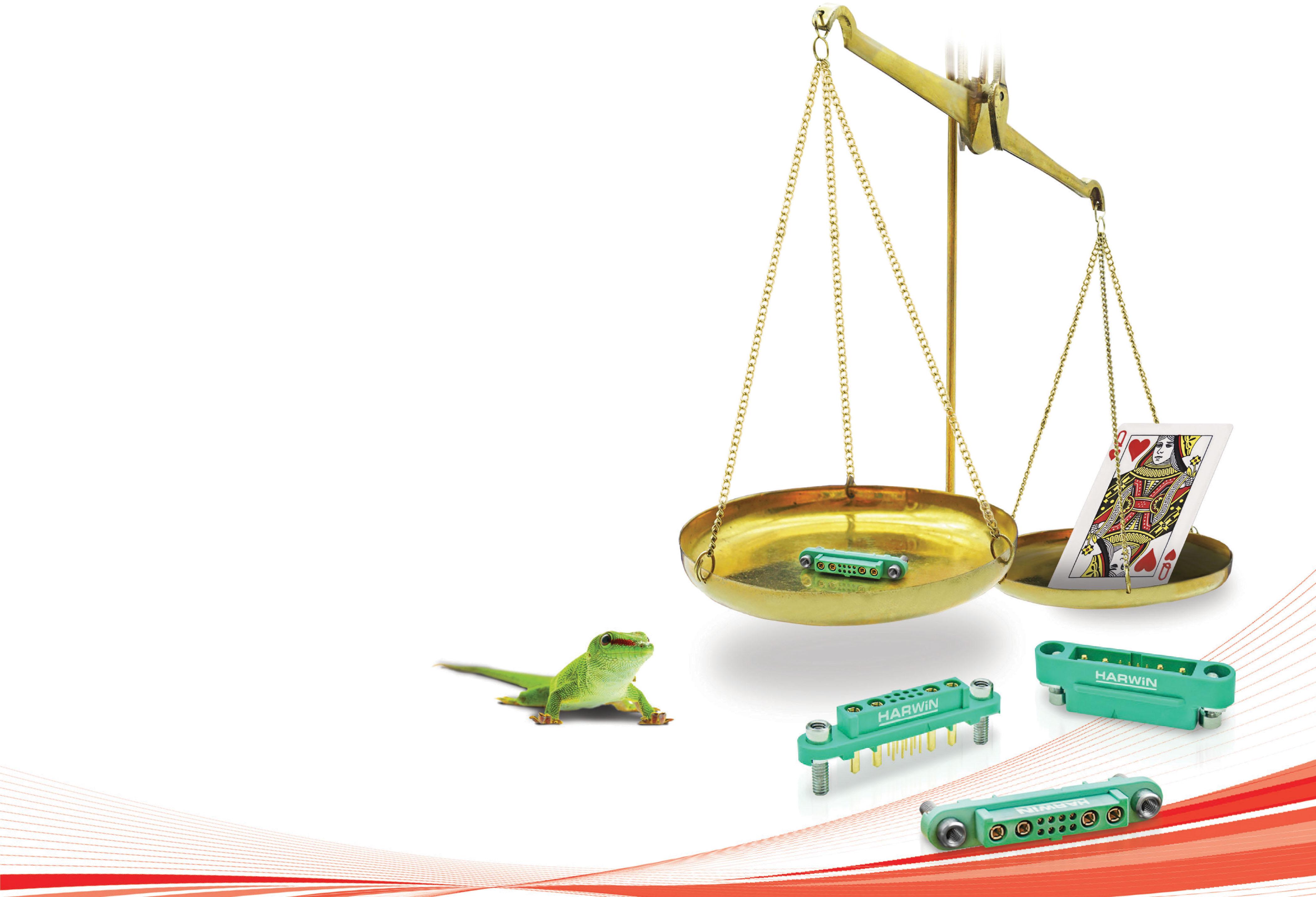
1 minute read
pp. 172–175
from Deep Learning for Classification of Mini-UAVs Using Micro-Doppler Spectrograms in Cognitive Radar
by Jinghua
[14] G. Klarenbeek, R. I. A. Harmanny, and L. Cifola, “Multitarget human gait classification using LSTM recurrent neural networks applied to micro-Doppler,” in Proc. 14th Eur. Radar Conf., 2017, pp. 167–170. [15] A. Bendale and T.E. Boult, “Towards open set deep networks,” in Proc. IEEE Conf. Comput. Vis. Pattern Recognit., Jun. 2016, pp. 1563–1572. [16] D. M. J. Tax and R. P. W. Duin, “Growing a multi-class classifier with a reject option,” Pattern Recognit. Lett., vol. 29, no. 10, pp. 1565–1570, 2008. [17] S. Akcay, A. Atapourabarghouei, and T. P. Breckon, “GANomaly: Semi-supervised anomaly detection via adversarial training,” in Proc. ACCV, Perth, Australia,
Dec. 2018. [18] T. Salimans, I. Goodfellow, W. Zaremba, W. Cheung, A. Radford, and X. Chen, “Improved techniques for training GANs,” in Proc. 30th Int. Conf. Neural Inf. Process. Syst., 2016, pp. 2234:2242. [19] A. Creswell and A. A. Bharath, “Denoising adversarial autoencoders,” IEEE Trans. Neural Netw. Learn. Syst., vol. 30, no. 4, pp. 968–984, Apr. 2019. [20] M. Buda, A. Makib, and M. A. Mazurowski, “A systematic study of the class imbalance problem in convolutional neural networks,” Neural Netw., vol. 106, pp. 249–259, 2018. [21] X. Chen, Y. Duan, R. Houthooft, J. Schulman, I. Sutskever, and P. Abbeel, “InfoGAN: Interpretable representation learning by information maximizing generative adversarial nets,” in Proc. 30th Int. Conf. Neural Inf. Process. Syst., 2016, pp. 2172–2180. [22] T. Schlegl, P. Seebo¨ck, S.M Waldstein, G. Langs, and U. Schmidt-Erfurth, “f-anogan: Fast unsupervised anomaly detection with generative adversarial networks,” Medical image analysis, vol. 54, pp. 30–44, May 2019.
Advertisement