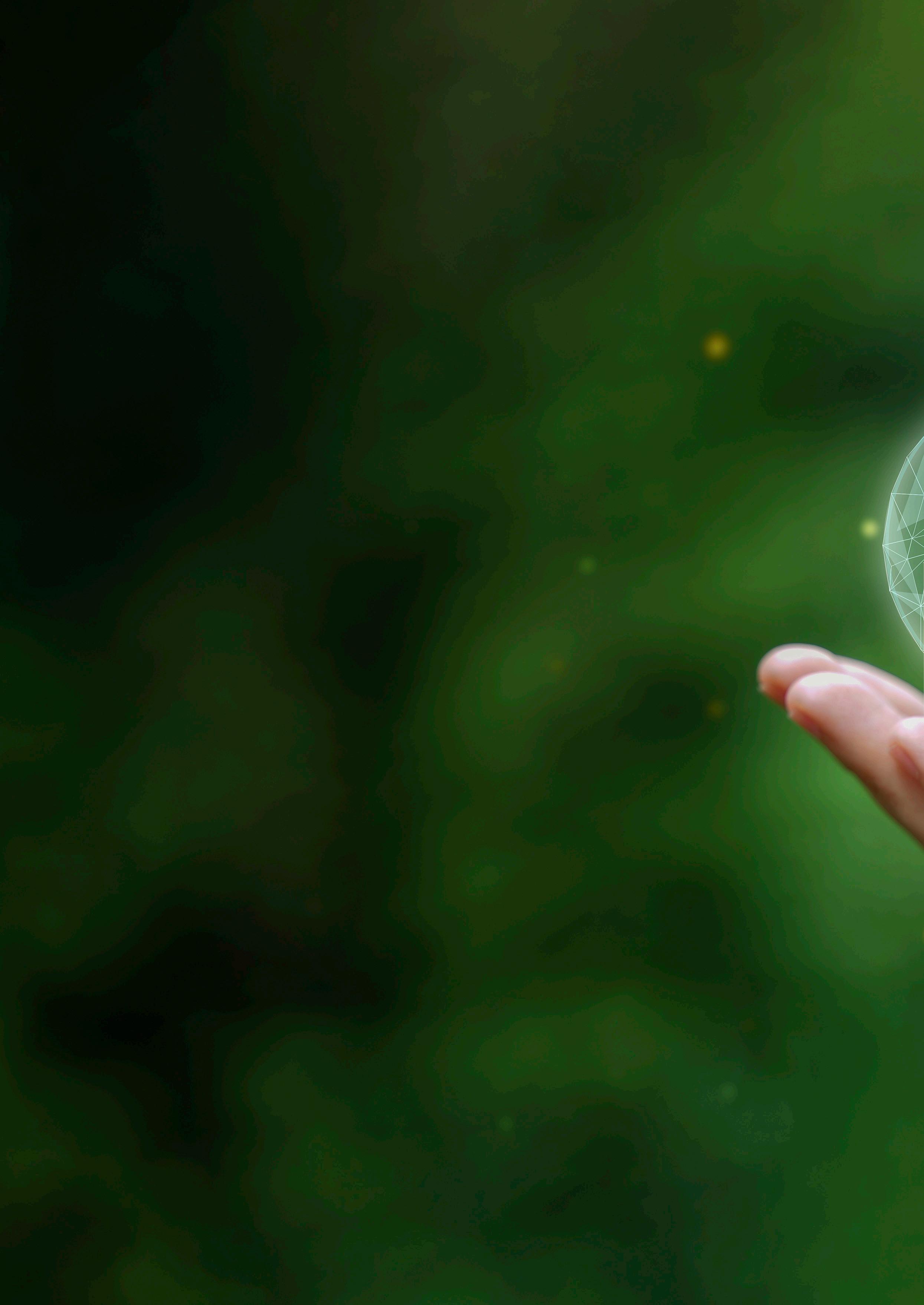
10 minute read
From wasteful to digital
aste incineration is the last resort of recycling management and is becoming more and more important. What cannot be reused or recycled is burned. Only little residual quantities should be dumped. The energetic utilisation of waste and residual materials has a long tradition; however, the inhomogeneous fuel involves some challenges. These can be mastered by means of digitalisation.
Waste-fired combined heat and power (CHP) plants, which are a variety of thermal waste treatment plants, currently have high utilisation rates. After all, a waste-fired CHP plant works just like other power plants. The fuel is transported to a furnace where it is burned continuously by means of a combustion grate while air is supplied. The heat drives generators that produce electricity; the waste heat is used in heat grids, and the steam is directly applied for industrial purposes.
In most cases, a higher energetic utilisation rate is only feasible by increasing the throughput. This, however, is only possible by optimising the combustion process. Thus, downtimes can be reduced and the hours of operation can be increased. One technique for this purpose consists in condition-based maintenance, which has been tried and tested owing to digitalisation and artificial intelligence (AI).
A largely trouble-free process of this kind is only possible by means of a process quality monitoring and condition monitoring of the thermal waste treatment plant. For this, all relevant process data has to be captured, processed, and provided to the control system according to the principles of big data and machine learning in real-time. This also means that anomalies can be detected, as well as creeping changes, and they can be reacted to as quickly as possible – also automatedly.
Inhomogeneous fuel requires more control
The fuel of waste-fired CHP plants requires pre-processing, such as grading and drying, in order to neutralise some of the inhomogeneity. In spite of this, it is impossible to achieve a gross calorific value similar to that of oil, gas, wood, or coal. Moreover, external factors such as the weather, quality, load, or contaminants contained in the waste, as well as others, need to be considered.
Here, only a continuous monitoring of the combustion process and regular interventions help to generate the products electricity, heat, and steam continuously and with due quality.
In 2009, STEAG Energy Services implemented a visual and digital monitoring of the combustion process at a waste-fired CHP plant in Spain. The PiT Navigator Waste applied here is a system developed by STEAG on the hardware and software side. It consists of a combination of a video camera and a thermographic camera in a refractory housing. The cameras are oriented from the rear towards the end-most combustion zones, the fire end line.
The data is sent to the process control system, which uses AI methods for the computationally intensive tasks, via a standard interface. At the same time, it is shielded from possible cyber-attacks from the outside. If the process control system has to be replaced, only the interface will be adjusted. The furnace capacity control will remain unchanged, which ensures an immediate and reliable operation.
The user interface of the PiT Navigator Waste enables programming either on a separate monitor or as an additional input screen in the process control system. Thus, all customer requirements for a clear visualisation can be fulfilled.
The benefits consist in:
F A lower fluctuation of the steam output.
F An improved burnout.
F Decreased emission peaks for carbon monoxide (CO) and nitrous oxide (NOX) emissions.
Simon Geiger, STEAG Energy Services, Germany, details how digitalisation can make waste incineration more efficient in waste-fired combined heat and power plants.
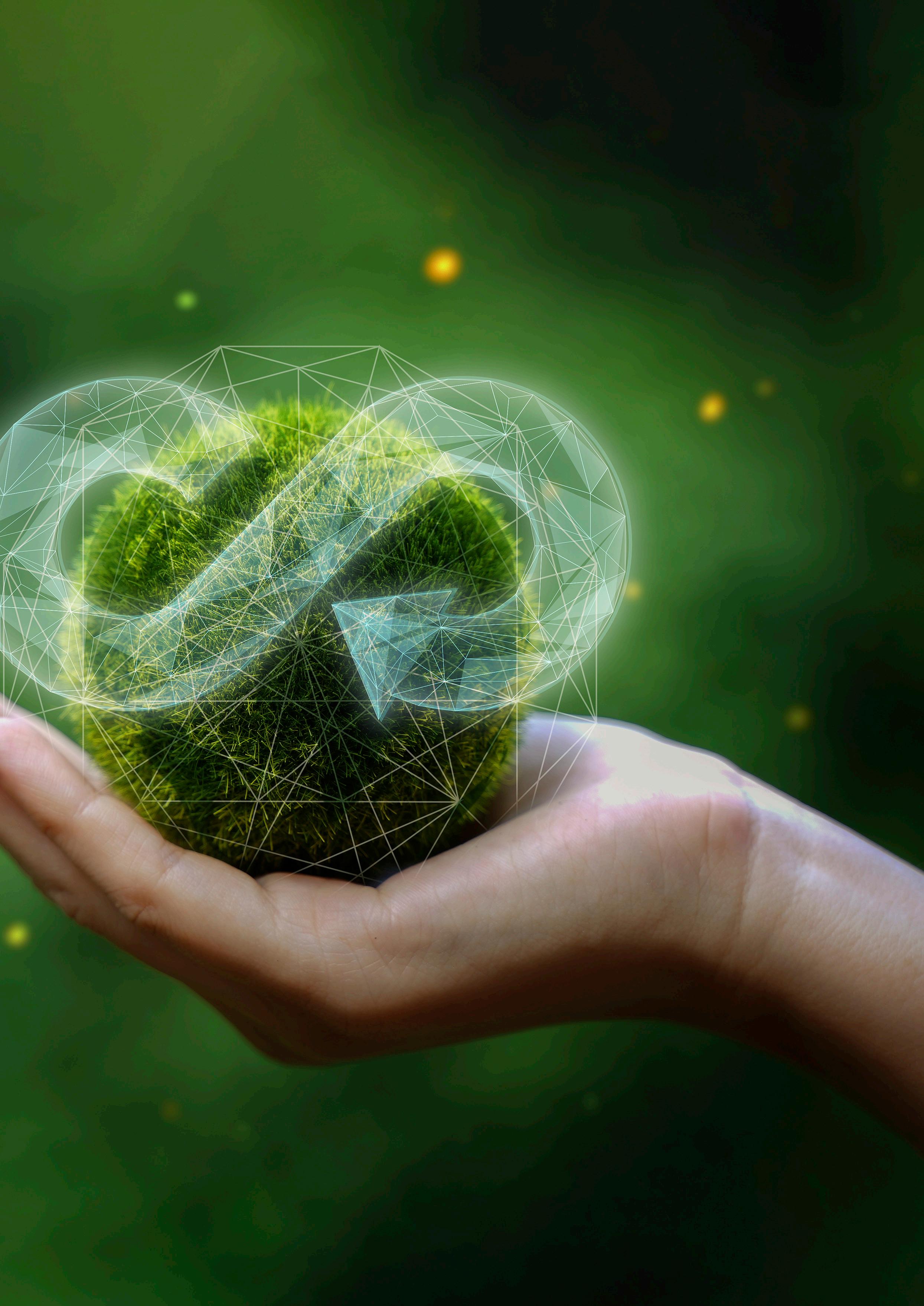
Neural networks control the plant
This, in turn, optimises the furnace capacity control. Owing to neural networks, an advanced process control, and intelligent data processing, the data thus gained enables an advanced steam projection for the following 2 – 5 mins. in each case. This again allows downstream controllers to react to a steam peak before it occurs by means of the actuating variables and to minimise the deviation of the generated steam quantity from the reference value. Thus, the system follows the methods of artificial intelligence (AI). However, it is possible to manually intervene in the process at any time. The procedure described here can also be applied to other important process variables of the plant.
This use of data, which is ground-breaking for waste-fired CHP plants, enables the simultaneous setting of parameters such as steam output and combustion temperature by means of the air quantity, air temperature, or grate speed. All controls are completely adaptive. They are retrained and optimised fully automatically at regular intervals. Grate trimming, air distribution by means of primary air and secondary air fan, as well as other parameters can be manually influenced by the plant operator at any time.
In 2021, an adjustment of the plant in Spain was carried out that mainly affected the hardware and software. The operation has been simplified significantly. Several options are now suggested to the plant operators, depending on the situation; they can still enter information manually.
One problem that can be solved this way is that of wet waste or waste of bad quality, which frequently occurs in rainy Spain. It would impede the combustion process. Owing to weather data, which are imported by AI-based systems as well and influence the control of the plant, it is now possible to detect when bad waste occurs.
The system of the PiT Navigator Waste is subject to continuous development. It has been implemented at other plants all across Europe. One advantage consists in the fact that this can be done during operation.
Start 2009
Objectives:
F Reduction of standard deviation of steam fluctuations by 25% (achieved: 45%).
F Increase in steam quantity by 3% (achieved: 5.9%).
F Reduction of the standard deviation for steam to 3.3 tph (achieved: 1.8 – 1.9 tph; 2021, after the update: 1.2 tph).
F After the update of 2021, the steam reference value of 44 t can be increased by 4 t or 10%.
General benefits owing to the PiT Navigator Waste:
F Stabilisation of the steam output, resulting in 3% higher waste throughput (control deviation for steam smaller than 3%).
F Improved combustion process, resulting in better greenhouse gas (GHG) emissions (40% less CO peaks, 10% less NO X standard deviation).
F Return on investment is achieved in less than one year.
More plant efficiency due to SR::SPC Predictive Analytics
Figure 1. HMI overview page of PiT navigator waste. Alongside the PiT Navigator Waste, the early warning system SR::SPC Predictive Analytics developed by STEAG also ensures the early detection of creeping and critical changes by monitoring processes and main components of a waste-fired CHP plant. SR::SPC combines state-of-the-art data analysis with machine learning and AI via big data in real time. From historical, sensor-based data, the system develops digital twins and continuously compares them to the current data. This also allows to immediately detect potential faults and anomalies in the operation or damages
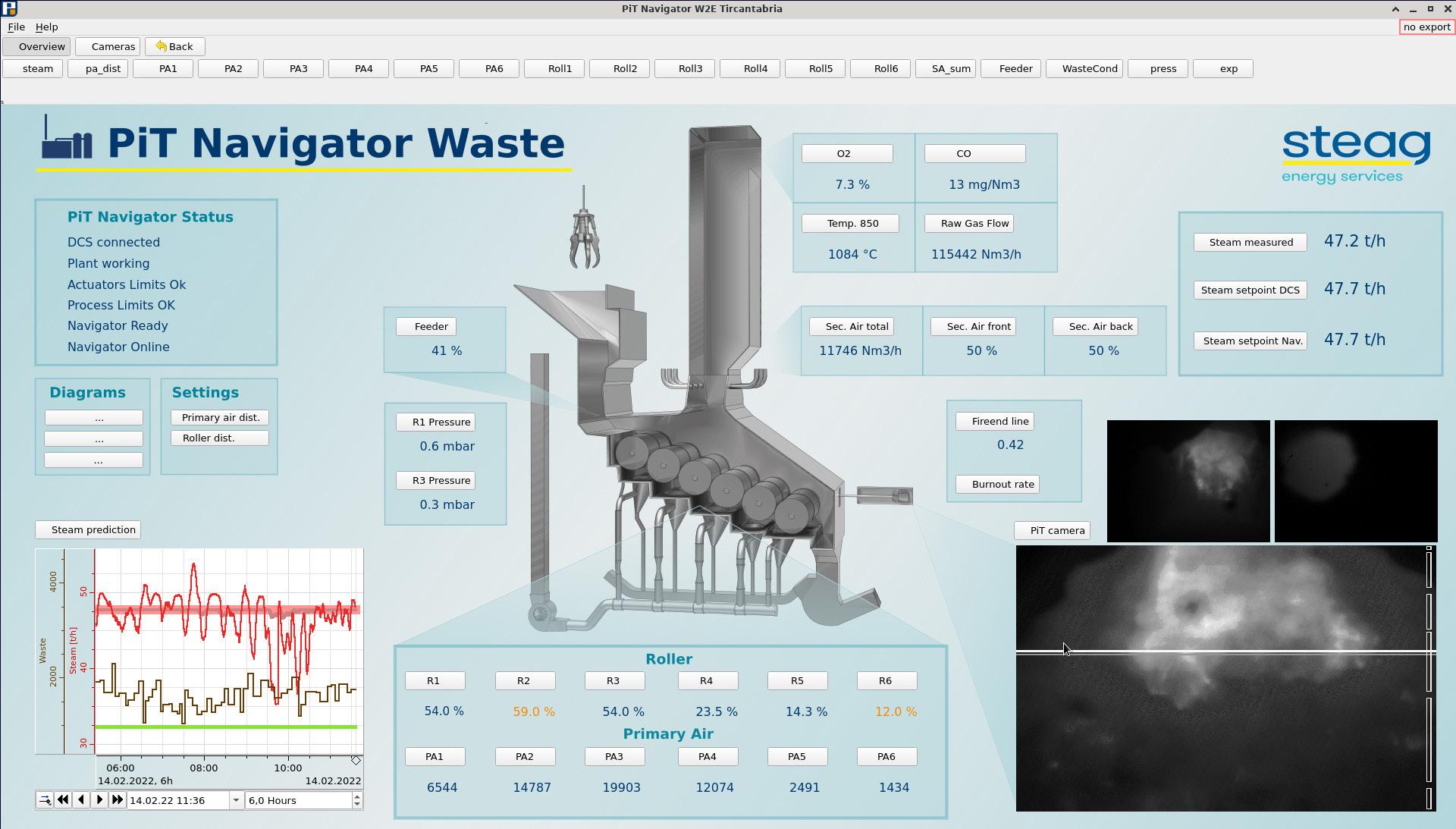
in the plant. This, in turn, enables predictive maintenance and repair of the plant, e.g., during scheduled downtimes or at times with low load.
SR::SPC Predictive Analytics offers the following benefits:
F Transparency owing to processed data and derived key performance indicators (KPIs).
F Early detection of inefficiency and damaged infrastructure.
F Prevention of consequential damage.
F Improved maintenance planning, as well as spare part supply chain.
F Optimisation of the staff deployment.
F Improved availability and efficiency.
F Increasing the availability, and reducing OPEX.
In the case of waste-fired CHP plants in particular, plant components are subject to faster wear and fouling due to the inhomogeneous fuel. This, in turn, leads to downtimes and losses.
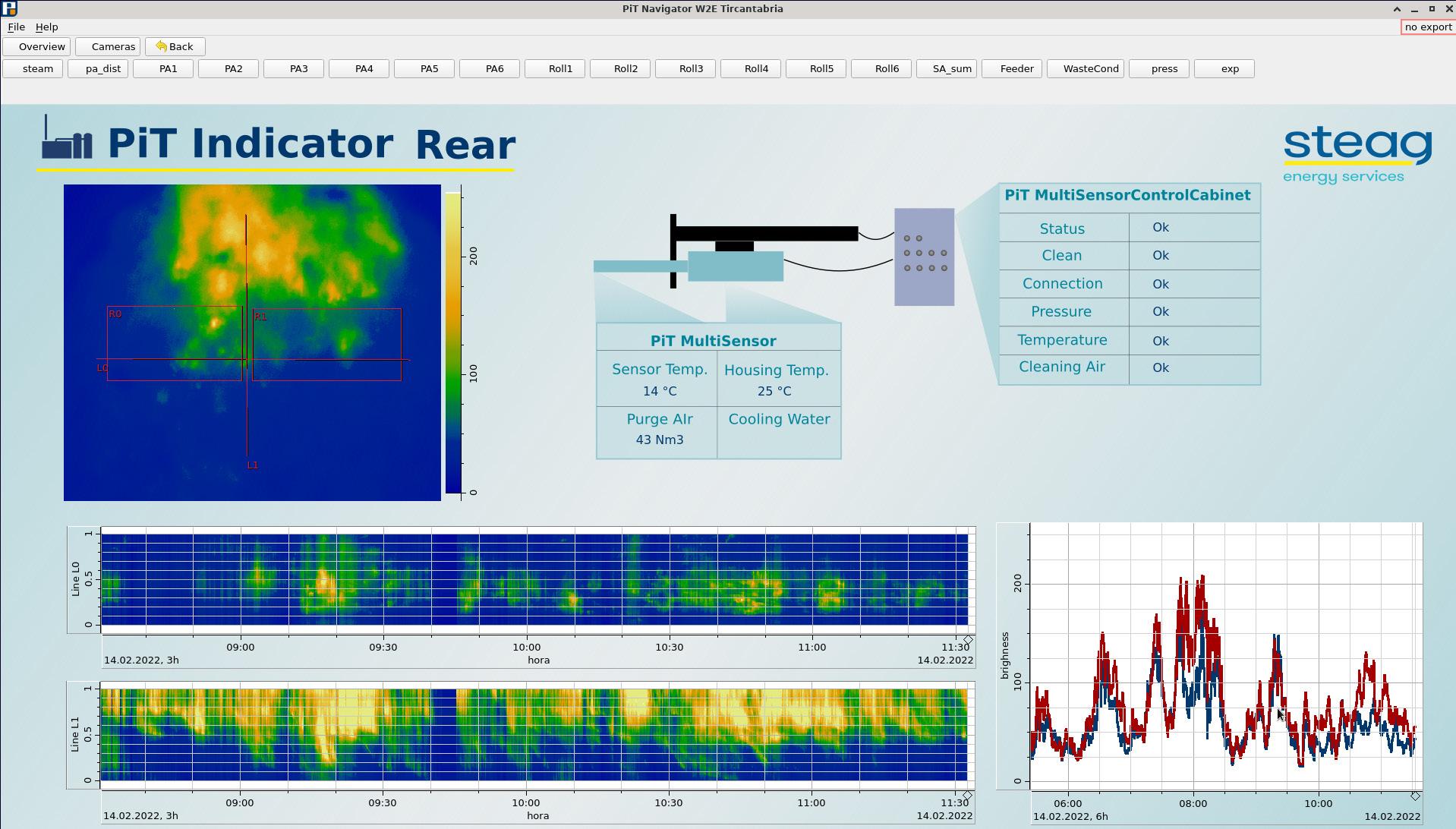
Figure 2. HMI camera page (PiT indicator rear).
Predictive Analytics at MVV
Such a system is already in operation at the utility company, MVV, in Mannheim, Germany. Most of the 467 MW electrical power plant output is generated by eight waste-fired CHP plants and four biomass-fired CHP plants. The production processes are fully automated. Several thousand sensors and actuators serve this purpose in each plant, providing approximately 25 000 measured values every 2 secs. since 1991.
Owing to these data, implementing SR::SPC Predictive Analytics was possible and reasonable here as the arising amount of data made an estimation of the parameters regarding both the process and the condition of the plants only by the staff difficult. Therefore, from October 2018 on, STEAG Energy Services implemented this early warning system at the thermal waste treatment plants of MVV at the Mannheim site over a period of six months.
In a first step, the process connections were upgraded to standardised interfaces and moreover, a central Industrial Internet of Things (IIoT) platform was set up. The sites process control systems were connected to the respective IIoT network segment via an OPC-UA server. Here, each segment has its own linking computer. It transmits the data from the control system to the central long-term data archive via virtual private networks (VPN). Basically, this data can be accessed from each office.
The embedded data management system ensures an adequate visualisation by means of which all processes can be monitored. Deviations will result in an automated alarming.
Essential benefits include:
F Compliance with the KPI nomenclature.
F Activation of all outlier filters.
F Creation of suitable selection filters.
F Existing load indicator.
F Input neurons of all relevant values available.
F Selection of a suitable training period.
F The number of the hidden neurons is consistent with the complexity.
F A match of at least 80% for the test data.
F Creation of a realistic documentation.
The following specific implementations were performed for the MVV plant:
F The superheaters of the steam generator were arranged as contact heating surfaces in the flue
gas stream. This involves a reduction of the flow cross-section due to fouling, which, in turn, needs to be regularly removed by means of explosive cleaning. The alarm values of the plant are adjusted in such a way that this is reported one week in advance.
F KPIs also monitor the bearing vibrations of the ID fan blower, enabling the detection of a higher flue gas humidity and dust load, which can lead to deposit build-up on the rotor of the blower.
F A turbo set of a turbine was analysed with a total of 170 individual values. In doing so, abnormalities of 16 measured values were detected. Their evaluation allowed to carry out a necessary maintenance or complete overhaul early on.
It was proven that AI-based methods and advanced algorithms, such as the deep learning auto-encoder, facilitate the physical modelling for predictive maintenance considerably.
“MVV has evaluated STEAG‘s predictive analytics IT solution, has proven its economic added value, and rolled it out feet-wide. We are delighted with the tool and the co-operation with the team of STEAG,” explained Sascha Schmitt, Planning Engineer for Technology, Engineering, and Digitalisation at MVV Umwelt GmbH in Munich, Germany.
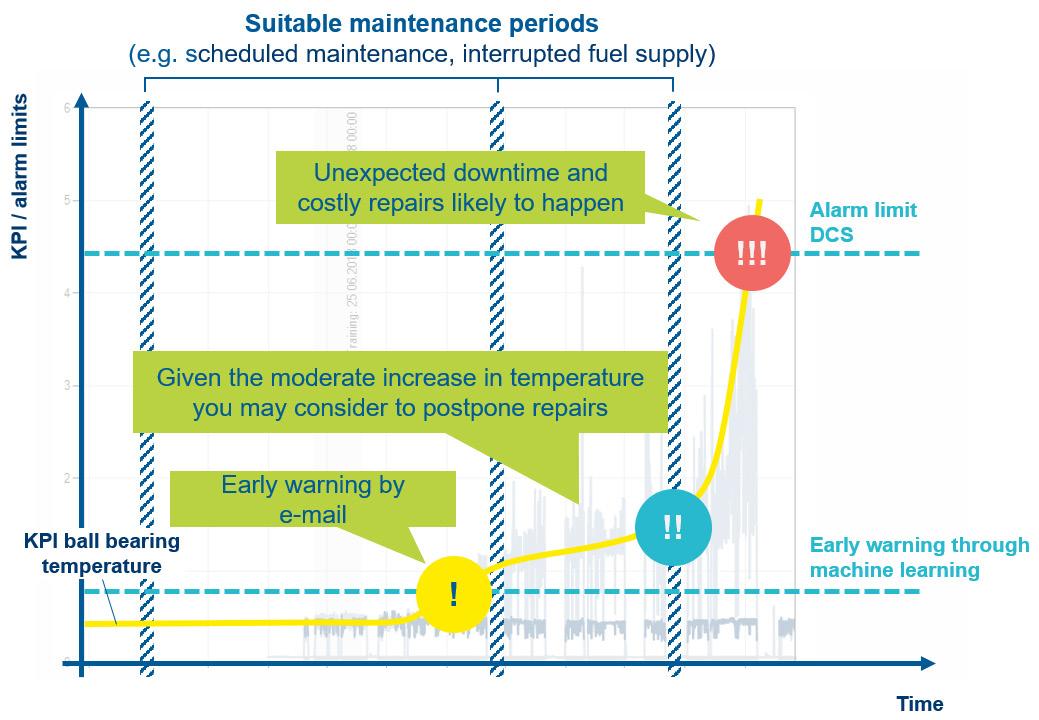
Figure 3. HMI actuator page of the primary air.
Figure 4. Intelligent maintenance planning through early prediction of inspection requirements.
Conclusion
Digitalisation allows the highly complex process of waste incineration and the related production of electricity, heat, and steam to proceed in a more continuous way. A combination of a permanent monitoring of the furnace and the automated control of the combustion process helps in doing so. This is based on the integration of data regarding the gross calorific value and the other properties and condition of the waste delivered and to be burned. This data enables a predictive adjustment of the combustion process. This, in turn, ensures a largely trouble-free operation of a waste-fired CHP plant and also allows for its maintenance, because the data regarding wear is collected automatedly this way as well. This relieves the operation and maintenance engineers as they are notified by the early warning system and can launch a highly efficient maintenance strategy. All this results in an increased plant availability, reduced downtimes, a higher throughput, and the desired longer productive operating times.
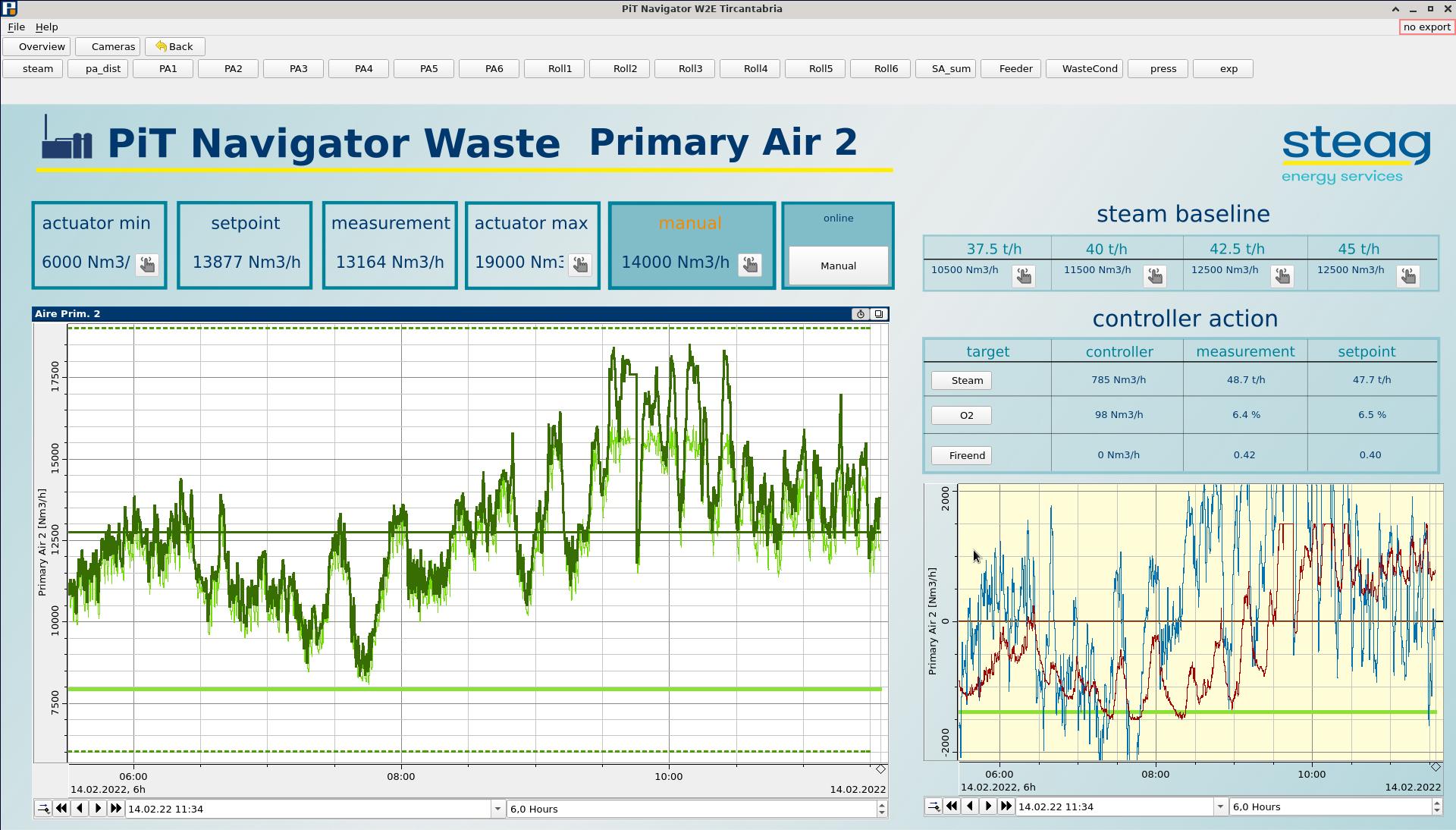
