
11 minute read
Predicting the Butterfly Effect
from DialOGue 2019
Professor Peter Haynes OG 1976 is Professor of Applied Mathematics in the Department of Applied Mathematics & Theoretical Physics, University of Cambridge. He was Head of the Department from 2005-2015. He has been a Fellow of Queens’ College since 1986 and was Director of Studies in Mathematics there for many years. In April 2019 he was made a Fellow of the Royal Society, an accolade given to eminent scientists in recognition of outstanding contributions to the field in which they work.
Here he outlines his journey from studying maths at the RGS to becoming a Professor at Queens’ College Cambridge and his theory of applied mathematics in predicting climate change.
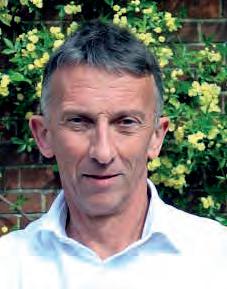
Professor Peter Haynes BA. MA. OhD. FRA
Starting at the RGS
As must be the case for many RGS students who go on to study mathematics at university I liked the subject in part because I was good at it and in part from the satisfaction of learning new material and then applying it to solve problems, particularly problems that seemed hard, or even impossible, at first sight. The RGS, through the excellent teaching of Alan Thorn and others, and through the companionship of and competition with my fellow students in the enthusiastic double mathematics class, gave me a good start in my mathematical life.
University mathematics
As a university student, one’s view of the subject changes. In some areas (pure) there was an ongoing sequence of introducing a new mathematical structure and establishing its properties and then building on that to generate new structures, and so on. In other areas (applied maths) there is interplay between considering recognisable ‘real-world’ problems (planetary motion, spread of disease, propagation of radio waves), but operating in an abstract mathematical world where the original ‘real world’ problem is temporarily put aside. One of the important aspects of the development of mathematics is that areas that might at some time have been regarded as ‘pure’ may eventually be used in ‘applied’. Therefore, it is very important not to draw too rigid a boundary between the two branches. Indeed, some of the most interesting areas of mathematics are those which are evolving from the domain of pure mathematicians into the domain of applied mathematics. Some of this evolution is enabled by increase in computational power. Most applied mathematics requires calculation and there are some mathematical structures within which calculation has become possible only in the last 50 years, or indeed only in the last 10 years.
Personal route into climate science
In early 1980s Cambridge, one of the subjects on offer to a research student in applied mathematics was geophysical fluid dynamics; the study of flows with physical ingredients that are relevant to the atmosphere or ocean. One approach might be to study a simple system in the laboratory. However I embarked on a more mathematical approach – essentially to reduce a physical problem to a minimal (but interesting) mathematical model, explore its properties and then on that basis draw conclusions about the physical system. This not only gave the opportunity for interesting mathematics, but also offered a fascinating context to that. My interest was reinforced by a summer spent at Woods Hole Oceanographic Institution, Cape Cod, where I and students in other sciences, as well as mathematics, were given a range of lectures on oceanography, completed project work (mine was on acoustic propagation in the ocean) and enjoyed many other adventures one might expect of students spending a summer together in a new place. (During the summer I went on an oceanographic cruise for a week which gave me the grounding insight that I was probably better doing calculations than trying to operate complicated measuring equipment).
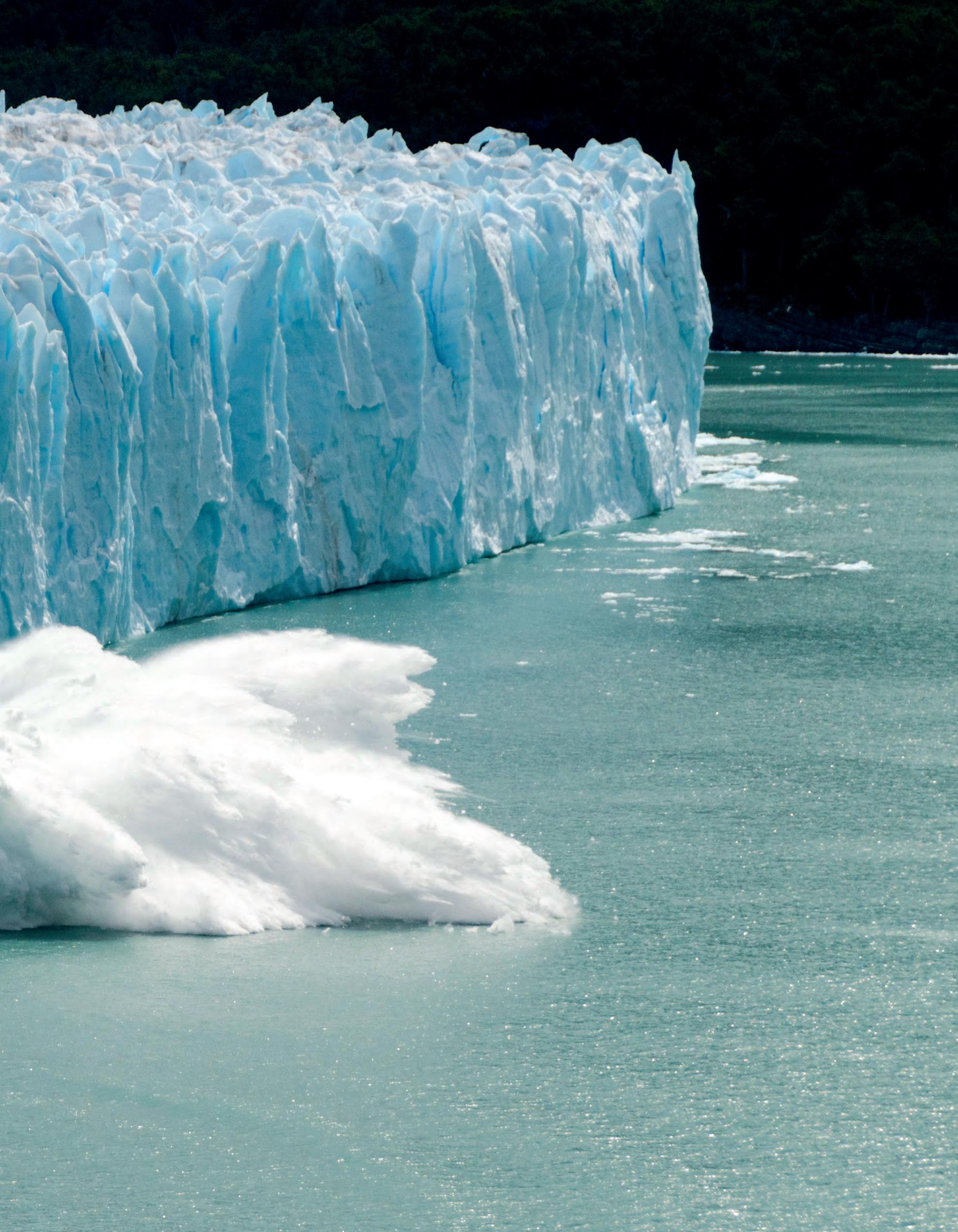
After finishing my PhD in Cambridge I spent two years at the University of Washington in Seattle, in the Department of Atmospheric Sciences. This gave me valuable exposure to the science of the real atmosphere, as distinct from the very idealised mathematical and laboratory models of the atmosphere that were studied in great detail in Cambridge. One of the big events in atmospheric science during this period was the discovery of the Antarctic ozone hole. I remember Dr Susan Solomon, a young scientist who two or three years later led observational campaigns to the Antarctic (and then to the Arctic to try to understand whether an ozone hole was likely there too), announcing to a fairly stunned audience, that some British Antarctic Survey scientists had published a paper in Nature reporting unexpected and very large decreases in ozone, and that no one had much idea how to explain this. The story of the ozone hole, including the international agreements to limit the emission of the chemicals that lead to ozone destruction is now well known. (Very recently Nature celebrated its 150th anniversary and chose the discovery of the ozone hole as one of ten highlighted papers from their archives.)
Following my time in Seattle I returned to Cambridge, where I have spent the rest of my career (so far), teaching and carrying out research, usually with some ingredient of mathematics, into different parts of the climate system.
Mathematics in climate science
There is a long tradition of the role of mathematics in the physical sciences. Phenomena such as the orbits of planets and ocean tides have been studied by deriving and solving equations which represent physical laws and then interpreting the solutions. This is not simply ‘mathematics in the service of physical science’ – often the development of completely new areas of mathematics has been stimulated by this process. An important part of the development of climate science has been to exploit this approach, applying it to the study of atmospheric radiation, to the behaviour of weather systems, to the flow of air over mountains, to the coupling of atmosphere and ocean in the tropics that leads to phenomena such as El Nino, and so on. The arrival of computers has meant the size and complexity of the systems that can be studied in this way has increased enormously. The importance of weather prediction has meant that over the last 70 years, climate science has been one of the scientific areas leading the way in the application of increasingly powerful computers, to the solution and exploitation of the equations of physics. Some of this application has in itself required new mathematics.
Alongside this traditional role for mathematics in climate science, other roles are becoming increasingly important. One, the mathematics of uncertainty, arises from the probabilistic nature of weather and climate. Most readers will have heard of the ‘butterfly effect’ – the idea that a butterfly flapping its wings in Brazil creates a hurricane arriving in Florida. This is a wider example of the phenomenon that in many physical systems, the evolution over some period of time is very sensitive to small changes in the initial configuration of the system. It follows that an accurate forecast can be made only over a limited time and on longer times the behaviour of the system appears to be random. (The term chaotic is sometimes used.)
In the context of weather forecasting, this aspect of the behaviour requires a distinction between deterministic forecasting and probabilistic forecasting. The question of ‘will it rain at 10am on the day after tomorrow?’ can reasonably be considered a problem in deterministic forecasting. Whether or not a useful forecast can be made will depend on the amount of data available on the present state of the atmosphere and on the accuracy of the model that is used to convert that information into a forecast. If the quality of the forecast is not very good there is the realistic possibility that it can be improved in future through improvements in the data and the model.
However, it seems very unlikely that the data and the models can ever be improved to the extent required for the same approach to be applied to answering the question ‘will it rain at 10am four weeks from today?’ But the apparently random behaviour can be acknowledged in a probabilistic forecasting approach to answer the question of ‘what is the probability that it will rain four weeks from today’. As the time over which a forecast applies increases it must become more probabilistic. Climate is the probability distribution of weather and a forecast of climate change is a forecast that the probability distribution of weather will change.
This introduces some important subtleties. First, how can we tell, from our experience of weather events, if climate is changing? It does not make any logical sense to deduce that climate change is happening on the basis of a single weather event: a month of flooding in Northwest England, a super-typhoon hitting Japan during the Rugby World Cup. These weather events might have taken place (as one of the many outcomes described by the probability distribution) in an unchanged climate. Of course, if these events were more usual over, say a decade or two, relative to 100 years ago, then one might start to conclude that the probability distribution, i.e. the climate had changed. But what can one say from an individual event? What is now possible is to discuss, and indeed estimate, whether the probability of this type of event has changed relative to the pre-industrial climate. This requires the use of models that simulate first the pre-industrial climate and second the current climate, in which greenhouse gases have increased substantially over their pre-industrial values. Each of these simulations generates a ‘probability distribution’ for the relevant climate. Then the actual probability of the observed weather event according to each of these distributions can be estimated. Using this kind of technique, it has been estimated that under the present climate compared to the pre-industrial climate, the 2003 heatwave in continental Europe, for example, was twice as likely, and the flooding in NW England and SW Scotland in late 2015 was 60% more likely.
A second subtlety is how to take account of the probabilistic nature of climate in adaptation to climate change. Design of flood defences will take account of the estimated ‘return period’ for floods of different magnitudes. A climate model prediction for an increased probability of flooding at a particular level, i.e. a shorter return period, will (in affluent and well-organised societies) be taken into account in the design of new defences. But consider the example of a dice that is loaded against throwing sixes. If the dice is thrown a ‘few’ times, say ten, then there may be several sixes. If the dice is thrown ‘many’ times, say a thousand, then the proportion of sixes will almost certainly less than 1/6. Correspondingly, if there was increased probability of flooding in a future climate then over ‘many’ years more flooding would be very likely. But over a ‘few’ years there might be drought.
The key question is how many years is ‘many’ (and how many is ‘few’). Recent climate model studies suggest that in some geographical regions (the western half of the US), even twenty or thirty years may be ‘few’. Now it might be that this is an artificial property of certain climate models and determining whether or not this is the case is a very tricky problem. But if the real climate system behaves in the same way, then the implication is that for some (not all) geographical regions, whether or not the next 30 years are significantly drier than previously or significantly wetter than previously is something that cannot be predicted – each possibility is simply a possible random outcome. This makes planning difficult – in such regions does one invest in new flood defences, or new water storage and distributions systems?
There are many other areas at the interface of mathematics and climate change that are currently being investigated. One is the need for radically new algorithms for computation and modelling. To a large extent the same algorithms are being used now as when computational approaches to weather and climate prediction began about 70 years ago. The massive increase in computational power over that time has been exploited by clever adjustments to algorithms rather than radical change. But future increases in computational power are likely to have a very different form, and radically different algorithms will be needed to exploit it. Another is the potential use of machine learning and related techniques. If a computer can learn (or taught to learn) the rules and strategy of chess and then apply that learning to beat the best players in the world, then why should it not learn to interpret the rapidly increasing amount of data on the climate system and make forecasts?
There are many reasons why this might be difficult, but past experience suggests that investigating questions like these will give much valuable insight, even if the question itself is never answered.