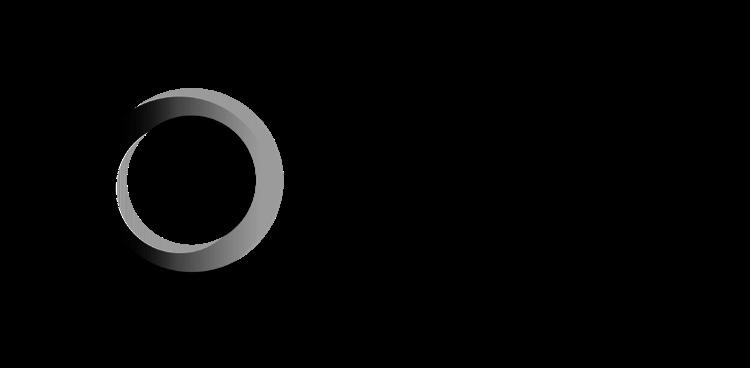
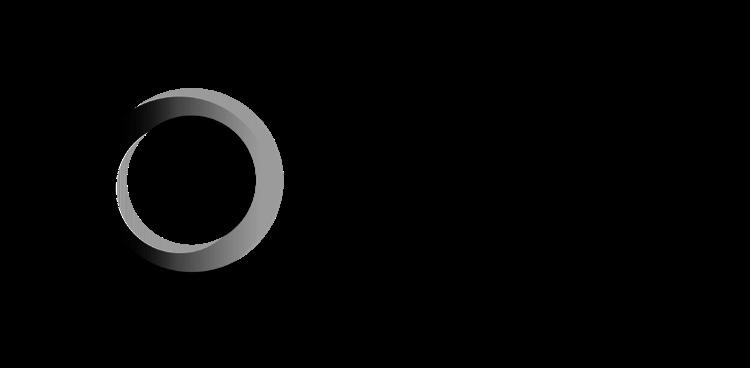
Agence française de développement
Papiers de recherche
Les Papiers de Recherche de l’AFD ont pour but de diffuser rapidement les résultats de travaux en cours. Ils s’adressent principalement aux chercheurs, aux étudiants et au monde académique. Ils couvrent l’ensemble des sujets de travail de l’AFD : analyse économique, théorie économique, analyse des politiques publiques, sciences de l’ingénieur, sociologie, géographie et anthropologie. Une publication dans les Papiers de Recherche de l’AFD n’en exclut aucune autre.
Les opinions exprimées dans ce papier sont celles de son (ses) auteur(s) et ne reflètent pas nécessairement celles de l’AFD. Ce document est publié sous l’entière responsabilité de son (ses) auteur(s) ou des institutions partenaires.
Research Papers
AFD Research Papers are intended to rapidly disseminate findings of ongoing work and mainly target researchers, students and the wider academic community. They cover the full range of AFD work, including economic analysis, economic theory, policy analysis, engineering sciences, sociology, geography and anthropology. AFD Research Papers and other publications are not mutually exclusive.
The opinions expressed in this paper are those of the author(s) and do not necessarily reflect the position of AFD. It is therefore published under the sole responsibility of its author(s) or its partner institutions.
The Proof is in the Pudding
Revealing the SDGs with Artificial Intelligence
Abstract
Seven years after the adoption of the 2030 Agenda, humanity is halfway through the framework of action it has set for itself.
into account by the entire sample, and we note a positive correlation between the size of PDBs’ balance sheet and their consideration for SDGs that are associated with environmental protection.
AUTHORS Jean Baptiste Jacouton Régis Marodon Adeline Laulanié Agence française de développement (AFD)While a growing number of Public Development Banks (PDBs) are integrating the Sustainable Development Goals (SDGs) into their activities, the lack of a common methodology for reporting on their commitments prevents from assessing the progress made in addressing the funding gap of the 2030 Agenda.
This paper explores the possibility of using artificial intelligence (AI) methods to analyze systematically, robustly and in a unified way, PDBs activity and sustainability reports. We introduce the SDG Prospector, which uses a language model that is more robust and more effective than keyword approaches to detect SDGs in a text.
We apply the SDG Prospector to the institutional documentation of 237 Public Development Banks over the 2016 2020 period. The results highlight that PDBs’ strategic and operational narrative is mainly structured around the “productive” Sustainable Development Goals such as SDG 8 “Decent Work and Economic Growth” and SDG 9 “Industry, Innovation and Infrastructure”. SDG 13 “Climate Action” is increasingly taken
Our work is part of the overall reflection on alignment measures, and the application of common methodologies for analyzing the non financial impacts of organizations.
Keywords
Public Development Banks, Sustainable Development Goals (SDGs), Artificial Intelligence
JEL codes
QO1, Q56, H54, G29
Original version
English
Accepted
October 2022
Acknowledgments
This article would not have been possible without the decisive contributions of François BULLIER (360° client) in the construction of the SDG Prospector. The authors warmly thank Bruce OLIVAUX (AFD) and Jean COMBOT (AFD) for their technical support. This article also benefited from the expertise (in alphabetical order) of Eric BEUGNOT (AFD), Lisa CHAUVET (Université Paris 1 Panthéon Sorbonne), Martin KESSLER (Finance for Development Lab ), and Hugo LAPEYRONIE (AFD).
Résumé
Sept ans après, l’adoption de l’Agenda 2030, l’humanité se trouve à mi chemin du cadre d’action qu’elle s’est fixé.
Si un nombre croissant de Banques Publiques de Développement (BPD) intègrent les Objectifs de Développement Durable (ODD) dans leurs activités, l’absence de méthodologie commune pour rendre compte de leurs engagements ne permet pas d’apprécier les progrès accomplis pour combler le déficit de financement de l’Agenda 2030.
Cet article explore la possibilité d’utiliser des méthodes expertes d’intelligence artificielle (IA) pour analyser de façon systématique, robuste et unifiée les rapports d’activités
et de développement durable des BPD. Nous introduisons le Prospecteur ODD qui emploie un modèle de langage, plus robuste et plus performant que les approches par mots clés, pour détecter les ODD dans un texte.
Appliqué à la documentation institutionnelle de 237 banques publiques de développement sur la période 2016 2020, le Prospecteur ODD met en évidence que le narratif stratégique et opérationnel des BPD est principalement structuré autour des Objectifs de Développement Durable
« productifs », tels que l’ODD 8
« Travail décent et croissance économique », et l’ODD 9
« Industrie, Innovation et Infrastructures ». L’ODD 13
« Mesures relatives à la lutte
contre le changement climatique » est pris en compte de façon croissante par l’ensemble de l’échantillon, et nous notons une corrélation positive entre la taille de bilan des BPD et la prise en compte des ODD associés à la protection de l’environnement.
Notre travail s’inscrit dans la réflexion globale sur les mesures de l’alignement, et l’application de méthodologies communes d’analyse des impacts extra financiers des organisations.
Mots-clés
Banques Publiques de Développement Objectifs de Développement Durable (ODD), Intelligence Artificielle
Introduction
The financial system arouses many expectations about its role in supporting the green and just transition. Adopted in 2015 by the United Nations, the 2030 Agenda for Sustainable Development materializes the priority objectives to reconcile human, economic and social development and the protection of the planet. The achievement of the 17 Sustainable Development Goals (SDGs) requires investments with important social and environmental components, whose risk characteristics, profitability and temporality may differ from the short term objectives of private investors.
Because they serve public policies, and because their main objective is not the pursuit of profit, Public Development Banks (PDBs) are a financial tool in the hand of States to promote the ecological transition, and contribute to social justice. Responsible for about 10% of annual global investments (Xu et al., 2021), they can act to offset certain market imperfections (Griffith Jones and Ocampo, 2018; Ocampo and Ortega, 2020), de risk investments targeted towards common goods (WWF, 2021), and could catalyze public and private funding. Their counter cyclical role in responding to the Covid 19 crisis is now well documented (Griffith Jones et al., upcoming; Gutierrez and Kliatskova, 2021; McDonald et al., 2020).
While climate disruption and the loss of biodiversity are the crises of the 21st century, the path of sustainable finance,
1
The Directive 2014/95/EU of the European Union notably asks large companies to publish their contributions on sustainable development.
which organizes the compatibility of environmental, social and economic issues, is to be invented. The integration of the SDG rainbow and its interactions into PDBs’ strategies and operations is a challenge for development finance. Several recent works (Riaño et al., 2021 (a); Riaño et al., 2021 (b); Marodon, 2020) conclude that SDG alignment, understood as a dynamic process, by which public and private actors would adapt their activities to national low carbon strategies, remains a challenge for Public Development Banks. The initiatives carried out by a few pioneering PDBs are meritorious, but still heterogeneous, and too few to allow scaling up (Riaño et al. (2021(a)).
Within the financial sector, there is no harmonized method for reporting on the impact of banks' activities in relation to the 2030 Agenda. While most financial institutions publish an annual activity report, if necessary accompanied by an extra financial report of their impacts 1, the content and structure of these documents vary significantly. Some banks mainly detail their corporate social responsibility (CSR), others break down their commitments according to the 17 SDGs according to their own methods, when others only highlight a few iconic projects they have funded. This lack of a common methodology makes it impossible to compare the quality and depth of PDBs’ commitments to the SDGs, and even less to assess the progress made in addressing the funding gap towards the 2030 Agenda.
Our research questions the possibility of using artificial intelligence (AI) methods to analyze systematically, robustly and in a unified way, PDBs activity and sustainability reports. It is about mapping the nature and intensity of the consideration of the SDGs in their strategic and operational narrative. This article introduces the SDG Prospector, a tool that uses recent Natural Language Processing (NLP) methods to identify texts that address one or more SDGs. The SDG Prospector uses DistilRoBERTa, a language model developed by Facebook (Liu et al., 2019). This allows us to infer the meaning of words according to the context of the sentence in which they are identified, and ensures a more accurate reading than a keyword detection approach (Pincet et al., 2019; LaFleur, 2019).
We apply the SDG Prospector to the institutional documentation of 237 Public Development Banks over the 2016 2020 period. The results indicate that SDG narrative is mainly structured around the “productive” Sustainable Development Goals, such as SDG 8 “Decent Work and Economic Growth” and SDG 9 “Industry, Innovation and Infrastructure”. Narratives related to the protection of biodiversity (SDGs 14 and 15), and those related to the reduction of poverty and inequalities (SDGs 1 and 10) constitute a negligible part of PDBs’ activity reports. SDG 13 “Climate Action” is increasingly taken into account across the sample, and we note a positive correlation between the size of PDBs’ balance sheet and their consideration for environmental SDGs. Finally, we complement our results with a cluster analysis which reveals that PDBs with
similar characteristics tend to share the same SDG narrative.
While existing works on alignment restrict their analyses to a limited number of financial actors, we present a comprehensive mapping of Public Development Banks’ positioning in relation to the SDGs. Indeed, the SDG Prospector’s semantic analysis allows to reveal PDBs strategic alignment preferences. To train our model to recognize the 17 SDGs, we established a specific learning base. Our article is at the crossroads of two contemporary questions: on the one hand, the integration of the 2030 Agenda by Public Development Banks, on the other, the growing literature on the use of artificial intelligence in favor of the SDGs (Chiky and Guisiano, 2021; Pincet et al., 2019; LaFleur, 2019). Our conclusions open the way to the application of common methodologies for the analysis of organizations’ extra financial impacts.
The article is constructed as follows: Section 1 contextualizes our research topic, and offers a cross literature review on Public Development Banks’ SDG alignment, and the use of artificial intelligence in support of the 2030 Agenda. Section 2 details our methodo logy, and section 3 presents the results. Finally, Section 4 discusses the limitations of our approach and the paper opens up on possible areas of application.
1. Literature review
Our work crosses two streams within the literature. The first is related to the enabling role of the public financial sector as a catalyst for achieving the SDGs, including Public Development Banks. The second relates to the emergence of artificial intelligence methods in social sciences, and in particular their ability to generate quantitative data from texts. First, our literature review questions the role of PDBs in the search for a financial model compatible with sustainability. Second, we review the current uses of AI methods for SDG mapping, to highlight the strengths and limitations of existing approaches
1.1 The mobilization of Public Development Banks
1.1.1. Public Development Banks’ renaissance
Spread over all continents, and present in almost every country, there are more than 500 Public Development Banks around the world (Xu et al., 2021) 2. With a variety of public mandates, such as financing infrastructure, rural development, or social housing, PDBs account for about 10% of global annual investments 3. Yet, these institutions, whose role is to decline through their funding, public policies decided by governments, have long been neglected under the influence of the Washington consensus and austerity policies (Ocampo and Ortega, 2021).
At the Monterrey Conference and in Addis Ababa (United Nations, 2002; UN 2015), the United Nations recognized the role of national and regional PDBs in directing funding to sectors in need of liquidity, in compliance with environmental and social standards. PDBs’ ability to address market failures (Griffith Jones and Ocampo, 2018) has taken on new meaning during the 2008 global financial crisis and in the aftermath of the global Covid 19 pandemic. By providing emergency funding and technical assistance (McDonald et al., 2020), PDBs have been a key instrument for many governments to support their economies and contribute to the recovery (Griffith Jones et al., upcoming). A theory of change has developed over the years to give these institutions a catalytic role in green and pro social transitions (Gutierrez and Kliatskova, 2021).
In particular, the last decade was marked by numerous creations and by a better structuring of PDBs to strengthen their actions in favor of sustainable development. Thus, the “Finance in Common” (FIC) coalition, created in 2020, aims to federate all PDBs and strengthen their capacity to catalyze public and private funding towards the Sustainable Development Goals in compliance with the Paris Agreement. Supported by regional development bank associations or groups such as the International Development Finance
2
The last update of the database (July 2022) displays 522 institutions in 154 economies.
3
Estimate based on the average turnover of public development banks' balance sheet (assumption: 6 years).
Club (IDFC) 4, the FIC coalition promotes the sharing of experience between institutions, and strengthens the recognition of Public Development Banks in the global financial architecture 5
1.1.2. Role in the 2030 Agenda
Beyond their traditional mandate to finance SMEs and infrastructure (Ocampo and Ortega, 2021), a growing number of Public Development Banks are integrating SDG financing into their activities (Riaño et al., 2021 (a); Riaño et al., 2021 (b)).
Structurally, PDBs’ ability to provide long term capital enables them to play a leading role in financing the water sector (Smits and Rodríguez, 2022), and the production of renewable energy (Munoz Cabré et al., 2020; Attridge et al., 2020). Several works also highlight the increasing action of PDBs in favor of cross cutting objectives, such as gender equality (AFD and UN Women, 2021), the fight against climate change (Fuchs et al., 2021) and the protection of biodiversity, both terrestrial and marine. Although most multilateral development banks and IDFC members have integrated climate issues into their activities, the implemented approaches lack coherence and coordination (Himberg et al., 2020). In general, the fight against climate change is more important on PDBs’ agenda than biodiversity protection (WWF, 2021). Thus, the integration of biodiversity issues seems to vary with the size of institutions: the larger the PDB, the more it would integrate biodiversity into its activities. Only 17% of national BPD have commitments targeted towards biodiversity (WWF, 2021), mostly through risk based approaches 6, rather than through positive contributions to nature.
The notion of SDG alignment depends on the socio economic and political conditions of the countries in which PDBs intervene (Morris, 2018). As a result, these institutions report very differently on their activities under the 2030 Agenda. Höfling et al (2019) provide a grid of the degree of SDG reporting. In most cases, banks highlight some of their flagship projects qualitatively, without specifying the amount of their commitments to the SDGs (SDG labelling). Others break down their funding by individual SDGs (Riaño et al., 2021 (a)). For example, for a single €10 million project that contributes to gender and climate simultaneously, some PDBs count twice the amount, and increase their contribution to SDG 5 “Gender Equality” and SDG 13 “Climate Action” by €10 million (SDG Flagging). Other institutions use complex methodologies to allocate their funding volumes across their entire portfolio (SDG mapping). Therefore, several studies call for a harmonization of SDG reporting standards (Riaño et al., 2021 (a); Morris, 2018; Riaño et al., 2021 (b)).
Given the above, it is difficult to have a comprehensive vision, based on a unified method, of PDBs’ positioning regarding the 2030 Agenda (Marodon, 2020). Existing studies focus, by necessity, on a limited number of institutions (Morris, 2018). The emergence of machine learning solutions offers the opportunity to exceed this limit.
4 Created in 2011, the International Development Finance Club (IDFC) is an association of 27 national and regional public development banks. Totalizing an average of USD 150 billion in climate finance each year, IDFC positions itself as a group of driving institutions to achieve the 2030 Agenda and the Paris Agreement on climate
5 This was materialized in 2021 by the official recognition of the FIC coalition by the G20 Finance Ministers.
6 “Do no harm”.
Artificial intelligence serving the 2030 Agenda
The adoption of the SDGs coincides with the rise of new methods of natural language processing (NLP). The latter is defined as a set of techniques that allow a computer to process and analyze human language for a specific purpose, such as lexical analysis or automatic translation. Applied to PDBs’ annual activity reports a wealth of information today under exploited these methods would make it possible to considerably broaden the analysis of extra financial commitments (Marodon, 2020). Several recent studies detail the application of NLP methods to analyze SDG related texts.
Box 1. Artificial intelligence, Natural Language Processing, …what do we talk about?
Appearing more than 60 years ago in the United States (McCarthy et al., 1955) the term artificial intelligence refers to a set of different technologies and algorithms, which aim at allowing machines to perceive, understand, act and learn at levels that enable them to support human beings, especially in the processing of large amounts of data.
Among the many applications of artificial intelligence, text analysis is a matter of natural language processing (NLP). The aim is to model how humans understand and use language as a means of communication, so that the computer can perform tasks such as automatic translation, or the identification of specific themes addressed in a text.
The first examples of application have emerged in the United Nations ecosystem, to facilitate the classification of documents, with regard to the 17 Sustainable Development Goals (LaFleur, 2019; Joshi et al., 2020; Pukelis et al., 2020). These approaches are mainly based on counting the frequency of key words related to each SDG. Relatively easy to implement in different languages, this method has two main limitations. First, it is likely to generate biases because the same word does not have the same meaning in the context of the sentence in which it is used. Thus, “business climate” is very different from “climate protection” despite the use of the word “climate”. Key words approaches do not allow interpreting words according to their context. Second, keyword approaches increase the risk of capturing positive communication in the reports that are analyzed. It would be sufficient for a key word to be repeated many times in a document to increase the relative importance of the theme it refers to. Led by the United Nations Environment Programme, Guisiano and Chiky (2020) propose a more robust classification method using BERT, a neural network specialized in language processing, developed by Google (Devlin et al., 2018). This language model can go beyond key words approaches, because it allows the computer to understand the meaning of the sentences it analyzes 7. However, the tool implemented by the authors does not allow analyzing texts greater than 512 words, which makes it unsuitable for reading annual reports of several tens of pages.
To our knowledge, Pincet et al. (2019) are the first to apply NLP methods, not to classify documents according to the SDGs, but to associate automatically official development assistance projects with the SDGs to which they contribute. To do this, the authors apply a TF IDF 8, trained on the statements made by international donors themselves to the Development Assistance Committee (DAC. Although the method uses bi grams 9, to capture partially the context of sentences, it remains dependent on key words counting. Interestingly, the authors highlight that multilateral development banks are primarily engaged in infrastructure financing.
The first applications of NLP methods to SDG identification, highlight three methodological difficulties that we seek to overcome in order to be able to analyze PDBs’ annual reports in a robust way: i) keyword approaches are likely to introduce significant biases, ii) the quality of the learning base is paramount to ensure the quality of the models, iii) in their primary version, language models do not allow the analysis of multiple page documents without any word limit.
2. Methodology
This section details the methodological choices underlying the construction of the SDG Prospector, and the way it works in practice. Finally, we describe the nature of the documents we analyze and the composition of our sample.
2.1. Beyond keyword approaches
Introduced in 2018, language models are among the latest developments in Natural Language Processing (Bender et al., 2021). In contrast to “naive” approaches using keyword counting, language models allow to interpret words depending on the context from which they originate, and thus allow to better understanding the complexity of a text.
Language models are a new generation of artificial intelligence models using deep neural networks. They are pre trained on very large volumes of texts coming mainly from the web. For example, BERT (Bidirectional Encoder Representations from Transformers), is trained on BookCorpus and all Wikipedia articles in English (Devlin et al., 2018). Thus, it relies on many examples to learn the grammar of English sentences and the meaning of words. These models make it possible to obtain a vector representation of each word. Synonymous words will have digital vector representations that are close. However, the representation of a word is not unique, and depends on the meaning of the word in the sentence. The models also provide a vector representation of each sentence.
8 Term Frequency Inverse Document Frequency
9 Two word associations, which put one next to the other, have a specific meaning. For example, “climate disruption” is a bi gram. Taken alone, the words “disruption” and “climate” have their own meaning. Put together, they refer to temperature variations and weather conditions over a long period.
The SDG Prospector is based on DistilRoBERTa, a light version of the RoBERTa model, which is itself a derivative of BERT, developed by Facebook (Liu et al., 2019). DistilRoBERTa shares the same training base as BERT, expanded to 63 million press articles, as well as two other textual databases. The significant increase in the training base improves the performance of the model. Less bulky, faster to train, this model achieves in most situations equivalent performances to those of BERT. In its initial development, DistilRoBERTa is able to process any type of document written in English, but it is not specialized in identifying the SDGs. It is therefore necessary to complete its training base. This is called transfer learning: from its ability to understand English, we train the model to allow it recognizing the SDGs in Public Development Banks’ annual reports.
2.2. The importance of the learning base
The learning base must enable the SDG Prospector to identify correctly the paragraphs that fall under the SDGs in Public Development Banks’ reports. It must also allow distinguishing the SDGs from each other. Thus, the accuracy of the SDG Prospector derives directly from the quality of the learning base. Exclusively built by selecting relevant texts manually, our learning base consists of over 8,500 paragraphs related to the SDGs, as well as an exclusion universe.
2.2.1 The semantic universes
We designate “semantic universes” the 17 groups of paragraphs related to each of the 17 SDGs. Each semantic universe has at least 500 paragraphs, to allow the SDG Prospector understanding each of the SDGs in its complexity. The selected texts come from various sources: United Nations documents, reports from international institutions (e.g. OECD, European Commission), Public Development Banks, government sites, NGOs, corporate websites, research articles, and press articles (Figure 1) 10
SDG Semantic Universe








SDG Prospector Learning Base
Exclusion
There is no textual database specific to each of the SDGs. To build their learning base, most existing studies (see section 1.2) use off the shelf automatic methods, such as paraphrase templates, to build their learning base (Pincet et al., 2019). Indeed, constructing semantic universes manually is often considered time consuming and too costly. However, we prefer to label each of the paragraphs using expert point of view in order to master the homogeneity and quality of the learning base. In practice, all paragraphs in the learning base are identified by an analyst and compiled into a spreadsheet. Using a 0/1 binary system, the analyst manually enters whether each text refers to one or more SDGs. As a given paragraph can be assigned to multiple SDGs, we enhance the quality of our learning base.
The 2030 Agenda is a complex system, and the understanding of each of the Sustainable Development Goals can vary from one analyst to the other (Pincet et al., 2019) 11. Manual labeling therefore carries a risk of bias, since it is based on interpretation. To minimize this bias, we systematically apply the same method to construct each of the 17 semantic universes: each universe contains the description of the objective and the description of the associated targets. Then, each selected paragraph must refer to one of the SDG targets. The


example, Le Blanc (2015) retains the definition and structure of the SDGs, as set out by the United Nations (2015), but discards all “implementation” targets.


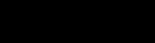
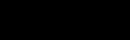








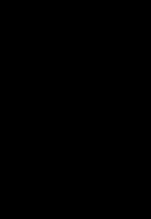
website of the United Nations Department of Economic and Social Affairs (UNDESA) offers open access to a corpus of reports on each SDG. These documents are the primary source for collecting useful paragraphs for the learning base. The semantic universes are then supplemented with texts from other international institutions, such as the World Bank, the OECD, specialized agencies (e.g. International Energy Agency), as well as specialized reports available on government sites. Finally, the semantic universes are enriched with SDG related statements from NGOs, press articles, and private companies.
Beyond their relevance regarding the 2030 Agenda, the paragraphs included in the learning base must respect certain technical characteristics. First, each text must be in English. Paragraphs must contain a minimum of numbers, dates and proper names. Before we include a text in the learning base, and as long as the meaning of the sentences is not altered, we remove the dates, and we replace the names of the continent or countries with words like “the continent”, “the region”, “the country”. Finally, the computational power of the algorithm does not allow it to integrate long texts; the semantic universes are composed of paragraphs that do not exceed 300 words each.
Box 2. Model performance
To assess the value of using a language model compared to a word frequency approach (TF IDF), we use a performance indicator. The MCC coefficient (Matthews Correlation Coefficient) is used to evaluate the model’s ability to correctly identify texts that deal with a given SDG, and vice versa, its ability not to wrongly associate a text with an SDG. The MCC lies between 1 and 1: the closer the score is to 1, the more effective the model. Table 1 presents the results obtained depending on the type of model. In both cases, we use the same learning base in order to capture only the performance variation induced by using a different model. Similarly, the results are obtained from the same test set
Table 1. Differentiated performance between TF-IDF and DistilRoBERTa
SDG TF IDF DistilRoBERTa Variation
0,71 0,80 0,09
0,82 0,82 0,00
0,71 0,87 0,16
0,83 0,89 0,06
0,92 0,95 0,03
0,87 0,89 0,02
0,88 0,91 0,03
0,67 0,66 0,01
0,58 0,80 0,22
0,78 0,75 0,03
0,76 0,87 0,11
0,68 0,80 0,12
0,75 0,83 0,08
0,90 0,98 0,08
0,86 0,94 0,08
0,76 0,89 0,13
0,55 0,68 0,13
Box 2. Model performance (contd.)
On average, using DistilRoBERTa will earn 0.08 MCC points. There are significant gains for some SDGs, such as SDG 9 (+0.22), SDG 3 (+0.16) and SDGs 16 and 17 (+0.13). The use of DistilRoBERTa is therefore more effective than a keyword approach. Through the language model, the average coefficient of most SDGs exceeds the 0.8 threshold and reflects the strong accuracy of the model (mean = 0.84).
SDG 8 “Growth and Decent Jobs” and 17 “Partnerships” have an MCC slightly below 0.7. This can be explained in particular by the cross cutting nature of these two SDGs. Therefore, the obtained results are accurate and do not jeopardize the quality of our approach.
2.2.2 The exclusion universe
contrast to the 17 semantic universes that allow the SDG Prospector to identify and differentiate the Sustainable Development Goals, the exclusion universe aims to point out
to the algorithm that certain sentences contained in PDBs’ annual reports, do not refer to the 2030 Agenda. Composed of more than a thousand paragraphs, only coming from Public Development Banks’ annual reports and labelled manually, the exclusion universe allows the SDG Prospector to avoid two errors.
First, the exclusion universe prevents the algorithm from systematically associating each sentence of the reports with one or more SDGs. If this were the case, the results would not be reliable because structurally, the documentation of Public Development Banks does not deal only with the SDGs. This is especially the case when a bank includes its financial results within its activity reports. It would be incorrect to associate the bank’s accounting elements with the 2030 Agenda. Similarly, when the annual activity report sets out the bank’s internal human resources policy. Thus, we distinguish between PDBs’ SDG narrative, which primarily refers to the impacts of the bank in its current activities, and ESG criteria, which meet the internal policies of the organizations in the conduct of their operations (e.g. parity of staff, inclusion of employees with disabilities, anti corruption measures, etc.).
Second, the exclusion universe reduces the risk of second tier error, that is, cases where the SDG Prospector would wrongly recognize an SDG. This can be the case when a sentence uses a vocabulary that is close to that of the SDGs, without making explicit reference to them. SDG 14 “Aquatic Life” particularly illustrates this point, as many import export banks employ a vocabulary related to marine navigation: “ship”, “shipping”, “marine”, “overseas”. Yet no SDG 14 target explicitly refers to maritime trade. Unlike the paragraphs that constitute the semantic universes, those integrated in the exclusion universe receive no form of reprocessing: names, and dates are kept as they appear in banks’ documentation.
2.3. Automating the reading of annual activity reports
In order to meet regulatory, accountability or voluntary requirements, Public Development Banks report on their activities using three main types of documents: annual activity reports, sustainable development reports, and financial reports. Being specialized and technical, the latter are not intended to provide qualitative information on the strategic alignment of institutions. For example, we exclude PDBs that only publish a financial report, and we focus our analysis on institutions that have published at least one activity or sustainable development report over the 2016 2020 period.
2.3.1. Analyzing long texts
As activity and sustainable development reports are sometimes several hundred pages long, we use a sliding window to cover the entire text. For this, the algorithm splits the text into paragraphs of maximum a hundred words. The SDG Prospector then determines whether each of these paragraphs refers to one, several or no Sustainable Development Goal.
Prior to annual reports analysis, we apply rules to clean up the text. For example, we remove lists of acronyms, titles, and tables. Applying these rules allows increasing the performance of the algorithm without generating bias in the results.
2.3.2. Sample construction
We apply the SDG Prospector on the institutional documentation of 237 Public Development Banks over the 2016 2020 period. These institutions account for 48% of the total number of PDBs worldwide, and for 95% of their total assets (Appendix). The focus on these banks is conditioned by the availability of their institutional documentation, and by the technical characteristics of the SDG Prospector
The algorithm is only applicable on documents written in English. Some banks from non English speaking countries do not have a translated version of their institutional documentation. This constraint affects the geographic coverage of our sample (Especially for countries in West Africa, Central Africa, and Latin America, which are under represented. See Appendix)
Among the different characteristics identified by Xu et al. (2021) the mandate is a variable likely to be correlated with SDG narrative. Figure 2 shows the distribution of mandates in our final sample. Overall, it reflects mandates’ distribution for all PDBs (Appendix). However, the MSME mandate is under represented by 6 points, while the INTL and EXIM mandates are respectively over represented by 5 points. Indeed, export credit agencies, and PDBs specialized in financing the private sector abroad are most likely to publish their annual reports in English (or bilingual), due to the international nature of their partners. Despite these differences, our sample remains representative of the diversity of public mandates, and our results can be interpreted for all PDBs by limiting the risks of bias.
Figure 2. Mandate distribution in the final sample
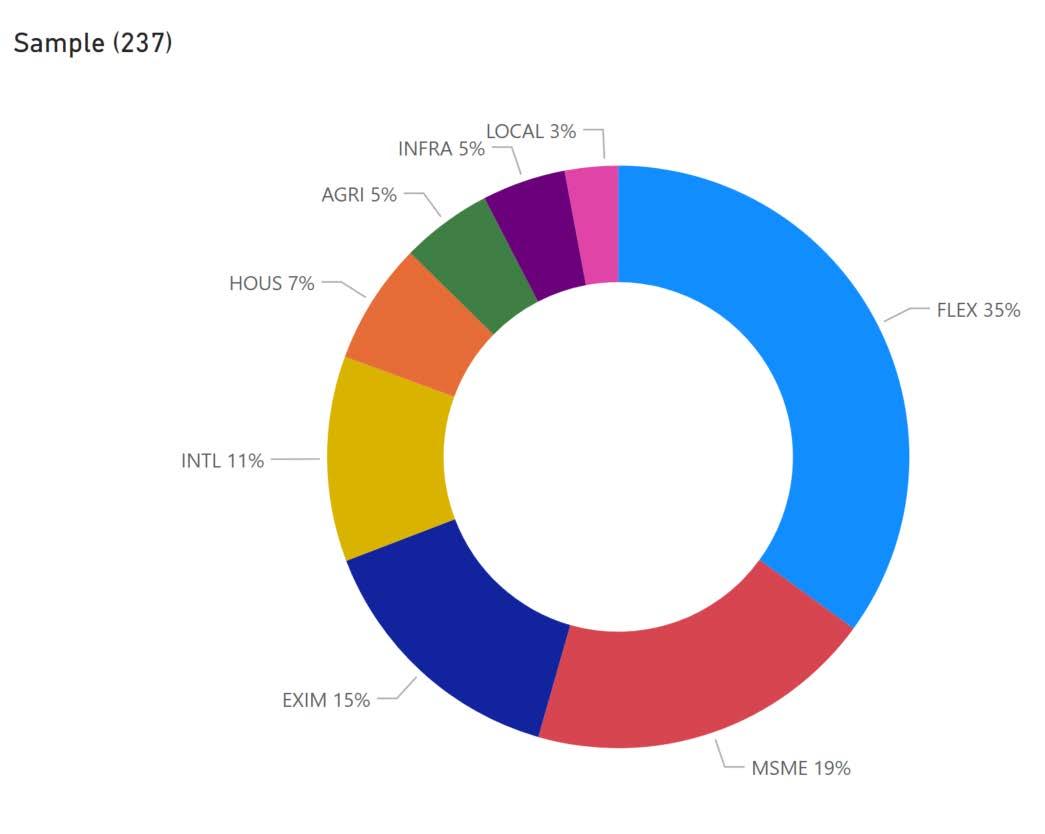
3. Results
The SDG Prospector allows mapping how Public Development Banks report on their activities in relation to the 2030 Agenda. The first results highlight interesting elements regarding the marked orientation of PDBs towards productive SDGs, and a low focus on biodiversity issues. The use of multivariate analysis shows that PDBs that share similar characteristics also display a similar SDG narrative, and highlights the robustness of our results.
3.1. Preliminary remarks
The SDG Prospector computes the number of paragraphs associated with each SDG per document. Because of the variable size of PDBs’ annual reports, absolute values are inconvenient for comparing institutions. In order to avoid interpretation biases related to the size of the reports, we express the Prospector’s results in relative value. Thus, the variable ODDp represents the share of an annual report dedicated to an SDG p ∈ [1;16]. In line with the Le Blanc (2015), we exclude SDG 17 “Partnerships” from our analysis. In the literature, it is identified as a crosscutting objective for achieving SDGs 1 to 16. Thus, we focus our study on the “thematic” SDGs, which better reflect the operational fields of action of Public Development Banks.
For some banks, reports are not available for all the years; this represents 226 documents in total 12. In order to avoid a sampling bias, which would have the effect of giving greater weight to the narrative of banks whose documents are all available, we balance the panel by affecting the average of the observations of each bank to the years for which we have no observations:
the end,
panel
observations. These data do not represent a measure
allow highlighting the SDGs which are the most relevant to the
When aggregated, these results make it
possible to draw an overall mapping of PDBs’ SDG narrative, and to compare the structure of their reports according to their typology.
3.2. Descriptive analysis
3.2.1 A primary focus on productive SDGs
Unequivocally, the SDG Prospector highlights that PDBs’ narrative (Figure 3) is dominated by their historical and productivist mandate, marked by the prevalence of SDG 8 “Decent Work and Economic Growth” and SDG 9 “Industry, Innovation and Infrastructure”. These two SDGs represent 51% of PDBs’ narrative. This may seem counterintuitive from development institutions that often justify their mandate as being “additional” compared to the private banking sector.
This result can be interpreted in two non exclusive ways. An initial interpretation relates to the historical raison d'être of Public Development Banks. As recalled by Ocampo and Ortega (2020), the modern PDB system developed following the Great Depression to support economic activity in the countries affected by the crisis. During the 20th century, numerous countries set up Public Development Banks to finance essential infrastructure to their economic development. For example, the China Development Bank, which is one of the largest PDBs in the world (USD 2.3 trillion of assets), contributes to the growth of the Chinese economy by financing major infrastructure projects such as the new Silk Roads, or the Three Gorges Dam.
Figure 3. Share of each SDG in PDBs’ institutional documentation
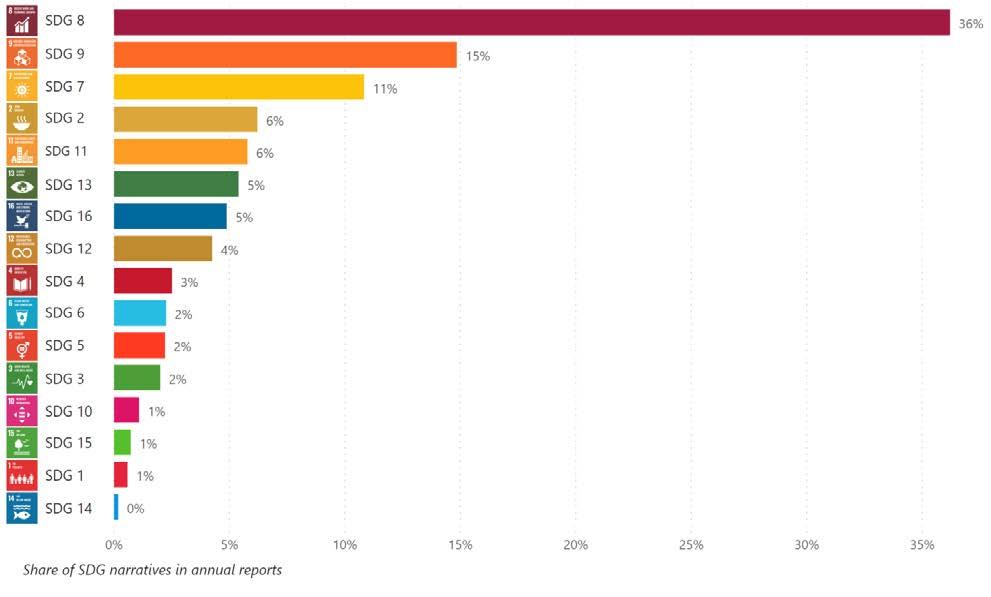
In addition, although PDBs are not intended to maximize their profits, they face financial health requirements. Their economic model is based on the fact that they do not weigh on public finances, at least for most of their activities. They must carry out their mandate in a sustainable manner. This financial constraint requires financing for productive activities, in sectors and with financially sound counterparties capable of servicing the debt resulting from their borrowing.
We also note that SDG 7 “Clean and Affordable Energy” occupies 11% of the narrative and comes in third place. Indirectly, this SDG is one of the most important contributors to the fight against climate change. Indeed, the energy sector is responsible for two thirds of greenhouse gas emissions 13 and the production of renewable energy is one of the levers to achieve carbon neutrality. The prevalence of SDG 7 within PDBs’ institutional documentation, illustrates their involvement in access to energy, and energy transition. For example, 82% of IDFC members' climate finance commitments, or USD 146 billion, were earmarked for financing green energy in 2020 (IDFC, 2021).
While the results point out that PDBs are primarily banks, looking for a solvent clientele, it is expected that they have a strong interest in social and environmental issues.
Regarding social SDGs, SDG 3 “Good Health and Well being” and SDG 4 “Quality Education” capture only 5% of the banks’ narrative and are thus among the least represented. One explanation lies in the fact that investments in health and education are directly part of States’ budget. Therefore, the concerned public funding does not necessarily flow through PDBs. This result is interesting, however, in that it illustrates that the notion of “SDG alignment” should be assessed against a set of public policies, specific to each country.
Finally, our findings question the degree to which natural resources and nature conservation are taken into account in financing operations. While PDBs have a certain level of requirement in project environmental impact assessments, SDG 14 “Aquatic Life” and SDG 15 “Terrestrial Life” are considered very marginally in their narrative. Globally, biodiversity suffers from a significant funding gap. Only USD 78 91 billion are dedicated to biodiversity each year, or just over 10% of the USD 700 billion that would be required for the conservation and sustainable use of ecosystems (IDFC, 2020). While a growing number of Public Development Banks are integrating biodiversity issues into their strategic thinking and risk analysis processes, the topic still seems far from having a significant impact on their portfolio. The most proactive PDBs take into account the increasing risks related to biodiversity loss (Svartzman et al., 2021), have safeguards to limit the negative impacts of their investments on biodiversity, but these mechanisms remain “defensive”. The latter are mainly based on avoidance strategies (e.g., use of exclusion lists) 14 rather than on the search for positive impacts (WWF, 2020). This result is consistent with the conclusions of the WWF,
The emergence of the Task Force for Nature related Financial Disclosures (TNFD) represents a significant step forward in encouraging PDBs to analyze and report more on their investments related to nature.
which points out that biodiversity is still very little integrated in the strategies of the largest PDBs and even less so in the smaller banks.
3.2.2 Significant differences according to bank characteristics
The way in which Public Development Banks integrate the SDGs, varies significantly according to two main characteristics: the size of their balance sheet and their ownership structure.
By breaking down the results by balance sheet size, as proposed by Xu et al. (2021) 15, there appears to be a positive correlation between the size of banks and the relative importance they attach to SDGs with a strong environmental and/or social dimension. This result corroborates the idea that smaller banks would be encouraged to finance a type of clientele that are most likely to maintain their financial balances, such as SMEs which operate on private markets. The share of the narrative given to the SDGs associated with environmental protection 16 is positively correlated (+0.23 17) to the balance sheet size of Public Development Banks (Figure 4). These results suggest that the larger the size of a bank’s balance sheet, the more it is asked to diversify its financing outside the productive sector alone. This is the case for Fannie Mae whose main mandate, as a mortgage bank, is to facilitate access to housing. Nevertheless, the size of its assets is systemic (USD 4000 billion in 2020), and the bank issued its first green bonds in 2012. Today, its green bonds amount to USD 901 billion. This marked orientation towards climate is reflected in the share of SDG 13 “Climate action” in the institution’s narrative (15%). Other iconic PDBs, such as the World Bank and KfW, allocate a significant share of their annual reports to the SDGs associated with environmental protection (20% and 42% respectively).
Conversely, smaller PDBs would have less opportunity to diversify outside their core mandate. Investing in climate change adaptation requires a long time horizon, with more risks than short term investments in productive sectors. It is therefore likely that the risks for smaller banks’ portfolio justify their higher difficulties in diversifying their activities. However, Figure 4 reveals the existence of small PDBs whose SDG narrative is pronounced towards environmental protection, such as the Namibian Environmental Investment Fund and the Nordic Development Fund (see Appendix).
15 Mega (balance sheet greater than $500 billion), large (balance sheet between $100 billion and $500 billion), medium (balance sheet greater than $20 billion and $100 billion), small (balance sheet greater than $500 million and $20 billion), and micro (balance sheet less than $500 million).
16
These include SDG 12 “Responsible Consumption and Production”, SDG 13 “Climate Action”, SDG 14 “Aquatic Life”, and SDG 15 “Terrestrial Life”.
17 Significant at 1% (t Student = 3.62).
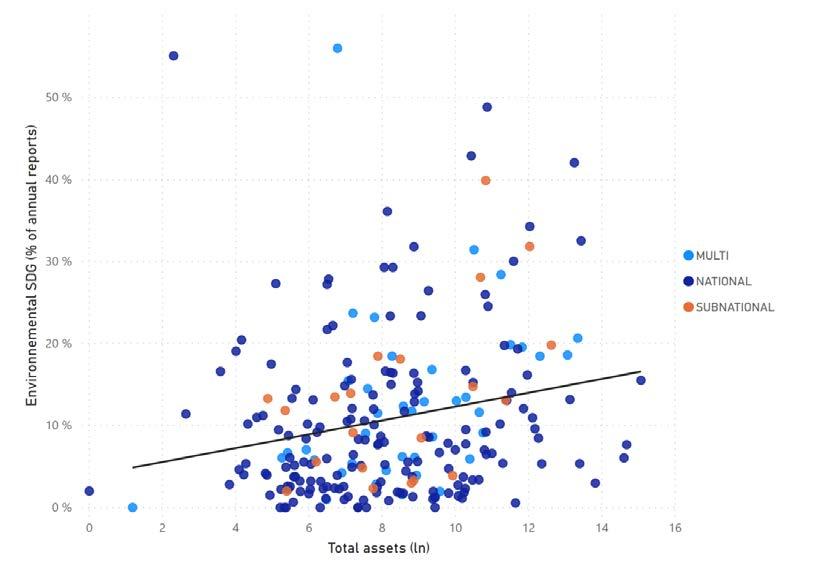
Box 3. Public Development Banks and SDG 13 “Climate action”
The adoption of the Paris Agreement a few months after the Sustainable Development Goals, makes SDG 13 one of the flagship Goals of the 2030 Agenda. Figure 3 shows that over the 2016 2020 period, SDG 13 concentrates 5% of PDBs’ institutional documentation.
Considering the temporal evolution of PDBs’ narrative, the SDG Prospector reveals an increasing attention paid to climate. The contribution of SDG 13 increases from 4.6% in 2016 to 6.6% in 2020. However, there are some disparities depending on the type of PDB considered. Firstly, there is a contrasting trend depending on the size of the banks' balance sheets. Figure 5 shows the evolution over the 2016 2020 period of the “climate” narrative depending on asset size. In 5 years, the share dedicated to SDG 13 in the annual reports of the 9 largest PDBs (balance sheet > USD 500 billion) increases by just over 6 points. This result illustrates that larger banks tend to have a stronger focus on SDGs related to global public goods such as climate, biodiversity, and governance.
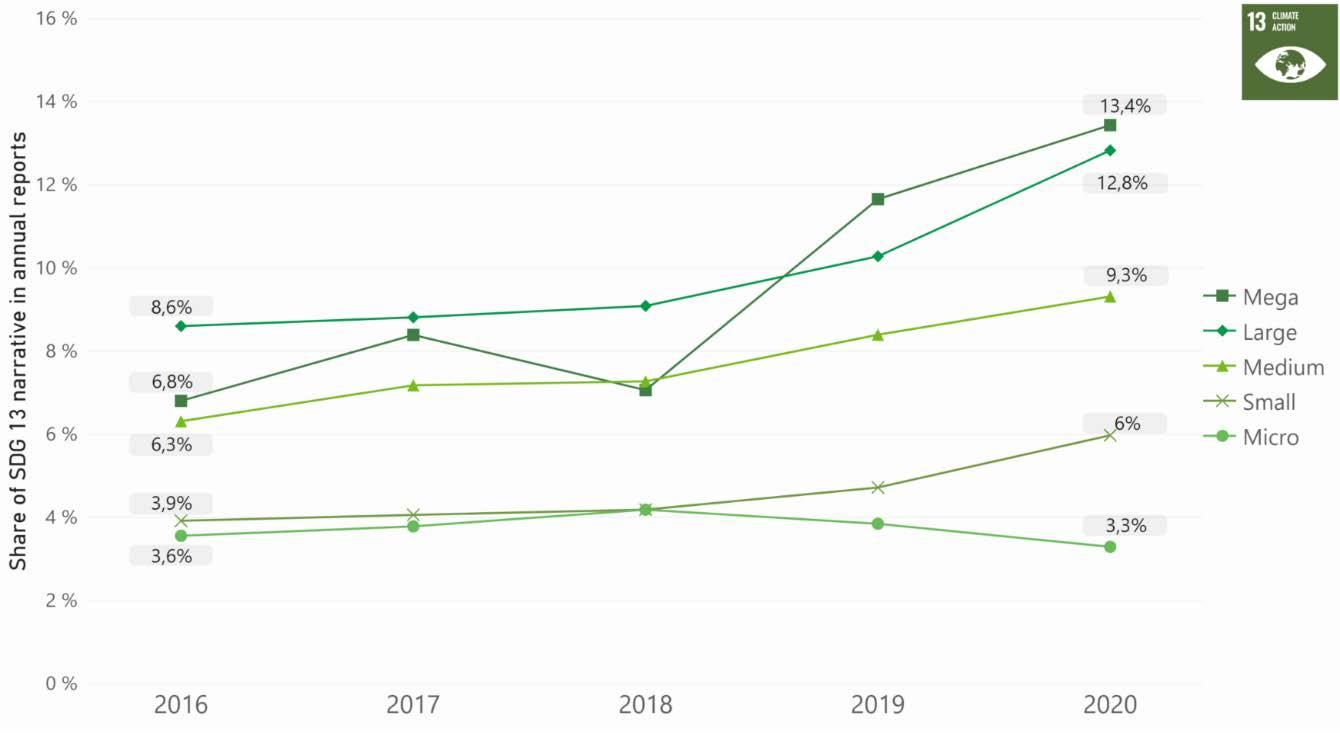
Box 3. Public Development Banks and SDG 13 “Climate action” (contd.)
There are also marked differences depending on banks’ mandate (Figure 6). PDBs specialized in infrastructure (+5.2 points), followed by institutions dedicated to the financing of non sovereign actors in developing countries (+4 points) concentrate the largest increases. The implementation of resilient infrastructure aims in particular to respond to climate change adaptation challenges. On the other hand, banks specialized in financing the agricultural sector do not integrate climate issues into the description of their activities. This result seems counterintuitive due to the heavy dependence of the agricultural sector on climatic hazards.
Figure 7 shows the degree of coherence in PDBs positioning vis à vis the productive SDGs, regardless of their ownership structure, that is, whether several governments (multilateral), a single state (national) or a local government (sub national), hold them. There are, however, notable differences in the narrative of the other SDGs.
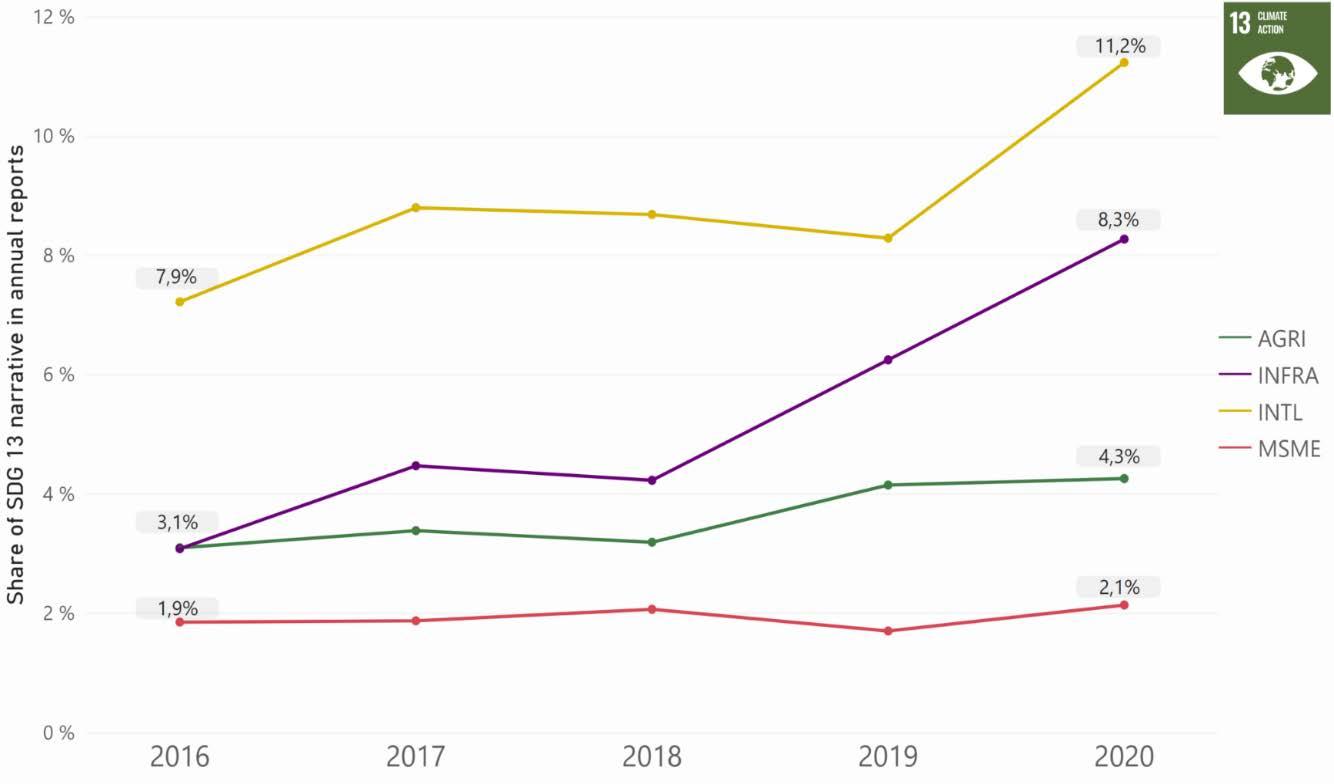
First, the inclination towards the environmental SDGs is twice as important in the narrative of multilateral banks compared to national banks. The rationale could be that multilateral banks are expected to include the issue of global public goods, such as environmental protection, in their mandate. This is also reflected in the higher prevalence of SDG 16 “Peace, Justice and Effective Institutions” in the narrative of multilateral banks. These results call for a better integration of the PDB ecosystem into a financing architecture that would allow national and sub national banks to benefit from the experience and strategic vision of multilateral institutions. It is also noted that while SDG 2 “Zero Hunger” does not occupy the same position in the revealed preferences of multilateral and national PDBs, its share in the narrative is relatively close in both types (6% and 7% respectively).
A focus on the narrative of sub national PDBs highlights two peculiarities. First, they allocate a significant portion of their institutional documentation to SDGs 6 “Clean Water and Sanitation” and 7 “Clean and Affordable Energy” (6% and 13% respectively). These results are partly due to the US Green Banks, which account for a significant share (24%) of the sub national PDBs in our sample, and whose mandate is specifically dedicated to financing renewable energies. The prevalence of SDG 6 is explained by the role of these institutions in funding urban infrastructure. This result is consistent with the share of SDG 11 “Cities and Sustainable Communities” (12%) in the narrative of sub national PDBs. Second, SDG 12 “Sustainable Production and Consumption”, whose targets explicitly refer to waste
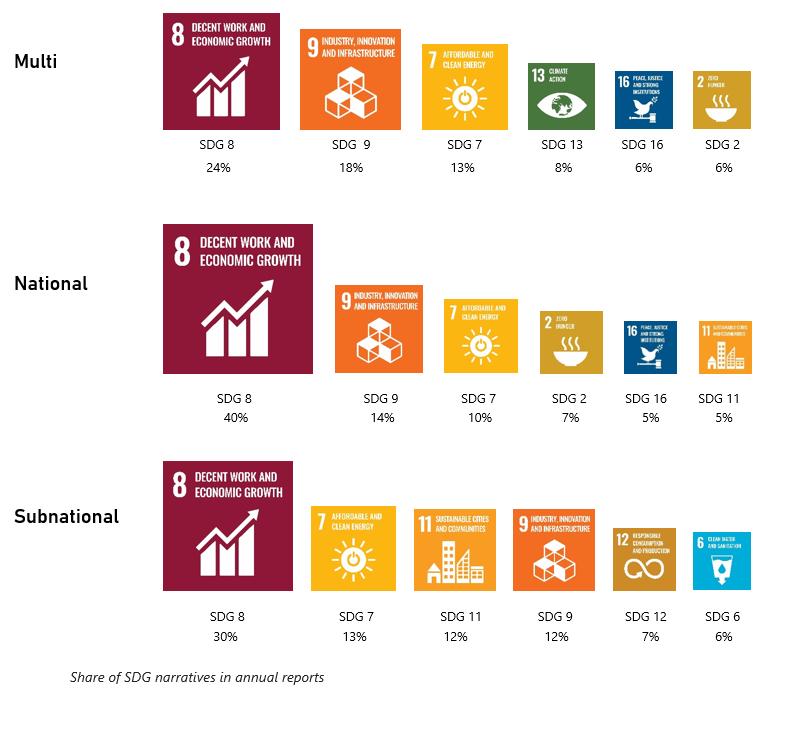
management, and SDG 13 “Climate action” together account for 13% of their institutional narrative. This is consistent with the mandate of sub national banks, which fund waste collection and management policies in many localities, and highlights the role of these PDBs in protecting the environment.
Finally, we analyze our results against other typological criteria: PDBs’ official mandate, their geographical location, and the income level of their country of origin. These criteria do not yield significant differences from one type to another and are consistent with what might be expected. In particular, there is very strong coherence between banks’ mandates and the structure of their SDG narrative (Appendix). Thus, SDG 11 “Sustainable Cities and Communities” occupies 38% of the mortgage banks’ narrative, and 63% of the banks’ reports specialized in infrastructure are granted to SDG 6 “Clean Water and Sanitation”, SDG 7 “Clean and Affordable Energy”, and SDG 9 “Industry, Innovation and Infrastructure”. There is also a negative relationship between the income level of countries and the share of the SDG narrative allocated to SDG 2 “Zero Hunger”. This reflects the relatively high share of the primary sector in the GDP of low income economies (27.6% versus 1.2% in high income countries 18). Finally, there is a positive relationship between the income level of the country in which PDBs operate and the share of their discourse given to SDG 13 “Climate action”.
Box 4. Do IDFC members have significantly different SDG narratives?
The application of the SDG Prospector to IDFC 19 members reveals that their narrative structure is relatively similar to that of other Public Development Banks. Productive SDGs (“Growth and Decent Jobs”; “Industry, Innovation and Infrastructure”) account for more than half of the SDG narrative of these institutions. However, we note that climate issues and sustainable production and consumption are more important on the agenda of IDFC members. Like all PDBs, the biodiversity related SDGs and reducing inequalities represent an almost negligible proportion of IDFC members’ annual reports.
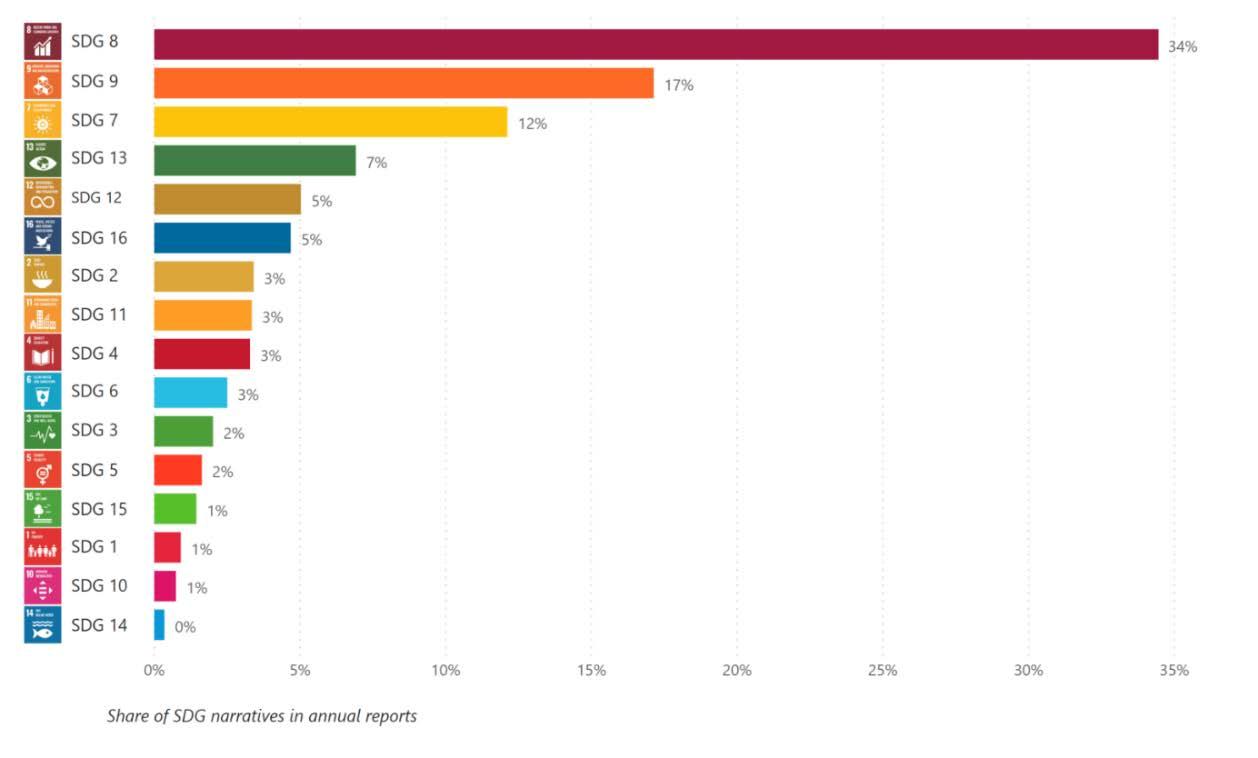
Box 4. Do IDFC members have significantly different SDG narratives? (contd.)
3.2. Multivariate analysis
To complete our observations, we use a clustering analysis. This multivariate analysis method makes it possible to identify the types of PDBs that share a similar position vis à vis the SDGs. It is no longer a question of interpreting the results of the SDG Prospector according to a defined typology, but rather to identify whether there are recurring patterns in the data. Our hypothesis is that multivariate analysis should confirm that PDBs with similar characteristics tend to share the same SDG narrative.
To partition the data into homogeneous groups, we use the non supervised classification algorithm K means. The algorithm automatically groups banks with a similar SDG narrative into different classes. In this type of approach, it is up to the analyst to select the number of
19
We only had access to 24 of the 27 members’ annual reports in English As such, the analysis does not cover all IDFC members.
relevant classes, depending on the interpretability of the results. After analysis, we identify 5 narrative structures which can be grouped in distinct classes. Table 2 presents the resulting classification.
There seems to be a strong core of PDBs committed to supporting SMEs, growth and employment. The narrative of these institutions is largely structured around SDG 8 “Decent Work and Economic Growth” which occupies more than 60% of their annual reports. In contrast, SDG 13 is systematically under represented in relation to the documentation of the rest of the sample.
Table 2. Results from clustering. Number of banks in brackets
Name assigned to each class
Number of BPD per class
Banks supporting SMEs, growth and employment, à la croissance et à l’emploi 84
General purpose banks mainly located in Europe 86
Agricultural banks 11
Housing banks 11
Middle class 45
Key Features
• 70% of BPD have a mandate to support SMEs
• High prevalence of SDG 8 “Growth and Decent Jobs”
• Environmental SDGs are systematically under represented
• SDGs 1, 6, 14 and 15 are absent from the narrative
• The majority is located in Europe
• The narrative covers the entire SDG rainbow
• SDG 13 “climate” occupies 10% of the narrative and is overrepresented relative to PDBs outside Europe
• SDG 8 share remains majority (24%) but relatively lower than other PDBs
• 100% of BPD have an AGRI mandate
• SDG 2 “Zero Hunger” occupies 52% of the narrative
• SDGs 1 “No Poverty” and 6 “Water and Sanitation” are overrepresented
• The place given to the biodiversity SDGs is low but in the average narrative of the other PDBs
• Most PDBs have a social housing funding mandate (HOUS)
• SDG 11 occupies more than half of the narrative
• Tackling inequality (SDG 10) is a predominant topic
• SDGs 1, 2, 3, 14 and 15 are missing
• No significant narrative
• Includes 50% of banks with INFRA mandates
The multivariate analysis highlights groups, which reflect several types of traditional mandates. We distinguish:
• Generalist institutions, mostly located in Europe, whose narrative covers the entire SDG rainbow, like British International Investment or the FMO.
• A group of 11 banks specialized in housing financing. Composed in particular by Fannie Mae and Freddie Mac, which are among the 3 largest PDBs in the world, these
banks have more than 550 billion USD in assets on average. SDG 11 “Sustainable Cities and Communities” is strongly prevalent, occupying more than half of the annual reports of the banks that make up this class, or more than 10 times the share attributed to SDG 11 by all PDBs.
• Institutions with an agricultural development mandate presenting a narrative characterized by a high prevalence of SDG 2 “Zero Hunger” (52%), coupled with a greater consideration of SDG 1 “No Poverty”. As such, this class integrates the Agricultural Development Bank of China as well as the IFAD, which is the only multilateral bank with a specific mandate on rural development. Smaller banks such as Tanzania Agricultural Development Bank and the Agricultural Bank of Zimbabwe also integrate this class.
• Finally, we observe a middle class, characteristic of clustering analyses. The banks that make up this class do not display a singular narrative. We note that more than half of the banks with EXIM mandates are in this class.
To summarize, the SDG Prospector highlights the prevalence of productive SDGs in PDBs’ strategic positioning. The use of clustering analysis underlines the consistency of the results. Despite the limitations outlined below, the SDG Prospector’s ability to analyze consistent and unified information flows suggests new applications, both academically and operationally.
4. Discussion
The use of artificial intelligence to map the content of Public Development Banks' annual activity and sustainable reports provides convincing results. However, this approach has a number of conceptual and methodological limitations, which we discuss here. Despite these, an emerging conclusion is that the use of AI methods to track the SDGs holds promising potential. Whether at the academic or operational level, more advanced versions of the SDG Prospector should allow mapping project descriptions, and link them with their expected impacts.
We distinguish conceptual boundaries, which question the assumptions underlying the interpretation of the results, from methodological limitations, which stem from the very structure of the tool.
Conceptual aspects
The use of an automatic text analysis method allows significant time savings but requires certain precautions in interpreting the results
First, the SDG Prospector does not understand whether references to a given SDG occur at the beginning, at the end or in the body of the documents. One could imagine a bank, which would be highly committed to climate action that would only indicate once, at the beginning of its report that all its projects comply with the Paris Agreement on climate, while another bank, less committed in practice, would mention its climate efforts throughout its report. The SDG Prospector would then assign a low score to the first, while the less committed but more dissected bank would get a higher percentage associated with the consideration of SDG 13 «Climate action».
Our method is strongly dependent on PDBs’ transparency and access to publicly available information. A first best solution would be to analyze project descriptions directly as they are likely to reflect what PDBs’ activities. However, only a limited number of banks publish information on all their projects. These are usually multilateral institutions, such as the World Bank, which are not subject to confidentiality obligations nor national bank regulations. We acknowledge that activity and sustainable reports are only proxies for PDBs’ concrete actions.
By simply analyzing narrative content, one could object that the SDG Prospector captures PDBs’ positive communication. However, this reservation falls on a large sample, since the results (see section 3.2) show that the majority discourse remains focused on the productive SDGs, and that the SDGs most prone to “sustainable washing” (climate, biodiversity, gender) occupy a relatively small proportion of banks’ narrative.
Furthermore, there is no evidence to justify a widespread suspicion of complacency on behalf of all PDBs. Our hypothesis is that, in all cases, discourse “precedes action”: as public bodies, PDBs are accountable to the citizens of their countries (Xu and Carey, 2015). Their reports are scrutinized and audited, most often by independent organizations. If annual reports involve a significant amount of communication, it cannot be completely disconnected from reality. One might add that, conversely, there is a very low probability that a PDB will not mention one of the SDGs in its institutional documentation, even though it contributes significantly to its funding.
Another limitation is that, while the SDG Prospector can identify and contextualize any SDG in a paragraph, it is not able to determine whether this SDG is evoked for a positive contribution (e.g., “our project contributes to poverty reduction”), or as a limitation (e.g., “our project does not aim to combat poverty”). However, the bias is negligible in the case of annual reports, which reflect on the activity of the past year. All institutions tend to favor a discourse around their contributions, contrarily to exposing the limits of their action. In addition, it is technically possible to exceed this limit through a sentiment analysis. We leave that for future research.
Methodological aspects
The SDG Prospector is limited to reading documents in English. This restricts our scope of analysis as the tool is not able to process PDBs that publish their report exclusively in another language. This results in some geographical bias in our sample (see 2.3.2). The possibility of applying the SDG Prospector to other languages is one of the developments to be foreseen. This will require translating documents into English using automatic translators, or using algorithms designed specifically to handle other languages, such as CamemBERT or FlauBERT for French texts.
As described in Section 3.1., we did not have access to annual reports for every banks and every year over the 2016 2020 period. To balance our panel, we assigned average observations for any gap in the data. Even though this technique allows avoiding sampling bias, it tends to freeze SDG narratives and reduces inter annual variability. However, unless a bank undergoes a sudden change in its mandate, we expect SDG narratives to evolve only marginally from one year to the other. This assumption is confirmed by the data when annual reports are available over the 5 year period.
Finally, one can be concerned by the environmental cost of language models, due to the energy consumption required for their training (Bender et al., 2021). The BERT training alone would require as much energy as flying an airplane across the United States of America. DistilRoBERTa is a light version of the BERT model and requires fewer energy. However, anticipating future developments in NLP methods, special attention will have to be paid to the environmental performance of the tools used.
Conclusion
At the time of high frequency trading based on data produced every second by the financial reporting system, how can frontier technologies help monitoring extra financial information? Thanks to the SDG Prospector, which uses a language model to identify the SDGs in a text, we explore the application of artificial intelligence methods to describe Public Development Banks’ narrative with respect to the 2030 Agenda. Our methodology is more robust than key words analyses and allows us to process large amounts of text.
Applying the SDG Prospector to the institutional documentation of 237 institutions over the 2016 2020 period, we show that PDBs’ strategic and operational narrative is mainly structured around “productive” SDGs, grouping together themes such as economic growth, employment, industrial development, infrastructure and innovation. In addition, the results suggest that the larger a PDB, the more its documentation refers to the SDGs associated with environmental protection, but climate ranks higher than biodiversity issues in their agenda. These latter as well as cross cutting objectives such as gender equality or poverty reduction concentrate a low share of PDBs’ narrative on average.
In resonance with the Finance in Common coalition 20, our research contributes to increasing knowledge on Public Development Banks. By estimating the degree of importance PDBs attach to the different SDGs, our results constitute a database for future research, and could contribute to the literature on official development assistance and donor priorities. More broadly, the SDG Prospector opens up other research opportunities in social sciences, which already apply machine learning methods (Forest & Foreste, 2022).
The SDG Prospector is an example of an effective and robust application of artificial intelligence methods to the 2030 Agenda. It paves the way for future research to strengthen the use of intelligent tools, both at the academic level and in the operations of development actors. As such, our work is part of the overall reflection on alignment measures.
SDG alignment is a major challenge for the financial sector and for public and private investors: to what extent do “green” portfolios contribute to the fight against climate change and the protection of biodiversity? Are the impacts of “green” bond backed projects mapped out based on secure and comparable data? Once the investment decision has been made, which system ensures the monitoring and guarantees that commitments are respected? All these questions require a robust, reproducible, and as little arbitrary information processing as possible. Our research shows that an artificial intelligence tool, backed by a homogeneous and high quality documentary source, can reveal content from massive documentation that would be difficult, otherwise impossible, to process manually with the same degree of consistency and precision.
In order to operationalize the SDG Prospector, in particular by allowing it to analyze a portfolio of projects in a relevant way, it would be necessary to expand its training base, and to adjust its model to permit to precisely identify the 169 targets set out by the 2030 Agenda, and their interactions. This would allow developing a holistic vision, in line with the framework of the SDGs.
Eventually, the use of frontier technologies in the field of sustainability is likely to accompany its visibility, its rise and the quality of the information that decision makers need to promote necessary transitions.
Abbreviations
AGRI Agricultural and rural development mandate
AI Artificial Intelligence
BERT Bidirectional Encoder Representation from Transformers
CSR Corporate Social Responsibility
DAC Development Assistance Committee
ESG Environmental, Social, and Governance
EXIM Export and trade promotion mandate
FIC Finance in Common
FLEX Generalist mandate
FMO Dutch Entrepreneurial Development Bank
HOUS Social housing promotion mandate
IDFC International Development Finance Club
IFAD International Fund for Agricultural Development
INFRA Infrastructure promotion mandate
INTL Funding mandate of the international private sector
KfW Kreditanstalt für Wiederaufbau
LOCAL Funding mandate for local authorities
MCC Matthews Correlation Coefficient
MSME Funding mandate for Micro, Small and Medium sized Enterprises.
NGO Non governmental organization
NLP Natural Language Processing
OECD Organisation for Economic Cooperation and Development
PDB Public Development Banks
SDG Sustainable Development Goals
SMEs Small and Medium Enterprises
TF-IDF Term Frequency Inverse Document Frequency
TNFD Taskforce for Nature related Disclosure
TSKB Turkiye Sinai Kalkinma Bankasi
UN United Nations
UNDESA United Nations Department of Economic and Social Affairs
WWF World Wide Fund for Nature
References
Agence Française de Développement and United Nations Entity for Gender Equality and the Empowerment of Women (2021), Public Development Banks Driving Gender Equality an Overview of Practices and Measurement Frameworks.
Attridge S., Xu J., Gallagher K.P. (2020),
Piloting and Scaling Up Clean Energy Transitions: the Role of Development Finance Institutions, AFD Research Papers No. 178.
Bender E.M., Gebru T., McMillan Major A. & Shmitchell, S. (2021),
On the Dangers of Stochastic Parrots: Can Language Models Be Too Big? , in Proceedings of the 2021 ACM Conference on Fairness, Accountability, and Transparency (pp. 610 623), ACM, New York, NY, USA, https://doi.org/10.1145/344218
8.3445922
Devlin J., Chang M W., Lee K. & Toutanova K. (2018),
BERT: Pre training of Deep Bidirectional Transformers for Language Understanding, Google AI Language, https://arxiv.org/abs/1810.04
805
Forest M. & Foreste C. (2022), Quels cadres interprétatifs autour des enjeux Genre et Climat? Enseignements d’une analyse bibliométrique, AFD Research Papers No. 255.
Fuchs S., Kachi A., Sidner L., Westphal M., Lawless B. & Ryfisch D. (2021), Aligning Financial Intermediary Investments with the Paris Agreement, Working Paper. Washington, DC: World Resources Institute. Available online at https://doi.org/10.46830/wriw p.20.00037
Guisiano J. & Chiky R. (2021), Automatic classification of multilabel texts related to Sustainable Development Goals, TECHENV EGC2021, Montpellier, France. hal 03154261
Griffith-Jones S., Barrowclough D., Mishra V. (2022), How are Development Banks managing the Covid 19 crisis and planning for a transition towards recovery? Lessons for the future.
Griffith Jones S. & Ocampo J.A. (2018), The Future of National Development Banks, Oxford: Oxford University Press.
Gutierrez E. & Kliatskova T. (2021), National Development Financial Institutions: Trends, Crisis Response Activities, and Lessons Learned, EFI Insight Finance. Washington, DC: World Bank.
Himberg H, Xu J. & Gallagher K.P. (2020), Climate Change and Development Bank Project Cycles, AFD Research paper no. 180.
Höfling H., Börner M. & Dangelmaier U. (2019), The Sustainable Development Goals SDG reporting by banks, KfW Research No. 267.
International Development Finance Club (2021), IDFC Green Finance Mapping. https://www.idfc.org/wp content/uploads/2021/11/idfc gfm2021 full report final.pdf
Joshi A., Gonzalez Morales L., Klarman S., Stellato A., Helton A., Lovell S. & Haczek A. (2020), A Knowledge Organization System for the United Nations Sustainable Development Goals, OpenReview.net
LaFleur M.T. (2019), Art is Long, Life is Short: an SDG Classification System for DESA Publications, DESA Working Paper No.159.
Liu Y., Ott M., Goyal N., Du J., Joshi M., Chen D., Levy O., Lewis M., Zettlemoyer L. & Stoyanov V. (2019),
RoBERTa:Facebook AI, https://arxiv.org/abs/1907.11692
Marodon R. (2021), Can Development Banks step up to the Challenge of Sustainable Development? , Review of Political Economy, Volume 34, Issue 2, pp. 268 285.
McCarthy, J., Minsky, M.L., Rochester, N. & Shannon, C.E. (1955), A Proposal for the Dartmouth Summer Research Project on Artificial Intelligence.
McDonald D.A., Marois T., Barrowclough D. (2020),
Public Banks and COVID 19: combatting the pandemic with public finance, Municipal Services Project (Kingston), UNCTAD (Geneva) and Eurodad (Brussels).
Miola A., Borchardt S., Neher F. & Buscaglia D. (2019), Interlinkages and policy coherence for the Sustainable Development Goals implementation An operational method to identify trade offs and co benefits in a systemic way, JRC Technical Reports, European Commission.
Morris S. (2018), The International Development Finance Club and the Sustainable Development Goals Impact, Opportunities and Challenges, Center for Global Development, Washington D.C.
Muñoz Cabré M., Ndhlukula K., Musasike T., Bradlow D., Pillay K., Gallagher K.P., Chen Y., Loots J. & Ma X. (2020), Expanding Renewable Energy for Access and Development: the Role of Development Finance Institutions in Southern Africa, Global Development Policy Center, Boston University. Ocampo J.A. & Ortega V. (2021), The Global Development Banks’ Architecture, Review of Political Economy, Volume 34, Issue 2, Pages 224 248.
Pincet A., Okabe S. & Pawelczyk M. (2019), Linking Aid to the Sustainable Development Goals A Machine Learning Approach, OECD Working Papers.
Pukelis L., Bautista Puig N., Skrynik M. & Stanciauskas V. (2020), Open Source Approach to Classify Text Data by UN Sustainable Development Goals (SDGs), https://doi.org/10.48550/arXiv.2 005.14569
Riaño M.A., Boutaybi J., Barchiche D. & Treyer S. (2021,a), Scaling Up Public Development Banks’ Transformative Alignment with the 2030 Agenda for Sustainable Development, Review of Political Economy, Volume 34, Issue 2, pp. 286 317.
Riaño M.A., Attridge S., Bilal S., Keijzer R., Erforth B., Fattibene D., Hege E., Evans M., Olivié I. & Barchiche D. (2021,b),
Financing the 2030 Agenda
An SDG Alignment Framework for Public Development Banks, European Think Tank Group.
Smits S. and Rodríguez M. (2022), Public Development Banks in the Water Sector Case studies from Latin America, AFD Technical Reports No. 65.
Svartzman R., Spain E., Gauthey J., Hadji Lazaro P., Salin M., Allen T., Berger J., Calas J., Godin A., Vallier A. (2021), A “Silent Spring” for the Financial System? Exploring Biodiversity Related Financial Risks in France, Banque de France Working Paper No. 826.
United Nations (2002),
The Monterrey Consensus, International Conference on Financing for Development, Monterrey, Mexico.
United Nations (2015),
Addis Ababa Action Agenda, Outcome Document of the Third International Conference on Financing for Development.
WWF & The Biodiversity Consultancy (2021), Public development banks and biodiversity: How Development Finance Institutions can align with the Post 2020 Global Biodiversity Framework. Full Report, WWF France.
Xu J. & Carey R. (2015),
Towards a Global Reporting System for Development Cooperation on the SDGS: Promoting Transformational Potential and Impact, IDS Working Paper No. 462.
Xu J., Marodon R. & Ru X. (2021), Mapping 500+ Development Banks Qualification criteria, stylized facts and development trends, AFD Research Papers No. 192.
Appendix 1. Geographic distribution of sampled PDBs
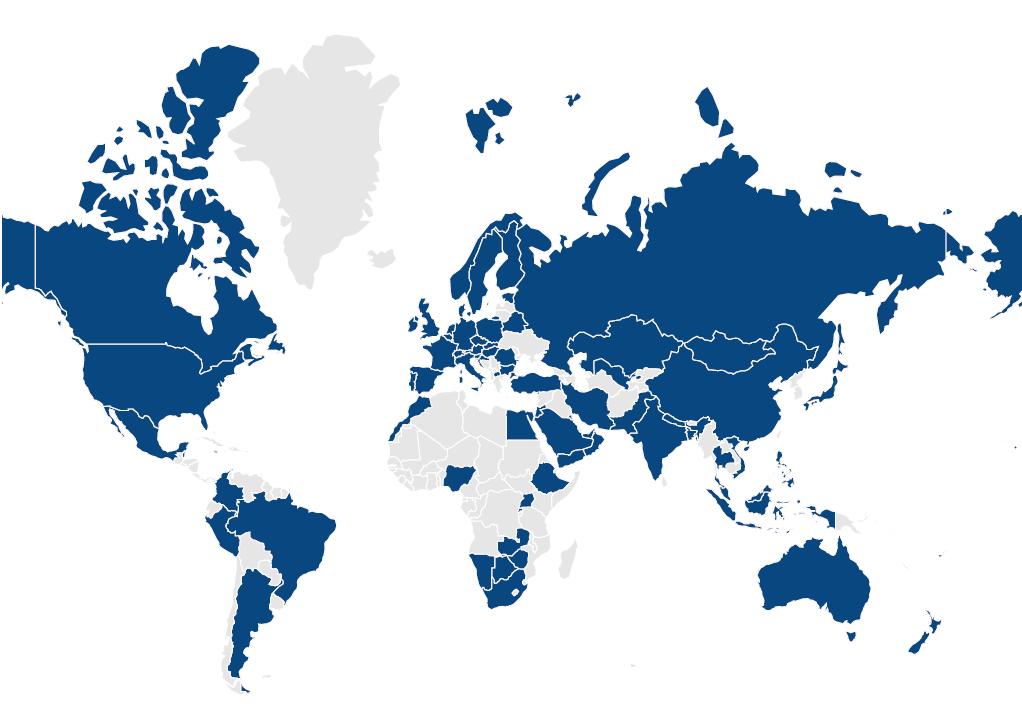
Banks’ main characteristics
PDBs ' total population
Sample Sample representation
Absolute value % Absolute value % % of PDBs total population
World 522 100% 237 100% 45%
Continent
Africa 102 20% 35 15% 34%
America 118 23% 32 14% 27%
Asia 148 28% 80 34% 54%
Europe 126 24% 77 32% 61%
Oceania 20 4% 8 3% 40%
World 8 1% 5 2% 63%
Income group*
HIC 175 34% 95 40% 54%
UMIC 134 26% 60 25% 45%
LMIC 137 26% 37 16% 27%
LIC 21 4% 4 2% 19%
Ownership structure
MULTI 55 11% 41 17% 75%
NATIONAL 356 68% 175 74% 49%
SUBNATIONAL 111 21% 21 9% 19%
Asset size**
Mega 11 2% 9 4% 82%
Large 20 4% 16 7% 80% Medium 43 8% 38 16% 88% Small 201 39% 118 50% 59%
Micro 186 37% 54 23% 29%
Mandate
AGRI 35 7% 12 5% 34%
EXIM 54 10% 35 15% 65%
FLEX 181 35% 83 35% 46%
HOUS 37 7% 16 7% 43% INFRA 31 6% 11 5% 35%
INTL 30 6% 27 11% 90%
LOCAL 17 3% 7 3% 41%
MSME 137 26% 46 19% 34%
National and sub national PDBs only.
data
institutions.
SDG




Name Country
Nordic Development Fund Multi
1% 53% 2% 1% 56%
Environmental Investment Fund Namibia 5% 37% 0% 13% 55%
Kommunalbanken Norway 8% 41% 0% 0% 49%
Swedish Export Credit Corporation Sweden 22% 20% 0% 0% 42%
Kreditanstalt für Wiederaufbau* Germany 21% 19% 0% 2% 42%
Kommuninvest Sweden 20% 20% 0% 0% 40% Municipal Bank (BNG Bank) Netherlands 19% 15% 0% 0% 34%
Caisse des Dépôts et Consignations France 8% 21% 0% 3% 32%
Nordrhein Westfalen Förderbank Germany 17% 13% 0% 2% 32%
Turkiye Sinai Kalkinma Bankasi (TSKB) * Turkey 16% 15% 0% 0% 31%
Name Country
Development Finance Institute Canada 0%
31%
The World Bank Multi 8% 7% 7% 7% 0% 30%

Islamic Development Bank Multi 4% 9% 11% 4% 0% 29%
Agence France Locale France 0% 5% 1% 17% 6% 28%
Local Investment Finance Company France 0% 8% 9% 4% 5% 26%
Latin American Bank for Foreign Trade Multi 0% 3% 20% 1% 2% 26%
Citizen Entrepreneurship Development Agency
Botswana 0% 8% 3% 5% 10% 26%
Japan International Cooperation Agency* Japan 2% 9% 10% 3% 1% 25%

Al Amanah Islamic Investment Bank of the Philippines Philippines 2% 1% 14% 6% 2% 25%
Development Bank of Nigeria Nigeria 1% 3% 1% 5% 13% 24%



Main SDGs




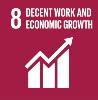


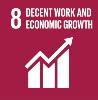
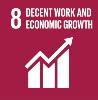





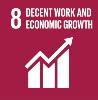

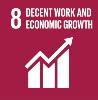

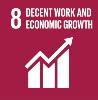

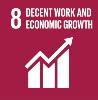

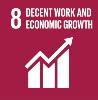

Number of classes Class type
clustering
SDG
5 classes
SDG
SDG
SDG
SDG
SDG
SDG
SDG
SDG
SME support banks (84) 0% 4% 2% 2% 1% 0% 6% 60% 12% 1% 2% 3% 2% 0% 0% 3%
General purpose banks mainly located in Europe (86)
Agricultural banks (11)
Housing banks (11)
Middle class (45)
TOTAL (237)
1% 5% 3% 3% 4% 4% 16% 24% 10% 1% 5% 6% 10% 0% 1% 7%
2% 52% 1% 2% 2% 3% 3% 14% 9% 1% 1% 3% 4% 0% 1% 3%
0% 0% 0% 1% 2% 3% 4% 17% 8% 2% 52% 2% 3% 0% 0% 5%
1% 4% 2% 2% 1% 3% 12% 26% 33% 0% 3% 4% 4% 0% 0% 4%
1% 6% 2% 3% 2% 2% 11% 36% 15% 1% 6% 4% 5% 0% 1% 5%
Note: Percentages represent the share of the narrative of each SDG in the PDBs’ activity reports. Values in green (red) identify SDGs with a narrative that is higher (lower) than what is observed at the sample level.
What is AFD?
Éditions Agence française de développement publishes analysis and research on sustainable development issues. Conducted with numerous partners in the Global North and South, these publications contribute to a better understanding of the challenges faced by our planet and to the implementation of concerted actions within the framework of the Sustainable Development Goals.
With a catalogue of more than 1,000 titles and an average of 80 new publications published every year, Éditions Agence française de développement promotes the dissemination of knowledge and expertise, both in AFD’s own publications and through key partnerships. Discover all our publications in open access at editions. afd.fr.
Towards a world in common.
Publication Director Rémy Rioux
Thomas MelonioLegal deposit
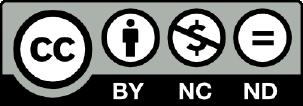
Rights and permissions
Graphic design MeMo, Juliegilles, D. Cazeils
Layout Denise
To browse our publications: