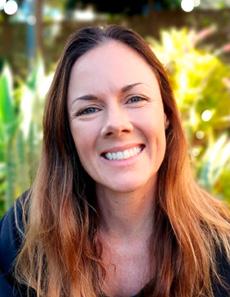
25 minute read
Features
CROSSBREEDING ARABIAN GULF CORALS TO ENHANCE CORAL HEAT TOLERANCE
Certain reef-building corals from the Arabian Gulf may provide other corals with the gene variants needed to survive rising sea temperatures caused by global climate change.
Advertisement
The Arabian Gulf is the hottest region where reef-building corals live, with coral populations adapted to high temperatures from global climate change that are predicted to hit tropical reefs at the end of this century. This provides scientists with the opportunity to leverage the heat-tolerant gene variants of local reef-building corals to enhance the resilience of other coral populations.
Coral reefs are large underwater structures built by colonies of marine invertebrates called coral. Reef-building corals live in symbiosis with microscopic algae, which work together to extract calcium from seawater to create their hard skeletons. Coral reefs play an important role in biodiversity and human life, absorbing wave energy to protect coastlines from erosion, shielding mangrove forests and seagrass beds that serve as fish nurseries, and hosting more than a quarter of all marine fish species, including several culturally and commercially important ones.
Corals are also extremely vulnerable to ongoing environmental changes, including rising sea levels, ocean acidification, and most notably, increased water temperatures, all of which are triggered by increasing greenhouse gas emissions from human activity. The most destructive and rapid impact on coral reefs from global climate change is the bleaching and dieoffs of corals when sea temperatures are abnormally warm.
Coral bleaching occurs when the sea temperature rises by just 1-2°C over the course of a few weeks, causing coral to become stressed and expel the microscopic algae they contain. Those algae live within the coral in a mutually beneficial relationship, enabling each other to survive. If a coral is without its algae inhabitants for a prolonged period of time, it will starve and die.
As average sea temperatures have been increasing, so too have coral bleaching events. Between 2014 and 2017, it was estimated that around 75% of tropical coral reefs experienced enough heat-stress to trigger bleaching. With a recent International Panel on Climate Change report warning that global climate may rise beyond 1.5°C in the next decades, enhancing the heat tolerance of corals may assist them to adapt to the increasing temperatures.
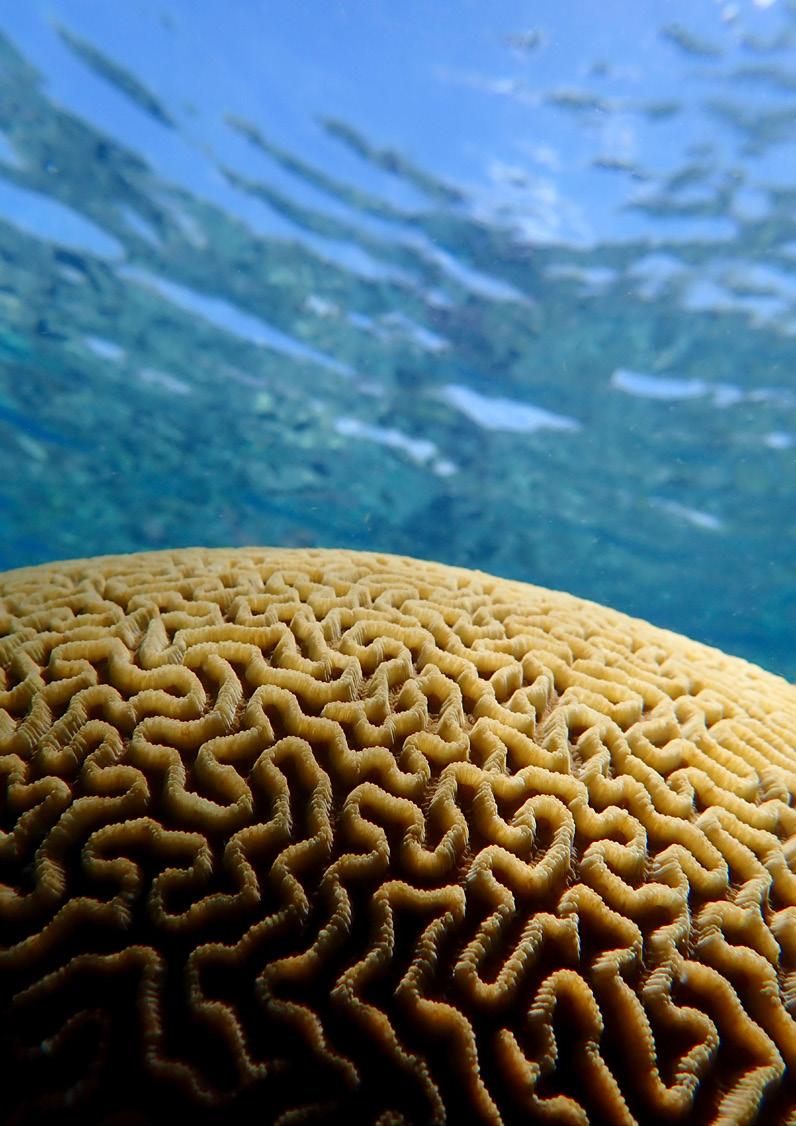
Dr. Emily Howells
Senior lecturer, Southern Cross University
“Reef-building corals are in global decline from the impacts of climate change and their future persistence is dependent on adaptation keeping up with the rapid rate of ocean warming. We had previously demonstrated that corals living on the hottest reefs in the world in the southern Arabian Gulf have genetically adapted to this extreme environment, where the water temperature reaches as high as 36°C, so for this study we wanted to test whether we could transfer their beneficial genetic variants for heat tolerance to corals living elsewhere,” explained Dr. Emily Howells, who was a postdoctoral researcher at New York University Abu Dhabi (NYUAD) during the course of the project and is currently a senior lecturer at Southern Cross University in Australia.
Dr. Howells was the lead author of a paper on the project titled “Enhancing the heat tolerance of reef-building corals to future warming,” which was recently published in the leading journal Science Advances. Her co-authors included former Zayed University Associate Professor of Natural and Health Sciences Dr. David Abrego, NYUAD Associate Professor of Biology Dr. John A. Burt, Commonwealth Scientific and Industrial Research Organization research scientist Dr. Yi Jin Liew, Oregon State University Assistant Professor of Biology Dr. Eli Meyer, and King Abdullah University of Science and Technology Associate Professor of Marine Science Dr. Manuel Aranda.
The project had four broad phases. The first phase focused on study sites and coral breeding. The team collected fragments of Platygyra daedalea coral colonies from specific sites off Abu Dhabi and Fujairah, where the average sea temperatures fluctuate by 3°C in the summer. The fragments were housed and spawned in controlled environment aquaria at NYUAD, producing families of regional purebred and crossbred corals.
The second phase of the project was heat stress testing the coral offspring, which numbered more than 12,000 individual coral larvae, to find out if they could withstand the maximum temperatures of their parents’ respective locations.
“We found an immediate transfer of heat tolerance when coral eggs from Fujairah were crossed with coral sperm from Abu Dhabi. These crossbred corals showed an 84% increase in survival at high temperatures relative to purebred Fujairah corals, and they were as equally heat tolerant as purebred Abu Dhabi corals,” Dr. Howells said.
The third phase of the project comprised a field survival experiment to evaluate the influence of parental origin on survivorship in the field setting. Larval offspring from the coral families were settled onto tiles deployed near the Fujairah fragment collection site and monitored for one month.
“In this reef environment, we found that crossbred corals with Abu Dhabi fathers survive equally as well as native Fujairah purebred corals, but both had higher survival than non-native Abu Dhabi purebred corals,” shared Dr. Howells.
The fourth phase of the project was parent and offspring genotyping, which
investigates the genetic constitution of an organism.
“Genome sequencing confirmed that gains in heat tolerance were due to the inheritance of beneficial gene variants from the Abu Dhabi fathers. Most Abu Dhabi fathers produced offspring that were better able to withstand heat stress, and these fathers and their offspring had crucial variants associated with better heat tolerance,” Dr. Howells said.
Explaining the implications of the project’s findings, Dr. Howells said, “These results demonstrate that corals can be selectively bred for enhanced heat tolerance using corals from populations in extreme or warmer environments which have a higher proportion of heat-tolerant genetic variants due to local adaptation.”
As a next step, she said longer-term studies are needed to evaluate any possible trade-off between thermal tolerance and other important traits in the coral. Additionally, any genetic risks involved in the introduction of selectively bred corals to populations must be identified and studied.
“Selective breeding has the potential to be used to enhance the resilience of targeted coral populations to climate warming but requires further testing before it can be implemented in intervention and restoration programs. However, the most important actions to enhance the resilience of all coral populations are those that limit the magnitude of climate change and other pressures,” Dr. Howells clarified, emphasizing the need for continued global climate change mitigation efforts. Title of published paper Enhancing the heat tolerance of reefbuilding corals to future warming
Published in Science Advances
Journal metrics Impact Factor: 14.14, Q1, H-index: 215, Scientific Journal Ranking (SJR): 5.923
Project funded by National Geographic Society grant (awarded to Dr. Emily Howells) and a grant from Tamkeen (awarded to Dr. John Burt), with support from the High-Performance Computing Center and Marine Biology Core Technology Platform at NYUAD
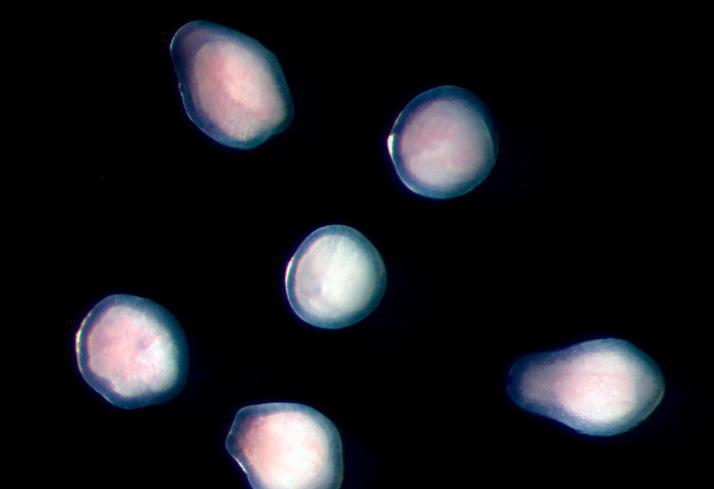
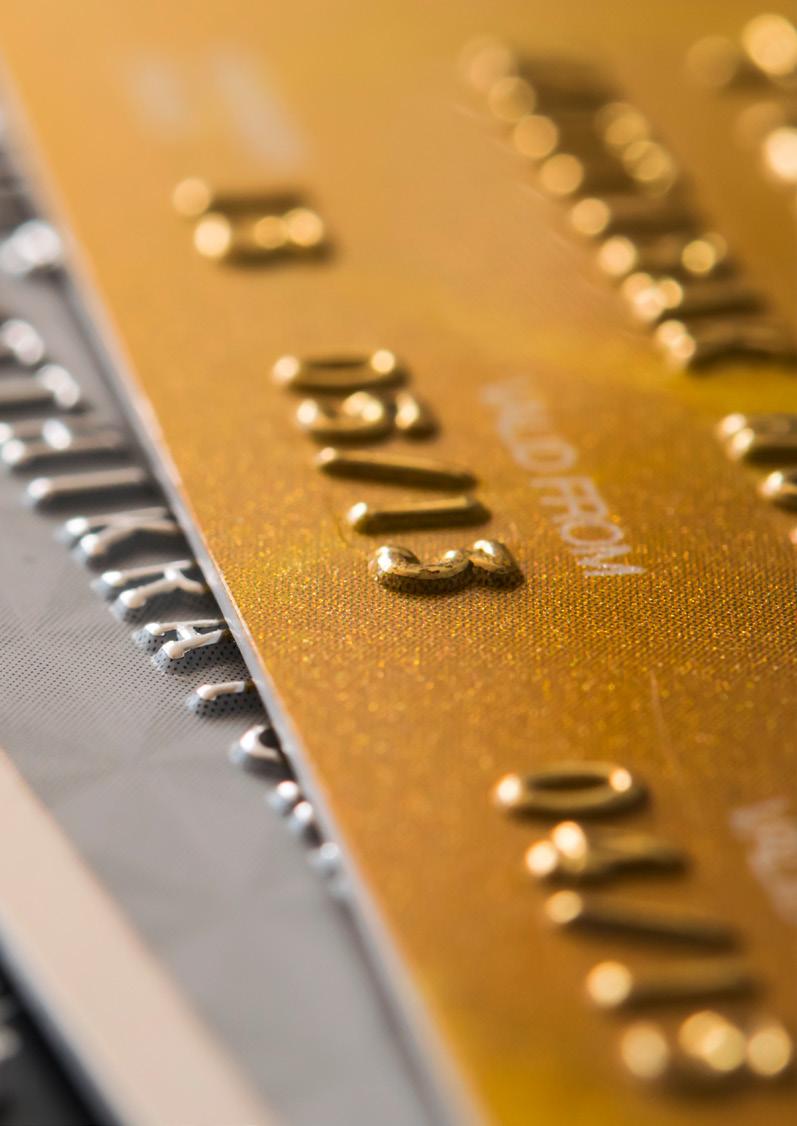
PREDICTING CREDIT CARD CUSTOMER BEHAVIOR USING MACHINE LEARNINGBASED MODELING
Banks today have vast databases that can be mined to analyze performance and even predict customer behavior. A Zayed University-led research team has developed a model that leverages machine learning and credit card customer data to predict more accurately how quickly a customer will repay the amount on their credit card.
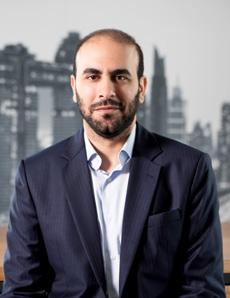
Managing risk is crucial to banks. They must quickly identify which customers are a bad risk and need to be cut off from taking on more debt, and which customers can continue to be granted credit. As credit cards represent a major proportion of bank business – and one against which no assets are secured – they present a liability that the bank must manage by careful identification of risk as either good or bad.
“Banks need to accurately and quickly predict consumer credit card default, and the best way to do that is to use advanced systems that automatically score customer behavior on credit card repayments. We believe behavioral scoring models that leverage the power of machine learning can better enable banks to make risk decisions and financial security decisions to reduce their losses,” explained Dr. Maher Ala’raj, Assistant Professor in the Department of Information Systems in the College of Technological Innovation (CTI) at Zayed University (ZU).
The team, which also comprised Dr. Maysam Abbod, Reader at the Department of Electronic and Computer Engineering at Brunel University London, and Dr. Munir Majdalawieh, an Associate Professor at the Department of Information Systems at the CTI ZU, developed a model for credit card customer repayment probability using behavioral scoring.
Behavioral scoring is a common method of analysis to predict a customer’s likelihood to default during a specific period. The strength of the prediction is often impaired by its subjective parameter choices, like outcome period and performance period, while the vast volume of credit card transaction data makes it difficult to apply traditional mathematical and statistical models for behavioral scoring.
“To construct behavioral scoring models, professionals must think about a few significant issues, such as the extensiveness of the dataset to model, the planning horizon,
and drivers of unwanted behavior. The literature does not contain solid suggestions on the most proficient method to respond to these questions,” the researchers wrote in their paper on the topic that was recently published in the Journal of Big Data.
They developed a Long-Short-Term Memory (LSTM) model to enable automated credit card behavior scoring for bank customers. An LSTM is an artificial recurrent neural network architecture used in machine and deep learning to classify, process, and make predictions based on time series data. The team’s proposed LSTM model framework consisted of several steps, starting with pre-processing and
Dr. Maher Ala’raj
Assistant Professor in the Department of Information Systems in the College of Technological Innovation
Left to right: Dr. Maher Ala’raj and Dr. Munir Majdalawieh formatting the dataset using a bidirectional LSTM classifier, which looks at a particular data sequence both from front to back and from back to front. Next, a fivefold validation technique was applied to get a prediction for all customers in the dataset. Then the performance measures were calculated for different groups of customers of financial interest to the banks, like those with an unsatisfactory repayment history.
To test the accuracy of their proposed bidirectional LSTM system, a public nontransactional credit cards dataset was analyzed, after which the results were benchmarked against five standard prediction classifier models. The dataset was of Taiwanese credit card transactions and included 30,000 records, with 23,364 non-default payments and 6,636 default payments. The classifier models included Gradient Boosting, Bagging Neural Network, Support Vector Machines, Random Forest, and Logic Regression.
The proposed bidirectional LSTM model and the five classifier models were then used to analyze the Taiwanese dataset, looking at four subsets of users to determine the models’ sensitivity, specificity, accuracy, balanced accuracy, and Brier score. Sensitivity refers to the ability of the model to identify missed payments. Specificity measures the proportion of missed payments that are correctly identified. Accuracy is the simplest method of evaluating the model’s preciseness. Balanced accuracy is a metric used to assess the quality of the model when the dataset classes are imbalanced. The Brier score reflects the discriminatory power of the model, or its certainty in predicting a customer’s missed payment, with the lower the Brier score, the better the model’s performance.
The results showed that the team’s proposed model was the most sensitive at 37.51%. It was the fourth most specific, with specificity at 95.15%, however, the
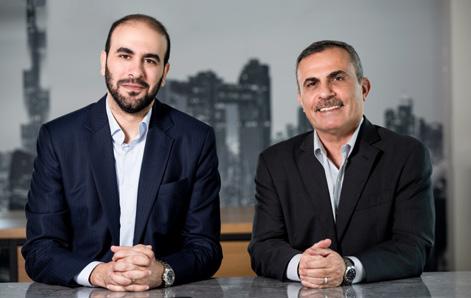
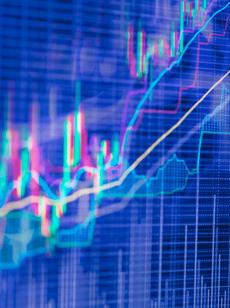
three classifier models with higher specificity had much lower sensitivity. The proposed model also had the third highest accuracy. Overall, the team’s proposed bidirectional LSTM had the highest balanced accuracy percentage and the lowest Brier score, proving the overall quality of the model.
“Our research emphasizes the importance of credit card scoring for assessing and decreasing bank losses. By conducting a detailed comparison procedure, we have proven that machine learning models such as LSTM can provide the highest accuracy in predicting late fees and missed payments,” Dr. Ala’raj said.
Given the volume of credit card customers and the potential risk they pose to banks from lost repayments, the research team asserted that the modest accuracy gain of their classifier model could lead to major savings for financial institutions.
They assert that banks could use their novel classifier model not only for its binary output to determine whether a customer will miss a payment in the next month, but also to score each client. The scores provided by the model can be used to group customers into appropriate risk groups, so the bank can offer the corresponding service and security depending on their risk. This can enable banks to efficiently assess financial risks and make financial decisions.
“Our results show that, compared with benchmark models, the LSTM neural network has significantly improved consumer credit scoring. It is up to the bank to set up the thresholds above which they would move a customer into the high- or medium-risk group with corresponding consequences to the customer, such as decreasing their credit card limit or blocking their card. Moreover, such scores can be used as missing payment probabilities, so bank management can calculate the potential losses from each customer and even whole credit portfolios,” Dr Ala’raj said.
Going forward, the team will be looking to validate their bidirectional LSTM model on other real-world banking datasets, particularly from the UAE. They hope to further prove the efficiency of their model and its ability to analyze different behaviors from credit card customers. They will also be working to extend their model to customer credit scoring for different types of loan products.
Title of published paper Modelling customers credit card behavior using bidirectional LSTM neural networks
Published in Journal of Big Data
Journal metrics Impact Factor: 11.09, Q1, H-index: 35, Scientific Journal Ranking (SJR): 1.03
Project funded by Zayed University Office of Research, Grant Number R20053
HYBRID CONVERTER SYSTEM FOR ENERGY- AND COST-EFFICIENT OFFSHORE WIND TRANSMISSION
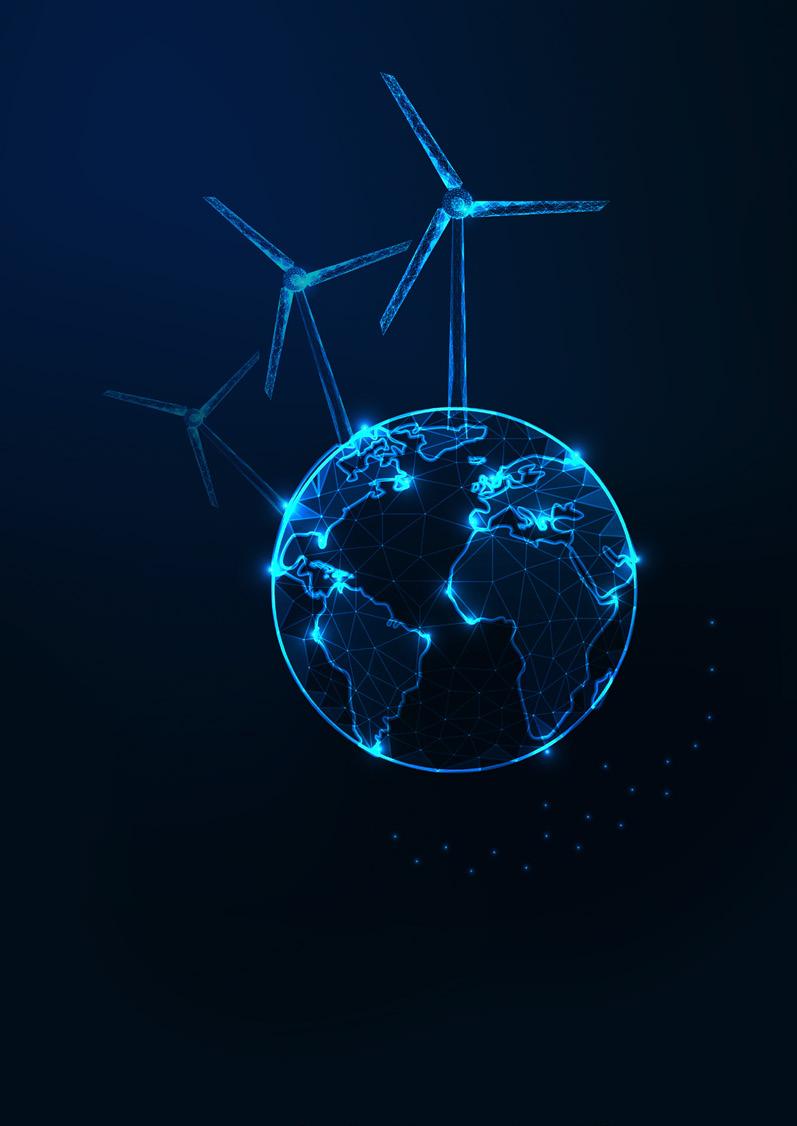
Increasing the integration of wind energy to the electricity grid requires the development of new powerconversion technologies that reduce cost and line losses to improve profitability and energy efficiency.
A Higher Colleges of Technology (HCT) led research project has proposed a new highvoltage direct current (HVDC) converter system to efficiently transmit power from offshore wind farms (OWF) to the onshore power grid.
OWFs are wind farms located on the ocean or seabed. As the speed of wind over water tends to be higher overall and more stable than wind over land, the average OWF can produce more than twice as much electricity as a mediumsized onshore farm. The International Renewable Energy Agency (IRENA) has reported that the global installed windgeneration capacity has increased by a factor of almost 75 in the past two decades, jumping from 7.5 gigawatts (GW) in 1997 to some 564 GW by 2018. The UAE has recently turned its attention to wind power, with His Highness Sheikh Mohammed bin Rashid Al Maktoum, Vice President and Prime Minister of the UAE, Ruler of Dubai, announcing plans to build the country’s first wind farm in Hatta.
Electricity can flow one of two ways. It is either one directional, which is called direct current (DC), or it can flow both backwards and forwards, which is called alternating current (AC). Electric grids supply electricity as AC, which is a legacy of the “war of the currents” waged by Thomas Edison and Nikola Tesla in the 20th century, while renewable energy (RE) systems that harness wind and solar energy produce electricity as DC power. For RE supplies to be integrated on an electric grid, they must first be transformed from DC to AC, which is done through power converters, which convert the voltage from AC to DC, and inverters, devices that invert the voltage from DC to AC.
“For OWF developers, HVDC transmission systems are an attractive way to get the energy from the windfarm to the grid, as they cost less and lose less of the electricity for transmissions over 50 kilometers. If you are using an HVDC transmission system, you need an HVDC converter to control and stabilize the operation and delivery of power between the offshore windfarm to the grid on the mainland. There are many HVDC topologies available, but each of them has its own inherent drawback. The topology we have proposed has a lower cost, loss, and volume compared to other HVDC converter systems, while still proving the fundamental operation of the HVDC system,” explained HCT Assistant Professor of Electrical Engineering Dr. Thanh Hai Nguyen. An HVDC converter system topology refers to the structure of electric circuits consisting of power electronic devices, inductors, capacitors, and transformers, which convert the voltage forms between AC and DC types.
Dr. Nguyen and his co-author, Can Tho University Electrical Engineering Department Deputy Head Dr. Ngoc Thinh Quach, recently published a paper on their hybrid HVDC converter topology in the International Journal of Electrical Power & Energy Systems.
In an OWF, a series of massive wind turbines are installed on the seabed or on floating platforms. When the wind blows, it pushes the blades of the turbine, causing the blades to spin. The blades are connected to a drive shaft, so when the blades turn, the drive shaft powers an electric generator to produce electricity. That electricity is then transmitted via a series of current conversion devices and cables to the onshore electric grid, where it can contribute to the available electricity supply.
To transmit the electricity produced by the OWFs to the electrical grid, an electricity transmission system is required. Transmission systems based on HVDC have been attracting attention from the electric industry due to the reduced line losses and lower costs they offer compared to the AC-based alternative. The two main ways HVDC topologies integrate OWFs to the main grid are line-
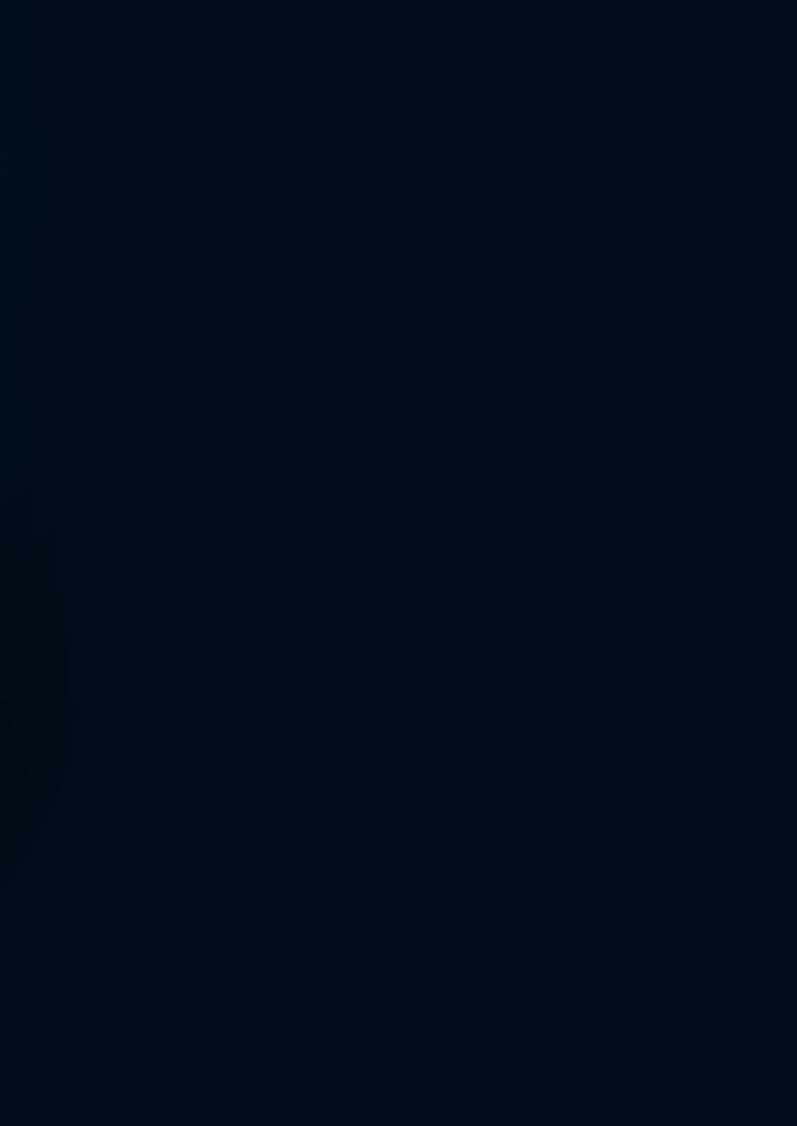
OFFSHORE WIND FARMS (OWF) ARE WIND FARMS LOCATED ON THE OCEAN OR SEABED, AS THE SPEED OF WIND OVER WATER TENDS TO BE HIGHER OVERALL AND MORE STABLE THAN WIND OVER LAND. THIS ENABLES THE AVERAGE OWF TO PRODUCE OVER DOUBLE THE ELECTRICITY OF A MEDIUMSIZED ONSHORE FARM
communicated converter (LCC) based and voltage-source converter (VSC) based. Both have documented limitations.
The system proposed by Dr. Nguyen and his team consists of a full-scale modular multilevel converter (M2C) at the grid site, and a hybrid scheme of a reduced rating M2C and 12-pulse diode rectifier (12PD) without DC capacitors in the offshore station. The HVDC transmission system would deliver the power from the OWF to the grid, where the M2C located in the onshore grid is used to maintain the HVDC link voltage without change, enabling the power delivered from the offshore converter to be fully transmitted to the main grid.
Explaining the benefits of their proposed system, Dr. Nguyen said, “The most surprising finding from this work is about the converter itself, which combines the diode rectifiers and the voltage-source converter. The diode rectifiers also don’t need DC capacitors, which are known to be costly and prone to breakdown. Without the DC capacitors, our converter system has a lower cost, loss, and volume compared to other existing topologies.”
The team has tested the feasibility of their proposed topology through simulations. They also assembled a smaller prototype, which has demonstrated the basic operations of the system.
Explaining the value of the system within the UAE, Dr. Nguyen presented examples of where it can be used, as well as how it can be adapted to other power systems.
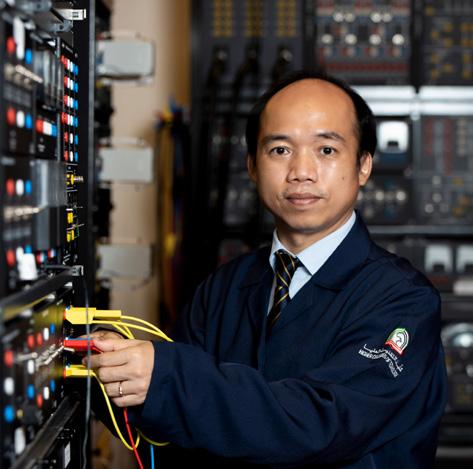
Dr. Thanh Hai Nguyen
Assistant Professor of Electrical Engineering Higher Colleges of Technology
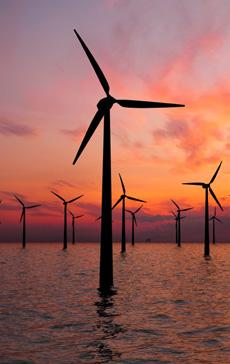
“I believe that this topology can be applied to some systems in the UAE. As the HVDC system is used to deliver power over a long distance, this topology is suggested for power transmission from offshore windfarms. In the UAE, there are many offshore oil platforms that require power from the mainland. Our topology can be modified to transmit the power from mainland to the oil platforms on the offshore side. In addition, the converter can be modified to apply in other applications such as photovoltaic (PV) power plants, machine drives, or battery charging systems,” Dr. Nguyen revealed.
Clean energy is a major focus of efforts to meet the UAE’s Energy Strategy 2050 goals, which include increasing the contribution of clean energy in the total energy mix from 25% to 50% by 2050 and reducing the carbon footprint of power generation by 70%.
To fully capitalize on the potential of the project’s findings, Dr. Nguyen will be exploring modifications and improvements to the novel topology for both high voltage and low voltage applications.
Title of published paper A hybrid HVDC converter based on M2C and diode rectifiers without DC capacitors for offshore wind farm integration
Published in International Journal of Electrical Power & Energy Systems
Journal metrics Impact Factor: 4.63, Q1, H-index: 130, Scientific Journal Ranking (SJR): 1.01
Project funded by Unfunded
OPTIMIZING PHOTOVOLTAIC PANEL CLEANING FOR MAXIMUM GAINS
Dust accumulation is a significant issue for photovoltaic farms, particularly in the Gulf region. An American University of Sharjah project leverages stochastic models to determine the ideal dust cleaning schedule to ensure it does not interfere with the farms’ operational efficiency.
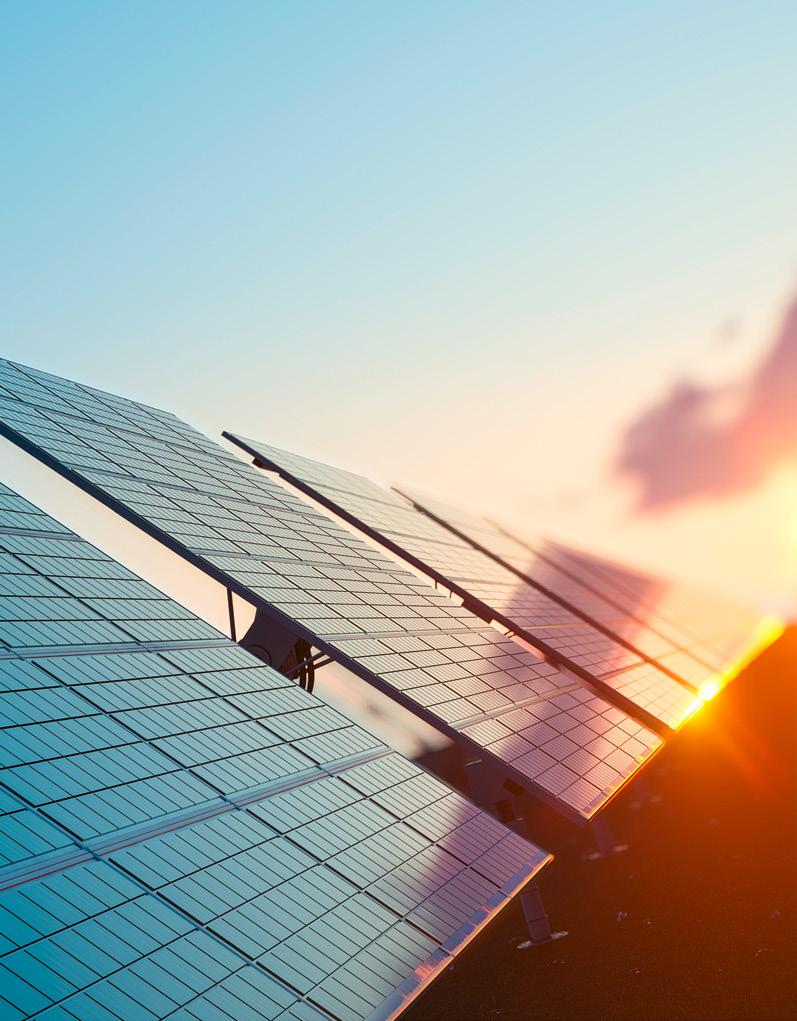
Among the various types of renewable energy being explored to reduce carbon emissions and improve overall sustainability, solar energy is perhaps the most well-developed, affordable, and prevalent. Within the solar energy domain, photovoltaic (PV) panels are the cheapest and most established technology. However, PV panels face many operational challenges, the most significant of which is the accumulation of dust on their surfaces.
PV panels are made up of layers of selected materials that enable sunlight to pass through and interact with photoelectric effect materials, like silicon, which absorb photons from sunlight and release electrons. These electrons are then captured to produce an electrical current that can serve as an energy supply.
When a PV panel is covered in dust, two problems occur: the dust blocks some of the sunlight from being captured, and it can also cause heat to accumulate. Both of these issues result in reduced operational efficiency for the PV panel. It has been estimated that in desert climates like that of the UAE, dust accumulation reduces the overall power output of PVs by nearly 40% over a year.
To mitigate the dust accumulation problem, PV panels must be cleaned regularly. However, the rate at which dust accumulates varies greatly depending on the temperature, wind speed, humidity, panel tilt angle, and other factors. Additionally, if the panels are cleaned too often, it will be a waste of resources like water and costly cleaning solution, and if they aren’t cleaned often enough, the PV plant will not generate the required energy.
An American University of Sharjah (AUS) research project has proposed an optimal scheduling approach for cleaning PV panels that maximizes profit and reduces downtime. The research team was composed of AUS Associate Professor of Electrical Engineering Dr. Mostafa Shaaban, AUS Professor of Electrical Engineering Dr. Mahmoud H. Ismail, and AUS graduate student Armaghan Cheema. They recently published a paper on their work in the reputed journal Applied Energy.
“Previous research has attempted to model PV performance and dust accumulation using numerous techniques and assumptions. For instance, some have begun with the estimated PV values according to the daily solar irradiance and then estimated dust accumulation based on the difference. Many such models look at dust as a constant factor, but this is incorrect, as dust accumulation varies throughout the day and season. We wanted to build a model that considered all the variables that impact dust accumulation to provide a more accurate prediction of how much dust accumulates on PV panels in a specific setting, and use that accurate prediction to guide cleaning schedules,” explained Dr. Shaaban.
To enable PV plant managers to better understand their panels’ dust accumulation rates and determine the ideal cleaning schedules, the AUS team sought to leverage a Markov chain stochastic model.
“A Markov model is a mathematical tool that assumes a future state depends only on the current state, not on the states that occurred before it. This tool is used
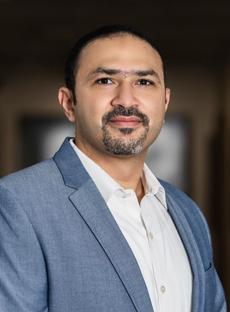
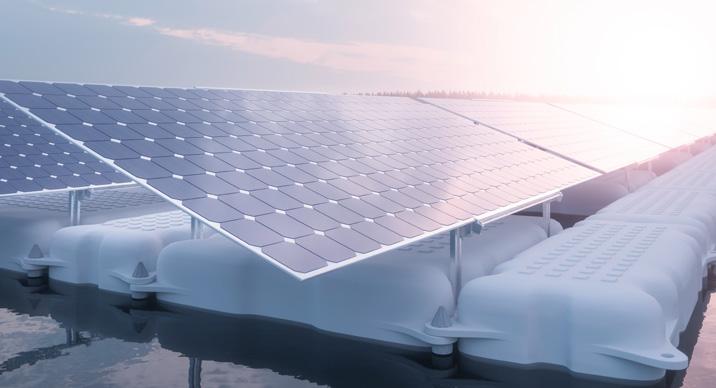
Dr. Mostafa Shaaban
Associate Professor of Electrical Engineering, American University of Sharjah
in many situations across our daily lives, for example, designing queuing systems for banks, call centers, and the like,” Dr. Ismail explained.
The first major step in the project was acquiring and processing the necessary data. The team first gathered PV power measurement and climate data from Arizona City in the United States, as such data from the UAE was not available. The PV measurements were made from a solar panel with a fixed tilt angle of 30 degrees, while the climate data included ambient temperature, solar irradiance, dust accumulation, and rate of dust accumulation, resulting in four data sets.
The data sets were then categorized according to the season, enabling the model to account for seasonal variations in each data set. The seasonal data sets were then further categorized into groups based on the dust level in the first hour of the day, assuming that the dust level would not change for the rest of the day.
The second major step was developing the Markov chain model to model the behavior of numerous weather factors and their impact on dust accumulation to analyze the performance of a PV panel. The third step comprised the generation of a cumulative distribution function, which is a method to describe the distribution of any random variable that enables virtual scenario generation. Finally, the fourth step was to use the model to develop virtual scenarios
showing dust accumulation levels across an entire year.
At the end of this four-stage development process, the model was able to produce virtually generated scenarios that can be used by PV plant developers and operators to decide on the optimal size of their PV system and cleaning frequency for each season.
To ascertain the impact of the model, the research team then used it to develop a case study for a 100MW plant. They simulated five different cleaning frequencies – daily, weekly, biweekly, monthly, and never – and two cleaning methods – automated and manual – to find out which combination of frequency and method resulted in the plant’s greatest operational efficiency and profitability.
For the scenario in which the plant followed a fixed PV cleaning schedule, weekly cleaning was found to be the best, resulting in a 26.2% increase in net profit compared to the no cleaning scenario. For the scenario in which PV cleaning can be varied seasonally, the optimum cleaning frequencies were found to be weekly in spring, daily in the summer and autumn, and weekly in the winter. This schedule would increase the plant’s profit by 27.3% compared to the no cleaning scenario.
The research team also provided estimates based on the specific cost of electricity per megawatt hour (MWh), following a basic pattern of increasing cleaning frequency as cost increased until reaching a daily cleaning schedule at $160 per MWh. The net profit increase varied from 23.90% to 36.2% for $40 to $160 per MWh, respectively. The team also determined that automated cleaning was more economical overall than manual cleaning.
Explaining the potential impact of the team’s dynamic model, Dr. Shaaban said it can help PV farm operators better plan their energy output and select cleaning schedules that optimize profits. Dubai-based solar system design and installation firm Rentech Systems LLC, which supported the project with equipment, will reportedly be the first company to adopt this model and use it to schedule cleaning events.
The next step of the project will be to incorporate more variables into the model, like wind speed and humidity.
“We hope our project will help contribute to the development and incorporation of clean and efficient power systems across the UAE, region, and beyond. We also want to provide data and guidance to enhance power systems and the renewable generation portfolio in the UAE. We want to build tools to help maintain renewable energy sources, save energy, and hence improve sustainability,” Dr. Shaaban added.
The UAE Energy Strategy 2050 has a goal of increasing the contribution of clean energy in the total energy mix from 25% to 50% by 2050 and reducing the carbon footprint of power generation by 70%.
Title of published paper A novel stochastic dynamic modeling for photovoltaic systems considering dust and cleaning
Published in Applied Energy
Journal metrics Impact Factor: 9.746, Q1, H-index: 212, Scientific Journal Ranking (SJR): 3.04
Project funded by American University of Sharjah