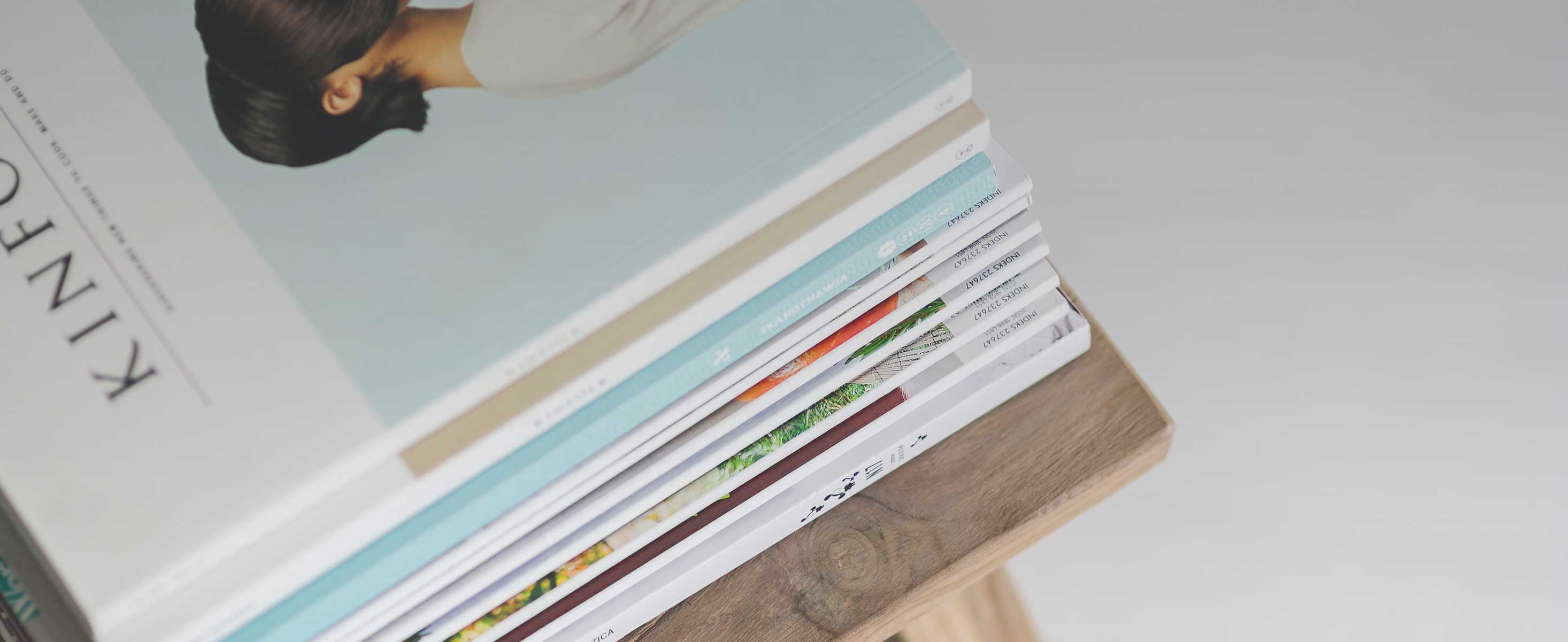
2 minute read
3.4.2 Standing challenges and barriers
from proprietary sources. These options are able to provide a high level of network visibility. Some other practices leverage existing equipment and data sources. Instead of networkwide monitoring, these practices employ a combination of data sampling and network modelling as a cost-effective solution to improve network visibility.
3.4.2 Standing challenges and barriers
While data can play a vital role in improving network visibility, there are several key gaps and challenges in employing network data and modelling the network for different use cases:
• Data quality, consistency and integration: Data quality issues can be caused due to reliance on third‑party data or use of manual or paper‑based processes. Also, inconsistency in the format of data provided by different third parties and delays in data collection and processing can make it difficult to implement use cases that require real‑time information. In addition, trust concerns related to customer and DER level data needs to be considered.
• Data granularity and accuracy: While AMI provides a new level of visibility to distribution system loads, it comes at a cost. Large volumes of AMI data must be effectively recorded, communicated, stored and processed for applications in distribution planning and operations. The volume of AMI is directly proportional to the time granularity at which the data is recorded and stored. While lower time granularities result in less burdensome data management, lower time granularities also provide less detailed view of the network.
• Cybersecurity: Increase in the deployment of data from different sources increases the involvement of third‑party systems, and hence, new capabilities to manage cybersecurity threats are increasingly required. • Location of smart meters: Smart meters are not always located in the areas where visibility is most needed, and their deployment can be sporadic. The sporadic deployment of smart meters obliges DNSPs to rely on cellular communications, instead of mesh communication networks, which can sometimes be unreliable. • Data procurement costs: Cost of procuring smart meter data can prevent implementation of use cases at‑scale. • Network and load modelling: The employed methods for load and generation modelling would have an influence in the accuracy of the hosting capacity calculation. A study by
EPRI [10] shows that modelling loads directly with their AMI active and reactive power recordings is likely to result in lower hosting capacity values. These hosting capacities might be up to 40% lower than hosting capacities obtained with conventional utility load modelling methods.