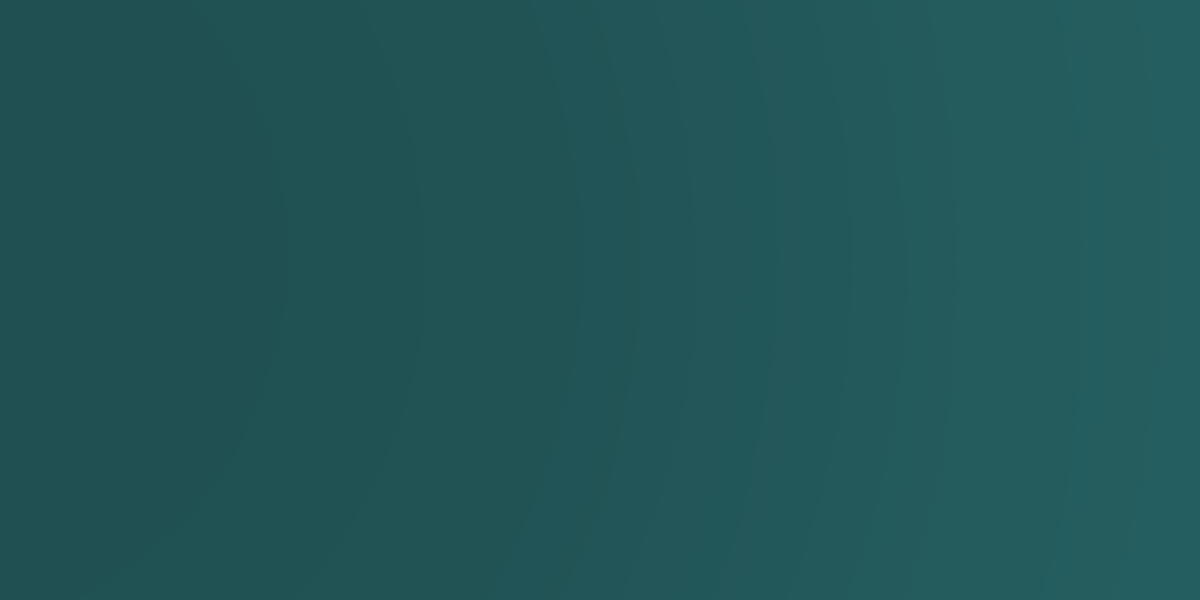
4 minute read
Utilizing Machine Learning for the Precise Mapping of Turfgrass Pests
Utilizing Machine Learning for the Precise Mapping of Turfgrass Pests
By Elisabeth Kitchin Graduate Student, Virginia Tech
Turfgrass pests, including diseases and weeds, are a constant challenge for turfgrass managers, causing aesthetic damage, reduced playability, and economic losses. Targeted pesticide applications, where treatments are applied only to areas affected by pests, offer a more sustainable alternative to traditional broadcast spraying. These targeted applications decrease environmental inputs and lower costs while maintaining sufficient control of target pests. The effectiveness of this approach, however, hinges on the accuracy and precision of pest maps that guide the targeted applications.
Creating accurate pest maps has long been a labor-intensive and time-consuming task. Turfgrass managers often rely on visual inspections to locate and quantify pest outbreaks, a process that is not only subjective but also inconsistent, as results can vary depending on experience and environmental conditions. The inefficiency of pest mapping presents a barrier to the widespread adoption of targeted pesticide applications despite their potential to significantly reduce inputs and environmental impact. This project addresses pest mapping inefficiencies by developing a machine learning model to automate the detection and mapping of turfgrass pests, providing a more efficient and objective solution for turfgrass managers.
The YOLOv8n-seg machine learning model was selected for the project due to its capabilities in object detection and segmentation. This dual functionality makes it particularly suited for turfgrass pest management, as it can both identify individual pest instances and map their precise spatial boundaries.
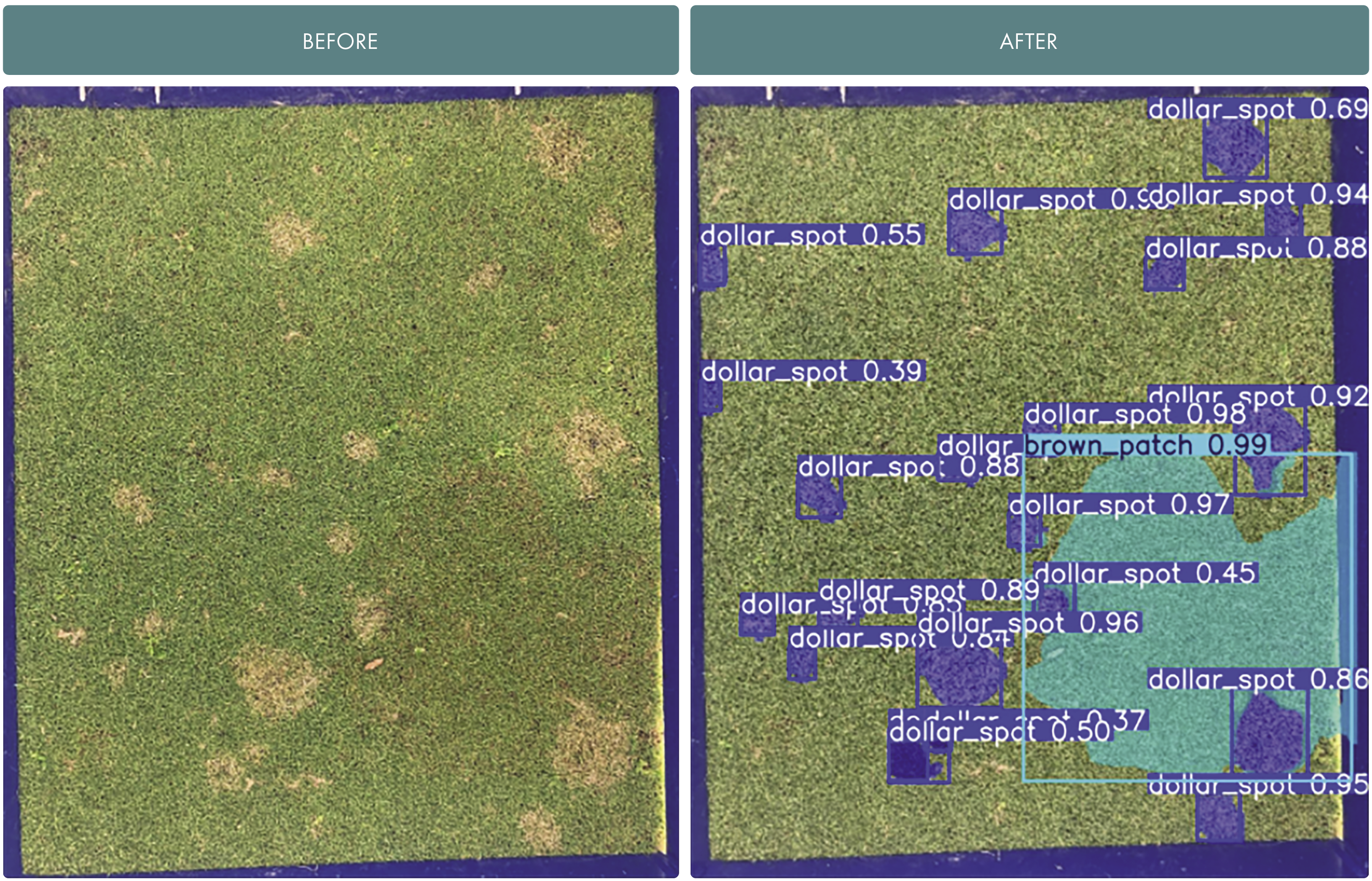
A dataset was compiled to train the model, comprised of over 2,500 images of common turfgrass pests from across the country. These pests include various turfgrass diseases, such as dollar spot and brown patch, as well as common turfgrass weeds, such as green kyllinga and white clover. The training set also included images of various turfgrass species, heights of cut, environments, and a range of management practices.
The model was trained on the image dataset and taught how to identify each pest and differentiate it from other pests or abiotic issues, including drought stress, nutrient deficiencies, or ball marks. Given an image, the model can identify and outline the borders of the pest outbreak. In images with multiple pests present, the model can differentiate between them and individually identify and map each pest in the image.
The model’s performance was then evaluated for accuracy, precision, and segmentation capabilities. It achieved 96% accuracy in detecting pests and identifying their locations. The model’s ability to delineate pest boundaries was assessed using a metric known as the mean Intersection over Union (IoU), which measures the overlap between the model’s predictions and ground-truth data. The mean IoU score of 70% indicates that the model provides reliable spatial mapping of pest infestations. Finally, the F1 score, a metric that measures the model’s precision and recall abilities, achieved 72%. This score indicates the model’s competency and reliability in both identifying and mapping pests.
These results showcase the transformative potential of machine learning in precision turfgrass management. This model offers a practical solution to save time, labor, and costs while delivering accurate, efficient pest maps to guide treatment decisions. By automating a traditionally manual and subjective task, the model addresses a barrier that has hindered the widespread adoption of precision techniques, including targeted pesticide applications. For turfgrass practitioners in Virginia and beyond, these advancements present a valuable opportunity to enhance operational efficiency, reduce environmental impact, and support the adoption of sustainable practices in the turfgrass industry.