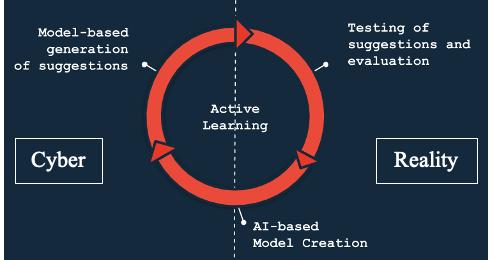
4 minute read
Rapid 3D Printer Settings Development Using AI
Matthias Kaiser, Exponential Technologies Ltd, www.x-t.ai Source: Andrew Kostryzhev, Queensland Branch of Materials Australia
In additive manufacturing (AM), and particularly in laser powder bed fusion (L-PBF), determination of a correct combination of printing parameters is a highly complex task. Interactions between the machine and the material are complicated and involve many different disciplines, such as metallurgy, laser science, mechanical engineering and many more.
As such, the development of AM technology for new, and even existing, materials becomes extremely time and cost intensive. This is why AM can offer only a small number of processable materials when compared to classical manufacturing methods. For AM to become a truly industrial technology, hundreds of new materials will have to be made printable over the next years. The artificial intelligence (AI) tool xT SAAM newly developed by Exponential Technologies Ltd (EU), is designed to help facilitate the research and development process, and significantly speed up transition of ideas, processes and products from laboratory to industry. xT SAAM is based on specially-tailored small data and active learning algorithms that are highly versatile and resilient. This allows application of the software in a wide range of material chemistry and technology development situations without any reprogramming. This makes it far more flexible than other Design of Experiments (DoE) and AI solutions on the market. The xT SAAM software can be used in a DoE-like iterative format or in a predictive mode, when the software uses existing data sets to build predictive models of the parameter interactions. However, it is most often used in a hybrid approach, when the existing limited data sets are used as a starting point for a new experimental campaign. With the integrated JSON API cloud storage, xT SAAM can be connected to any machine, database or software application. This allows for the full automation of the analysis and optimisation process. The built-in Python scripting engine enables the development of custom routines and workflows. Titanium alloys such as Ti-6Al-4V and others are used in a variety of industries including aviation, automotive, space, and others. Due to its light weight but high strength, Titanium is the perfect material in applications with high mechanical requirements under weight restrictions. This makes Titanium and AM a perfect match. If performed correctly, AM has the ability to decrease weight of printed parts while at the same time maintaining or even improving mechanical properties. Additionally, AM allows the integration of cooling channels within the walls of the end part, making it ideal for applications in engines and propulsion systems. However, in order to produce Ti alloy end parts for aviation, automotive or space applications using the AM process, the part quality has to be high. More importantly, the production results have to be very consistent. This is often a problem with AM as 3D printing processes in general, but especially L-PBF, are extremely sensitive to even minor changes in processing parameters or environmental factors. To ensure the consistent production of high quality end parts, the printer parameters have to be optimised for specific applications. A pilot project with a German L-PBF machine manufacturer aimed to optimise the printing parameters for alloy Ti-6Al-4V to achieve the highest possible density while maintaining high production speeds. The optimisation was carried out for five technological parameters: laser power, scan speed, hatch distance, stripe width, and stripe overlap. The layer thickness was kept constant. To avoid too many failed prints, a search constraint was added in the form of an approximated energy density function that included the laser power, layer thickness, scanning speed and hatch distance. In this way, prints in which the material did not fuse at all or burn could be avoided from the start. As optimisation objectives, the following were defined: the maximum pore size and their number density, solid material density, build rate, and a subjective expert opinion. The expert opinion was introduced through the observation of the printing process by an expert carrying out in-situ assessment of the melt pool and the component microstructure after printing and cutting. However, there was no objective measurement available that could identify these problems consistently. The budget for the experimentation was fixed to six iterations with 20 density cubes each.
The best performing parameter set was successfully found during the fourth iteration. This parameter set resulted in a significantly reduced pore size, increased build rate (~5%) and a slightly increased part density, when compared to the company’s previously developed parameter sets. Surprisingly, at least three parameter sets with very different values of each parameter were found, leading to extremely similar end part properties. Having options is particularly important in industrial conditions, because the probability that an optimised parameter set results in unstable production process is high. A possibility to manufacture the end components with similar characteristics using vastly different sets of processing parameters facilitates the technology development and allows faster introduction of the product to market. In this project with a German L-PBF machine manufacturer, it was possible to demonstrate that the solution found significantly reduced the time and cost of AM technology development. Finding reliable manufacturing parameters with only 80 density cubes is an extremely fast research and development process—the standard procedure typically requires several hundreds of density cubes, can take several years, and cost millions.
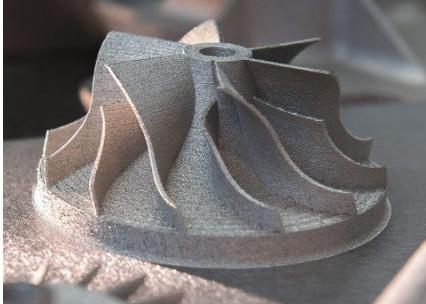