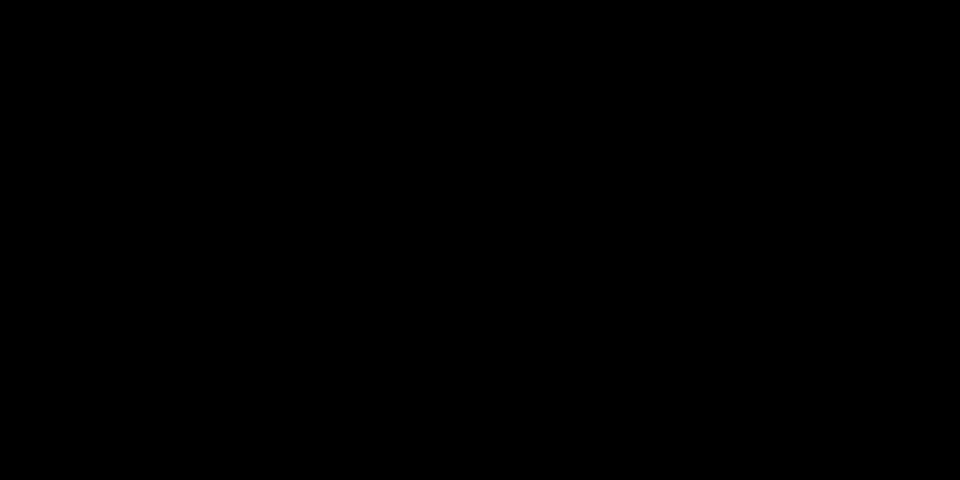
6 minute read
A Flock of Birds and the Complex Systems: Unfolding the Characteristics of Complex Systems in Sports Injury Rehabilitation
A FLOCK OF BIRDS AND THE COMPLEX SYSTEMS:
Advertisement
FEATURE / KATE YUNG
Introduction
Since the development of the injury prevention model with the complex systems model [1], there has been increasing interest in the complex systems theory. Practitioners have been trying to understand how the model is relevant to their practice and how will it change their practice. The article aims to explain what the complex systems theory is and how it is relevant to the daily practice and operation in football.
What is the complex systems theory?
• The complex systems theory, with more than 50 years of history [2], acknowledges the multifaceted nature of sports and seeks to understand the interactions among different factors and the outcomes of the systems [1, 3]. • Complex systems are dynamic, open systems [4]. They are characterised by non-linearity due to feedback loops and interaction among the factors. This means that outputs are not always proportional to the inputs, and a small adjustment may lead to a large change in the systems and vice versa [5]. • Complex systems are composed of a large number of interacting components which give rise to global behaviour and make up a system that exhibits novel characteristics [4]. • In the context of return to sport (RTS), these units could include age, wellness, biological healing of injured tissue, stress, external pressure and injury history. The units interact and define the space and dimension of the systems [6]. • This means that complex systems can not be understood by studying their parts.
Instead, it may be better to be studied from multiple perspectives. • Examples of complex systems are flocks of birds, ecosystems and immune systems.
Complex systems explained with a flock of birds
• All individual birds follow a simple rule: maintain proximity without bumping into each other. That results in emergent behaviour known as flocking (Figure 1).
Complex systems Complicated & mul�factorial only.
Figure 1. A flock of birds and the complex systems
• The flock emerged without any lead bird directing each bird’s action. • Multiple perspectives are required when viewing complex systems. The systems are three dimensional and interactions within the systems often occur at different scales and levels [7]. These include the environment, ecosystem and human activity (Figure 1).
Complex systems in rehabilitation
• Human systems, like a flock of birds, are also complex systems with distinctive characteristics. Using anterior cruciate ligament (ACL) rehabilitation as an example, we have described the characteristics of complex systems in our paper “Characteristics of Complex Systems in Sports Injury Rehabilitation: Examples and Implications for Practice”[8]. • In the context of RTS, the interacting factors could include age, wellness, biological healing of injured tissue, stress, external pressure and injury history. The factors will interact with the environment and other factors and consequently, different systems within systems emerge. • These systems may be categorised based on their nature, for example, biomechanical, physiological and psychological. • They are also of multiple levels, namely individual, organisational and environmental. The individual level represents factors related to the individual athlete, from tissue healing to personal traits. The organisational level represents external factors related to the sporting club, organisation and support team, e.g., the coaching and medical team. The environmental level covers factors beyond the organisational level, such as the weather, playing schedule and competition level.
FAQ 1: I know rehabilitation is complex and multifactorial, why do I have to bother about complex systems?
• The complex systems approach provides a theoretical framework for interpreting the patterns that emerged from biopsychosocial and other external factors. It is a tool for analyzing a problem more thoughtfully and efficiently.
• In ACL rehabilitation, conducting independent clinical tests and functional assessments may provide useful information regarding the athletes’ physical and mental status. However, a complex systems approach facilitates a more complete picture of the problem and increased awareness of how different factors may interact. • We encourage practitioners to consider multiple perspectives and come up with a solution or protocol that is broad and may address the root cause of a problem.
FAQ 2: If return to sport is so complex, is there anything we can do?
• When assessing the test result for clinical and functional tests, practitioners should also be aware of the dynamic systems evolving around injury rehabilitation and endeavour to understand the full picture. • There are at least two challenges in understanding and explaining the behaviour of systems: 1) The high degree of complexity and 2) it is nearly impossible to isolate a portion of the larger systems (i.e., isolation of the biological healing process from broader biopsychosocial factors). • We may have to rely on computerbased decision support systems that have the capacity of incorporating features of complex systems in their design and utility. For example, the use of machine learning techniques that could accommodate non-linearity association. • Machine learning is often characterised by five major approaches (i.e., association, classification, clustering, relationship modelling and reinforcement learning), each having already been applied for injury risk assessment and/or performance prediction in sports [9-13].
Conclusion
• Complex systems are dynamic, open systems with distinctive characteristics. • We encourage a shift in paradigm to a complex systems approach when making decisions regarding return to play. • The use of computational modelling and machine learning techniques may have the capacity to identify the regularities of the pattern that emerged as a whole. Machine learning 5 major approaches Clustering Relationship modelling
Association Classification Reinforcement learning
Figure 2. Major approaches of machine learning techniques
IMPORTANT POINTS ABOUT COMPLEX SYSTEMS
Organisa� onal level
• Complex systems is a theory in general science and has been applied in other fields as well. • They are dynamic, open systems with distinctive characteristics.
• It is more than complicated or complex. It is a way of thinking and understanding the systems that we are living in. • It is challenging to analysis using a complex systems approach. Analytical techniques and machine learning model may be helpful in solving the problems.
1. Bittencourt NFN, Meeuwisse WH, Mendonça LD, NettelAguirre A, Ocarino JM, Fonseca ST. Complex systems approach for sports injuries: moving from risk factor identification to injury pattern recognition—narrative review and new concept. British Journal of Sports Medicine. 2016;50(21):1309-14. 2. Bertalanffy LV. General System Theory: Foundations, Development, Applications. New York, USA: George Braziller Inc; 1969. 3. Philippe P, Mansi O. Nonlinearity in the Epidemiology of Complex Health and Disease Processes. Theoretical Medicine and Bioethics. 1998 December 01;19(6):591-607. 4. Von Bertalanffy L. The Theory of Open Systems in Physics and Biology. Science. 1950;111(2872):23-9. 5. Philippe P, Garcia MR, West BJ. Evidence of ‘‘essential uncertainty’’ in emergency-ward length of stay. Fractals. 2004;12(02):197-209. 6. Rickles D, Hawe P, Shiell A. A simple guide to chaos and complexity. Journal of Epidemiology and Community Health. 2007;61(11):933-7. 7. Joanna B, Alex P, Pete B-J, Martha B, Dione H. The visual representation of complexity: Definitions, examples & learning points. RSD7, Relating Systems Thinking and Design 7; 2018 23-26 Oct 2018; Turin, Italy; 2018. 8. Yung KK, Ardern CL, Serpiello FR, Robertson S. Characteristics of Complex Systems in Sports Injury Rehabilitation: Examples and Implications for Practice. Sports Medicine - Open. 2022 2022/02/22;8(1):24. 9. Ruddy J, Maniar N, Cormack S, Timmins R, Opar D. Predictive modelling of non-contact lower limb injuries in elite Australian footballers. Journal of Science and Medicine in Sport. 2018;21:S18. 10. Claudino JG, Capanema DdO, de Souza TV, Serrão JC, Machado Pereira AC, Nassis GP. Current Approaches to the Use of Artificial Intelligence for Injury Risk Assessment and Performance Prediction in Team Sports: a Systematic Review. Sports Medicine - Open. 2019 July 03;5(1):28. 11. Alessio Rossi LP, Paolo Cintia, F. Marcello Iaia, Javier Fernàndez, Daniel Medina. Effective injury forecasting in soccer with GPS training data and machine learning. PLoS ONE. 2019;13(7). 12. Cust EE, Sweeting AJ, Ball K, Robertson S. Machine and deep learning for sport-specific movement recognition: a systematic review of model development and performance. Journal of Sports Sciences. 2019 2019/03/04;37(5):568-600. 13. Fältström A, Kvist J, Bittencourt NFN, Mendonça LD, Hägglund M. Clinical Risk Profile for a Second Anterior Cruciate Ligament Injury in Female Soccer Players After Anterior Cruciate Ligament Reconstruction. The American Journal of Sports Medicine.0(0):0363546521999109.