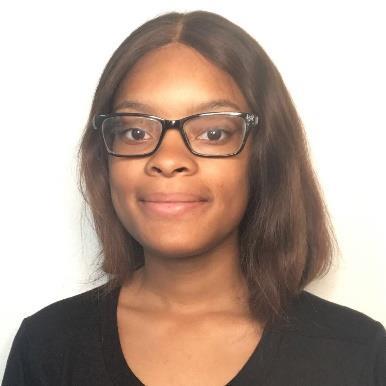
2 minute read
Katerra M. Stamps
from 2021 Research & Innovation Week- Undergraduate Student Research Booklet- Prairie View A&M University
Deep Fake Detection Methods Research
Katerra M. Stamps and Yonghui Wang (Faculty Mentor)
Department of Computer Science, College of Engineering
Prairie View A&M University
Until recently, face swapping technology was a thing of the feature. The rising demand for more advanced face altering technology came with a demand for detection methods as well. The emergence of deep fake videos has significantly added to online disinformation. Deep fake videos are falsified videos created by AI technologies. These falsified videos utilize techniques that can superimpose face images onto a video of a different person in order to create a fabricated video. There are cases as recent as 2019 where the court and judge discovers that evidence submitted was false and generated using deep fake apps. From public figures to celebrities, artificial videos can, when in the wrong hands, spread misinformation and compromise the credibility of the targeted individuals. This research explores various deep fake detection methods that can be used to differentiate deep fake videos from the original, unaltered videos. The objective includes research on deep fake detection methods introduced by various researchers and the testing of those methods. Detection methods vary and can fall into categories of physical/physiological, signal- level, or data-driven. A large portion of detection methods relies on the ability to discern inconsistencies in original media and the fabricated media. From the exploitation of biological signals to the studying of phoneme and viseme, there are numerous techniques that can be used to accurately identify deep fakes. There are detection methods that focus entirely on non-visual components to identify key inconsistencies in videos. Understanding the techniques and terminology is important when it comes to knowing why specific detection methods work with deep fakes created. Detection methods were written with the purpose of analyzing facial features and or facial x-rays as the focal aspect. Researchers use the data received and attempts to distinguish inconsistencies between data received from the original video and the altered video. The results of these methods are not fail proof, however, the ability to identify altered videos has proven efficient. Deep fake detection methods are still being developed to mediate and help curb the spread of disinformation. The impact of the research has been the expansion of coding knowledge and the wide range of methods available to detect deep fakes or altered videos.
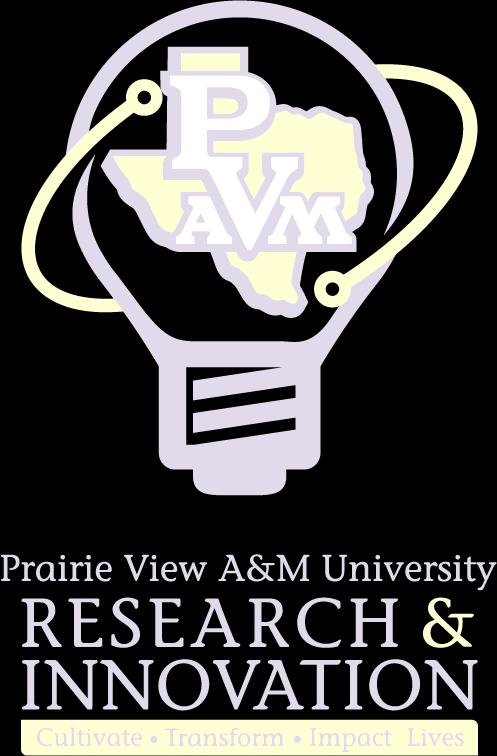
Keywords: Computer Vision, Deep Fake Detection, Face Swapping, Fabricated Media, Deep Learning
Comparison of Artificial Intelligence Approaches to Diagnose Breast Cancer
38 | Page