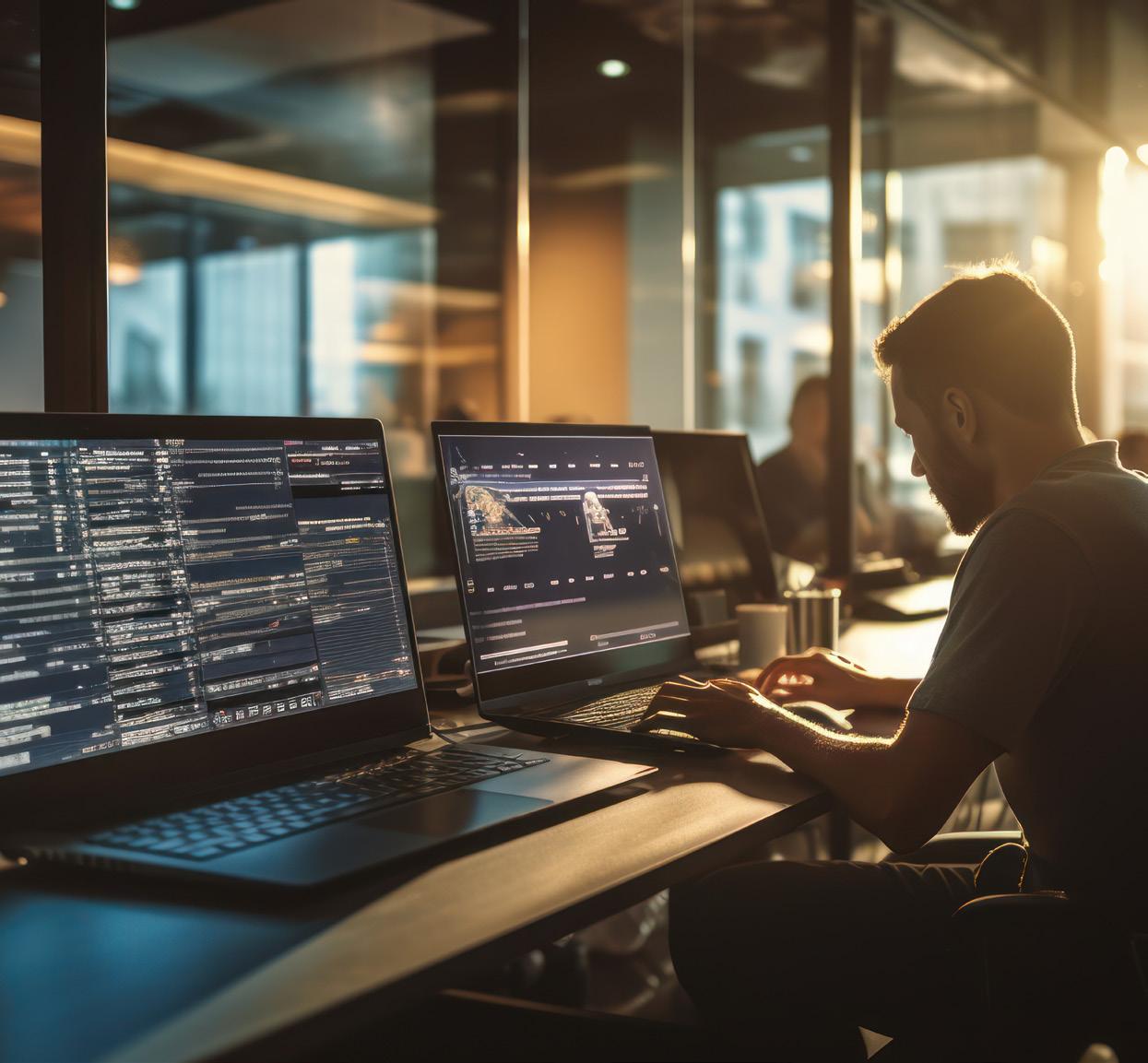
5 minute read
Artificial intelligence – taking the human out of HR?
Melika Soleimani and colleagues from Massey University summarise how artificial intelligence (AI) is currently used in HR and offer practical implications for its application.
Technological transformation promises hope in response to existential challenges such as climate change and population ageing, but also fears such as fewer and poorer quality jobs. One of today’s biggest controversies concerns AI. An AI system has a degree of autonomy based on machine learning (ML), whereby computers are trained with large datasets to evaluate new situations without recourse to further programming. Generative AI (such as ChatGPT or DALL-E) produces original text or images based on previous works, whereas predictive AI employs statistical analyses to make forecasts and, if set up as such, recommendations and even decisions.
The use of AI systems in business and management is now ubiquitous across functions such as marketing, finance, design, engineering, logistics and increasingly HRM. The principal HR applications are threefold:
recruitment and selection, where AI analyses textual and visual data to screen, interview and assess applicants.
algorithmic work management to direct workers (through task ordering and labour scheduling), evaluate workers (by performance monitoring and rating) and discipline workers (through rewards and penalties). This is widely deployed across sectors such as logistics, manufacturing, retail, hospitality and call centres. The shift to homeworking under COVID-19 also accelerated its deployment in white-collar occupations.
people analytics, whereby data are analysed to identify patterns and make predictions to inform decisions around, for example, training and development, turnover intentions and incentives.
These technologies can be used to improve objectivity as well as efficiency in decision-making. It is well known that individuals commonly rely on intuition and heuristics, especially in situations like recruitment and selection, where there is little direct information to rely on. Recruiters may also deploy cognitive biases, often unconsciously, that result in a ‘similar-to-me’ effect favouring some groups and individuals over others.
The more perspectives you have, the more diversity you have in building algorithms, the more representative it might be.
There are also worries about algorithmic bias. This can enter AI systems through the training data (based, say, on older white males) and the reductive nature of the algorithms. This means the application of AI in employment requires close supervision to investigate potential bias, as well as other errors and limitations, and reformulate algorithms accordingly.
To explore how well this is happening, we interviewed 22 senior HR managers and 17 AI developers about the use of AI in recruitment and selection. We found that AI was routinely used, but two sets of problems emerged. The first was communication difficulties between the groups because of educational, professional and demographic divergence. Most of the HR managers (all but five of whom were women) had limited technical competency and relied on the developers to articulate what could or should be done. Of the AI developers, all but two were male, and they were a much younger cohort with relatively little workplace experience, an average total of four years as opposed to fourteen for the HR professionals.
A second issue inhibiting organisational contextualisation was the shortage of HR datasets, especially from Aotearoa, for training and developing ML models. To some extent, this can be mitigated by techniques such as data augmentation or aggregation, or the use of ‘synthetic data’ to expand dataset size. However, these creative solutions also require greater effort and cost. Instead, generic and proprietorial systems were more likely to be deployed.
Further research is required to assess how far these issues might compromise the objectivity and equity of AI-based recruitment and selection systems.
In short, more effective collaboration is required. Many of the AI specialists appealed for this so that HR can help them build and annotate relevant data as well as supervise the testing and evaluation of the models. As one developer put it, “It’s important to have diverse groups of HR and AI experts working on building those algorithms. The more perspectives you have, the more diversity you have in building algorithms, the more representative it might be.’’
The professional identity of the HRM function is that it serves the business, and it does this through the equitable and responsible treatment of employees. Algorithmic HRM is shifting decision-making responsibility from human to machine, raising ethical issues around transparency, accountability and potentially embedded discrimination based on race, gender, age, or even subtle characteristics such as personality type. The everyday use of AI means that mathematical skills will increasingly be required of HR professionals to help shape and monitor AI tools that are fit for purpose. This will better serve the business, employees, and the HR profession itself, and thereby keep the human at the forefront of HR.
PRACTICAL IMPLICATIONS
First, employers should cooperate to build relevant New Zealand-focused datasets. New Zealand has unique characteristics in terms of its culture, economy and size that data needs to better encapsulate.
Second, the HR profession needs to further embrace ‘hard’ technical skills as well as people-focused competencies, if it is to credibly drive conversations with developers and IT specialists within the organisation.
Third, both AI specialists and HR professionals would benefit from training to understand the perspectives and vocabulary of their partners. The AI developers we spoke to tended to problematise in specific ways, whereas HR practitioners perceive issues and situations as complex and ambiguous.
Dr Melika Soleimani completed her doctorate at Massey Business School (MBS) in 2022 and is currently a data analyst at Southern Cross Health Care. Her supervisory team was Professors Jim Arrowsmith and David Pauleen (MBS), Dr Ali Intezari and Dr Nazim Taskin.