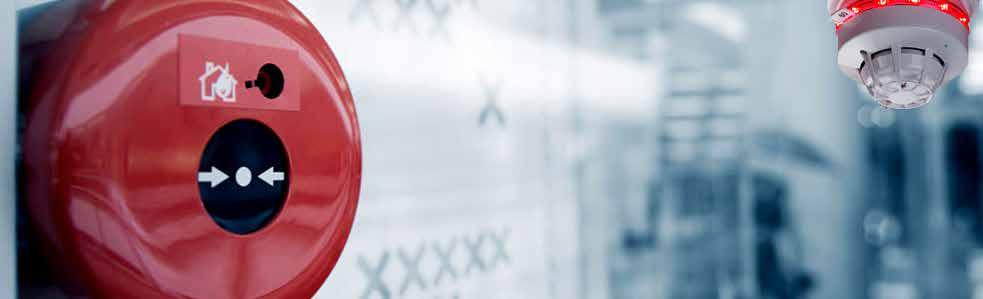
2 minute read
RESEARCHERS USE MACHINE LEARNING FOR EARLY FIRE WARNING
from The 6th Issue of the Innovation @UAE Magazine: Advancing Humanity (Through Science and Technology)
A researcher from the United Arab Emirates University (UAEU) has been working on the UAE’s green mobility by focusing on optimal fast charging stations planning in preparation for the future high penetration of electric vehicles (EV) in the UAE.
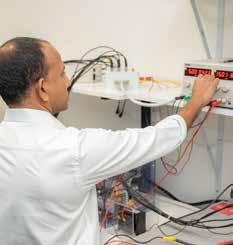
Advertisement
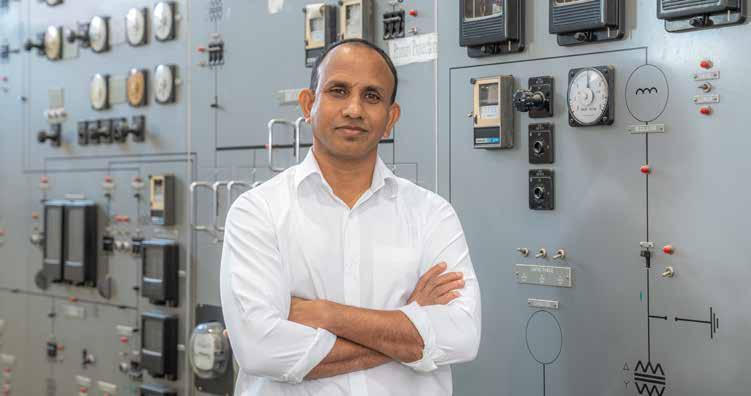
Dr. Hussain Shareef, Professor of Electrical and Communication Engineering at the University, has been concentrating his work on optimum locations for fast charging stations across the country to reverse the current low penetration due to a lack of infrastructure available. Such work is crucial as an increase in charging stations will inevitably lead to cost-effectiveness for each station erection going forward.
Dr. Shareef’s project, in collaboration with the Emirates Centre for Transportation, also tackles users’ satisfaction by reducing their waiting time, pinpointing locations with stability and minimal impact on the power grid. His research is timely and significant, considering the UAE’s Energy Strategy 2050, which aims to increase the contribution of clean energy in the total mix from 25 to 50 percent by 2050 and reduce carbon footprint of power generation by 70 percent, saving Dh700 billion. Depleting fossil fuel reserves and rising petrol prices represent other incentives for the importance of such work.
Dr. Shareef believes more than 40 percent of vehicles in the UAE will be electric by 2050, as the infrastructure in the country has already begun developing. Currently, the UAEU possesses a few free EV charging stations that serve as an incentive and a motivation for students and faculty to increasingly adopt green mobility.
Reference Link: https://www.uaeu.ac.ae/en/research/research-highlights/optimal-fast-charging-stations-planning-for-future-high-penetratio-of-electric-vehicle.shtml
Indoor fire disasters cause severe property damage and cost human lives. Yet although commercial fire alarms are effective in detecting flaming fires, they cannot detect the presence of smoldering fires, which develop very slowly and do not emit much heat when compared to flaming fires. As a result, by the time a fire alarm detects them, they are already out of control, having caused severe damage. This is the field of research undertaken by Dr. Mohammad Nazir, Associate Professor at the College of Technological Innovation at Zayed University, in his paper entitled “Early fire detection: a new indoor laboratory dataset and data distribution analysis”. By focusing on machine learning, he addressed the issue at hand. “Today, video scenes from a surveillance camera are being used in almost all advanced fire detection systems to solve this issue. However, surveillance cameras breach people’s privacy and are very costly to deploy and maintain. Surprisingly, very few researchers and scientists really have explored how to analyse chemical properties such as humidity, temperature, MQ139, Total Volatile Organic Compounds (TVOC) and eCO2 to detect smoldering fires,” he explained. Upon further investigation, Nazir and his team observed that this is due to the limited data available on indoor fire scenarios. As a result, and for the development of future machine learning-based fire warning systems, machine learning practitioners urgently need a complete fire experiments dataset that covers fire types and scenarios of flammable materials commonly found in homes and offices. To address this issue, the team gathered important chemical data from controlled realistic fire experiments conducted in an indoor laboratory environment. The experiments were conducted in a controlled manner by triggering the source of fire using electrical devices and charcoal on paperboard, cardboard or clothing. In fact, these researchers were the first to collect such data, and, to date, their publicly available dataset has been used by machine learning researchers around the world to develop their fire detection systems. The project was also supported by EXPO 2020, Dubai, through EXPO Live Agreement EL-RA013-. In the future, the team plans to create additional experiments to include various other fire scenarios and the addition of sensors, such as LPG gas leakage sensors. Expanding its datasets will help machine learning practitioners develop more accurate and robust early warning fire detection systems. “One day, we hope that the fire warning systems will be so accurate and sophisticated that we will no longer hear any news on the loss of human lives or severe property damage from fire disasters,” Nazir concluded.