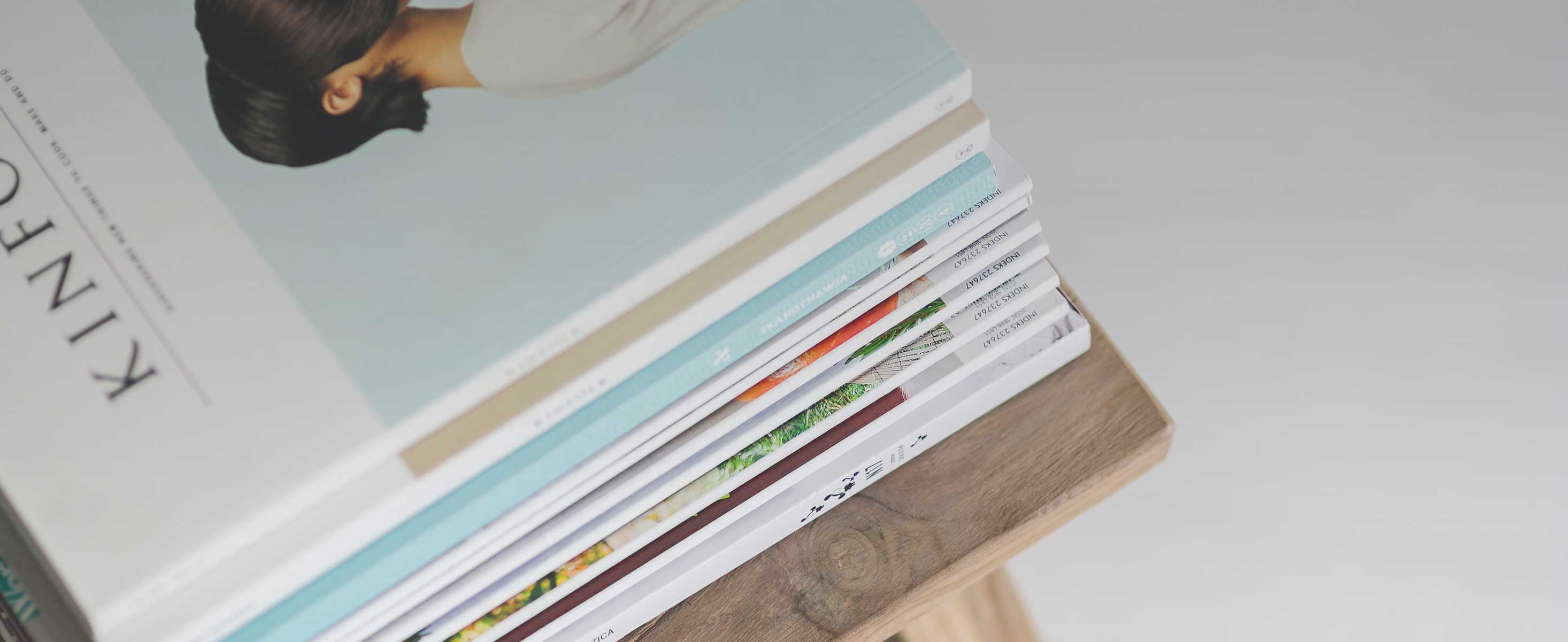
5 minute read
4.2.2 Optimal mix of data and models
factors. The range of applied impact factors can change depending on the considered feeder metrics. Hosting capacity can be assessed for each of these feeder metrics independently to provide visibility into each metric contribution. Most important in applying the results from a hosting capacity study is to access each metric's hosting capacity. The feeder metrics mapped to the impact factors to determine hosting capacity boundary conditions recommended by EPRI [13] are presented in Table A 2-1 in the Appendix.
The accuracy of a hosting capacity calculation increases with the number of impact factors considered. However, consideration of multiple impact factors increases the computational burden and complexity in assessing hosting capacity. Utilizing particular grid and DER assumptions, boundary cases can be identified that calculate the minimum and maximum hosting capacities without needing the execution of repetitive cases. Identifying the lowest (worst-case) and highest (best-case) hosting capacities is essential for assisting with interconnection requests and informing DER installers and project developers. A summary of the impact factors, and their relative ranking as recommended by EPRI [14] is provided in Table 2-1 in Appendix 2.
In Australia, Energeia [15] recommended several impact factors and their related metrics to calculate the hosting capacity as (i) power quality (i.e., over-voltage, under-voltage, flicker, and total harmonic distortion), (ii) reliability (i.e., thermal overload, safety protection, mal-operation, and islanding), (iii) system security (i.e., disturbance ride-through, and under frequency load shedding), and (iv) cost/efficiency (i.e., phase imbalance, and forecasting error). The effect of miscoordination of protection devices on hosting capacity has not been analysed thoroughly. However, based on the reviewed national projects, it noticed that some or all of the following impact factors are used to calculate the hosting capacity such as configuration, voltage regulation, thermal limits, connected load, connected DER, control-managed, DER portfolio, DER location-site specific, and/or time (see Table 4-4-4). In addition, Horizon Power in [16] used more specific impact factors, such as the output fluctuation factor, diversity factor, and other appropriate factors for time intervals, to determine the hosting capacity.
4.2.2 Optimal mix of data and models
To perform hosting capacity across an entire LV distribution network system, a large amount of validated data is required. Missing and/or inaccurate grid data is a significant concern to utilities undertaking hosting capacity analyses. Unlike some conventional planning studies, hosting capacity assessment and the interconnection review process requires a higher degree of grid model accuracy in connectivity information and electrical parameter data. Besides, rectifying data quality and completeness issues can be highly time-consuming and expensive for DNSPs. Capturing the needed datasets for network segments composed of hundreds to thousands of customers, devices, and characteristics that are dynamic in time is not trivial. There is a need to validate existing data and gather new, previously untracked data based on the significant impact factors for a use case. Multiple source systems like Geographic Information Systems (GIS), Customer Information Systems (CIS), Asset Management Systems, and engineering design tools are involved in data collection and gathering processes. A wide range of techniques is employed, including correlating information from disparate repositories, searching paper records, leveraging validation algorithms, and performing extensive field surveys. After gathering and validating data, one of the critical challenges is to convert data to the planning tool format while ensuring consistency and accuracy of data. A certain level of manual support is needed to complete this process. Achieving a grid model with the requisite level of data integrity and completeness is often a multi-year, multi-tensof-million-dollar process.
The lack of visibility of the LV network is a primary concern for most of DNSPs in Australia, as it affects the accuracy of representing the network segments and may lead to over- or underestimate the hosting capacity. For instance, some assumptions are considered to represent the network, such as neglecting the phase imbalance effects. Therefore, an optimal mix between the data and model should be considered to model the behaviour of the network as accurate as possible considering trade-off between accuracy and cost. Interestingly, with nearly 100% smart meter penetration level in Victoria, a supervised (i.e., gradient decent) univariate regression model based on smart-meter driven approach that represents the DER hosting capacity estimation model for the analysed LV network is proposed by [17]. However, the accuracy of the smart meter-driven approach depends on the volume of smart meter data as more data helps to capture the variance of a larger sample of network conditions (i.e., voltage vs active power). This approach is not suitable for planning studies that involve testing various network solutions that change the fundamentals of the feeder. Therefore, it is recommended to build explicit models (i.e., model-based approach) for the components, which consider phase imbalance, to be able to apply “what-if” scenarios and avoid over or under investment due to inaccurate quantification of hosting capacity.
The model-based approach contains explicit electrical models of the corresponding components in the distribution network (i.e., bus, branch, loads, generators, DERs, shunts, etc.), including the network topology and parameters. The explicit electrical models should be characterized to allow running power flow simulations using a software package for different demand/generation scenarios, or “what if” scenarios. This approach is the most used modelling approach by DNSPs in Australia and worldwide for designing, planning, and improving the distribution network. However, this approach is limited for the HV networks and critical demand/generation scenarios. The smart meter-driven approach could be a promising alternative to model-based approaches that can save both time and effort in assessing hosting capacity [17]. Unlike a model-based approach, the smart meter-driven approach does not require explicit electrical models, i.e., does not require power flow simulations, rather it uses only historical smart meter data. Here, the advantages and disadvantages of network representation approaches can be summarized as shown Table 4-1.
Table 4-1. Advantages and disadvantages of LV modelling approaches
Model Advantages
Model-Based
Approach • It is adaptable, network elements and participants can be added, removed or changed. • Control techniques can be implemented and tested through simulations. • It is typically limited to HV feeders and to critical demand/generation scenarios. • Model simplifications arising from lack of critical visibility or network information can lead to grossly incorrect estimates of hosting capacity.
Disadvantages
Smart Meter-
Driven Approach • It does not require explicit electrical models, i.e., does not • Impractical for networks without high penetration of smart meters