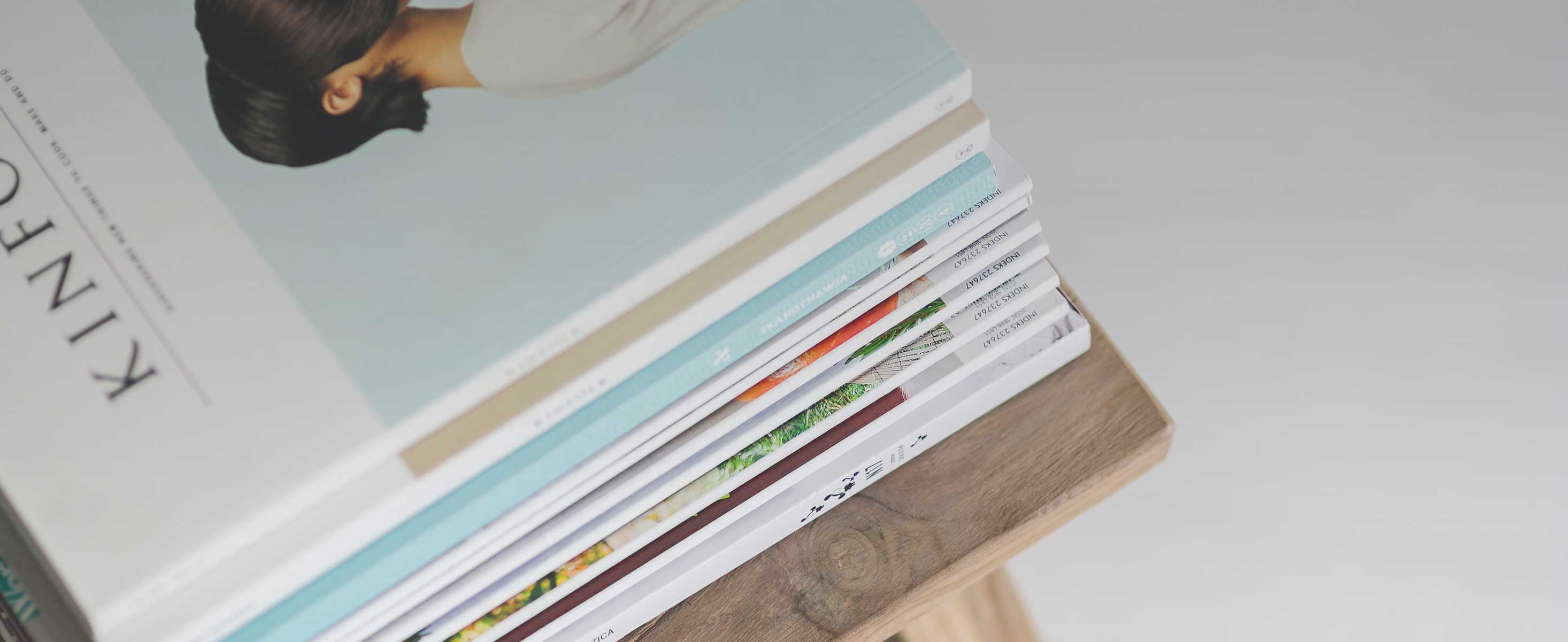
4 minute read
Can AI Really Resolve the Disruption Challenges in the Retail Industry?
How AI is Disrupting the Retail Industry Disruption
EU KWANG CHIN
Advertisement
Fellow, SCS IT Director, DFI Retail Group
CHERIE TEO
Product Line Analyst, Data Lake & Analytics, DFI Retail Group The world has changed since the first COVID case was reported in 2019. All businesses including the fast-moving consumer goods (FMCG) industry, despite being an essential business, are hugely impacted. Notably, some key challenges were also amplified – volatile stock levels resulting from panic buying compromise the accuracy of stock forecasts while border restrictions and lockdown measures disrupt production and timeliness of shipments. Question is, how can we leverage technology to overcome these challenges in a quick and effective manner?
Machine learning and Artificial Intelligence (AI) hold the answer to this question – use machine learning and AI algorithms to transform big data into smart data and infer from patterns to learn and adjust. This continuous and robust learning makes use of data to generate prescriptive actions that can be executed operationally while sustaining efficient and effective supply chain and store operations.
FROM BUILDING TRUST TO BUILDING PRODUCTIVITY
In the context of the retail industry, earning customer trust through consistent delivery of great value and service is quintessential. Often, this means going back to the fundamentals of placing products customers want at the right place, right time and right price. However, existing systems and operational processes present multiple ordering and replenishment challenges that result in out-of-stock situations and untimely replenishments. These frustrate customers who made an effort to go to the stores (both online and offline) but cannot find the product they want – a pain point.
In a recent pilot implementation, DFI deployed machine learning capabilities to minimise out-of-stock situations without building excessive stockpile. Up-to-date and precise stock information is fed into the machine learning engine and aligned with existing operation rhythm before machine learning algorithms are used to predict optimal order quantity for each store. The projections are then sent to suppliers with delivery lead time built-in to ensure that stores receive the right order quantities on the right day, and in a sustainable manner. Significantly, the adjusting of existing operational tempo to eliminate manual processes and streamline operational processes also led to increased productivity.
FROM IMPROVING EFFICIENCY TO IMPROVING SALES PERFORMANCE
As proof of concept, the pilot implementation adopted a scientific approach to compare results between a test product group and a control product group. The products in both groups were identified on the basis that reliable performance comparison can be made between the current ordering process and the machine learning-based ordering process. Additionally, the machine learning ordering system went through four weeks of learning based on actual business data prior to comparison.
In the ensuing two weeks after learning, superior sales performances and reduction in shrinkage (write-offs) were observed in the machine learning ordering system. And even during a special event day (Halloween), the system was able to adjust automatically in response to increased demand – leading to higher sales performance.
This superior performance comes on the back of higher efficiency with less reliance on human interventions, as key data inputs are fed into the machine learning ordering system daily and each store’s optimal order quantity is sent to suppliers automatically. Several manual steps are eliminated.
Furthermore, in just four weeks of learning, the machine learning ordering system is already outperforming the existing ordering process. As the machine learning prediction becomes more accurate over time, the performance is expected to improve further.
FROM SHARPENING BUSINESS ADVANTAGE TO SHAPING BUSINESS SUSTAINABILITY
By using available data, machine learning has provided better and new actionable insights to help retailers curate a superior and fresher product range, as well as achieve more competitive pricing and healthier stock levels. These data-driven capabilities coupled with changes to existing business processes enable retailers to sustain high performance in an increasingly competitive environment.
There are many use cases where retailers have benefitted from the deployment of machine learning capabilities. Besides leveraging advanced analytics like customer basket analysis to predict, upsell or cross-sell complementary products, promotions and offers can now be tailor-made according to customers’ purchase patterns. Strategic product placement can also be made based on customers’ propensity to purchase both products if they are within sight at the same time. Similarly, non-performing products can be replaced based on recommendations provided by such capabilities.
Most importantly, as customer purchase patterns continue to be captured, modelled and refined daily, not only can customers always get the products they want in the store they choose to go to, businesses are also assured of delivering good customer service every time. Undoubtedly, machine learning capabilities are key to ensuring sustainable business performance in the current COVID-shaped business landscape.
SALES DOLLARS (PRE PILOT VERSUS PILOT)
PILOT START HALLOWEEN
9/16/20 9/26/20 10/6/20 10/16/20 10/26/20 11/5/20