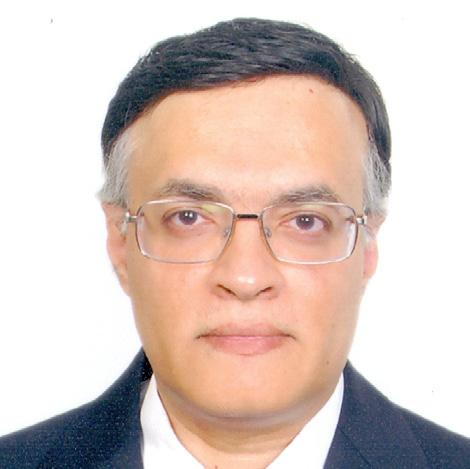
7 minute read
DR. PRATAP NAIR
PRESIDENT & CEO, INGENERO INC.
DATA ANALYTICS KEY TO TRANSFORMATION
Advertisement
The chemical industry has digitized its operations over the past three decades. The large volumes of a variety of historical data collected and stored have been growing exponentially with time and every second more data gets added. However, less than 10 per cent of the data is typically utilized and the rest archived. Despite the wealth of information that resides in this archived data, effective utilization and analysis have typically not been happening. Digitally enabled advanced analytics breaks this paradigm and allows utilization of the traditionally archived data to extract insights that are otherwise missed.
Analytics Paradigms in Chemical
In the chemical industry’s manufacturing step, chemical transformations and separations happen within pipes and vessels and the details of what is happening come from the sensor data. Market dynamics and changes to inputs to the process make manufacturing decisions even more complex. Chemical engineering fundamentals can explain the most complex interactions. For the past three to four decades first principle simulations capture this knowledge and describe expected process and equipment behaviour. However, while these analytics tools based on fundamentals have helped transform the chemical manufacturing plant design process by orders of magnitude over the years, the use of simulation to modify and improve ongoing operations has been limited.
Certainly, attempts have been made to use fundamental-based simulation models by combining them with data collected from the plants to predict the behaviour of the manufacturing process, to better control the process, to optimize operations, and to perform what-ifs to troubleshoot or plan future improvements to operations and design. Combining raw data with these fundamental models has been a challenge due to inherent errors in the data collected, model convergence issues, and limitations in getting timely inputs for decision making. Statistical data-driven models are often used when these issues are present or for processes and unit operations where fundamentals-based predictions are not available. Using limited data sets can make data-driven models tractable to solve. Likewise, simplified linearized models are used for planning purposes depending only on infrequent updates based on actual data. However, the disconnect among the various models from using different databases typically results in gaps between plans, schedules and response to market demands.
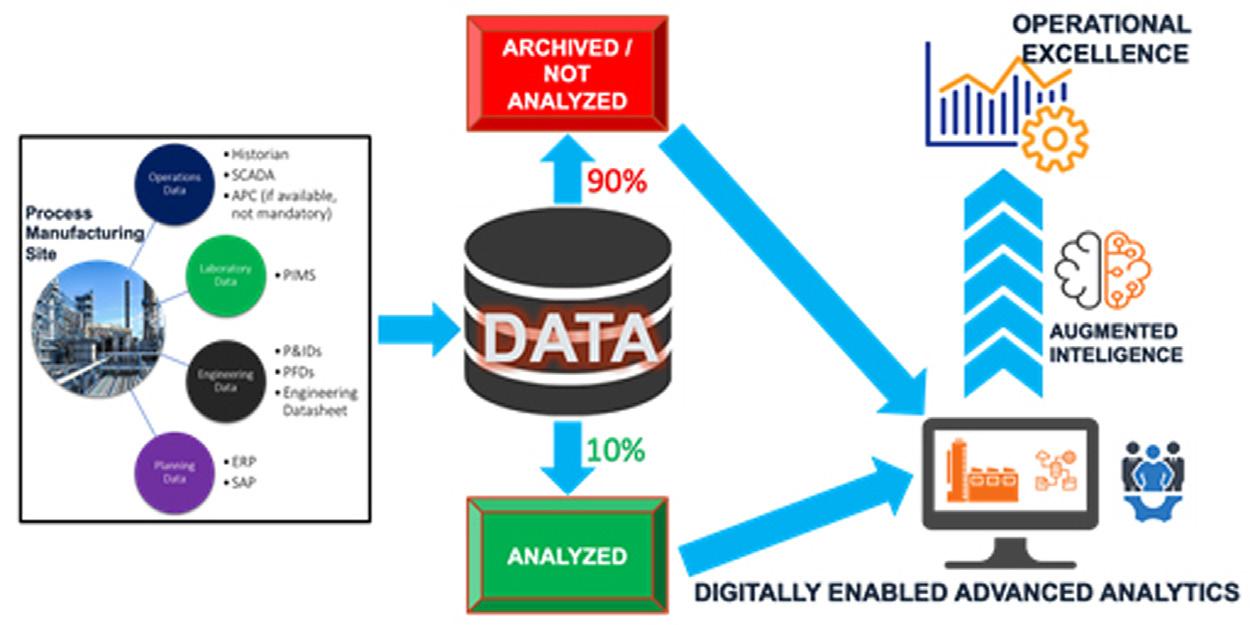
Advanced Analytics Paradigm Shift
The dramatic increase in computational power, communication speed and lower
mark with the previous best under similar conditions and how to get there in real-time for both continuous processes as well as batch processes (Identifying and replicating the performance of the “Golden batch”). • Revising and optimizing plans to changes in market conditions, faster • Integrating models to plan, schedule and operate, based on a consistent cost of data storage and computations ronmental impact, improve the reli- and updated data set over the past decade have been driving ability of operations, reduce the gap • Better handling of instrument drifts the development of technologies that between plans and actual and respond and equipment fouling in real-time enable making data available anywhere, faster to changes in the market or con- – minimize quality excursions, lowincreasing computational speed of large ditions. With digitally-enabled advanced er emissions volumes of data and better visual pre- analytics, chemicals companies have far • Better manufacturing operations sentation of data in real-time. This is wider and deeper insight than ever be- performance – production, yield, transformational. The application of tra- fore, enabling more informed strategic availability, costs (energy, specific ditional statistical techniques now pro- decisions. Digitally-enabled advanced raw material usage), lower operavide timely results using larger data sets analytics make sense of the data to drive tional risks and can include intelligence from fun- action on the physical assets and across • What-ifs at operations, engineering damental models. This has given rise different stages of the value chain. Data and planning levels, using updated to the wide use of technologies like Ma- about chemicals processes, asset perfor- models chine Learning (ML), Artificial Intel- mance, energy use and supply • Better emissions, energy and waste ligence (AI) and hybrid models chain operations are being management (combining fundamentals used to draw meaningful • Better demand forecasting with data models and selec- “Disconnect among the insights that can guide • Faster R&D and product developtively simplified models). various models from informed decision ment When combined with using different databases making. • Faster concept to execution other Industry 4.0 tech- typically results in gaps Specific opportu- • Data analytics to augment buyernologies like IIoT, more between plans, schedules nity areas where the seller arrangements with dynamic comprehensive databas- and response to market chemical industry is value-based pricing es, enhanced visualization demands” beginning to leverage • will likely impact the way tools and advanced data ana- digitally-enabled ad- chemicals companies operlytics, applications can greatly vanced analytics include: ate and grow their businessenhance decision making that for- • Improving Asset Per- es, as they shift away from merly required approximations or intu- formance the pay-by-the-ton revenue ition. Digitally enabled Advanced Data • Predicting reliability issues long model to provide value-added Analytics is a key ingredient to be able to before they occur, giving time to act products and services to their ultimately glean value from any Indus- on it proactively, preventing unan- customers. try 4.0 implementation and makes the ticipated downtime – increases pro- Dynamics of Advanced Analytics Impleconnection between the physical and the duction and asset effectiveness mentation digital world to deliver value. • Identifying and weeding out errors Domain expertise is a critical aspect of in data, using a larger data set, data setting up digitally-enabled advanced Impact of Digitally-enabled Advanced science techniques as well as intel- analytics. Software solutions require not Analytics ligence from fundamental models just software programmers but also doApplications are being made that dra- – decision quality information for main experts who can marry chemicals matically improve productivity, reduce engineers and planners domain knowledge with software capathe risks on account of safety or envi- • Dynamic benchmarking – bench- bilities. Converting analytics tools and
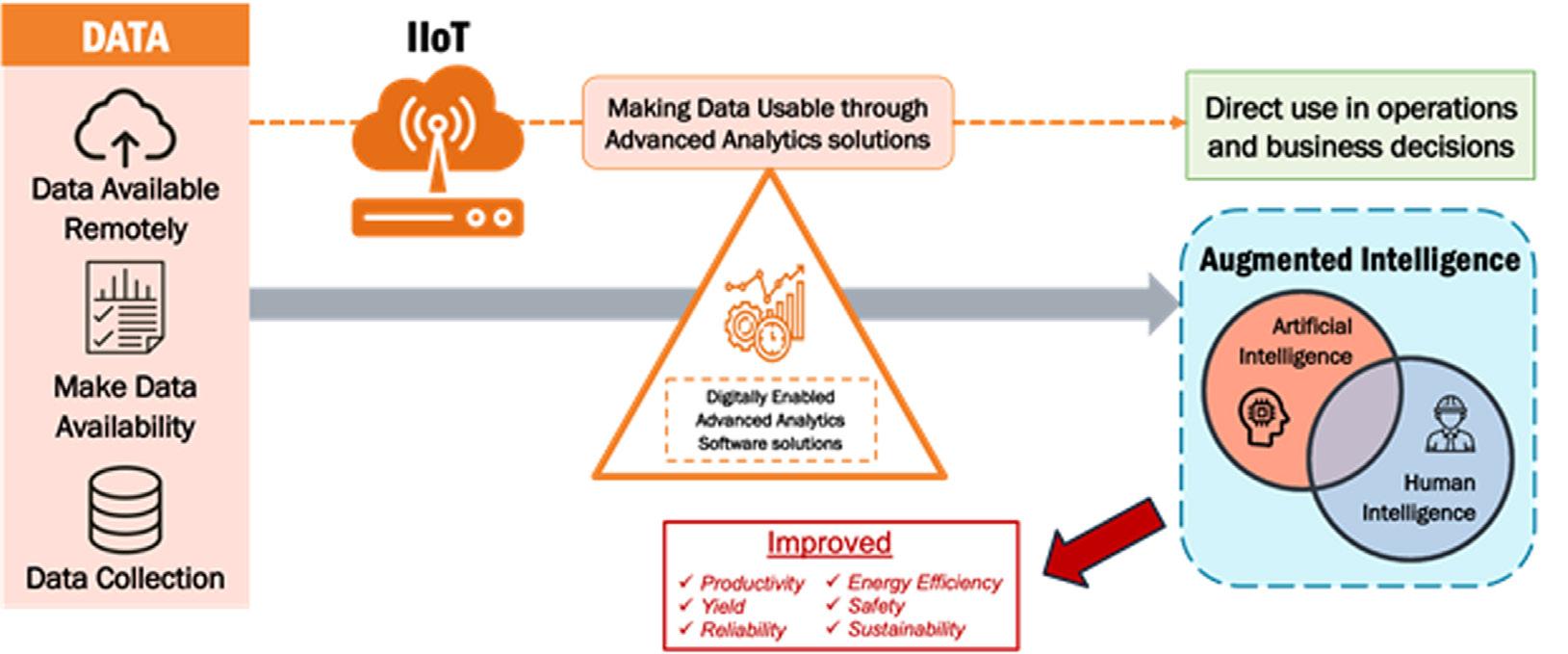
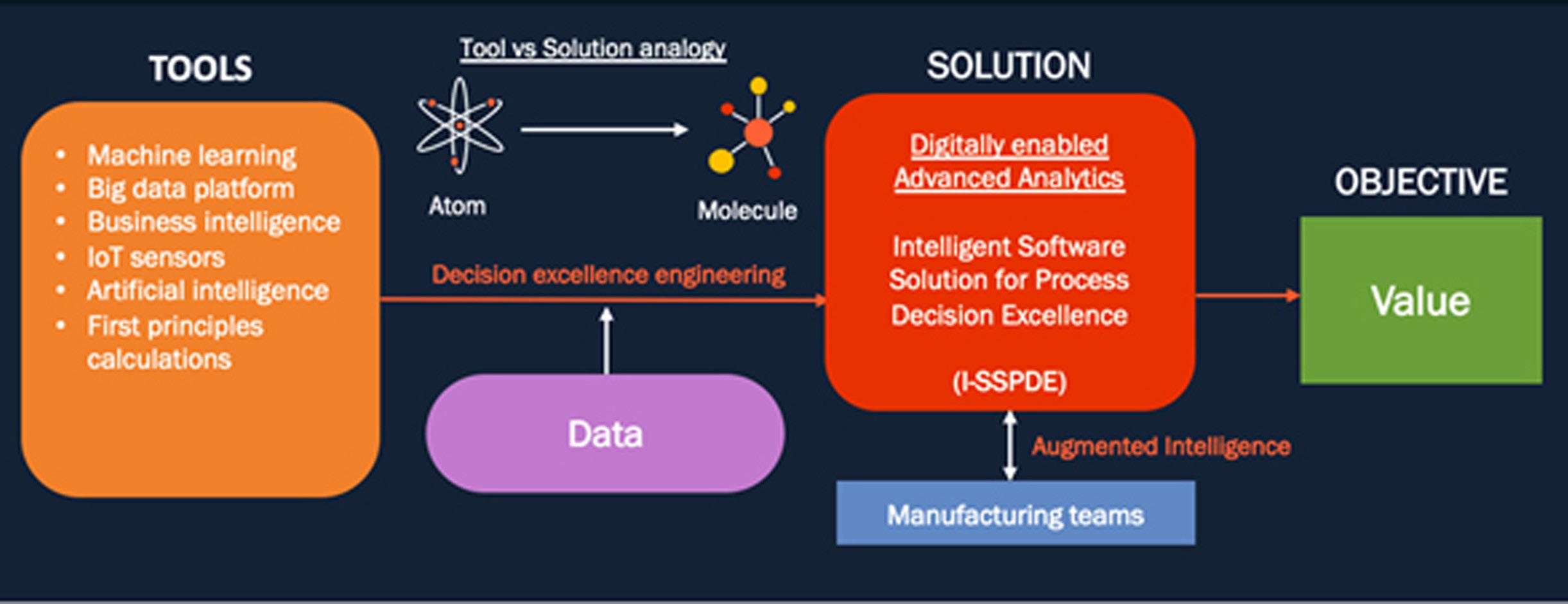
techniques into a solution that can provide Augmented Intelligence requires a specialized Decision Engineering Process.
Case Examples from Chemical Industry from Early Adopters
Combining engineering design, process engineering, software engineering and data science domain expertise allows powerful solutions to be built. These digitally-enabled advanced analytics ap-
plications with the appropriate decision engineering utilized have delivered impressive value: Increased capacity with better sustainability: • A long Chain Alcohol facility in
Louisiana, USA was able to overcome off-spec product challenges
and better manage multiple product transition complexity and was able to enhance capacity by 30per cent without CAPEX, improve first-pass quality by 11 per cent and stop external tolling, all of which improved the Carbon footprint, through use of continuous use of advanced analytics on their operations data. Increased profitability and efficiency: • Two ethylene facilities in the Middle
East of a major US Petrochemical
major were able to improve yield, plant availability, throughput and efficiency, saving US$ 250 million over 5 years, while simultaneously improving Sustainability parameters, through the utilization of a Hybrid Digital Twin using fundamental models and Machine Learn-
ing, along with remote tracking. Better visibility and quicker implementation: • A Midstream company in the USA, operating the largest NGL pipeline network in the USA, was able to increase profitability by 22 per cent, using Ingenero’s Digital Twin deployed over 40 facilities, providing centralized asset visibility, allowing a systematized identification and prioritization of process/operations requirements and optimization capabilities. Reduced product loss: • Advanced Analytics helped minimise ethylene losses in a process at a Petrochemical facility in India, addressing cyclic ethylene use and batch process challenges that the manufacturer was facing through concept development and follow through cost-effective engineering and implementation, providing an annual savings of US $ 650,000. 1.5 tons per day of ethylene loss was reduced to 100 kgs/day. Combining simulation with statistical data analytics (Machine Learning), domain expertise with software expertise and real-time data with thoroughly analyzed and modelled data enables powerful solutions for the chemical industry to be built using these tools drives significant improvement in terms of profitability and sustainability.