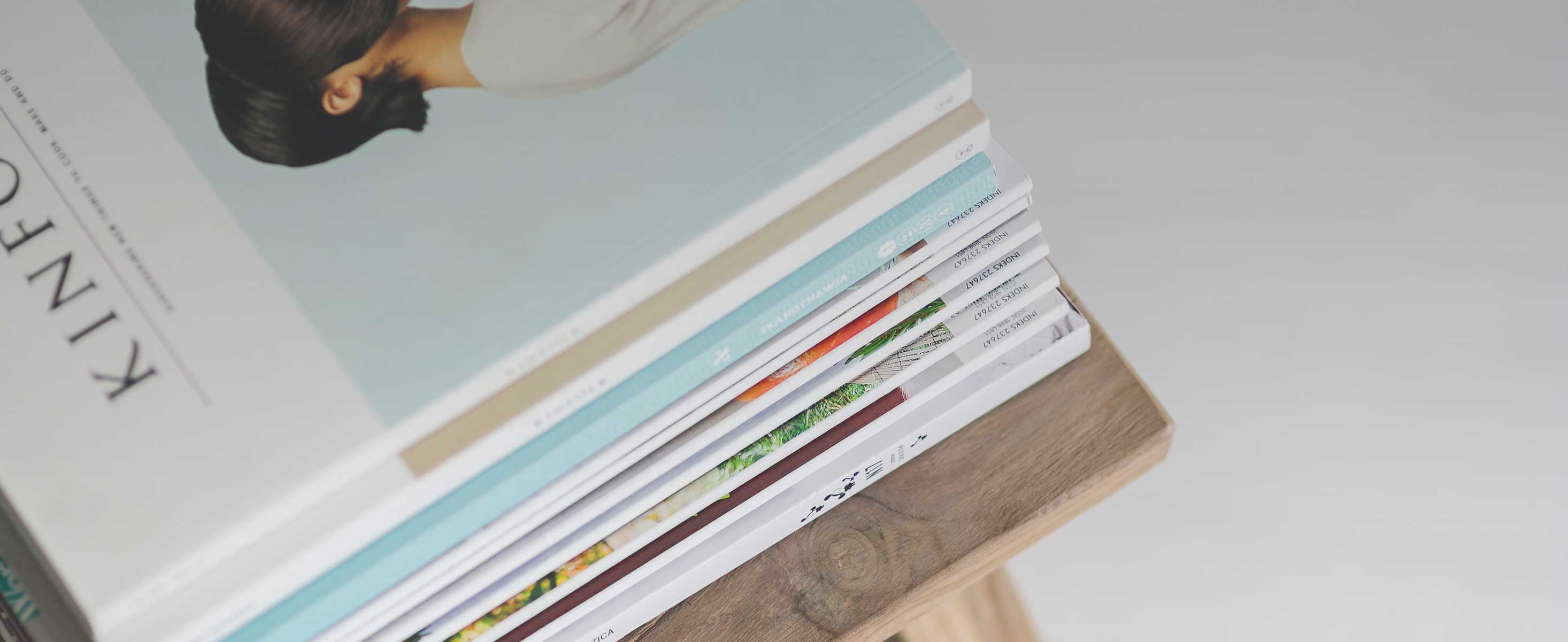
6 minute read
Table 7: Summary of key Kuunika achievements and challenges at midline
The table below summarises key Kuunika achievements and challenges identified at the independent midline evaluation.
Table 7: Summary of key Kuunika achievements and challenges at midline
Key achievements
1. In sampled districts, good evidence that end users had been trained on routine health data collection and data entry into paper registers, with on-the-job training across the board. All of the data entry respondents at health facilities with
EMRs confirmed they had been trained on EMRs. 2. Good progress in design and implementation of the HMIS smartphone application (app), along with training in linking the new app and digital services to health workers’ routine work needs and procedures. 3. While paper records remained by far the most popular source for looking up data in 2019, the use of DHIS2 had risen from less than one in five (18%) of all respondents to almost one in four (23%). Use of DHIS2 to enter data had increased since 2017 (baseline) by 7% to 10% of respondents. More data producers/entry users of EMRs and DHIS2 said they found it easier to enter data in 2019 compared to 2017. With regards DHIS2, 61% of those who reported accessing it at midline found it ‘fairly’ or ‘very’ easy, compared to only 15% at the 2017 baseline. 4. Both paper and EMR systems seemed to be taking less time overall for data entry than at baseline. The main reason for not being able to enter data in 2019 was the absence of an internet connection or other IT system failures. The problem of lack of power (cited in 2017 as the main reason) had substantially reduced; however, the frequency of respondents reporting a problem with digital data entry in the previous month had risen. 5. There has been a general increase in ‘high frequency’ searching for health data since 2017, with almost half (47%) of all respondents reporting they look up data ‘more than once a day’, compared with under a third (30%) in 2017. 5 By midline, there had been some improvement in the verification or triangulation of EMR and paper register health data.
By 2019, the Data Cluster Meetings convened by the Kuunika District M&E Officer provided an opportunity for a more representative group of data users to get together (beyond senior cadres) to assess data quality issues and how to address them.
6 By 2019, there was some evidence of a stronger and more active data culture. For example, managers requesting information at least once a week has risen from just over one in five (22%) in 2017 to over one in three in 2019. The proportion of respondents strongly agreeing that their colleagues thought data use for decision making had risen from 7% at baseline to 19% in 2019; however, more respondents identifies personal incentives and rewards as key drivers for data use than at baseline.
7 In 2019 as in 2017, we found almost complete agreement from our KAP respondents (98% and 96% respectively) that they knew where and how to find the information they needed for their job. 8 Although sharing of data between healthcare providers had shown some decline by midline, this appeared to be associated with an increased provider awareness of issues of data privacy and confidentiality.
Key challenges
1. The introduction of the EMRs, with frequent system down-times, was disrupting the use of paper registers and potentially reducing the data quality – with this reported as an increasing problem at midline than at baseline. Not only were power outages and connectivity challenges affecting EMR use and causing data back-filling problems, the recent updates of the ART and ANC modules of the EMRs now contain several coding errors. At midline, no respondents yet felt confident to replace paper-based registers with EMRs. 2. Growing concerns about the limitations of the EMR technology being rolled out to target districts. By 2019, there were increased user complaints of repeated EMR / data entry failures and significant backlogs of client data that needed to be ‘back-entered’. Users reported more time being spent on managing failing digital systems that was compromising their ability to provide and manage client services. 3. There was very little verification or triangulation of EMR and paper register health data by facilities in advance of sending monthly reports to the DHA (although there was some evidence of a small improvement in this by midline). 4. Use of other Kuunika target databases (to enter data) were falling compared to 2017. 5. The main reason for not being able to enter data in 2019 was now the absence of an internet connection or other IT system failures.
Annex 3: Data Quality Audit methodology and key findings
This annex describes the key features of the endline DQA (including the heuristic User Assessment) methodology and findings. The full DQA protocol and a more comprehensive account of the DQA methodology and findings are available from Mott MacDonald upon request
Description of the modified endline DQA
Due to the COVID-19 pandemic and associated travel restrictions, the endline DQA was conducted remotely. Access was given to the DHIS2 system which was fully accessible online. All relevant paper forms for the data elements (described below) from each of the 15 facilities were photographed by an enumeration team and uploaded to a shared online folder where they could be viewed remotely. The three-month period from 1 January 2021 to 31 March 2021 (first quarter 2021) was selected as the sample period.
Due to the remote nature of the evaluation, some of the components of the baseline DQA that involved facility visits could not be replicated, and so as an alternative, an in-depth ‘heuristic assessment’ of the DHIS2 website was conducted. For the quality assessment of indicators, rather than the evaluation of paper forms at facility level as conducted in the baseline DQA, the endline DQA focused on the data in DHIS2.
We have learnt that, since 2020, Kuunika has been involved in the monitoring and reporting of the COVID-19 pandemic in Malawi, creating a digital One Health Surveillance Platform (OHSP) that also sits on DHIS2. In order to capture and evaluate this activity, the endline DQA also included an assessment of the OHSP website. This assessment was less in-depth than that of the DHIS2 website as we understand it is a work in progress.
The following data was assessed as part of the endline DQA:
● A comparison of 3 health metrics, or ‘proxy indicators’, in the DHIS2 system: – Indicator 1: Number of pregnant women newly testing HIV positive – Indicator 2: Number of HIV positive clients initiated on ART for the first time – Indicator 3: Number of nurses [posted] at the facility ● Trends in HMIS/DHIS2 user metrics from 2017 to 2021 ● An ‘heuristic assessment’ of the user look and feel of the DHIS2 website ● A lighter assessment of the user look and feel of the OHSP website.
The three proxy indicators above were assessed for four dimensions of data quality –completeness, accuracy, availability, and timeliness – with definitions appropriate to DHIS2, rather than paper registers. The dimensions of data quality in the baseline and endline DQAs are described in Table 8 below.