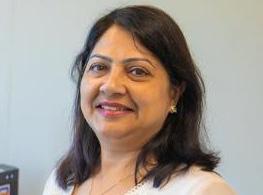
6 minute read
Optimizing the versatile utility of data science: meet researcher Richi Nayak
Associate Professor Dr Richi Nayak of the Science and Engineering Faculty at the Queensland University of Technology, who is the Applied Data Science Program Leader of the University Centre for Data Science (CDS), is a globally renowned expert in data mining, text mining and web intelligence. A Steering Committee member of the Australasian Data Mining Community since 2012, she has spearheaded research projects across a multitude of disciplines such as agriculture, health, transport infrastructure, marketing and online dating. Having a penchant for research in machine learning, she has focused her recent research initiatives on text mining, personalization, automation, and social network analysis. She was appointed the 2017 IT ambassador of the Queensland Women in Technology (WiT) association and has been conferred with many awards for her teaching, research and service activities.
In an exclusive interview to India News writer Deepika Banerjee, Dr Nayak talks about her specific area of research in data science, her passion to optimize data science applications to find feasible solutions to real life problems, the vast scope of data science and the pivotal role it can play in shaping the future.
Advertisement
1. How did you get interested in data science? What was the inspiration behind your taking up this area of research?
I was always interested in mathematics from a young age. For my Master of Engineering research thesis at the Indian Institute of Technology, Roorkee, I applied a Neural Network model (a machine learning algorithm in Data Science) for a Power Distribution problem. This fascination continued during my PhD where I developed
several Machine Learning algorithms. I am inspired by the capabilities and benefits that data science brings to society and organizations by understanding their data. Data is an asset in the 21st century and can be proved as being more precious than gold and oil if analyzed and interpreted effectively by data science methods. I have a driving passion to address pressing societal problems by innovating data science technologies underpinned by fundamental research in Data and Text Mining.
2. What is the specific area of your research in the field of data science? Are there any other areas related to data science on which you would like to focus in future?
I am a computational data scientist. Complex, multi-faceted datasets - generated through the interaction of machines and humans - pose new challenges to data science methods. My research interest involves generating innovative datadriven solutions by developing novel and efficient Machine Learning methods to discover new advances. In the last five years, my research has focused upon developing novel, cuttingedge algorithms and systems to facilitate ‘Automated Information Extraction’ and ‘Knowledge Discovery from Domain-specific complex datasets’.
3. What are the various applications of data science in the modern world context, particularly with respect to your areas of research and expertise? Can data science help in improving the quality of education and its delivery to people, especially to the underprivileged sections of the world?
My research has resulted in the development of novel data science solutions to address industry-specific problems in Marketing, K-12 Education, Agriculture, Digital humanities, and Mining. For example, industries like Agriculture, where the IT resources are scarce, may not possess a centralized repository with temporal and spatial information. In one of my projects, we developed a system to automate the data acquisition, processing and reporting of cotton sustainability indicators information that is available on multiple heterogeneous data sources. The system provides access to social, economic and environmental sustainability indicators, enabling users to generate information and graphics. Another example is Robotic Marketer (https://www. roboticmarketer.com/) ‘the world-first machine learningbased data-driven marketing strategy automation technology’. Machine learning methods allow a data analyst to understand user behaviour and identify common usage patterns. In the education domain, I have developed data mining models understanding factors leading to better academic performance (e.g., Naplan) and understating early leaver students’ behaviour patterns. These models provide agencies and authorities with data-informed decision-making strategies. Data science has a strong potential to understand patterns of education delivery models and customize them to specific groups or modes.
4. Can data science help in dealing with economic problems faced by the modern world such as poverty, unemployment, inequality, social justice, etc.? Would you like to be a part of any such research endeavour?
Indeed, there have been several successful attempts to unite data and domain scientists to work on focused projects that are designed to harness the power of data science in the service of humanity and impact public policy for social benefit. I am highly interested in such research endeavours. In one of my collaborative projects with social scientists and lawyers, we developed an autonomous system that can detect the posts with misogynistic content on Twitter. While currently, the onus is on the user to report abuse they receive, we hope that this machine learning solution can be adapted to automatically identify and report this content. Automating this process can reduce the emotional and cognitive load on users and moderators, and influence platform-level policy to protect women and other user groups online.
5. Are there any ethical considerations which data scientists must be particularly careful of while dealing with them?
Of course, there are data privacy and confidentiality considerations that data scientists should be following the best practices for. There are inherent data and algorithms biases that a data scientist should be aware of. Data analytics outcomes are as good as the data are. A data scientist should carefully choose the data for the underlying task. In recent years, we have heard the stories of big technology platforms facing the consequences of not including the diverse datasets. For example, high-paid jobs are displayed less frequently to women than men on LinkedIn; Google had to block gender pronouns from its predi¬¬ctive text functionality of Alexa; and the facial-detection technology implemented on Amazon and Facebook have been reported to misidentify women, particularly women with darker skin. These misinformed outcomes are results of not
having all possible scenarios while training the machine learning algorithms. Diversity of point of view is very important for machines to learn from examples as in data science.
Lastly, a data scientist should not fall into the common human fallacies of interpreting patterns over insufficient data and over reading correlation as causation; or adding bias that can skew answers. If not careful, one can easily draw incorrect or biased or even hazardous conclusions from the data insights.
6. Finally, how will applications of data science help in shaping the world we live in? Do you reckon that data science and artificial intelligence will impact all aspects of life in future?
With every organization now housing massive data sources, data science has become an increasingly popular way to turn an organization’s data into useful information and knowledge about their customers and their behaviour. There is almost no limit to where data science can be applied. With the rise of deep learning methods and acceptance of these methods for decision making in workplaces, data science is heading towards making the world a better place.
With the increased dependency of machine- led autonomous tasks, it becomes our responsibility to represent all perspectives and skills in technology development. Women need to engage and lead the data science projects sothat diversity is included in the development. Inclusion of diverse interpretations and biases can be significant when it comes to the analysis and input of data.

Richi Nayak