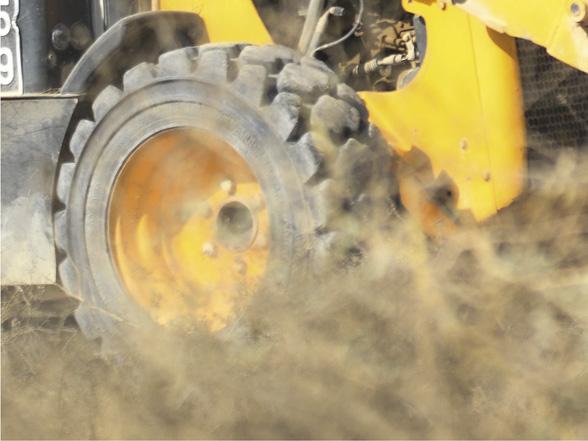
9 minute read
Storytelling for the digital age
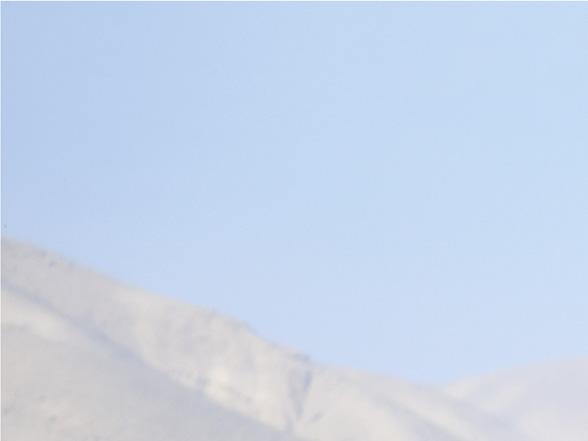
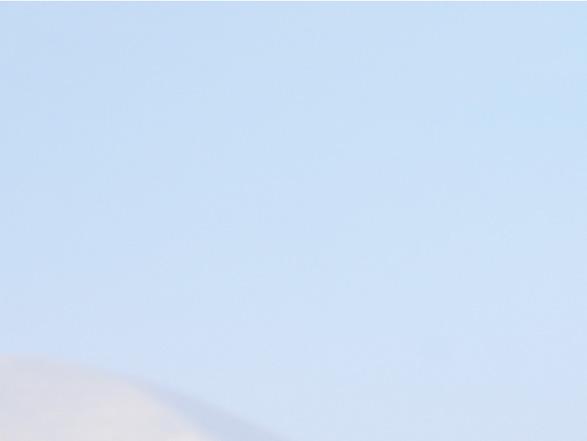
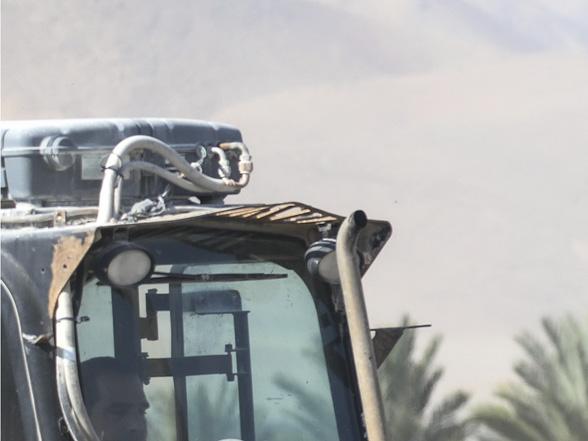
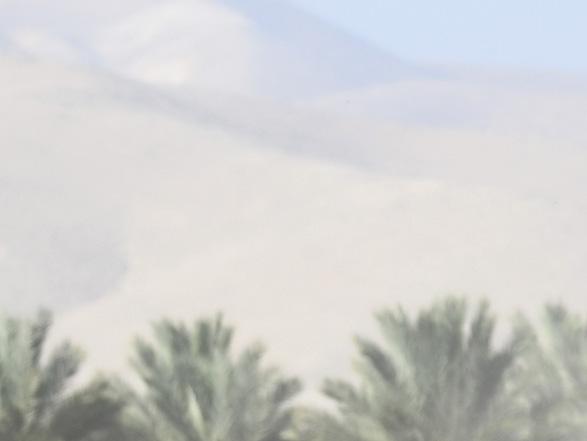
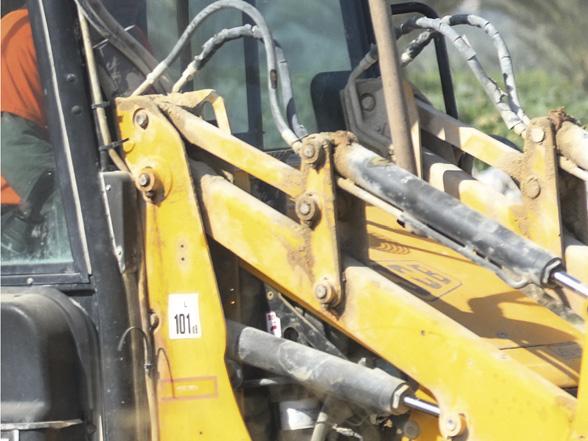
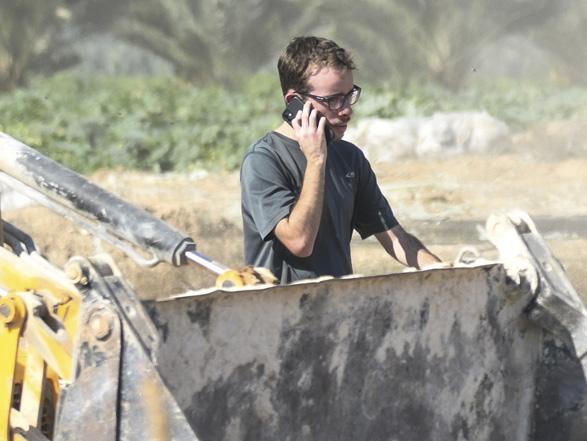
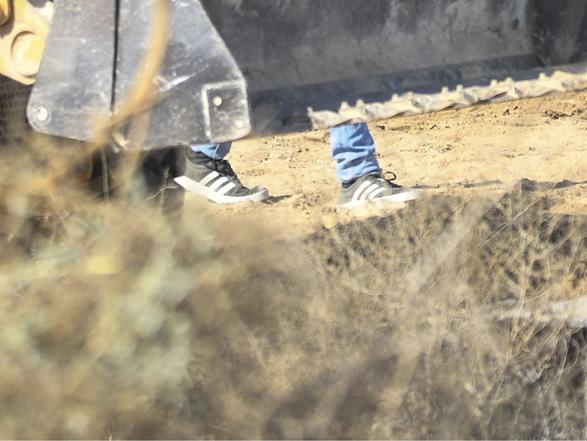
Dr. Eran Inbar and Eitan Elkin, Prisma Photonics, Israel, describe unlocking pipeline digitalisation with next-generation fibre sensing.
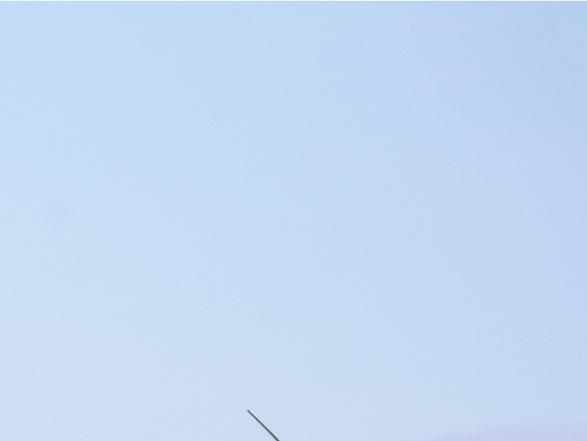
Figure 1. Testing for multiple intrusions in a small area.
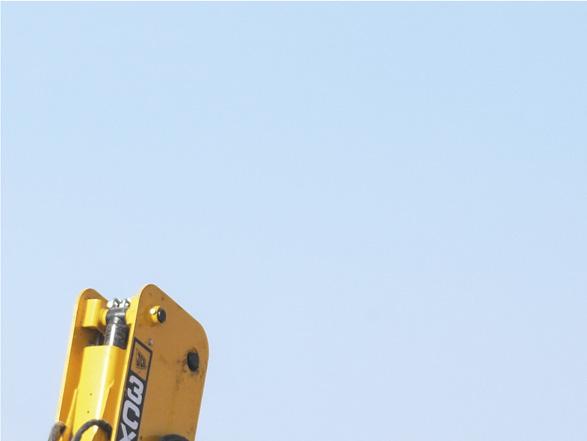

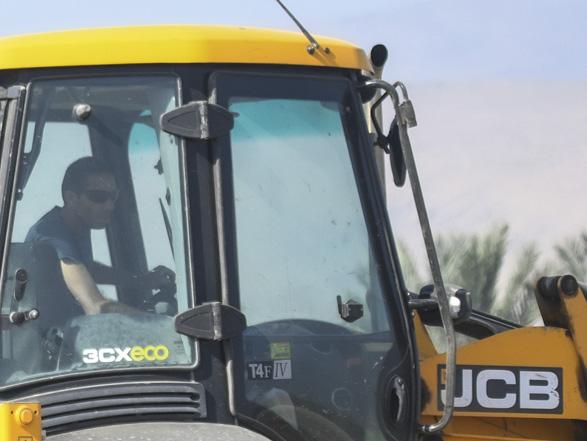
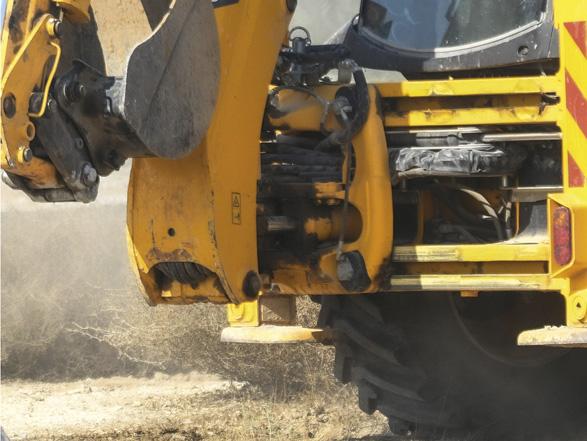
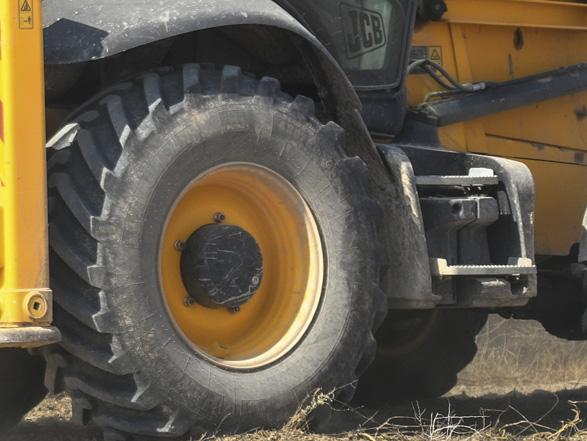
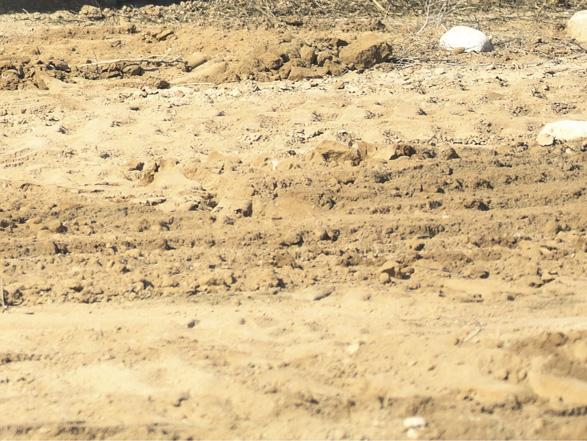
From afar, it looked like a ballet: two digging machines and a single person. The person was trying to sneak his way through, trying to fool the pipeline monitoring system. The system, based on nextgeneration fibre sensing, registered all three of them. Since none of them was an immediate threat to the pipeline buried underneath, it just noted the activity as three possible risks to follow. It continued working, monitoring the entire length of the pipe, leaving the three to continue their intricate drill (while keeping an open eye if they come closer to the pipeline).
This event took place near a buried gas pipeline, testing the capabilities of PrismaFlowTM, a next-generation fibre sensing solution. Using the pre-deployed optical fibre, it detected all activities remotely from the operations centre, tens of kilometers away. The fibre – a regular
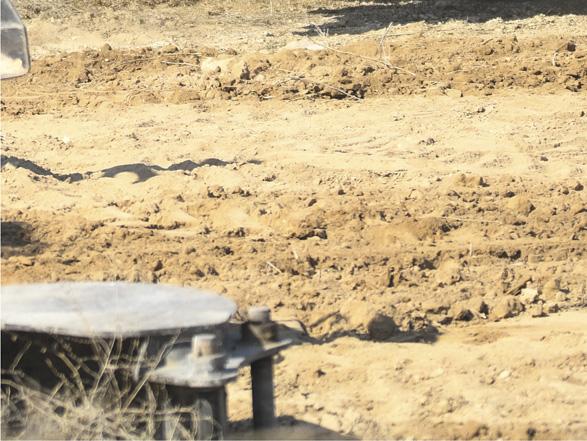
telecom optical fibre – had been buried along with the pipeline a long time ago. Similar fibres have been placed alongside pipeline infrastructure for the last 20 or so years. All it took was hooking up a PrismaFlow sensing unit to an end of the fibre in a valve station, and tens of kilometers of pipeline infrastructure were immediately able to be monitored. Data pipelines and digitalisation Most pipeliners will have a satisfied look on their faces looking at a well-operated pipeline, thinking of the product flowing smoothly inside. Operation managers will have a similar look, thinking of the data that flows smoothly through this same pipeline.
Data and smart use of it are becoming a top priority in today’s oil and gas companies. Whether it is to improve on operations and performance, prevent product loss and theft, or enable emerging commercial models – data and the digitalisation of the pipeline are key. Driven by regulatory pressure or required internally to improve and perform better, oil and gas companies understand the need to gather, correlate and glean information from all the data they can get from the infrastructure they operate.
But not all data is created equally, as not all petroleum products are equal. There is a matter of quality. The data needs to be of high quality and detailed enough to provide actionable insights, information that will guide forward and fit the needs of advanced algorithms and machine learning models. Think of an old 1990s phone camera image compared to the latest smartphone photos. High-resolution data with enough details can open up a new age of pipeline digitalisation.
Digitalisation is a data-centric concept. It’s all about unlocking the power of Big Data, smart sensors, and emerging technologies to enable the intelligent, realtime management of assets and products. Furthermore, digitalisation will also support the proliferation of new business models through increased innovation in the market.
At the source of digitalisation are next-generation sensing technologies that are able to supply machine learning-quality data and details.
Sensorless monitoring, all along the pipeline PrismaFlow is Prisma Photonics’ next-generation fibre sensing solution for pipeline operators. Even for those acquainted with traditional fibre sensing technologies, PrismaFlow opens a new level of data and monitoring abilities. Its Hyper-Scan Fibre SensingTM technology is a far cry from existing fibre sensing.
PrismaFlow uses the pre-deployed optical fibres along pipelines, whether they sit directly on the pipeline or inside a conduit in the pipeline’s vicinity. These fibres can be interrogated optically to sense abnormal events. As the fibres are already there, deployed with most pipelines, fibre sensing offers superior and more cost-effective monitoring abilities than point sensors. From a deployment perspective, the fact that measurements are evenly distributed along the asset means that monitoring is more accurate than using point sensors such as pressure gauges in stations, and acoustic or electric sensors installed directly on the pipe. Think of it as a sensor that is always there, at all places, at all times, and in any condition without any installation hassle.
Most traditional fibre sensing technologies offer limited data granularity, indicating, with some success, pipeline leaks, and third-party intrusion events. PrismaFlow can detect and generate highly detailed data, monitoring assets for hundreds and even thousands of kilometers.
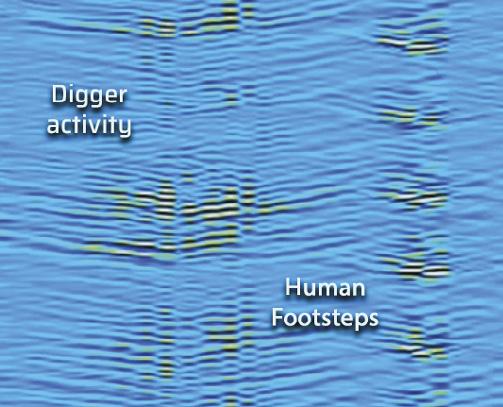
Figure 2. Internal representation detecting human and excavator individually.
Figure 3. Alert showing on PrismaFlow user interface.
Figure 4. Confirming alert information in the field.
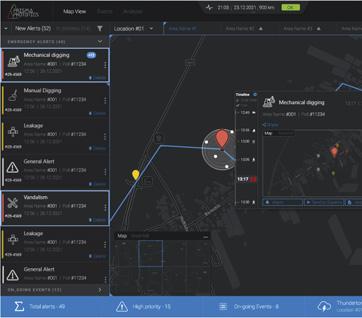
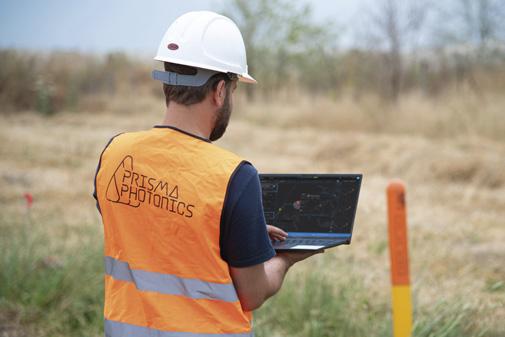
It also employs machine learning models that accurately classify events in context. It features accuracy with nearzero false alarm rate (FAR) for leak detection, third-party intrusion, and distributed integrity check (along the entire length of the pipeline).
The data richness and high signal to noise ratio (SNR) enable PrismaFlow to use storytelling techniques, making sure that only actual events raise the alarm. This is the real value of an asset monitoring system – the ability to alert on true events and avoid false alerts that are a nuisance and lead to a shutdown of such a system.
Weaving data into stories to avoid false alarms Let us get back to the opening scene, where various excavators and people are moving near a buried pipeline. PrismaFlow has detected several sets of activity as depicted in the internal system representation seen in Figure 2. On the right, we can see the footsteps of a walking person, and on the left side, the image shows the engine and digging bucket of an excavator starting to scrape the ground. The activities are not blended into one big blob; the high resolution of the data allows distinction. Using its machine learning-based classification, PrismaFlow understands that these are a person and excavators and starts to build a story.
A story is made out of many steps: an excavator arriving at the area, movement along the asset, the initial scrape on the ground, etc. The alertness of this location is heightened as suspicious activities may occur there. Only when the person or excavator starts digging (consecutive hits to the ground with a shovel or the digging bucket) will an alarm be raised in the operations centre (or any other connected application) with the location and all the needed details – in real-time. Storytelling is the key to a low false alarm rate. It is made possible by highly detailed data, enough for the machine learning models to classify it and identify what happens and where. It could be a farming machine passing by, a train, a busy road – all these will not trigger an alarm as they lack all the steps of an actual intrusion. The humanmachine interface, the UI, will present the real alarms and may (if configured to do so) show the unfolding stories and other events that are taking place but do not require attention yet. These events are displayed and may (or may not) evolve into a real threat to the pipeline integrity and operation.
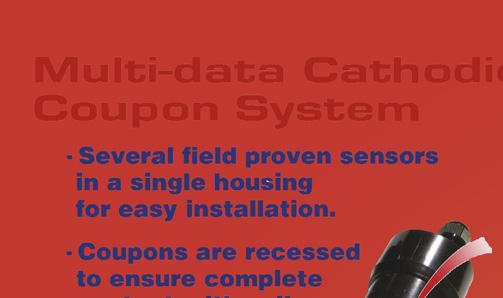
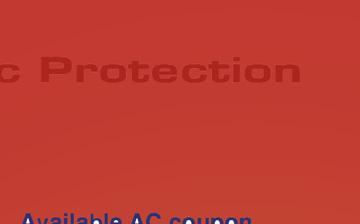
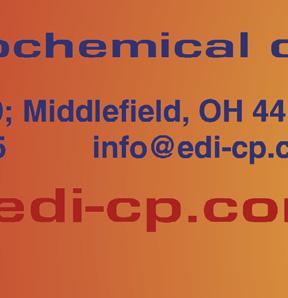
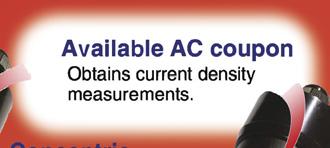
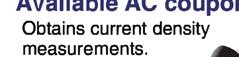
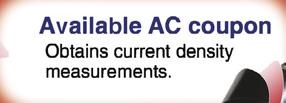
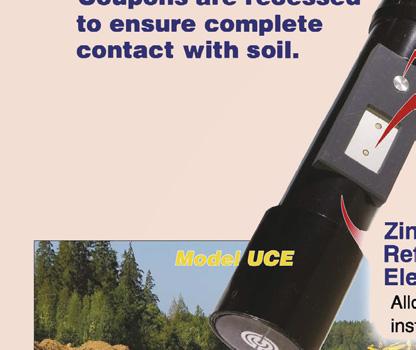
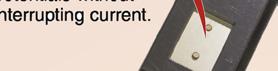

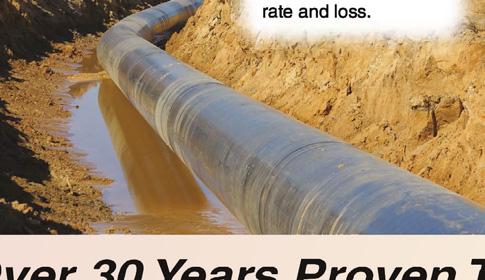

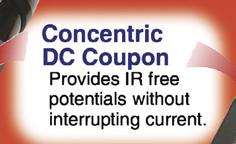
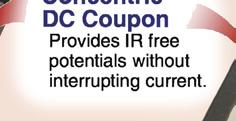
When dealing with product theft, there is always a series of such stories. Even a single person on foot with a drill and a welder can be detected. The classification stages are essential to identify friends or foes. Since pipeline right-of-ways often pass through public areas, there are certain innocent behaviours and/or wildlife activities that must not trigger an alert. A monitoring system that raises an alarm (and a response team) for the movement of a few boars will not be considered reliable.
For a pipeline operator to establish a higher-level view of the assets, classified events and alarms can be integrated and sent further up, feeding an aggregated view and unifying information from several sources. The data from PrismaFlow, generated by Hyper-Scan Fibre Sensing and classified by machine learning, can be merged, using data fusion with input from other monitoring systems, to create a comprehensive view or be kept for further analysis.
Data fusion for leak detection Leak detection is another primary example of pipeline monitoring systems. Such leaks are a nightmare for any oil and gas company.
PrismaFlow has proved outstanding product leak detection abilities. In testing, gas leaks of 200 standard litres per minute (SLPM) were detected in about one minute from the moment the leak started. Liquid leaks as low as 20 litres per minute (LPM) were detected, which is about 0.02% of a change in the flow. The detection was carried out without using a momentary and transient effect such as a negative pressure wave, only Hyper-Scan Fiber Sensing.
Many operators require a dual-solution mechanism. This level of monitoring is often dictated by governmental regulations while also providing the most effective leak detection with complementing systems.
This is where the digitalisation of the pipeline really shines through. The aggregation and correlation of all monitoring data can grant an operator peace of mind that they have a reliable solution in place that will alert on time and only when needed.
Conclusion Digital technology and large amounts of data are not new to the oil and gas industry. The sector has been using seismic survey data and underground modelling for years. However, for the data to be used for better decision making, optimised operations and monitoring, it needs to be detailed and machine learning grade.
Next-generation fibre sensing can offer this level of data, pushing pipeline digitalisation further. PrismaFlow is an example of where detailed data meets real-time machine learning analytics to respond to safety, preventive and predictive maintenance, and safety scenarios.
Pipeline digitalisation will reach every oil and gas company operating in this market. Operators will embrace it, responding to regulations or their own needs to remain competitive. Big Data and machine learning technology, reliable and affordable sensors, and scalable platforms that can process and analyse data: all these define a new digital age for pipeline operators.
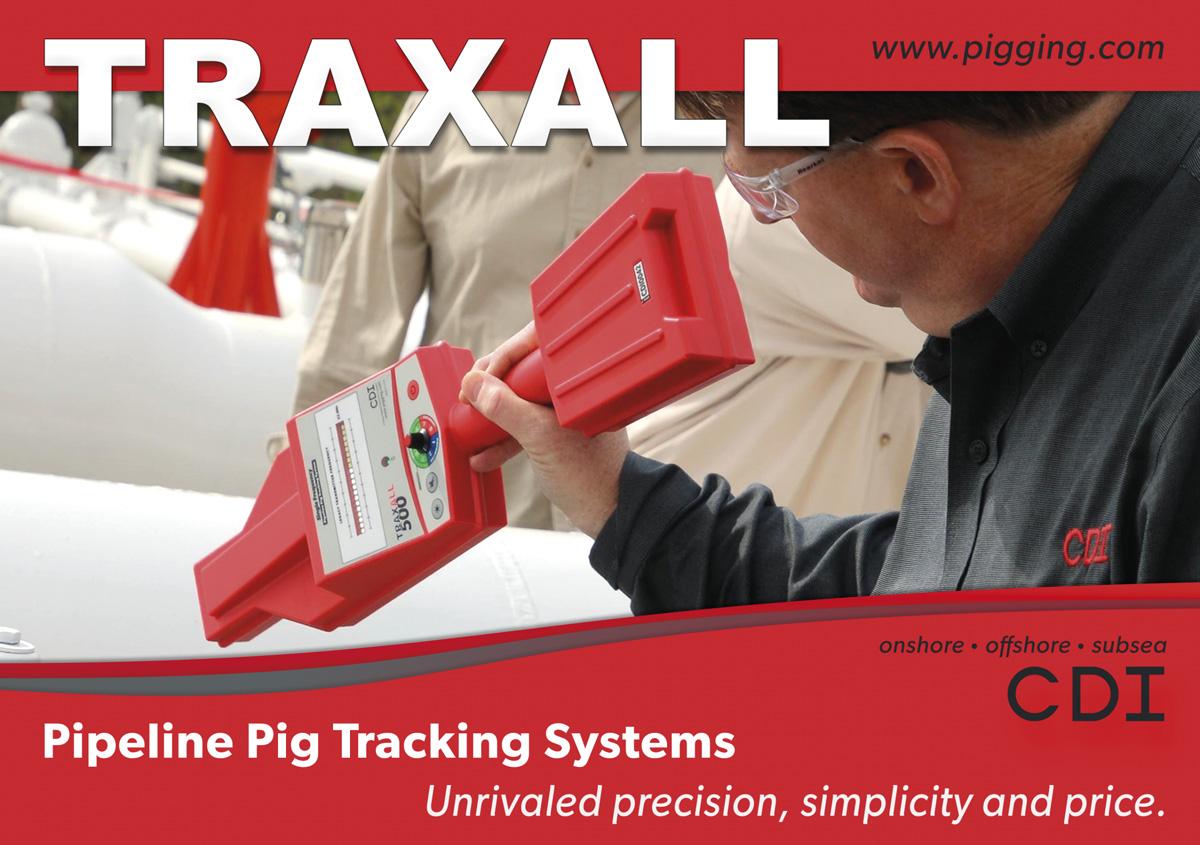