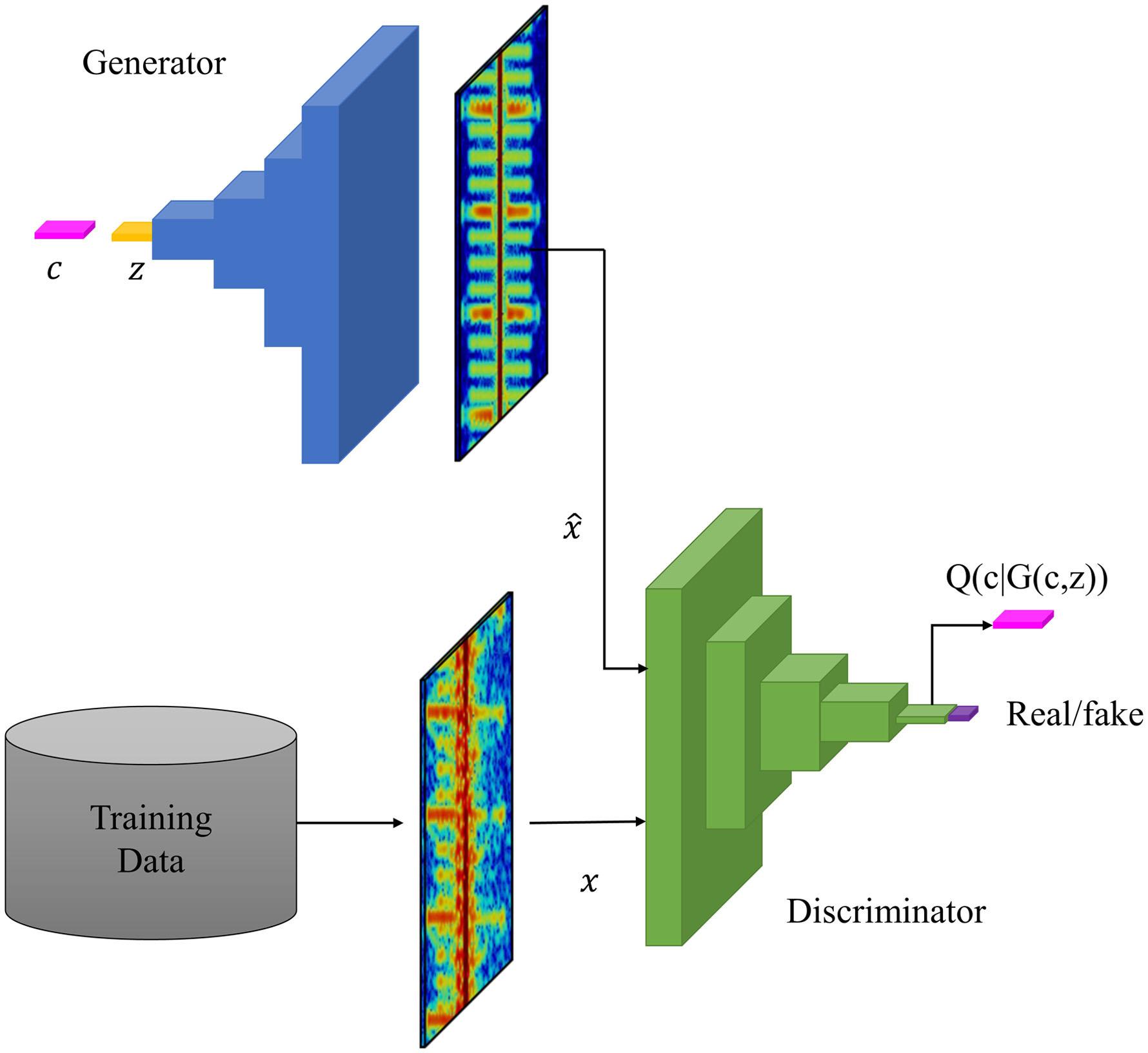
1 minute read
B. Dekker, S. Jacobs, A. S. Kossen, M. C. Kruithof
from Deep Learning for Classification of Mini-UAVs Using Micro-Doppler Spectrograms in Cognitive Radar
by Jinghua
Figure 9. InfoGAN architecture.
that consists of a generator, a discriminator, and an auxiliary unit.
Advertisement
The generator input vector consists of the concatenation of two parts: a noise vector (z) and a latent code (c). The generator tries to fool the discriminator by generating a realistic spectrogram, whereas the discriminator tries to distinguish the synthetic and real spectrograms. In addition to the probability that an input is a real or a fake spectrogram, the discriminator also computes a distribution Q(c j G(c,z)) that measures the mutual information between the latent code c and the generated spectrogram.
Based on an information-based regularization term within the overall training cost function, a structure is introduced in the latent variable that enables the aggregation of spectrograms characterized by similar characteristics. This is apparent in the generated spectrograms shown in Figure 10 for nine different categories associated with nine different states for the latent code (c). In addition, since clustering is based on spectrogram appearance, instances of the same class characterized by differing spectrograms will be associated to different categories. For example, both examples from category 2 and 3 belong to the T-REX 550 helicopter class, although characterized by a different Doppler bandwidth. When the specified categories are higher than the level of diversity among the dataset examples, then overlap between categories can be expected, for instance as observed for categories 5 and 9.
CONCLUSION
The results of several experiments in this paper have demonstrated the potential of deep learning techniques for the classification of mini-UAVs with a cognitive radar. Deep neural networks, such as CNNs and RNNs can provide an accurate classification performance after being trained on a set of measured micro-Doppler spectrograms that are stored in the long-term memory of a cognitive radar.
The analysis of the classification performance of different types of deep neural networks and different preprocessing parameters showed that the choice of the coherent
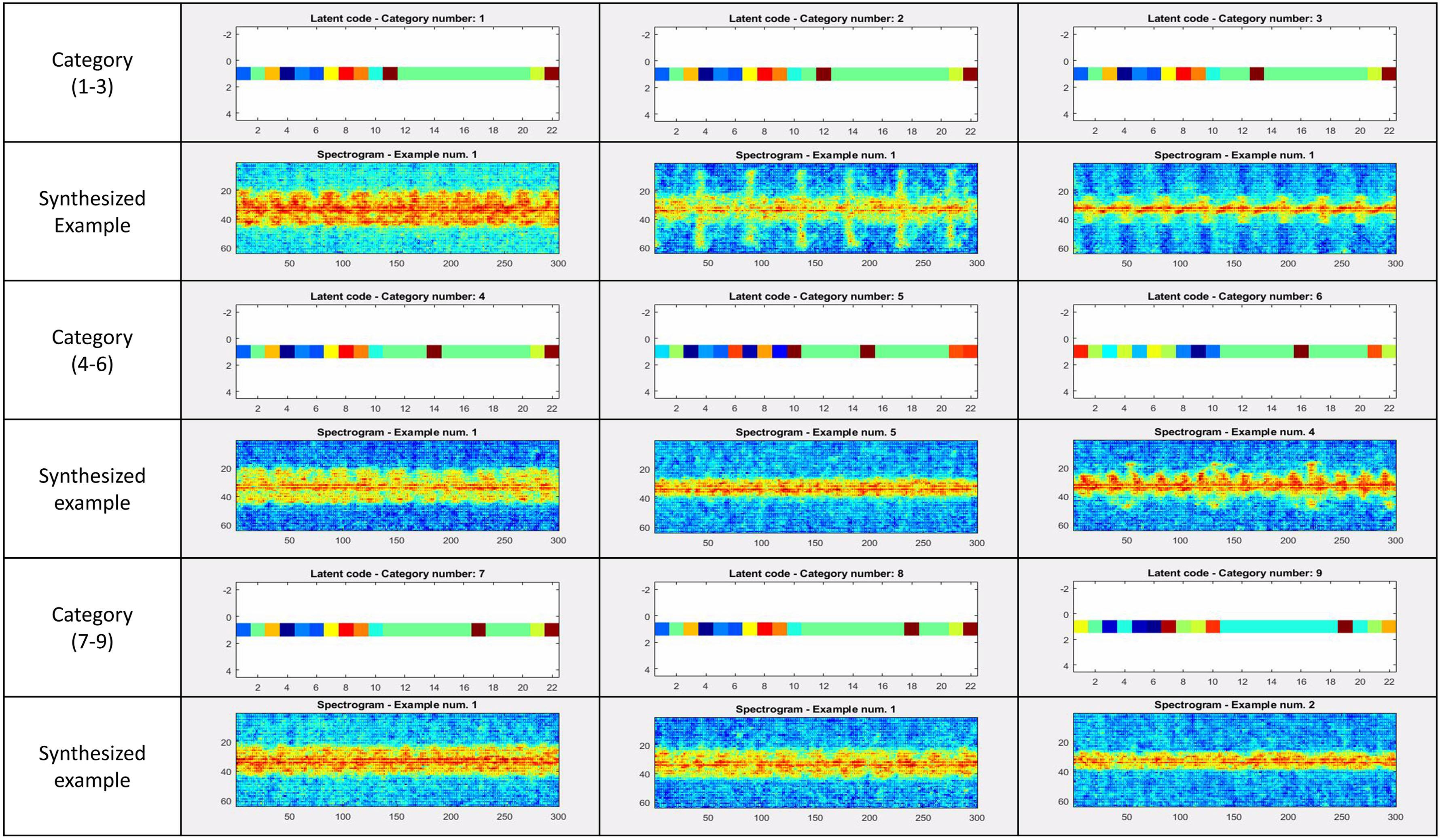
Figure 10. InfoGAN applied to micro-Doppler spectrograms with examples of synthesized spectrograms per category of the latent code.