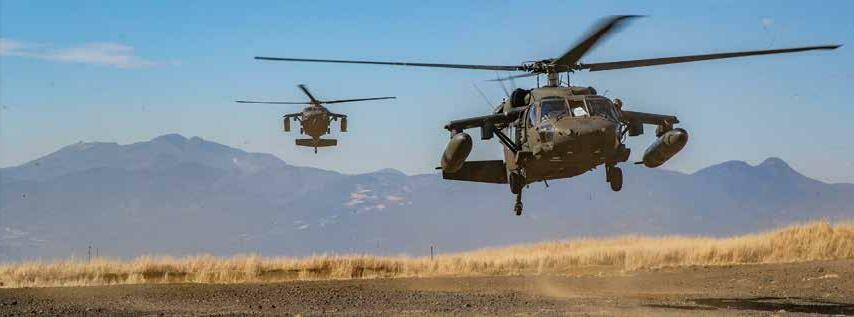
4 minute read
Intelligent Edge Sensors – Ultra Performance in Constrained Environments
Intelligent Edge Sensors
Ultra Performance in Constrained Environments
By Author Rodney Feldman, VP Business Development and Marketing, SECO USA
Deployed sensors enable situational awareness and early warning detection of emerging battlefield threats. Ideally, a wide variety of sensors communicate and correlate data to automate and accelerate the detection of changing conditions. In reality, this is limited by several factors, including the traditional size, weight, power, and cost (SWaP-C) as well as associated limitations on intercommunication and processing capability. Current advances in processing technology along with artificial intelligence, computer vision, and machine learning are changing the dynamic – enabling intelligent sensors at the edge.
Making Sensors Intelligent
Spanning a wide array of technologies, sensors include cameras (whether electro-optical, infrared, or hyperspectral), sound or vibration detectors, radar, environmental (such as gas, temperature, humidity, and pressure), positional (GPS, accelerometer, gyroscope, magnetometer), and more. Each type of sensor generates specific data formats, which differ in frequency, amount, and structure. To be useful, data must be analyzed and the results communicated or automatically made actionable.
In the non-military world, we’re very familiar with the artificial intelligence (AI) model where data collected at the edge is transmitted via a network to a data center where highperformance computing infrastructure with AI capability processes it and returns a result. This paradigm minimizes intelligence required at the edge, but requires network connectivity, incurs added latency to get a result, and limits the amount of data that can practically be handled and correlated against other data. There is also the risk of interception of communications.
For military and intelligence operations, edge autonomy is often preferred. With a computing platform capable of local high-performance processing, the problems of the cloud computing model are eliminated. Larger data sets can be processed, multiple data sets can be correlated, and faster results can be utilized that enhance operational performance.
Current advances in microprocessor technology, artificial intelligence, machine learning, computer vision, and algorithm development make it increasingly viable to implement
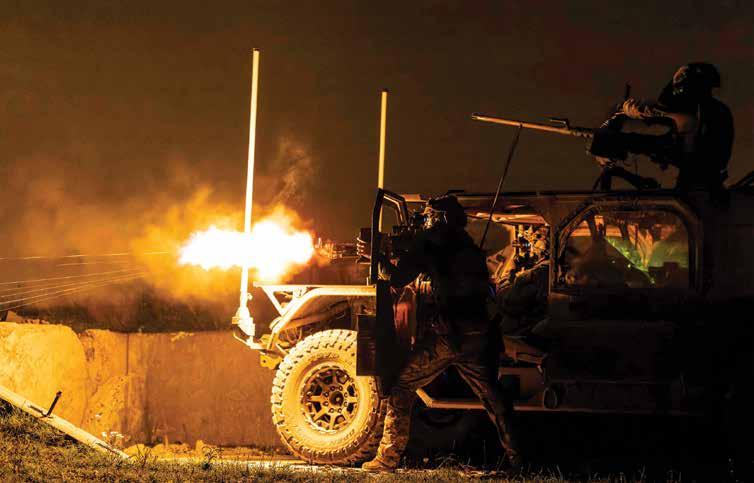
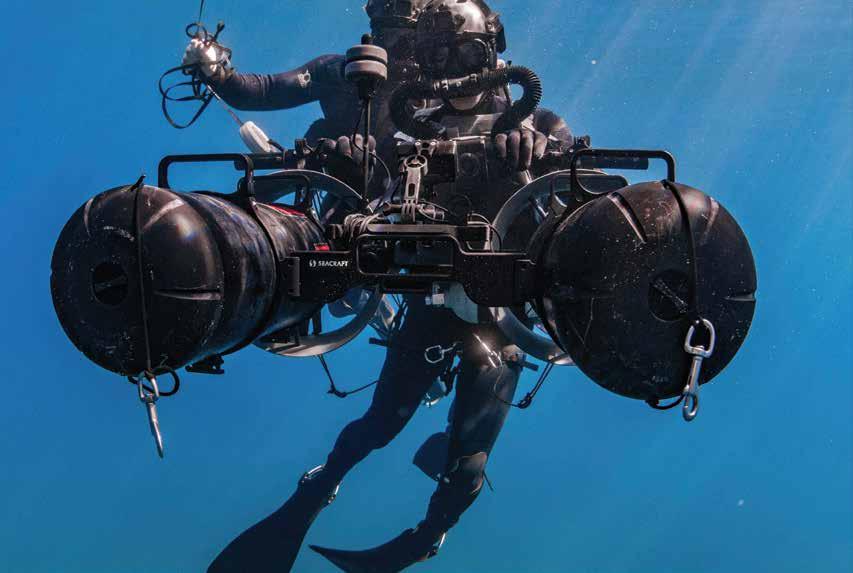
intelligent sensors at the edge with lower power, smaller packages, and for a lower cost.
Processor Platforms That Enable Intelligence
Emerging microprocessor platforms enable increasingly power-efficient instruction sets in conventional processing cores, and additionally provide hardware accelerators for artificial intelligence, computer vision, and machine language operations – along with associated software libraries that enable easy access by algorithm developers.
For example, Intel’s 11th generation Xeon W-1100E series (formerly Tiger Lake-H) and 11th generation Core 1100 series (formerly Tiger Lake Up3) offer high-performance processors with industrial temperature and long-term availability. Featuring DDR4, PCIe 4.0, USB4, and more, these processors enable very high bandwidth interfaces. Integrated graphic cores can be used for display rendering as well as general-purpose number crunching. Intel Deep Learning Boost provides hardware acceleration for training and inferencing along with Vector Neural Network Instructions (VNNI) - usable for on-the-fly learning from sensor data. Intel offers a distribution of OpenVINO toolkit (Open Visual Inference and Neural network Optimization) that enables efficient development of machine learning and deep learning applications. Tiger Lake-H Xeon processors offer 4, 6, and 8-core options with thermal design power (TDP) ranging between 12W and 45W. Tiger Lake Up3 Core processors offer 2 and 4-core options with thermal design power (TDP) ranging from 12W, 15W, and 28W.
For even higher performance in a similar power envelope, Intel’s 12th generation Intel Alder Lake P series introduces Performancecore (P-cores) that provide high-performance processing and Efficiency-cores (E-cores) for managing multitasking – for optimal processor core loading that leads to power efficiency. Added graphics execution units and further implementation of Intel Deep Learning Boost, VNNI, and OpenVINO to enhance AI capabilities. Core counts range from 5 to 15 with 15W, 28W, and 45W TDP options.
ARM-based processors are also emerging that are useful for intelligent edge sensors. For example, NXP’s i.MX 8M Plus microprocessor provides up to four Cortex-A53 cores and a neural processing unit for machine learning applications. While it has far less PCIe and USB interface capability than the above Intel processors, its two MIPI-CSI streaming cameras and various serial interfaces, along with integrated image signal processors, make it useful for less extensive, and lowerpower, intelligent sensor applications.
Some FPGA devices, such as Xilinx’s Zynq UltraScale+ family, incorporate a multicore ARM processor with a programmable logic fabric that enables very powerful sensor applications. With large numbers of flexible interfaces, a wide variety of sensors, from very high bandwidth to relatively low speeds, can easily be incorporated, analyzed, and synchronized utilizing programmable logic and traditional processor code.
Intelligent Algorithms Perform the Heavy Lifting
The performance and power advances of leading-edge processors provide a hardware platform that can interface with and crunch data from a multitude of sensors. It is the advanced algorithms that these processors are capable of executing which enable the intelligence in edge sensors.
The need for real-time intelligent algo-rithms is practically endless. Applications include aerial detection, classification, and tracking of in-flight vehicles and weapons, ground surveillance of people and vehicles, identification of munitions fire sources and explosions, and countless others.