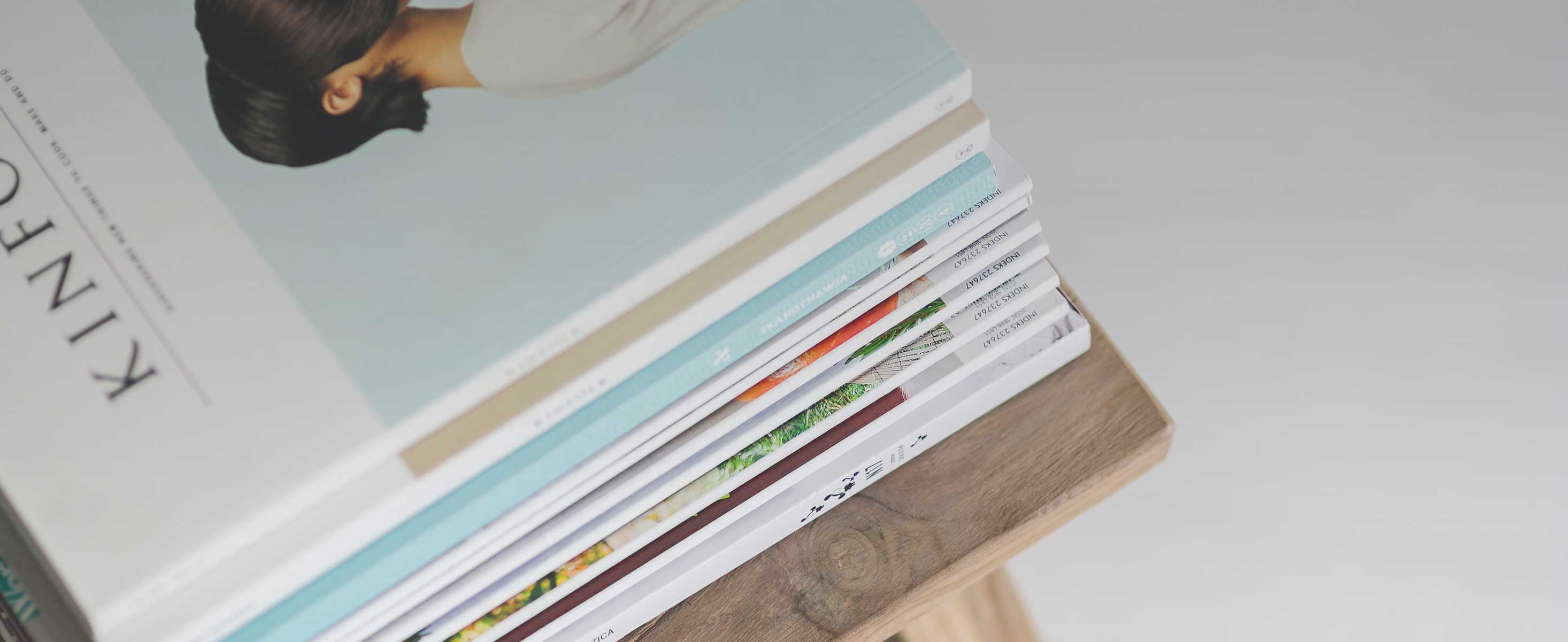
6 minute read
Machine learning at scale will be the norm
Engineering ranks said to embrace the emerging discipline
By Kevin Parker
Advertisement
In September, Baker Hughes Co. and C3.ai launched BHC3 Reliability, the first artificial intelligence (AI) software application developed by the BakerHughesC3.ai joint venture. More recently, Baker Hughes, C3.ai and
Microsoft in November announced an alliance to make adoption of advanced analytics easier by bringing together cloud infrastructure, an AI platform and domain-specific applications.
“Use of analytics, machine learning and artificial intelligence is not new to the oil & gas industries. But today, we’ve reached an inflection point, to deploy these technologies at scale,” said Dan Brennan, SVP & COO, BakerHughesC3.ai.
Baker Hughes is a nearly $23 billion provider of integrated oilfield products, services and digital solutions. C3.ai is an AI software provider. The core of the C3.ai offering is a model-driven AI architecture that enhances data science and application development. In June the two companies announced a joint venture to combine Baker Hughes expertise with C3.ai’s AI software suite, for application in the oil & gas industry.
BHC3 Reliability machine-learning models identify anomalous conditions that lead to equipment failure and process upsets. Application alerts enable proactive action by operators.
BHC3 Reliability can scale to assets and processes across offshore and onshore platforms, compressor stations, refineries, and petrochemical plants, reducing downtime and increasing productivity.
“If you look at the not-too-distant past,” Brennan said, “a lot of investment went into automating facilities to enable things like condition monitoring to reduce non-productive time. Extensive use was made of physics-based and rules-based models to better understand equipment operation. Therefore, we’re at a different point of maturity today, where more than 40% of unplanned downtime originates from non-critical equipment, which even today is not highly instrumented.“
Things and kinds of things
The kinds of analytics include principles-driven and data-driven, with two kinds of each. Physics-based analytics incorporate the physical and thermodynamics laws of how things work, while rules-based analysis is a kind of principles-driven analysis based on observation and domain expertise, including failure mode effect analysis (FMEA).
On the other hand, data-driven analytics include, first, statistical models based on techniques like linear and other type regression, and second, advanced analytics that include artificial intelligence and machine learning, often based on pattern recognition.
Roughly put, the domain of principles-driven analytics are the pumps, heat exchangers and myriad other equipment types whose workings are well understood by the engineering community. Root-cause analyses are often part of the picture.
On the other hand, the realm of machine learning and artificial intelligence is the complex problems and to-be-discovered challenges native to process, plantwide and enterprise systems, where custom configurations of complex systems lead to unknowns.
Thus, while physics-based models still play a crucial role, Brennan said, that’s being augmented by a data-based approach, to better understand, for example, what a normal operating range is, or, drilling down in detail, to better understand the correlations involved. But while machine learning may uncover otherwise unrecognized correlations, it’s not necessarily able to recognize cause-and-effect relationships.
One aspect of scale is the data amounts involved. A machine learning model can exist for any given valve or pump, and for every instance of it, which may involve hundreds or even thousands of instances, or it may be looking at a system of systems.
“The models may be used to better understand how equipment performance correlated with past events, such as changes in operating conditions like a change of feed stocks. In many instances, what’s important is that the users are gaining a unified, federated view of the data. They’re gaining visibility into a data set that they would otherwise struggle to get their arms around,” said Brennan.
The human dimension
One issue often part of discussions related to machine learning and artificial intelligence is the relationship between domain expertise that the engineering community brings to bear and the work of data engineers and data scientists who better understand the ideal world of mathematical statistics.
“What you want to avoid is a polarized view,” Brennan said. “Bear in mind that physics-based models still play an important role here and the background the engineering community commands is critical to success. There is a certain ‘dialect’ spoken by those in the oil & gas industries.”
What’s most wanted today in these advanced technology endeavors is compressed time to value, said Brennan. “You don’t want to spend 60 days explaining the vocabulary to the uninitiated. It’s the engineers who can quickly determine how to model a process based on the probability or consequences of failure.”
An example of compressed time to value, notes Brennan, is that Reliability was launched within 90 days of Baker Hughes announcing the partnership. “When you put the two kinds of people together things accelerate.”
Moreover, engineers are exhibiting real interest in involvement in advanced analytics efforts. “Fifteen years ago there was no such thing a college-degree major in data science. Today there is. However, it’s interesting the large number of engineers’ mid-career interested in engaging in data science-related work. For the younger engineers just embarking on careers, providing access to these types of tools and systems is a good recruiting tool. This includes our relationship with Nvidia [developer of graphical processing units], which has been a good draw and a compelling proposition,” Brennan said.
Connelly said that Baker Hughes is pleased that 50 of its employees have gone through C3 training. “We’re deploying them in areas as diverse as inventory management and receivables and collectibles. We’ve been a little surprised at the sheer volume of demand for resources to deploy. What concerns us amidst this activity is forging the right governance model for command and control.”
Finally, application development is moving to low-code or no-code environments.
To date, one challenge with machine learning is moving something developed in the lab to operations scale, especially when operations change rapidly.
“You do encounter citizen data scientists building out analytics,” Brennan said.
Adding Microsoft and its cloud platform, Azure, into the mix is another means to speed time to value. Microsoft platforms already occupy a substantial footprint in industrial enterprises. Besides Microsoft’s data center expertise, Azure makes available microservices that including Power BI embedded in Azure for data set visualization; Azure Kubernates Service for containerization and moving legacy applications to the cloud; and Cognitive ML services.
Other advancing technologies
The Baker Hughes news release announcing availability of BHC3 Reliability mentions several other technologies as bearing on the company’s efforts related to machine learning use in oil & gas industries.
Avitas Systems provides solutions for achieving asset integrity management through robotics inspection, including the use of drones, and analytics that incorporate computer vision, machine learning and physics-based modeling.
In just one example of the techniques involved, machine vision can be applied to photo-realistic models made from RGB and IR images captured using drones, such as of a refinery. Computer vision techniques can be used to associate image and video findings back to an asset’s 3D model, to detect anomalies.
Natural language processing comes into play when one of the inputs into a machine learning model are maintenance records, constituting a species of unstructured data, generated by humans in natural languages such as English.OG