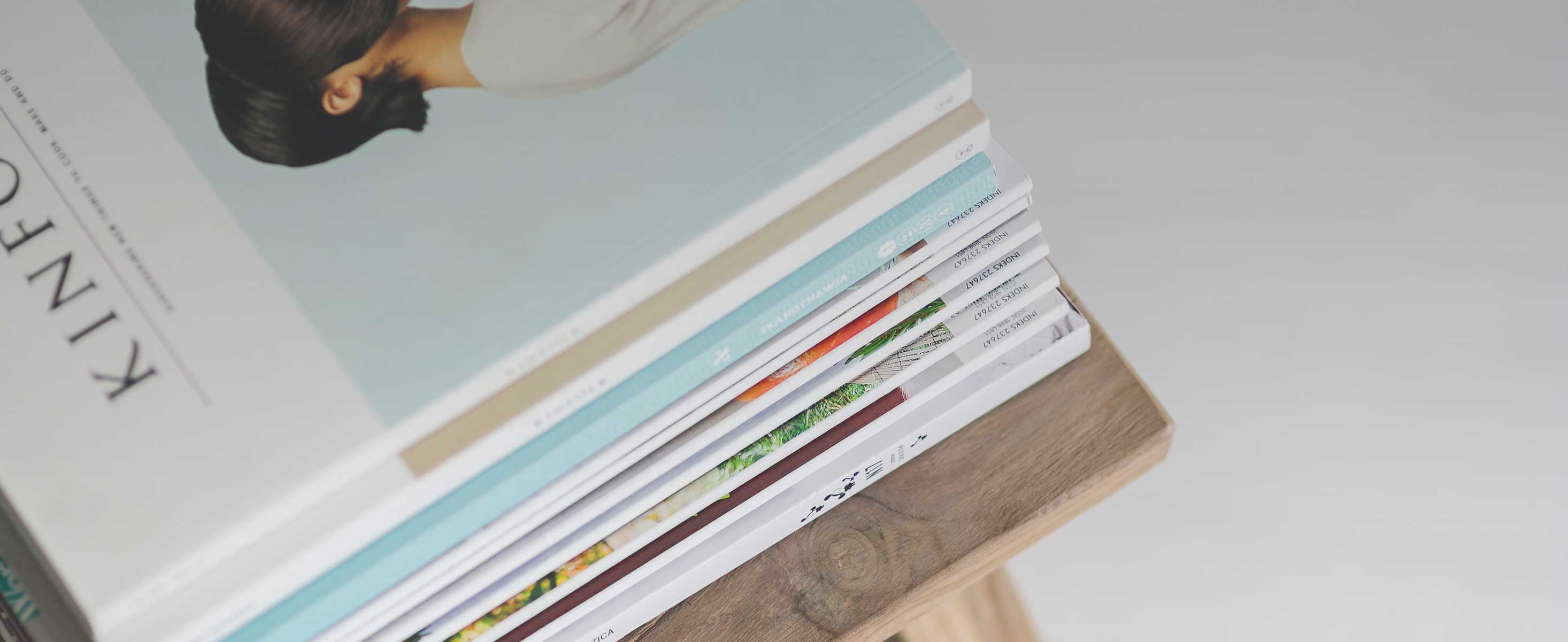
2 minute read
Box 4-1: Contribution to economic research and methodology
Box 4-1: Contribution to economic research and methodology
Large, complex datasets, from which we can retrieve valuable information, expand our knowledge of the world. The contribution of big data to economics is significant, due to the fact that aggregated data become decomposable, and a number of questions become clearer for policymakers. Since we can observe economic phenomena more efficiently, it will be more and more easy to estimate the impact of policies. This not only facilitates the testing of existing economic theories, the development of new ones also becomes simpler (Harding – Hersh, 2018).
Questions also change in the research – “why” is replaced by “what". In practice this means that the role of experts is revalued; it is no longer important to understand, for instance, why consumers prefer the respective product – the most important question is what they like. On the other hand, data scientists come to the front in many places, although this does not mean that professional experience will not matter at all in the future, only that specialist skills become less important (Cukier – Mayer, 2014).
New analysis methodologies, such as machine learning (ML), are more and more common. ML methods improve the out-of-sample estimates, i.e. improve forecasts. This partly originates from the aforementioned phenomenon; the search for causal relations (i.e. the question of “why”) lose significance to the benefit of the simple correlations (the question of “what”).
The performance of the econometric methods on small sample, traditional datasets outstrips the machine learning techniques (Chart 4-7). However, in parallel with the rise in the elements of a sample ML methodology performs better
and better, and finally outperforms the econometric methods.
Chart 4-7: Performance of machine learning and econometrics upon increasing the number of elements in the sample
Predic�on accuracy / model performance
Standard data sets
Source: Harding – Hersh 2018, MNB compilation. ML methods
Tradi�onal econometrics/sta�s�cal methods
Big data
The econometric approach primarily focuses on the statistical, data generating process (Table 4-1). By contrast, machine learning does not intend to explore the data generating process, but is rather built on algorithms. Econometrics focuses on theory, while ML on data fitting. At the first, the model is selected on the basis of the fit within the sample and the parameter significance, while in the latter along the cross validation of the out-of-sample estimate. Thus, econometrics is strong in the identification of causal relations and small sample analysis, while the ML technique is strong in forecasting and large sample analyses.