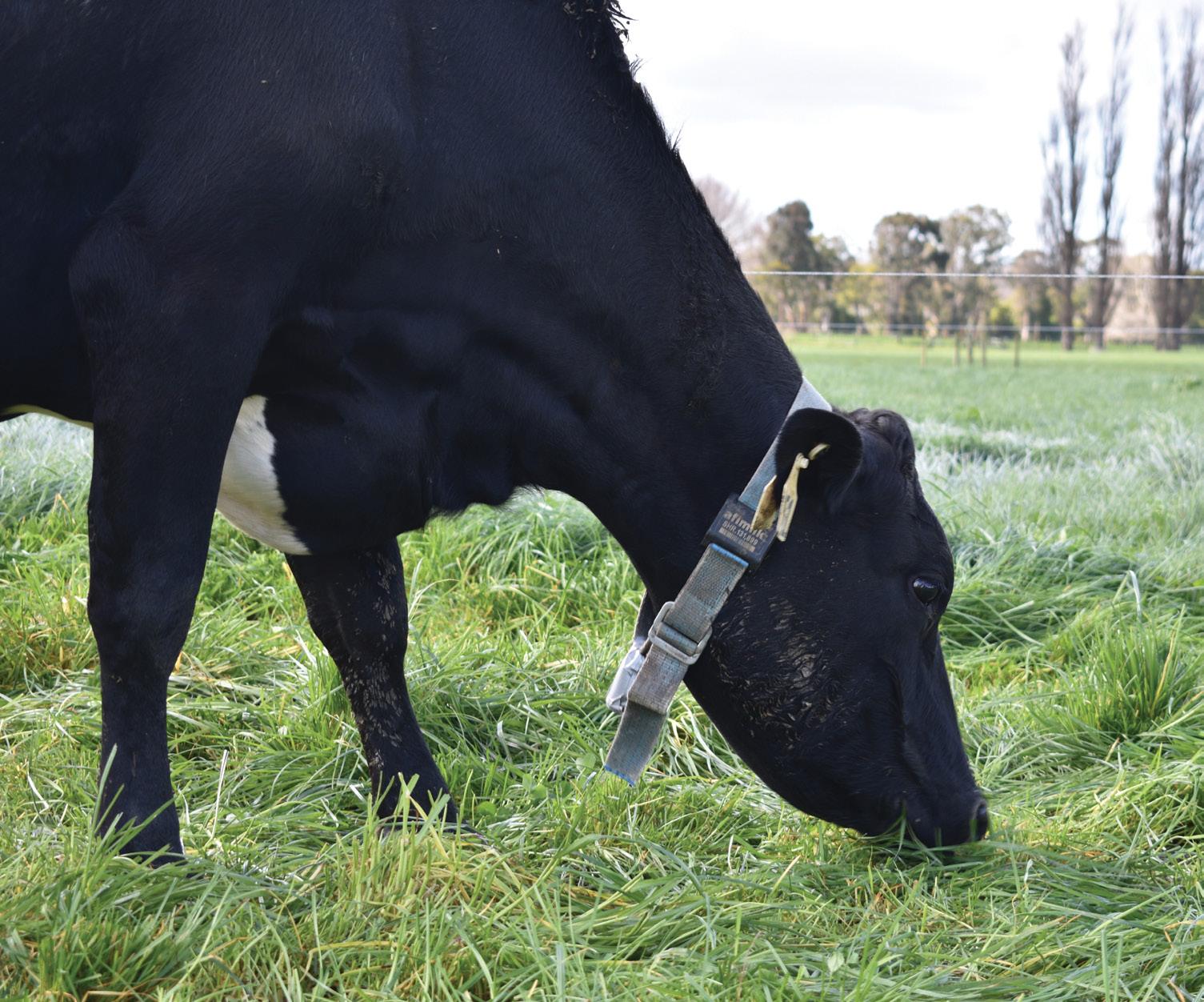
5 minute read
Massey research update
by VetScript
MASSEY UNIVERSITY RESEARCH UPDATE
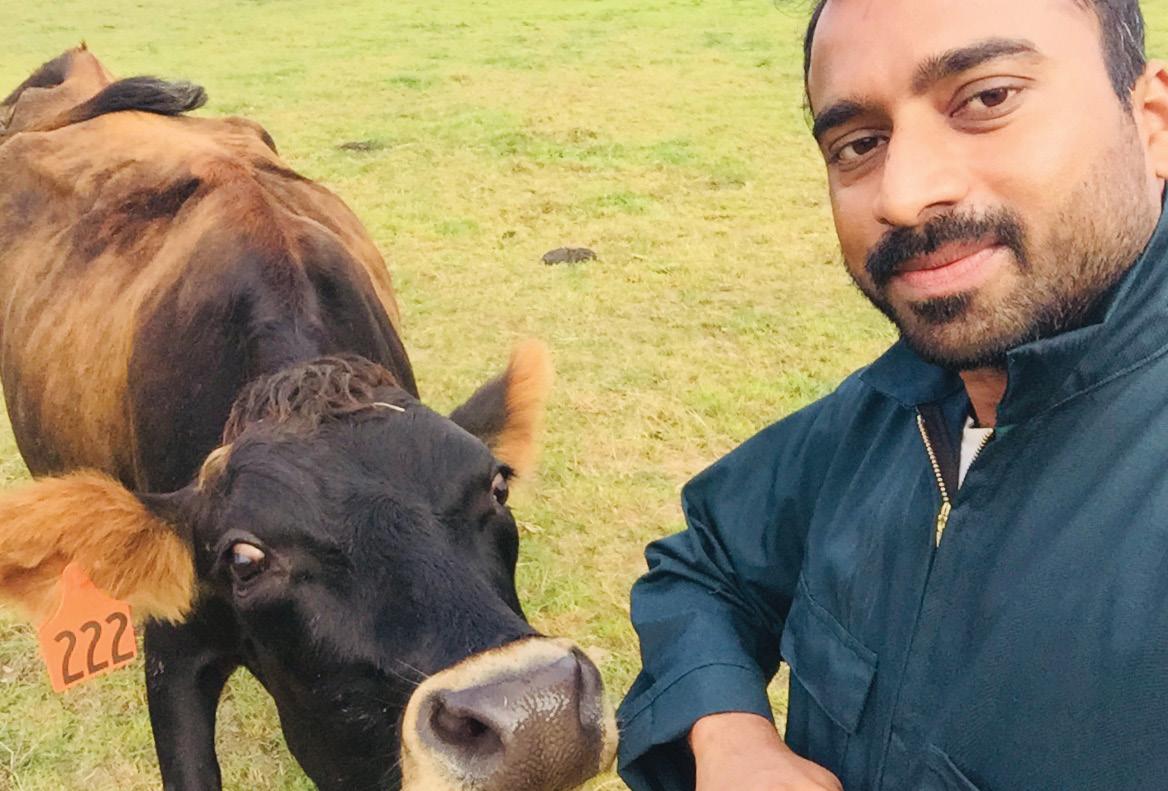
Advertisement
Can cow behaviour
influence performance?
Muhammad Wasim Iqbal, a PhD student at Massey University’s School of Agriculture and Environment, is using leading-edge technology to study the variation between individual dairy cows’ behaviour and their contributions to overall herd productivity.
BACKGROUND
To ensure the competitiveness of the dairy industry in New Zealand, a consistent focus is required on lifting dairy production by considering it more in terms of quality, not quantity. In terms of value, not volume.
Ultimately, an individual animal’s performance (productivity) has a key role in the overall herd’s performance and the profitability of the farm. Having data on animal performance can help farmers to adjust housing conditions and feed so that their animals produce more, or better-quality, products. It can also highlight the best- and worst-performing individuals, and aids in decisions on which cows to keep.
An individual’s performance is influenced by several factors including genetics, feeding style, reproductive status, health and the overall management of the farming system, each of which has been widely explored (Bareille et al., 2003; Walker et al., 2004). Dairy farming in New Zealand is founded on grass-based grazing production, in which animal performance is believed to be influenced by the animals’ behaviour, particularly grazing and rumination.
Grazing behaviour refers to grass intake, and it’s influenced by grass type, climatic conditions and the social status of the animals in the herd (Sowell et al., 1999; Beauchemin, 2018). Rumination behaviour indicates an animal’s digestive efficiency and health status and varies depending on grass quality, type and size. Intake and digestive efficiency (ie, grazing and rumination behaviour) affect the animals’ performance substantially. It’s therefore useful to explore the contribution of animal behaviour to dairy cow performance.
Until now it’s been difficult to measure the effects of individual animals’ behaviour on their performance, owing to a lack of tools to measure their behaviour in a commercial grazing-based system.
PRECISION LIVESTOCK FARMING
Precision livestock farming (PLF), also referred to as the ‘per-animal approach’, uses advanced technologies to optimise the contribution of each animal in a herd’s overall herd performance. It can supplement the eyes and ears of the farmer through real-time monitoring of behaviour, milk production, temperature, and whether the animal is in heat. In some cases it can predict the onset of disease (Rutten et al., 2013).
This information can be used by farmers to improve animal performance, welfare, physical health and reproduction and in the overall management of the farming system (Morota et al., 2018).
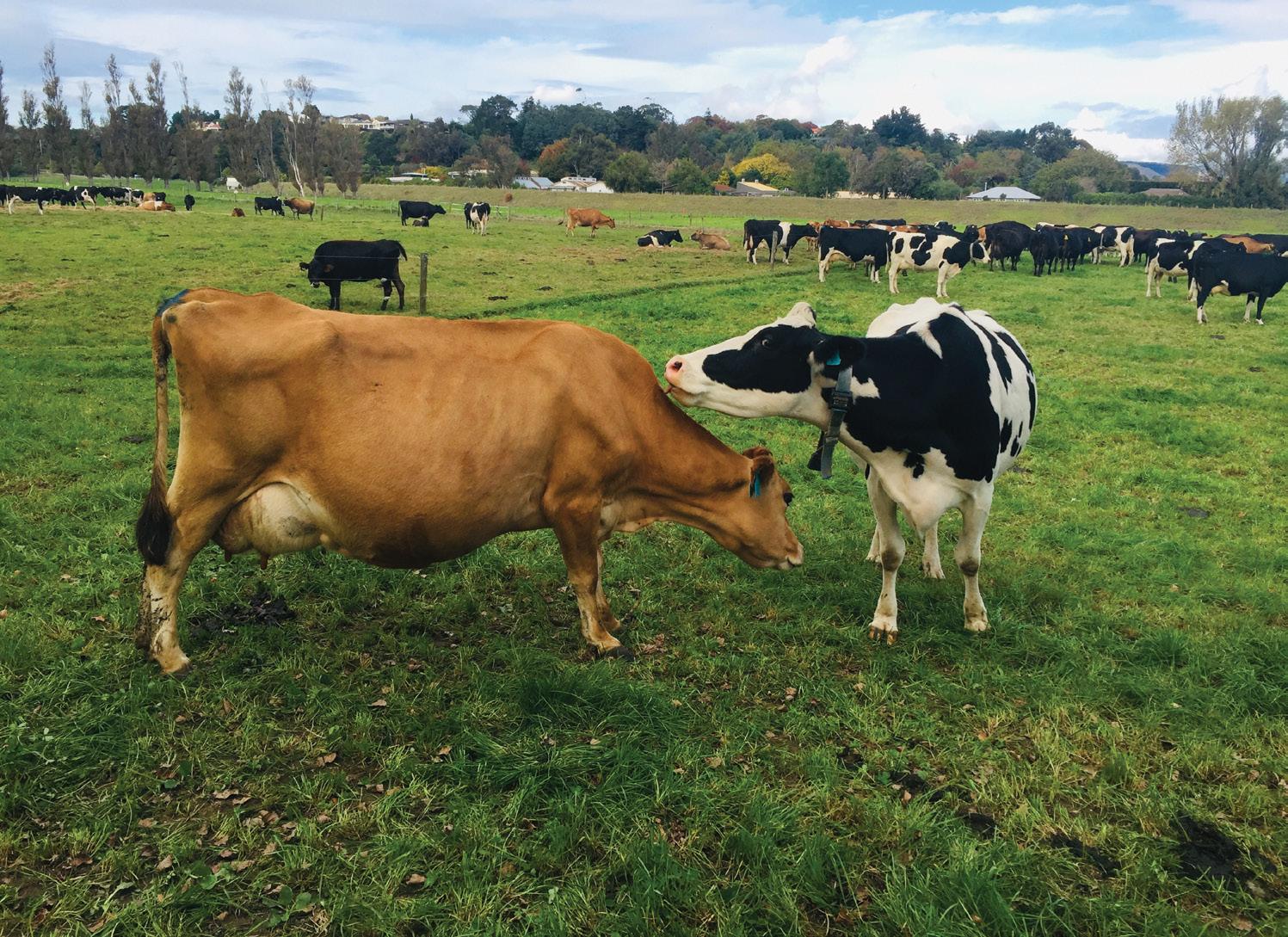
However, while devices that specifically measure behaviour have been tested overseas in indoor, confined dairy systems, there’s little evidence of their accuracy in outdoor grazing systems like those found in New Zealand. This and the system’s cost are barriers to the farming community adopting the technology.
GOALS OF CURRENT STUDY
This study primarily aims to validate a behaviour-monitoring collar for dairy cows in a grazing-based system in New Zealand. It involves examining individual animals’ variations in grazing and rumination behaviour throughout the lactation season and evaluating whether behaviour can improve performance prediction in dairy cows.
THE SENSOR-EQUIPPED TOOL
The study is using a herd of dairy cows from Massey University’s Dairy 1 farm. The herd comprises three breeds (Holstein-Friesian, Jersey and KiwiCross) in different lactations. We are collecting data on individuals’ hourly/daily grazing times, hourly/daily rumination times, daily live weights, monthly body condition scores and monthly milk production and composition for the whole lactation.
Behaviour data is being collected using an automated device called the Afimilk Silent Herdsman collar. The collar monitors and records daily and hourly grazing times and rumination times on a real-time basis. The collar is fitted around an animal’s neck, with the sensor positioned on the right side of the neck. The collar has a triaxial accelerometer sensor capable of identifying specific patterns in different behaviour categories, such as grazing and rumination. Built-in generic algorithms process the collected data and express it as grazing time and rumination time. The collar wirelessly transmits the collected data to a base station when the cow is in the range of ~500 metres, and a wireless internet connection in the farm environment is used to download it.
IMPLICATIONS
Data have already been collected for the 2018–19 and 2019–20 lactation seasons. Data for this season (2020–21) are currently being collected, with completion expected by March 2021. We’ll then undertake statistical modelling to evaluate the variations in grazing and rumination behaviour in different breeds, ages and lactation stages. We’ll also evaluate how variations in pasture quality affect grazing and rumination behaviour.
The study will provide information on the association of grazing and rumination behaviour with animal performance, body weight and body condition score, and the extent to which animal behaviour can predict animal performance. Investigations of intraand inter-animal variations in dairy cow behaviour and performance throughout the lactation season will contribute to further studies aimed at selecting more efficient animals. In turn, the selection of alternative, high-yielding dairy cows with appropriate indices will help to meet the future demands for milk volume and quality.
A preliminary analysis of the data already collected has revealed that breed and lactation number don’t affect grazing and rumination time significantly, but generally Jerseys spent more time grazing and less time ruminating compared to Friesians. Time spent grazing appears to decrease with the number of lactations, and the time of the year seems to have significant effects on grazing and rumination behaviour.
The study’s next steps include collecting and analysing the remaining 2020–21 season data. The outcomes of that analysis will help determine any further investigation.
REFERENCES: Bareille N, Beaudeau F, Billon S, Robert A,
Faverdin P. Effects of health disorders on feed intake and milk production in dairy cows. Livestock Production Science 83(1), 53–62, 2003
Beauchemin KA. Invited review: Current perspectives on eating and rumination activity in dairy cows. Journal of Dairy Science 101(6), 4762–84, 2018
Morota G, Ventura RV, Silva FF, Koyama
M, Fernando SC. Big data analytics and precision animal agriculture symposium: Machine learning and data mining advance predictive big data analysis in precision animal agriculture. Journal of Animal Science 96, 1540–50, 2018
Rutten CJ, Velthuis AGJ, Steeneveld
W, Hogeveen H. Invited review: Sensors to support health management on dairy farms. Journal of Dairy Science 96, 1928–52, 2013
Sowell BF, Mosley JC, Bowman JGP. Social behaviour of grazing beef cattle: Implications for management. Proceedings of the American Society of Animal Science 78, 1–6, 1999
Walker GP, Dunshea FR, Doyle PT. Effects of nutrition and management on the production and composition of milk fat and protein: A review. Australian Journal of Agricultural Research 55(10), 1009–28, 2004
PhD candidate Natalie King compiles research projects underway at Massey University.