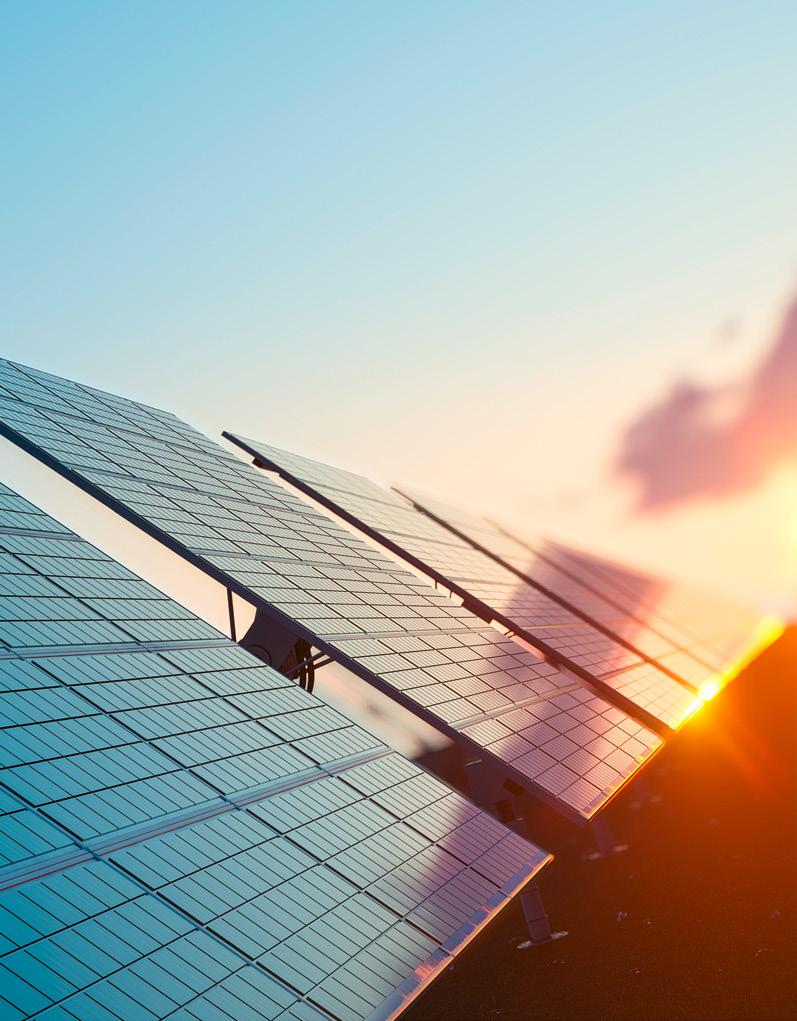
6 minute read
OPTIMIZING PHOTOVOLTAIC PANEL CLEANING FOR MAXIMUM GAINS
Dust accumulation is a significant issue for photovoltaic farms, particularly in the Gulf region. An American University of Sharjah project leverages stochastic models to determine the ideal dust cleaning schedule to ensure it does not interfere with the farms’ operational efficiency.
Among the various types of renewable energy being explored to reduce carbon emissions and improve overall sustainability, solar energy is perhaps the most well-developed, affordable, and prevalent. Within the solar energy domain, photovoltaic (PV) panels are the cheapest and most established technology. However, PV panels face many operational challenges, the most significant of which is the accumulation of dust on their surfaces.
Advertisement
PV panels are made up of layers of selected materials that enable sunlight to pass through and interact with photoelectric effect materials, like silicon, which absorb photons from sunlight and release electrons. These electrons are then captured to produce an electrical current that can serve as an energy supply.
When a PV panel is covered in dust, two problems occur: the dust blocks some of the sunlight from being captured, and it can also cause heat to accumulate. Both of these issues result in reduced operational efficiency for the PV panel. It has been estimated that in desert climates like that of the UAE, dust accumulation reduces the overall power output of PVs by nearly 40% over a year.
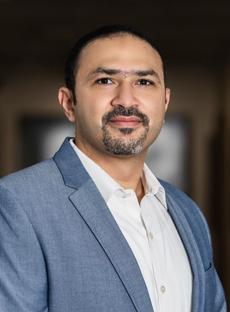
To mitigate the dust accumulation problem, PV panels must be cleaned regularly. However, the rate at which dust accumulates varies greatly depending on the temperature, wind speed, humidity, panel tilt angle, and other factors. Additionally, if the panels are cleaned too often, it will be a waste of resources like water and costly cleaning solution, and if they aren’t cleaned often enough, the PV plant will not generate the required energy.
An American University of Sharjah (AUS) research project has proposed an optimal scheduling approach for cleaning PV panels that maximizes profit and reduces downtime. The research team was composed of AUS Associate Professor of Electrical Engineering Dr. Mostafa Shaaban, AUS Professor of Electrical Engineering Dr. Mahmoud H. Ismail, and AUS graduate student Armaghan Cheema. They recently published a paper on their work in the reputed journal Applied Energy.
“Previous research has attempted to model PV performance and dust accumulation using numerous techniques and assumptions. For instance, some have begun with the estimated PV values according to the daily solar irradiance and then estimated dust accumulation based on the difference. Many such models look at dust as a constant factor, but this is incorrect, as dust accumulation varies throughout the day and season. We wanted to build a model that considered all the variables that impact dust accumulation to provide a more accurate prediction of how much dust accumulates on PV panels in a specific setting, and use that accurate prediction to guide cleaning schedules,” explained Dr. Shaaban.
To enable PV plant managers to better understand their panels’ dust accumulation rates and determine the ideal cleaning schedules, the AUS team sought to leverage a Markov chain stochastic model.
“A Markov model is a mathematical tool that assumes a future state depends only on the current state, not on the states that occurred before it. This tool is used
- Dr. Mostafa Shaaban, Associate Professor of Electrical Engineering, American University of Sharjah
in many situations across our daily lives, for example, designing queuing systems for banks, call centers, and the like,” Dr. Ismail explained.
The first major step in the project was acquiring and processing the necessary data. The team first gathered PV power measurement and climate data from Arizona City in the United States, as such data from the UAE was not available. The PV measurements were made from a solar panel with a fixed tilt angle of 30 degrees, while the climate data included ambient temperature, solar irradiance, dust accumulation, and rate of dust accumulation, resulting in four data sets.
The data sets were then categorized according to the season, enabling the model to account for seasonal variations in each data set. The seasonal data sets were then further categorized into groups based on the dust level in the first hour of the day, assuming that the dust level would not change for the rest of the day.
The second major step was developing the Markov chain model to model the behavior of numerous weather factors and their impact on dust accumulation to analyze the performance of a PV panel. The third step comprised the generation of a cumulative distribution function, which is a method to describe the distribution of any random variable that enables virtual scenario generation. Finally, the fourth step was to use the model to develop virtual scenarios showing dust accumulation levels across an entire year.
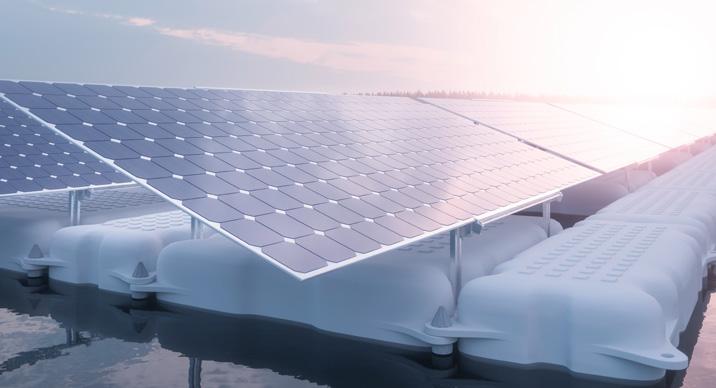
At the end of this four-stage development process, the model was able to produce virtually generated scenarios that can be used by PV plant developers and operators to decide on the optimal size of their PV system and cleaning frequency for each season.
To ascertain the impact of the model, the research team then used it to develop a case study for a 100MW plant. They simulated five different cleaning frequencies – daily, weekly, biweekly, monthly, and never – and two cleaning methods – automated and manual – to find out which combination of frequency and method resulted in the plant’s greatest operational efficiency and profitability.
For the scenario in which the plant followed a fixed PV cleaning schedule, weekly cleaning was found to be the best, resulting in a 26.2% increase in net profit compared to the no cleaning scenario. For the scenario in which PV cleaning can be varied seasonally, the optimum cleaning frequencies were found to be weekly in spring, daily in the summer and autumn, and weekly in the winter. This schedule would increase the plant’s profit by 27.3% compared to the no-cleaning scenario.
The research team also provided estimates based on the specific cost of electricity per megawatt-hour (MWh), following a basic pattern of increasing cleaning frequency as cost increased until reaching a daily cleaning schedule at $160 per MWh. The net profit increase varied from 23.90% to 36.2% for $40 to $160 per MWh, respectively. The team also determined that automated cleaning was more economical overall than manual cleaning.
Explaining the potential impact of the team’s dynamic model, Dr. Shaaban said it can help PV farm operators better plan their energy output and select cleaning schedules that optimize profits. Dubai-based solar system design and installation firm Rentech Systems LLC, which supported the project with equipment, will reportedly be the first company to adopt this model and use it to schedule cleaning events.
The next step of the project will be to incorporate more variables into the model, like wind speed and humidity.
“We hope our project will help contribute to the development and incorporation of clean and efficient power systems across the UAE, region, and beyond. We also want to provide data and guidance to enhance power systems and the renewable generation portfolio in the UAE. We want to build tools to help maintain renewable energy sources, save energy, and hence improve sustainability,” Dr. Shaaban added.
The UAE Energy Strategy 2050 has a goal of increasing the contribution of clean energy in the total energy mix from 25% to 50% by 2050 and reducing the carbon footprint of power generation by 70%.