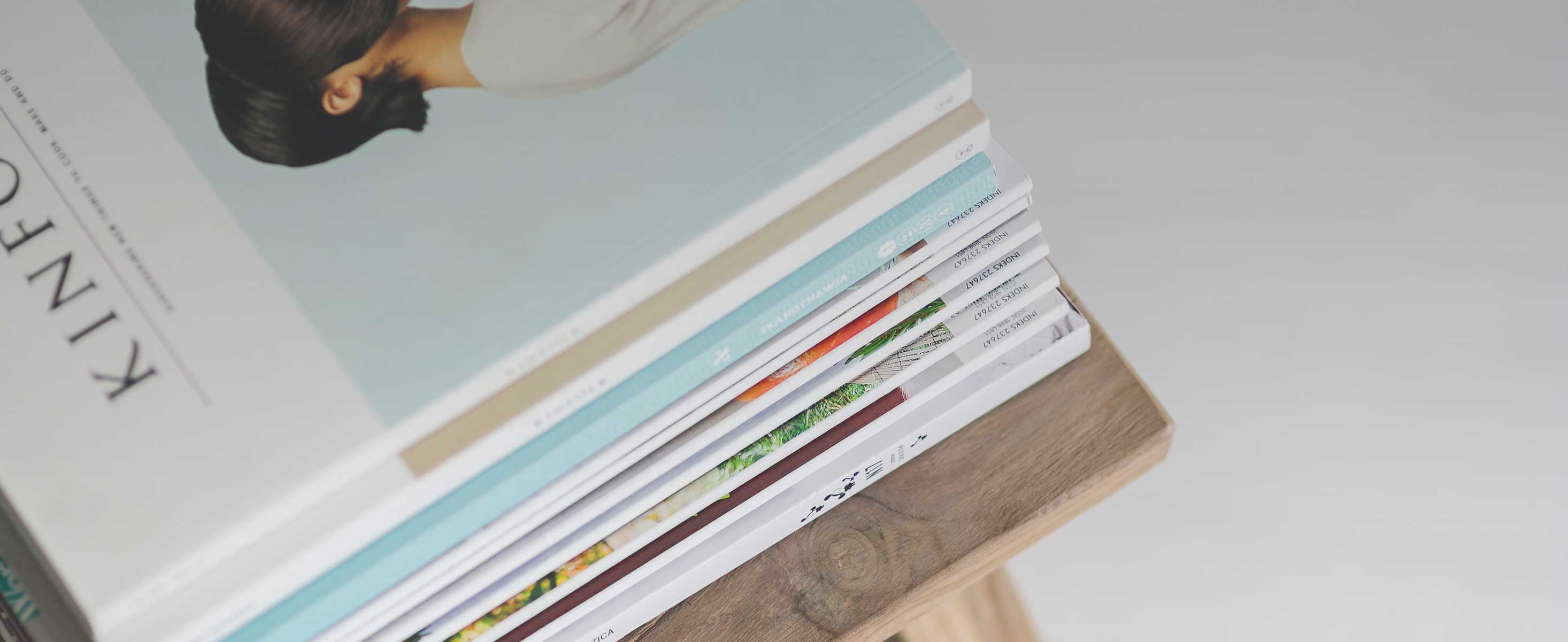
3 minute read
MACHINE LEARNING REACHES NEW HEIGHTS IN DATA PRIVACY
from The 6th Issue of the Innovation @UAE Magazine: Advancing Humanity (Through Science and Technology)
In today’s world, in which power systems are continuously being upgraded by integrating renewable energy sources (RES) to reduce the carbon footprint, the need for prediction algorithms has become all the more vital. Accurate prediction algorithms have increasingly proven beneficial in estimating stochastic processes, such as the weather, the stock market, and energy, among others. These accurate predictions can be utilised in a multitude of ways, namely, to reduce loss due to uncertainty. This critical work is being undertaken by a team of researchers, including Dhananjay Kumar, H. D. Mathur and S. Bhanot, alongside Ramesh C. Bansal, professor in the Electrical Engineering Department at the College of Engineering at the University of Sharjah. Through their project, “Forecasting of Solar and Wind Power using Long Short-Term Memory Recurrent Neural Networks (LSTM RNN) for Load
Frequency Control in an Isolated Microgrid”, the team focuses on ensuring the stability of modern power systems, including RES, such as solar and wind. If left unmonitored, these sources become highly unreliable due to a heightened level of uncertainty. Poor inertia also makes them highly sensitive to power system disturbances, such as a sudden rise or fall in supply or demand. In addition, the increased penetration of RES on a daily basis renders the existing grid all the more sensitive to disturbances from such sources.
Advertisement
The sudden rise or fall in the generating capacity of solar or wind power plants may create frequency instability in the power system and lead to a cumulative blackout. As a result, accurate monitoring becomes critical to ensuring the frequency stability of supply or demand in microgrids. This entails searching for an efficient prediction algorithm. The team’s work, published in the International Journal of Modelling and Simulation, proposed a forecasting method, the LSTM RNN, based on deep learning. Such a method more accurately predicts the renewable power coming from RES when compared to other reported methods. Consequently, the predicted power from solar and wind are fed into a microgrid inertial model, and the frequency response against load rise or fall is recorded. Such cutting-edge research is vital for the future, where maintaining the stability of a power system will become of great concern. As the inertia of power networks is expected to be too low to remain stable on its own, the role of forecasting algorithms based upon neural networks will play a crucial role in monitoring and controlling secondary or backup sources of energy. Several organisations have already started procuring their own microgrids to reduce their dependency on the main grid – within such conditions, the forecasting of RES in isolated microgrids becomes paramount for voltage control and frequency stability.
A new branch of machine learning is being explored by a professor in the UAE through his research on Federated Learning , also known as collaborative learning. In his study, entitled “How can we progress the machine learning field if we might lose access to data due to privacy requirements?”, Dr. Samuel Horvath, Assistant Professor of Machine Learning at the Mohamed bin Zayed University of Artificial Intelligence (MBZUAI), focuses on machine learning models that are collaboratively trained in a decentralised fashion by many clients, such as mobile devices or whole companies. Within this realm, data is never transferred nor exchanged, it is kept locally, while the learning objective is meant to be achieved using focused updates intended for immediate aggregation.Currently, the dominant paradigms in machine learning are centralised techniques where all the local clients’ datasets are uploaded to one server. Such a process carries a severe disadvantage to it as it creates negative client experience due to the privacy implications of this ever-increasing centralised data collection. In addition, several government entities have already enforced privacy policies to protect users’ data, such as the GDPR (General Data Protection Regulation) in the European Union. “These government regulations and users’ reluctance to share their private data will eventually lead to the limited availability of data, breaking one of the core pillars behind machine learning success. This is where Federated Learning comes to help us by bringing training to the edge, with decentralised clients, enabling collaborative training by multiple actors to build a common, robust machine learning model without sharing data, thus allowing us to address these critical privacy and data-access issues,” Dr. Horvath explained.
Federated Learning has already been used in several commercial applications, such as Apple’s ‘Hey Siri’ and QuickType, or Google’s ‘Hey Google’ and Gboard. On the other hand, the concept is still relatively new, with more to come in the future. Ultimately, Dr. Horvath hopes that Federated Learning will revolutionise areas where data privacy is the priority. A few examples he shared include applications such as smart health or fintech, where, historically, there would
Reference Link: https://mbzuai.ac.ae/news/powerful-predictions-and-privacy/ be no access to large data corpora due to data-sharing issues. As a new paradigm in machine learning, Federated Learning brings with it specific challenges which differentiate it from standard centralised machine learning. In particular, an essential aspect Dr. Horvath is actively researching, is reducing the communication burden during the Federated Learning process. Another critical challenge includes clients’ system heterogeneity, such as the network connection speed, bandwidth, storage and computational capacity. The challenge also encompasses statistical heterogeneities,like non-identical data distributions across the federated network, that introduce obstacles to designing efficient architectures and training or optimisation methods for Federated Learning. Finally, privacy is also a major concern in Federated Learning applications, where one of the critical research directions is to design efficient methods with formal privacy guarantees. In his research, Dr. Horvath aims to overcome some of these issues with a unified goal of enabling efficient collaborative machine learning without centralised data.
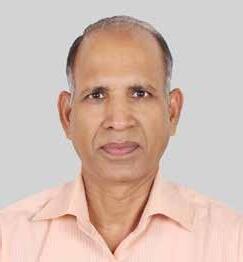