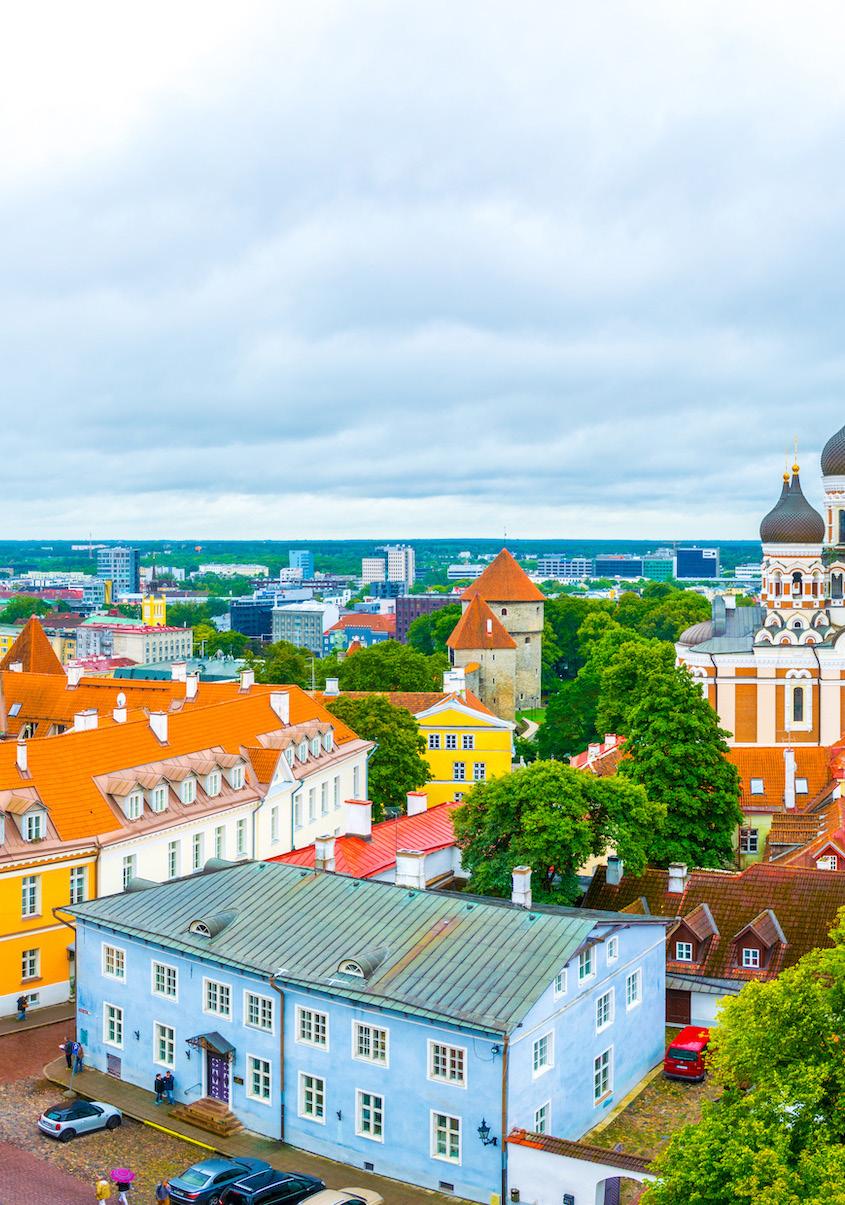
3 minute read
SMART CITIES NEED AI & MUST CONSIDER THE RISKS OF AI BIAS
BY MIKE BARLOW
Our son began playing ice hockey when he was 9 years old. As hockey parents, we learned quickly that rinks are freezing cold and games are incredibly fierce. We also learned that rules and referees are absolutely essential for safety—and that they actually make the games better.
Like ice hockey and other violent sports, our society functions more smoothly when there are rules to follow and institutions to enforce the rules. But wait, what does this have to do with smart cities? Give me a moment to explain.
Smart Cities are giant data science laboratories. They collect, ingest, and analyze data. Smart cities use the results of their data-crunching efforts to optimize services. Today, there is simply too much data, and it’s coming in too fast for traditional analysis methods. We’re not talking about old-fashioned data that’s all neatly arranged in rows and columns. We’re talking about big data.
And when you’ve got big data, you need automated tools and techniques that can sift through huge sets of unstructured data from multiple sources. You need advanced capabilities such as machine learning, deep learning, neural networks, natural language processing, image recognition, and machine vision. These advanced capabilities are the components—the building blocks—of artificial intelligence solutions.
The simple truth is that you cannot manage the 21st century without some form of artificial intelligence. But AI comes with risks. AI magnifies and distorts our biases.
AI is not inherently fair or neutral. Why? Because AI is trained on historical data sets, which reflect the prejudices and inequities of our society.
If you’re a municipal official, you need to know the weaknesses and vulnerabilities of AI – before you meet with vendor sales reps whose job is to sell you an AI solution. And when you do meet with an AI solution provider, here’s my advice:
• Ask a lot of questions.
• Don’t sign agreements that do not include regular monitoring for bias.
• Make sure the vendor commits to a regular schedule of bias audits. Once you’ve got an artificial intelligence solution in place, set up your own bias bounty competitions to encourage local engagement in the process of uncovering AI bias. Gamifying or crowdsourcing bias detection are also effective tactics. Remember, there aren’t just one or two kinds of bias—there are dozens and dozens of biases (e.g., confirmation bias, hindsight bias, availability bias, selection bias, outlier bias, survivorship bias, and many others). Bias is ubiquitous, which means you have to continually monitor results. If you begin to see indications of unfairness or prejudice in your results, that’s a signal that your AI solution is biased.
The Clock Is Ticking
AI is in its infancy, but the clock is ticking.
The good news is that plenty of people in the AI community have been thinking, talking, and writing about AI ethics. Examples of organizations providing insight and resources on ethical uses of artificial intelligence and machine learning include the Center for Applied Artificial Intelligence at the University of Chicago Booth School of Business, LA Tech4Good, The AI Hub at McSilver, AI4ALL, and the Algorithmic Justice League.
The White House’s Office of Science & Technology Policy recently published the Blueprint for an AI Bill of Rights. The blueprint is an unenforceable document. But it includes five refreshingly blunt principles that, if implemented, would greatly reduce the dangers posed by unregulated AI solutions. Here are the five basic principles:
1. You should be protected from unsafe or ineffective systems.
2. You should not face discrimination by algorithms, and systems should be used and designed in an equitable way.
3. You should be protected from abusive data practices via built-in protections, and you should have agency over how data about you is used.
4. You should know that an automated system is being used and understand how
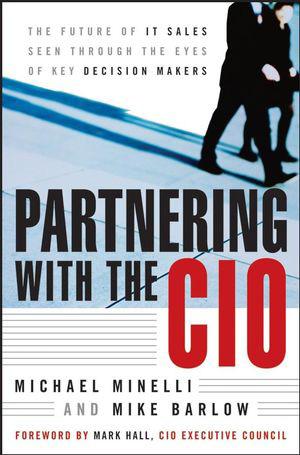
Tallinn, Estonia and why it contributes to outcomes that impact you.
Tallinn, the capital of Estonia, is a smart city that retains elements of its medieval past.
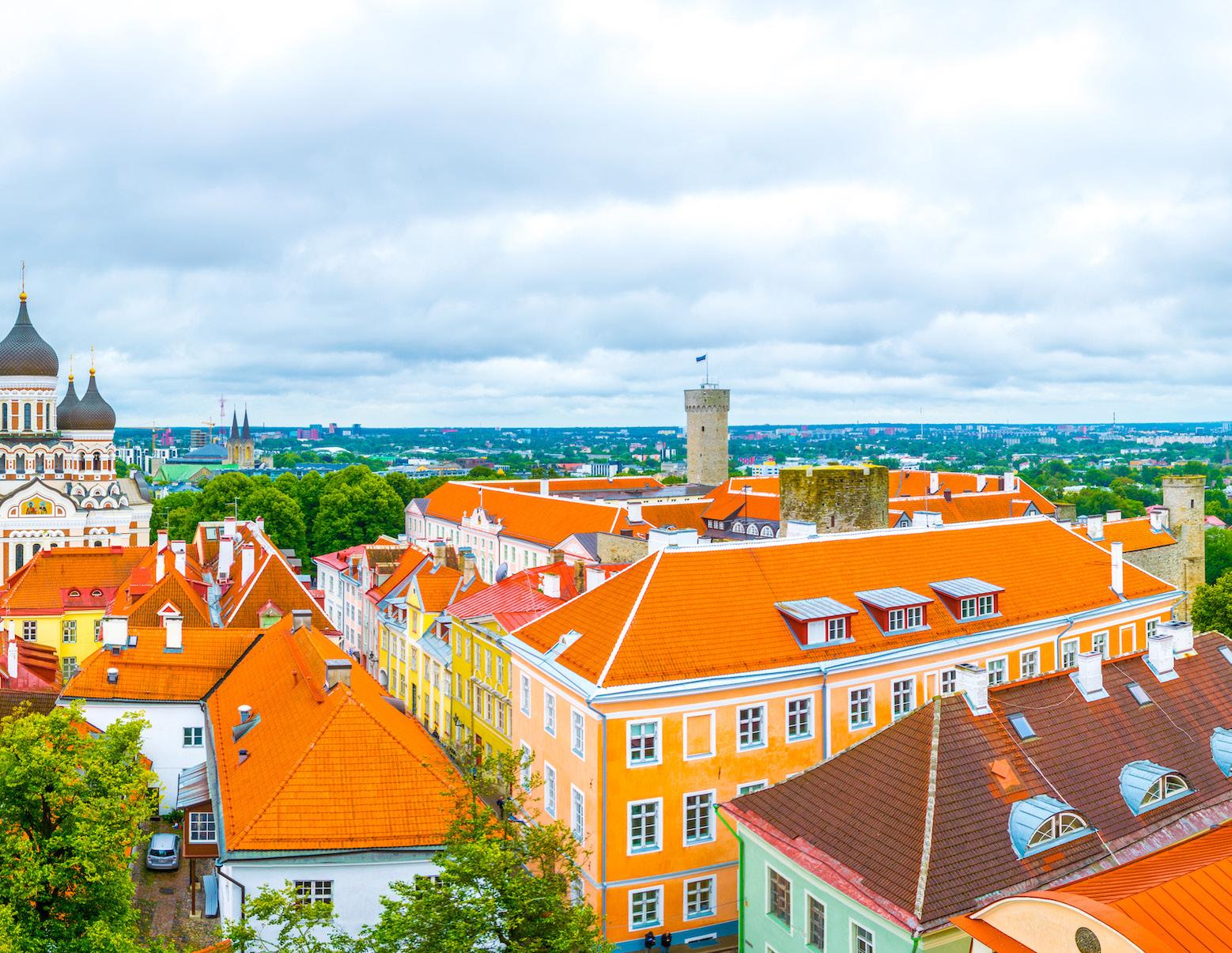
5. You should be able to opt out, where appropriate, and have access to a person who can quickly consider and remedy problems you encounter.
Shifting the Responsibility Back to People
It’s important to note that each of the five principles addresses outcomes rather than processes. Focusing on outcomes instead of processes is critical since it fundamentally shifts the burden of responsibility from the AI solution to the people operating it.
Why does it matter who—or what—is responsible? It matters because we already have methods, techniques, and strategies for encouraging and enforcing responsibility in human beings. Teaching responsibility and passing it from one generation to the next is a standard feature of civilization. We don’t know how to do that for machines. At least not yet.
An era of fully autonomous artificial intelligence is on the horizon. Would granting AIs full autonomy make them responsible for their decisions? If so, whose ethics will guide their decision-making processes? Who will watch the watchmen?
For smart cities that will rely on AI solutions to make better decisions for their citizens, those questions deserve answers.
Mike Barlow is an award-winning journalist, prolific writer, and editorial consultant. He is the author of Learning to Love Data Science (O’Reilly, 2015) and coauthor of Smart Cities, Smart Future (Wiley, 2019), The Executive’s Guide to Enterprise Social Media Strategy (Wiley, 2011), and Partnering with the CIO (Wiley, 2007). His feature stories appeared regularly in The Los Angeles Times, Chicago Tribune, Miami Herald, Newsday, and other major U.S. dailies.

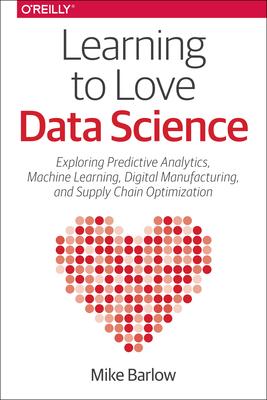
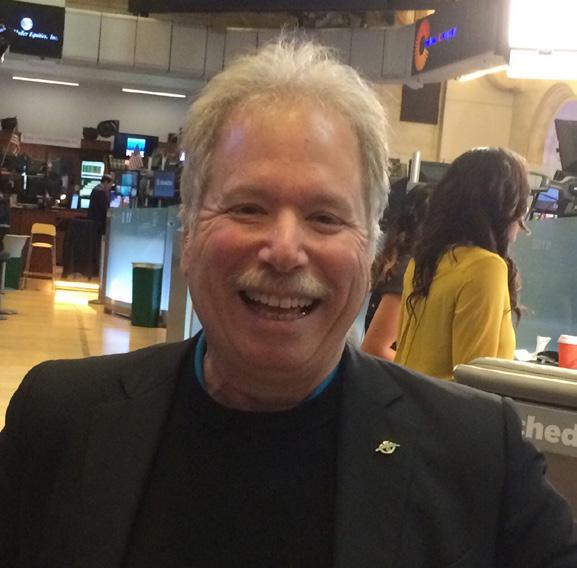