chronic pain management.
David Dias Neto (PhD) is a Lecturer at Instituto Superior de Psicologia Aplicada –Instituto Universitário. He has published in the areas of process research in psychotherapy and clinical and health psychology. He is the current president of the clinical and health psychology division of the Portuguese Order of Psychologists. He also works as a psychotherapist in private practice.
David Daniel Ebert (PhD, 2013) leads the E-Mental Health Unit and Behavioral Health Promotion & Technology (PROTECT) Laboratory at the Friedrich-Alexander-University Erlangen-Nuremberg. His work focuses on the prevention of mental health disorders in different settings (e.g., work, university), with a focus on the evaluation of Internet and mobilebased behavioral health technology interventions.
Tom Van Daele (PhD) is the head of the Expertise Unit Psychology, Technology & Society at Thomas More University of Applied Sciences (Antwerp, Belgium), a research fellow at KU Leuven (Leuven, Belgium) and the convenor of the EFPA TF on e-health. His research focuses on e-mental health, m-health, and their relevance to mental health care and (mental) health prevention and promotion.
Tine Nordgreen (PhD, 2011) has been actively involved in scientific publications, grant applications, supervision of students on a master and PhD level and held presentations on national and international conferences in the field of Internet-based treatment. She is currently the PI of a cross-disciplinary project (http://intromat.no/), including 14 partners, with the aim to develop new technology supported treatments for various settings.
Stefan Höfer (PhD) is Associate Professor of Psychology at the Innsbruck Medical University, Department of Medical Psychology, Austria. He is an internationally well-regarded expert in the field of patient-reported outcome assessment, psychometrics, and health psychology. He served from 2015–2017 as convenor of the EFPA standing committee of psychology and health.
Svein Oeverland is a Clinical Psychologist. He is a specialist in both child/adolescent psychology and forensic psychology. He is both the manager of the digital health company Superego and Head of the national forensic unit of mandatory care. He is the editor of the Journal Sexology of the Norwegian Union of Clinical and Scientific Sexology.
Kit Lisbeth Jensen is Psychologist and Clinical Psychologist and works in her private practice in Denmark. She has worked in the field of ehealth since 2010 and is member of several Danish committees. She has also been a member of the e-Health Task Force 2015–2017.