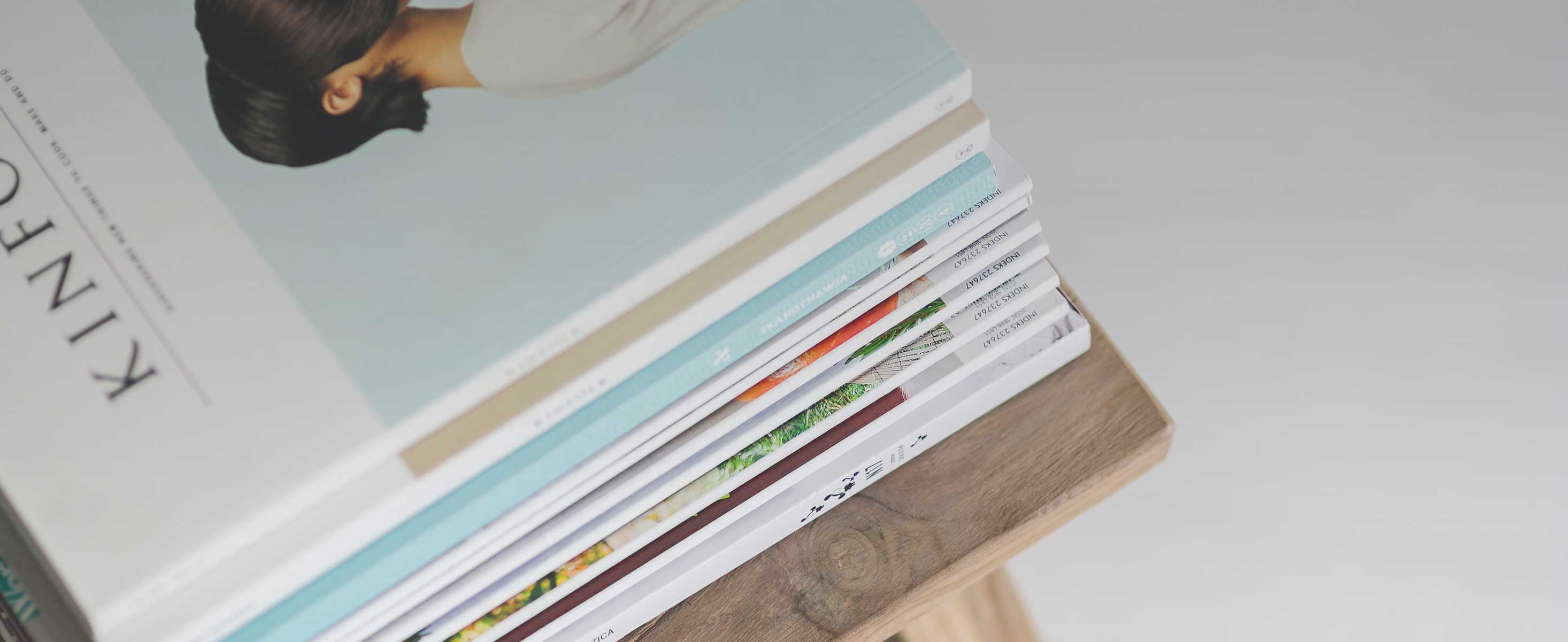
3 minute read
THE DETERMINATION OF ERGOT ALKALOIDS IN CEREAL SAMPLES
ION MOBILITY-MASS SPECTROMETRY TO EXTEND ANALYTICAL PERFORMANCE IN THE DETERMINATION OF ERGOT ALKALOIDS IN CEREAL SAMPLES
L. Carbonell-Rozas1,2, M. Hernández-Mesa1,2, L. Righetti3, F. Monteau2, F.J. Lara1 , L. Gámiz-Gracia1 , B. Le Bizec2, C. Dall’Asta3, A.M. García-Campaña1, G. Dervilly2
Advertisement
1Department of Analytical Chemistry, University of Granada, Campus Fuentenueva, 18071 Granada, Spain. 2Oniris, INRAE, LABERCA, 44300 Nantes, France. 3Department of Food and Drug, University of Parma, Parco Area delle Scienze, 43124 Parma, Italy
rozas@ugr.es
This work evaluates the potential of travelling wave ion mobility spectrometry (TWIMS) to improve the analytical performance, in terms of selectivity and concentration sensitivity, of liquid chromatography-mass spectrometry (LC-MS) workflows applied to the determination of ergot alkaloids (EAs). EAs are mycotoxins produced mainly by fungi of the Claviceps genus, prevalent in cereals whose ingestion can cause ergotism in humans and animals. The simultaneous determination of EAs with current LC-MS methods has some limitations since several of these compounds are epimers and show similar retention times and an identical mass-to-charge ratio (m/z). In this regard, taking advantage of the third dimension offered by TWIMS, which provides the measurement of the rotationally averaged collision cross section (CSS), we report the first CCS database for the main EAs to support their unequivocal identification. The generated CCS database was inter-laboratory cross-validated and compared with CCS values predicted by machine-learning models. In this context, slight differences were observed in the experimental CCS values obtained for ergotamine, ergosine and ergocristine and their corresponding epimers (ΔCCS/CCS between 3.3 and 4 %), which were sufficient to achieve peak-to-peak resolution in the TWIMS dimension. In addition, a LCTWIM-MS method was applied to the analysis of the main EAs in cereal samples. The integration of TWIMS in the LC-MS workflow improved the signal-to-noise ratio (S/N) between 2.5 and 4-fold; therefore, signal sensitivity was improved. Furthermore, cleaner mass spectra were observed as EAs were also separated from the chemical background in the TWIMS dimension. The analysis of cereal samples resulted in the finding of positive samples in EAs with concentration levels between 8.3 and 36.8 μg kg-1 in barley samples, and between 5.2 and 65.0 μg kg-1 in wheat samples.
Acknowledgements: Research Project RTI2018-097043-B-I00 financed by MCIN/AEI /10.13039/501100011033/ FEDER “Una manera de hacer Europa” and Junta de Andalucía-Programa Operativo FEDER (B-AGR-202-UGR20). Keywords: travelling wave ion mobility-mass spectrometry, ergot alkaloids, cereal samples, collision cross section.
19
CLASSIFICATIONOFINDIVIDUAL WHEAT KERNELS ACCORDING TO FUSARIUM SYMPTOMS ANDDEOXYNIVALENOL BYNEARINFRAREDHYPERSPECTRAL IMAGING
A. Femenias, A.J. Ramos, V. Sanchis, S. Marín
Applied Mycology Unit, Food Technology Department, University of Lleida, AGROTECNIOCERCA Center, Av. Rovira Roure 191, 25198, Lleida, Spain
antoni.femenias@udl.cat
Farmers, importers and cereal processors remain interested on a rapid and cost-effective technology able to detect Fusarium damage and associated mycotoxins, as deoxynivalenol (DON). Near infrared hyperspectral imaging (HS-NIR) not only has the faculty of detecting chemical changes of cereal batches, but also the ability to analyze individualized areas within the sample. This ability permits to look beyond the traditional cereal bulk analysis to focus on the individual kernel management. In this way, HSI-NIR would be able to detect, by individual kernel analysis, the high-contaminated fraction of a batch as a cereal sorting strategy. Keeping this idea in mind, the main purpose of this work was to build classification models based on multivariate analysis able to discriminate between healthy and Fusarium and DON contaminated kernels by using NIR data. To reach this aim, NIR spectra of 300 wheat kernels were extracted by the imaging system prior to UHPLC analysis. Several spectral pretreatments were applied to NIR data to highlight valuable attributes and to reduce noise and scatter effects. For kernel Fusarium damage classification, the most accurate discrimination precision reached was 85.8 % by the use of Artificial Neural Networks (ANN) classifier and the absorbance spectra. Otherwise, for DON contaminated kernels discrimination, the precision was reduced to 76.9 % of correctness for Standard Normal Variate pretreated spectrum, probably due to the low correlation observed between fungal symptoms and mycotoxin production. The achievements demonstrated the potential of HSI-NIR to analyze physical and chemical variations of wheat kernels for fungal damage and mycotoxin management.
Acknowledgements: This work was supported by the Spanish Ministry of Economy, Industry and Competitiveness (MINECO/AEI/FEDER, UE, project AGL2017- 87755-R and PID2020114836RB-I00). Antoni Femenias acknowledges the financial support of the University of Lleida (predoctoral grant).
Keywords: Hyperspectral imaging; near infrared; single wheat kernels; Fusarium; deoxynivalenol
20