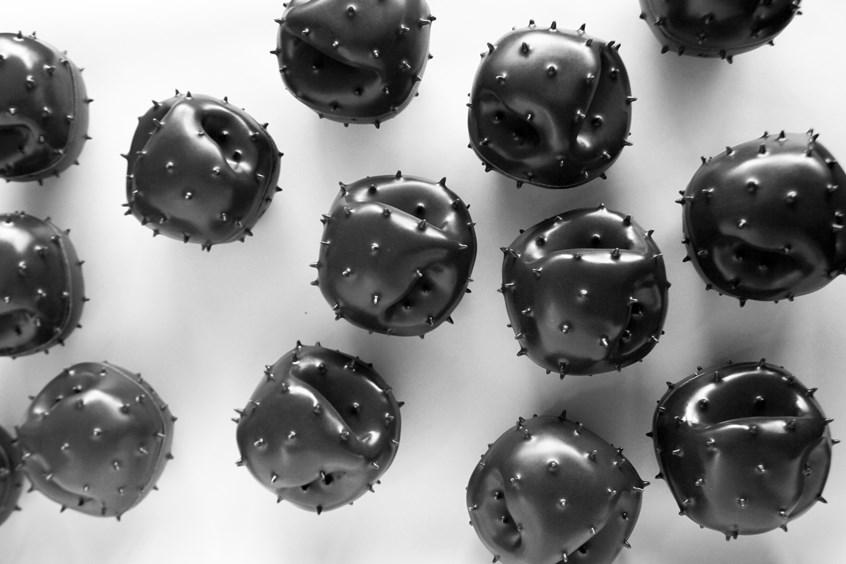
2 minute read
Space Parsing and Data Types
from Orthopolis
by SCI-Arc
When it came to formulating our intervention, we needed a lense through which to see the space we inhabit or wish to inhabit. This is particularly important within a critical framework, as data often inhabits our space and impacts the way we experience it, without an ability to see it. Further, even if we could share the data visually, people's ability to see an interpolation of the layers of data impacting their way of life still isn't necessarily layered in a meaningful way for them.
However, this system has to digest a variety of data types. Among many other examples, the first example below demonstrates the localized focus of gridded and discretized pieces of data that inevitably impact density and the air we breathe, while the second example highlights the interconnectedness and long reaching sinues of commuter traffic. Both must operate to inform the work at hand.
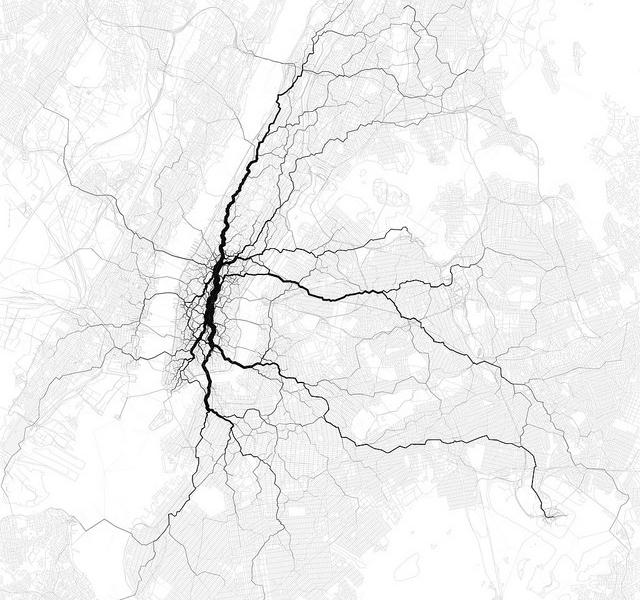
Our primary data factors include an aggregation based on localized demand and an erosion of existing and intervention building stock that is undervalued. Additional systems include a point grid of core services and elevators that contain utility services, a park and recreation data field that attempts to identify portions of the city than can be turned to nature or parkland, as well as concentrated sources of entertainment like stadiums.
As we formulated a data field of collected data sets to form three dimensional urban ether that determines the weight with which the next units are aggregated to the urban intervention, it became apparent
Housing pressure controls density of aggreagation Universal Public space: roads, plazas, places connect aggreggation
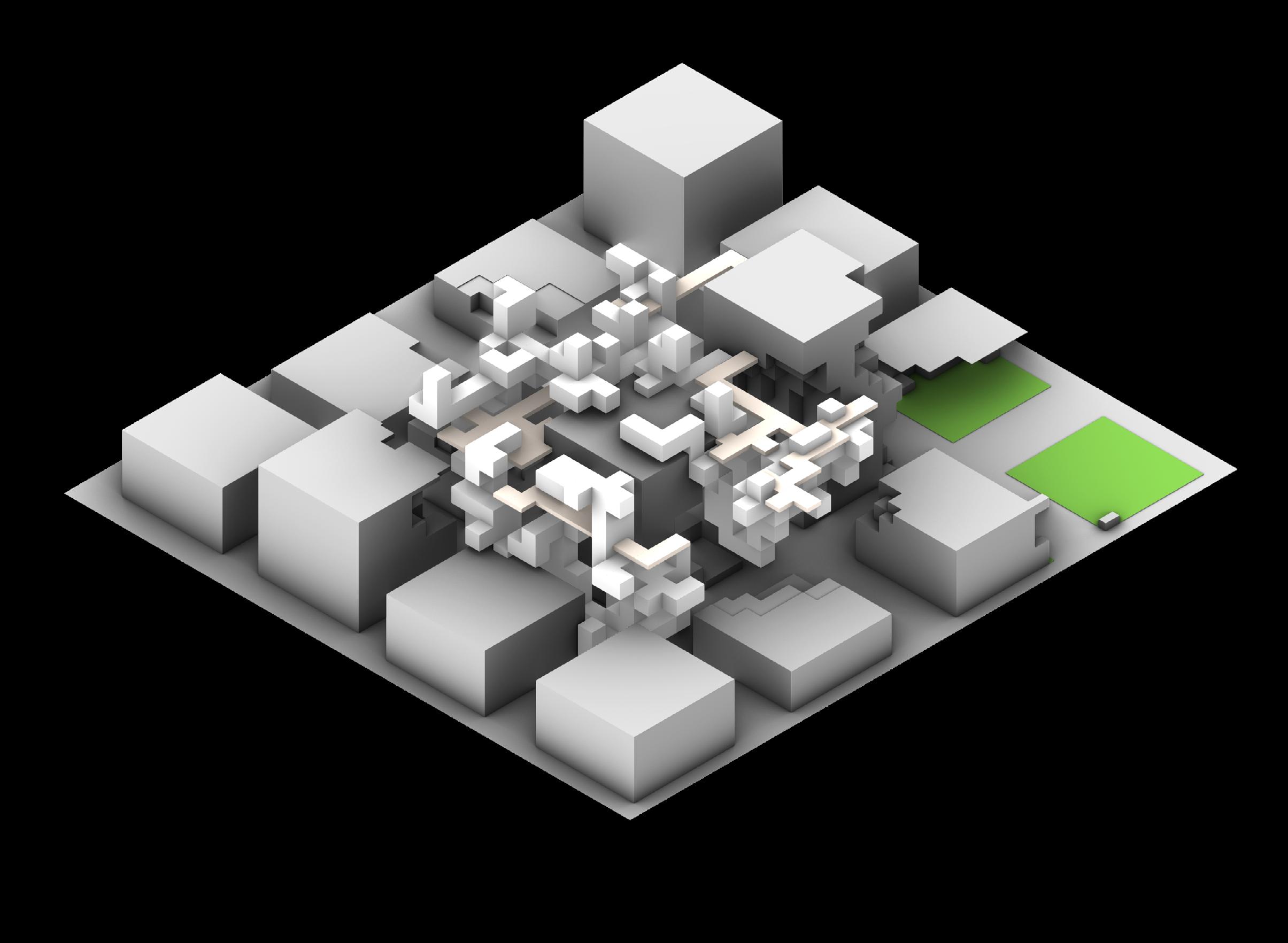
Under-utilized buildings dissolve
Park Space used as low density infill
that this data set needed an underlying connecting force in order to keep the intervention from being sporadic, and thus, inefficient. Here we took some lessons from Diffuse Limited Aggregation, which is essentially just an algorithm of applied Chemistry. Here instead of particles spawning randomly, they spawn based on a set of weighted data. So each possible cell has a particular probability of being picked that is attached and is dictated by a layering of as many datasets as the designer feels is necessary to remedy the health of the urban ecosystem.

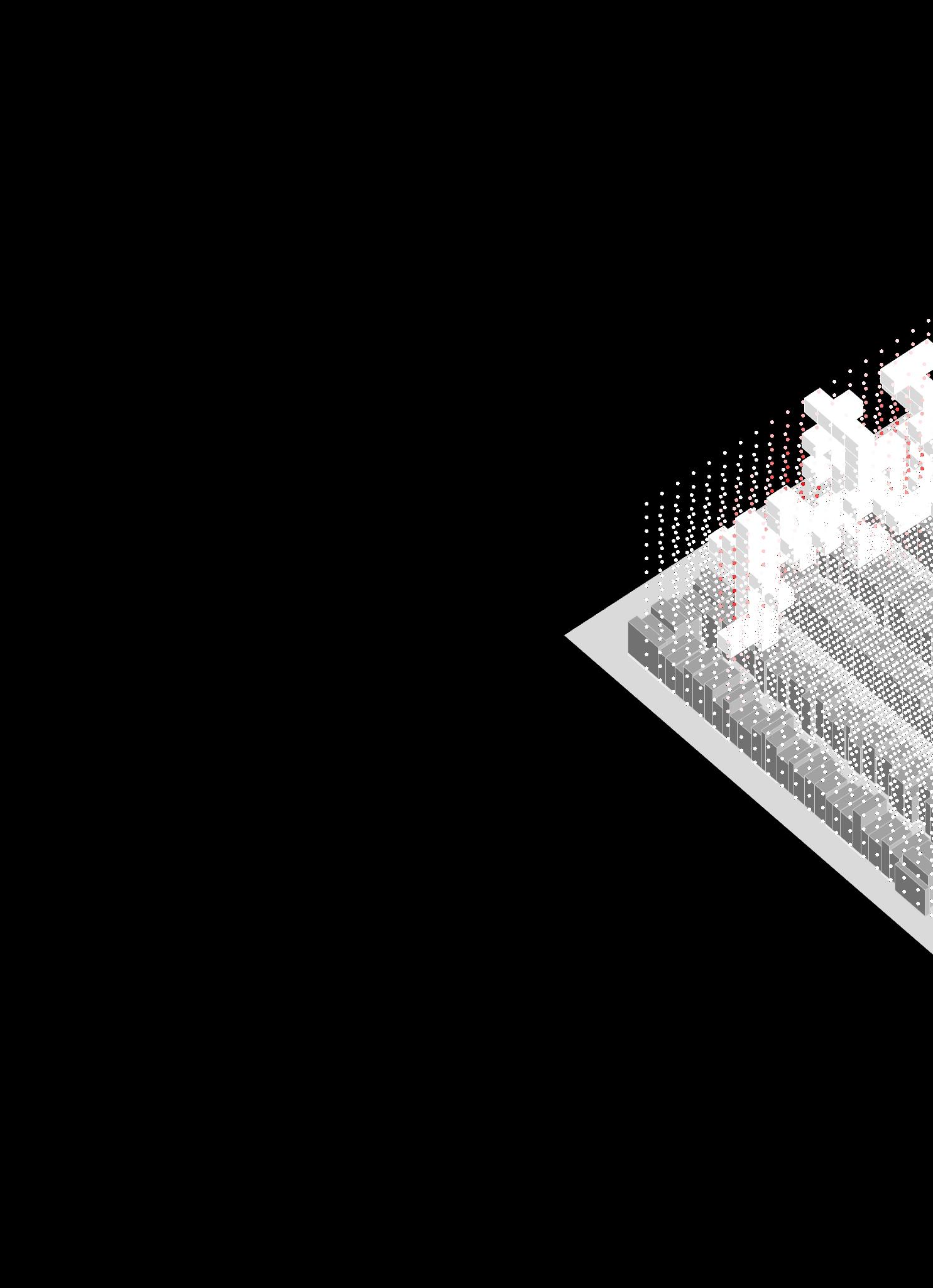
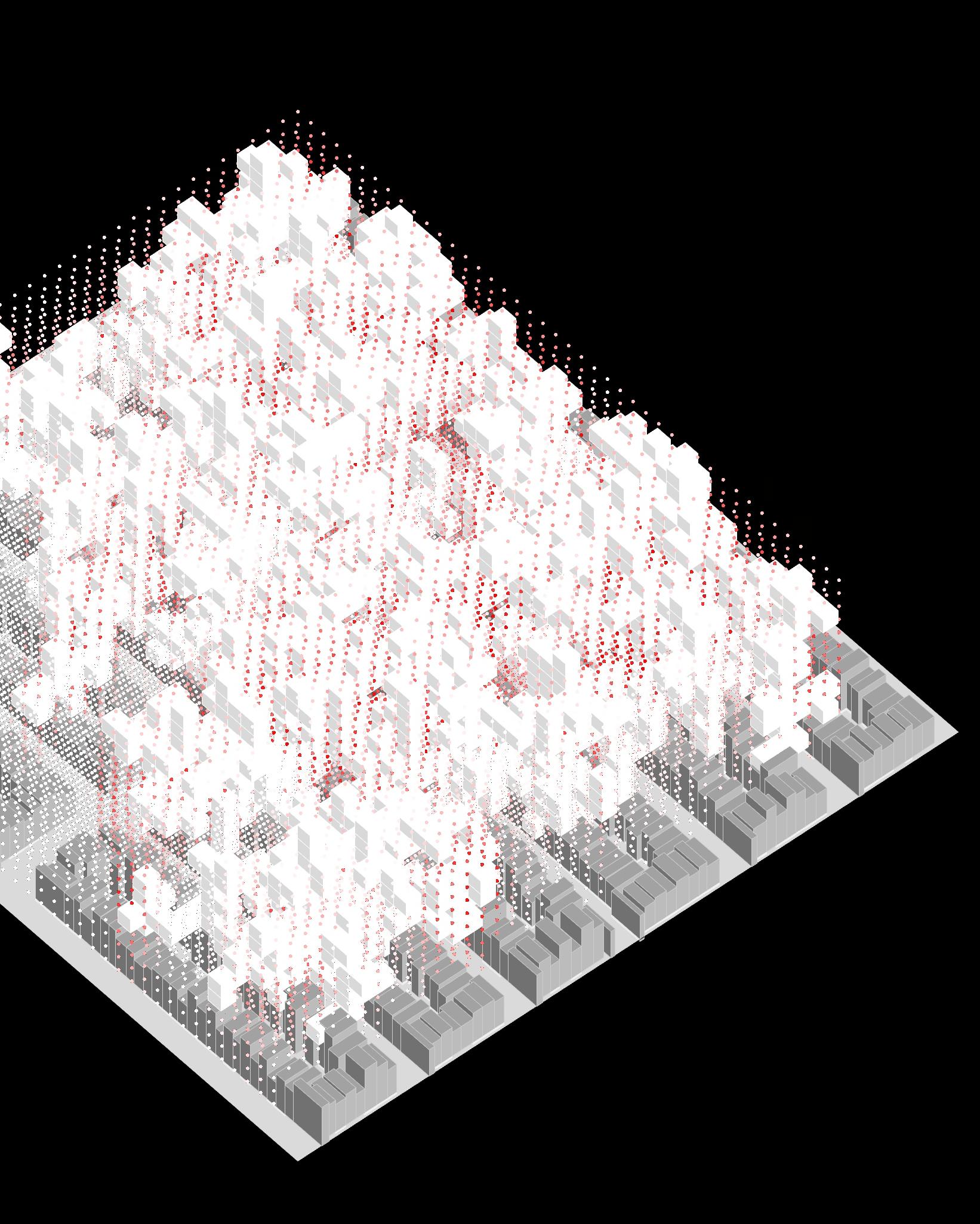
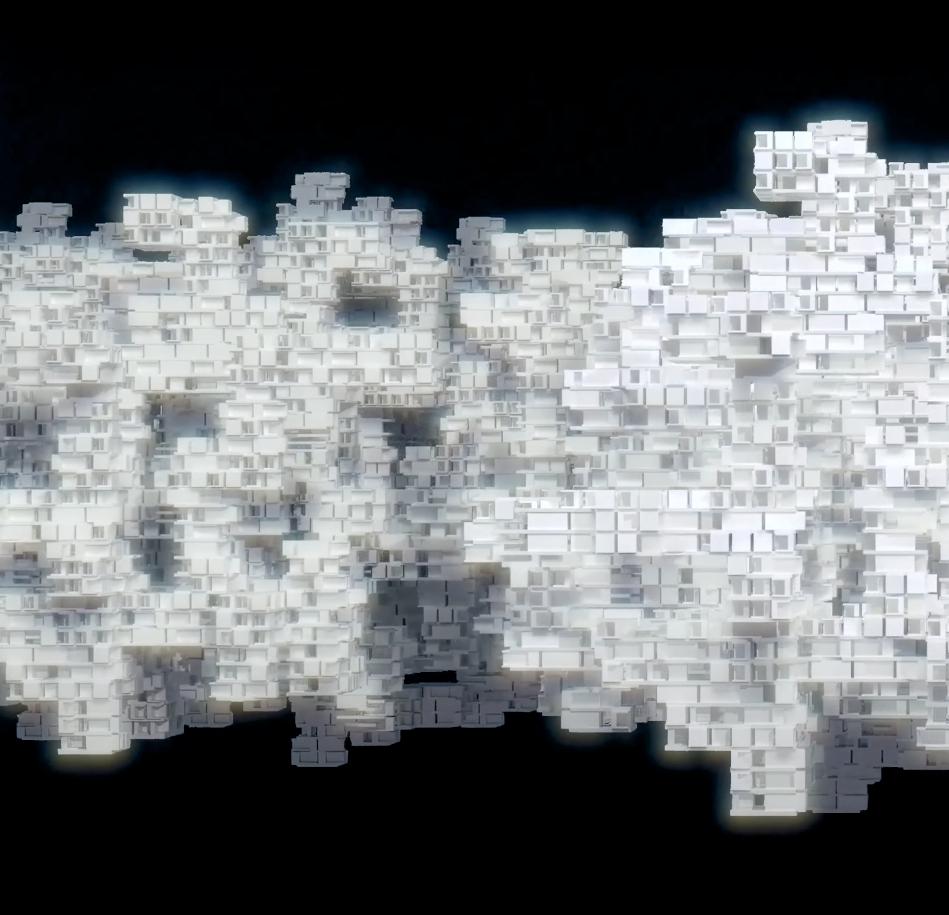
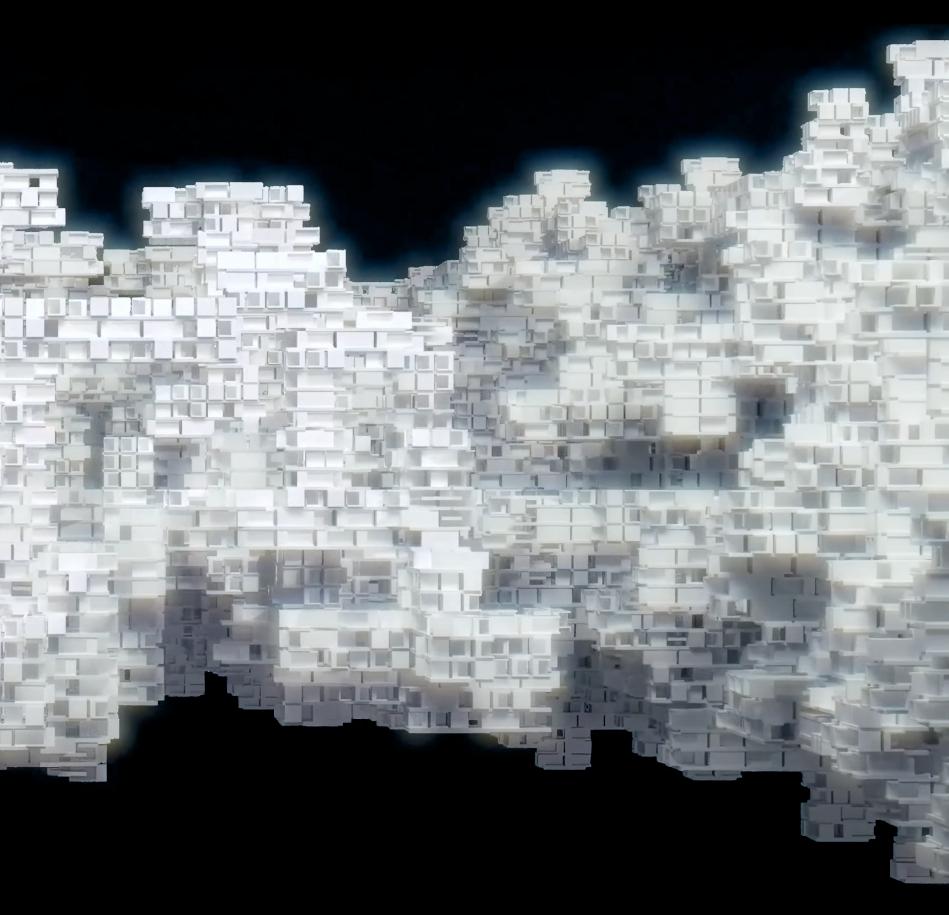