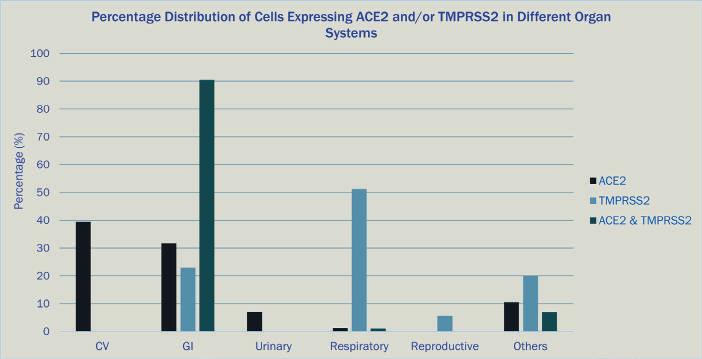
12 minute read
Powerful, Large-scale Analytics brings Single-cell Omics into Clinical Reality
from IPI Winter 2020
by Senglobal
Abstract / Summary Recent advances in sample preparation, biochemistry, and informatics tools for single-cell analysis have enabled the rapid adoption of single-cell omics in both biomedical research and, more recently, in clinical practice. While empowering the development of better therapeutics and diagnostic tools, the ongoing evolution of such methods, including single-cell DNA and RNA sequencing and proteomics, has resulted in vast, ever-growing datasets that require powerful data management and computational capabilities to capture clinical value. Here, we highlight the challenges imposed by current single-cell computational methods in handling vast datasets from disparate sources, and what is needed from an analytics platform for robust and reliable scientific data modelling, storage, and large-scale computation. We present the results of a recent study demonstrating the utility of such a platform, which enables the rapid profiling of key genes involved in COVID-19 infection.
Growth of Single-cell Analysis Developments in single-cell analysis have substantially improved our understanding of disease mechanisms in recent years. The single-cell analysis market is projected to reach USD 5.6 billion by 2025 from USD 2.1 billion in 2019, with its growth attributed to technological advancements in single-cell analysis products, increasing government funding for cell-based research, growing biotechnology and biopharmaceutical industries, wide applications of single-cell analysis in cancer research, growing focus on precision medicine, and the increasing incidence and prevalence of chronic and infectious diseases.1 Single-cell analysis has therefore become a major focus for translational and pharmaceutical research, enabling multi-omics analysis at the singlecell level and allowing the identification of minor subpopulations of cells that may play a crucial role in various biological processes.
As a highly-sensitive tool, single-cell analysis can clarify specific molecular mechanisms and pathways, and even reveal the nature of cell heterogeneity. With this advancing technology, researchers and clinicians can look for insights into the transition from ‘healthy’ to ‘disease’ states, study potential biomarkers, and assess response to drug targets or available therapeutic regimens. Market Shift The notion of precision medicine – designing healthcare strategies according to a person's genes, lifestyle, and environment – is not a new one. Over the last two decades, significant advances in genomic, proteomic, transcriptomic, and epigenomic sciences, in conjunction with the growing availability of vast patient data repositories, have gradually facilitated a landscape of data-driven clinical decision-making. As well as predicting personal risk factors for particular diseases and how individual responses to various treatments might differ, this precision medicine methodology is slowly extending into the drug discovery paradigm.
In 2018, a record number of 25 new molecular entities (NMEs) approved by the U.S. Food and Drug Administration (FDA)’s Center for Drug Evaluation and Research (CDER) were categorised as personalised medicines (42% of all 2018 new drug approvals).2 In addition, governments around the world are recognising the considerable potential of precision medicine to transform patient care. Former US President Barack Obama launched the Precision Medicine Initiative in 2015, which has since evolved into the National Institute for Health (NIH) All of Us Research Program that aims to gather health data from more than a million US volunteer-citizens to enable individualised treatment and healthcare.3 The ‘Cells-patients-data’ Relationship Despite the potential of single-cell omics to bring precision medicine approaches into routine clinical practice, the lack of analytics solutions available to cope with large-scale single-cell datasets poses a significant barrier. Single-cell DNA and RNA sequencing produce vast amounts of data. Information from tens of thousands of cells per patient is available and while this provides clear opportunities in terms of increasing the statistical power of growing datasets, the technical and interpretative challenges associated with such ‘Big Data’ are currently limiting accessibility to biological insights. To unlock the value of recent advances in single-cell technology, life scientists will need to tackle the variety of omics layers (genomes, epigenomes, transcriptomes, and proteomes), along with reference maps like the Human Cell Atlas (HCA), at unprecedented levels of resolution, specificity, and volume. As the scale of single-cell datasets continues to increase, there is an unmet technological need to develop database platforms that can evaluate key biological hypotheses by querying atlases of single-cell data.
However, current single-cell data are generated from a small number of individuals, and statistical significance relies on the number of patients studied, rather than the number total of cells. This is because cells from the same patient are ‘siblings’ and not true biological replicates, so datasets with 100,000s of patients/ treatment conditions will necessitate technology to manage billions of cells. For example, the Immune Cell Survey in the HCA – an initiative aiming to map the numerous cell types and states comprising a human being – currently contains 780,000 cells from only 16 individuals. The HCA itself has fewer than 400 patients, with very few patients donating cells from more than one organ system. This ‘cells-patients-data’ relationship is further compounded by a lack of scalability of single-cell software, as well as temporal factors, as researchers study the evolution of cell (sub) populations and the effects of treatments over time. Overcoming the Data Dilemma As well as moving beyond the small number of patients currently used in the available datasets, the pharmaceutical industry must take several steps in order to overcome the data management and analysis roadblocks in single-cell workflows. Firstly, there is a need for a single unified repository to store an organisation’s entire single-cell data – both raw and normalised – that is
organised intuitively and enables secure data transactions from unlimited users, in contrast with the persistent use of files and silos of data. Current tools also limit cross-study analysis, which is crucial for confirming results.
As a root cause, data sparsity limits the extraction of maximum value from single-cell omics data. For example, in single-cell RNA sequencing, when a given gene in a given cell has no unique molecular identifiers or reads mapping to it, large numbers of observed zeros occur in the measurement values.4 The proportion of observed zeros, or degree of sparsity, can hinder downstream analysis performance and requires significant bioinformatics expertise to handle storage and computation. Statistical models that inherently model the sparsity, sampling variation, and noise modes of single-cell RNA sequencing data with an appropriate data generative model are needed. Empowering Drug Discovery To accompany the growing focus on precision medicine, the pharmaceutical industry is embracing the challenge of Big Data. The ability of single-cell analysis to assist the creation comprehensive tissue and cell atlases is driving discoveries in pharmaceutical research. For example, singlecell RNA sequencing in respiratory research has led to the detection of a transcriptionally novel cell type – termed a ‘pulmonary ionocyte’ – that expresses large quantities of CFTR (cystic fibrosis transmembrane conductance regulator), the causal gene of cystic fibrosis.6,7 Findings such as this have had and will continue to have important implications for gene-therapy approaches to cystic fibrosis, and change the way drugs are developed to treat such diseases.
Despite such discoveries, challenges still exist regarding the mapping of single cells to reference atlases, as they are under constant development. New solutions are emerging that aim to overcome this barrier, to enable biological meaning to be derived from subpopulation identification and facilitate operation at various levels of resolution of interest. An ideal platform would also cover continuous, transient cell states, quantify the uncertainty of a particular mapping of cells of unknown type/state, scale to increasing numbers of cells as well as broader coverage of types and states, and ultimately integrate information generated through multiple types of measurements.4 Case Study: COVID-19 and the Need for Rapid Data Analysis The recent advances in high-throughput single-cell analysis technology have expedited the HCA Project – an international collaborative effort that aims to define all human cell types in terms of distinctive molecular profiles (e.g., gene expression) and connect this information with classical cellular descriptions (e.g., location and morphology).8 The translational promise of the cell atlas ranges from basic biology of the human organism, to disease mechanism, diagnosis, prognosis, and treatment monitoring, to immunotherapy, drug development, and cell and organ replacement.9 Sophisticated single-cell analysis platforms are allowing researchers to gather crucial insights from databases such as the HCA, which is now emerging as an important contributor to the effort to better understand mechanisms underlying coronavirus disease-19 (COVID-19).10
Evaluating the expression profile of key genes, such as those encoding key viral entry associated proteins, in large single-cell datasets can facilitate testing for diagnostics, therapeutics, and vaccine targets. For example, initial work aimed at understanding SARS-CoV-2 pathology at the single-cell level – some of which used previously generated HCA data – focused on understanding which cell types expressed the gene encoding the SARS-CoV-2 receptor, ACE2. While large single-cell datasets like the HCA and COVID-19 Cell Atlas (CCA) are excellent resources for profiling target genes involved in infectious diseases like COVID-19, collating data from multiple research groups internationally is a significant feat, and the full utility of these atlases is limited due to the lack of a database management strategy. Such a strategy would facilitate cross-comparison of the distribution and levels of specific gene expression between samples and projects, without a significant bioinformatics and computational effort. Elucidating Relationships The development of scalable platforms is now enabling researchers to make simple queries in reasonable timeframes and, for example, to access and evaluate multiple single-cell sequencing datasets to develop improved therapeutic targets for clinical studies. This is particularly pertinent to the ongoing effort to develop successful COVID-19 treatments and vaccines. Recent research has indicated that in addition to the well characterised respiratory disease resulting from SARSCoV-2 infection, the virus can cause systemic pathology including in the gastrointestinal tract,11 cardiovascular system,12 endocrine system,13 and central nervous system.14 Elucidating the relationship between these observations and tissue/cell distribution of receptors and gene expression profiles is time-critical.
One study assessed the capabilities of a new single-cell data analysis platform in querying all cells in the HCA and CCA databases that express either the ACE2 receptor, TMPRSS2 – the entry facilitating enzyme, transmembrane serine protease – or co-express both markers15 (Figure 1). The majority of cells expressing ACE2 had a cell type tag of PC_vent1 (heart tissue); the majority of cells expressing TMPRSS2 had a cell type tag of AT2 (alveolar epithelial type II cells found in the lung parenchyma); and most cells co-expressing both ACE2 and TMPRSS2 were tagged as gallbladder cells.
Rapid Gene Profiling The results go some way to explaining the multi-organ involvement in infected patients observed globally during the
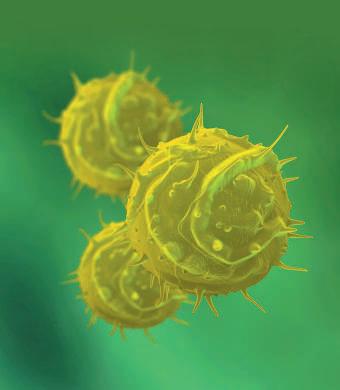
ongoing COVID-19 pandemic, as multiple cell types in the human body express genes utilised by SARS-CoV-2 for infection. The analysis platform enables quick profiling of key genes involved in the infection and supports additional use cases that require evaluation across a large database of single-cell expression datasets such as vaccine candidates for infectious diseases, biomarkers for oncology patient stratification, and immunology-related disorders. Single-cell in the Future Single-cell omics technologies, such as single-cell RNA and DNA sequencing, have evolved significantly in recent years, resulting in enormous datasets that require powerful, optimised processing and data management and analysis methodologies to extract translational value. Classifying cells into cell types or states is essential for many secondary analyses, for example to study and classify how expression within a cell type varies across different biological conditions, to understand the cellular basis of off-target drug effects, and to find new indications for approved drugs. Cell atlases like the HCA and CCA, as reference systems that systematically capture cell types and states, must be able to embed new data points into a stable reference framework that allows for different levels of resolution. In turn, pharmaceutical R&D requires a nextgeneration analytics platform that enables scientific data modelling, storage, and large-scale computation to allow single-cell analyses to drive future clinical decisionmaking.
Such advances are being demonstrated today in the ongoing COVID-19 research effort, which has posed a unique challenge to scientists collaborating at an unprecedented scale. Coordinating, pooling, and sharing datasets from laboratories across the world, and integrating existing repositories such as the HCA and the COVID Cell Atlas, would not be possible without powerful computational tools that are now providing insights into the impact of SARS-CoV-2 on different cell types in the body. This single-cell level analysis gives hope of addressing key questions in the virus’ transmission, epidemiology, and pathogenesis, ultimately informing pharmaceutical research.
For more information about how to empower your single-cell-based research, please visit https://www.paradigm4.com/. REFERENCES
1. Single-cell Analysis Market by Cell Type (Human, Animal, Microbial), Product (Consumables, Instruments), Technique (Flow Cytometry, NGS, PCR, Mass Spectrometry, Microscopy), Application (Research, Medical Application), End User - Global Forecasts to 2025, MarketsandMarkets, 2020. Personalized Medicine at FDA: A Progress & Outlook Report, Personalized Medicine Coalition, 2018. http:// www.personalizedmedicinecoalition.org/ Userfiles/PMC-Corporate/file/PM_at_FDA_A_ Progress_and_Outlook_Report.pdf [Accessed 30/09/20] 2. National Institutes of Health (NIH) All of Us Research Program. https://allofus.nih.gov/ [Accessed 30/09/20] 3. https://www.marketsandmarkets.com/MarketReports/single-cell-analysis-market171955254.html 4. Lähnemann D, Köster J, Szczurek E et al. Eleven grand challenges in single-cell data science. Genome Biol 21, 31 (2020). https://doi. org/10.1186/s13059-020-1926-6 5. A new approach to R&D at GSK, GlaxoSmithKline, 2018. https://www.gsk.com/media/5041/ rd-update-slides-hal-barron.pdf [Accessed 30/09/20] 6. Alexander MJ, Budinger GRS and Reyfman PA. Breathing fresh air into respiratory research with single-cell RNA sequencing, European Respiratory Review, 2020; 29(156):200060. 7. Plasschaert LW, Žilionis R, Choo-Wing R et al. A single-cell atlas of the airway epithelium reveals the CFTR-rich pulmonary ionocyte. Nature, 2018;560: 377–381. 8. Regev A, Teichmann SA, Lander ES et al. The Human Cell Atlas, bioRxiv. doi: https://doi. org/10.1101/121202 9. The Human Cell Atlas White Paper, The HCA Consortium, 2017. https://www.humancellatlas. org/wp-content/uploads/2019/11/HCA_ WhitePaper_18Oct2017-copyright.pdf [Accessed 30/09/20] 10. Teichmann S and Regev A. The network effect: studying COVID-19 pathology with the Human Cell Atlas. Nat Rev Mol Cell Biol, 2020;21: 415–416. https://doi.org/10.1038/s41580-020-0267-3 11. Cheung KS, Hung IFN, Chan PPY et al. Gastrointestinal Manifestations of SARS-CoV-2 Infection and Virus Load in Fecal Samples From a Hong Kong Cohort: Systematic Review and Meta-analysis, Gastroenterology, 2020;159(1): 81-95 12. Zhou L, Niu Z, Jiang X et al. Systemic analysis of tissue cells potentially vulnerable to SARSCoV-2 infection by the protein-proofed singlecell RNA profiling of ACE2, TMPRSS2 and Furin proteases, bioRxiv, 2020. doi: https://doi. org/10.1101/2020.04.06.028522 13. Somasundaram NP, Ranathunga I, Ratnasamy V et al. The Impact of SARS-CoV-2 Virus Infection on the Endocrine System, Journal of the Endocrine Society, 2020;4(8): bvaa082 14. Najjar S, Najjar A, Chong DJ et al. Central nervous system complications associated with SARS-CoV-2 infection: integrative concepts of pathophysiology and case reports. J Neuroinflammation, 2020;17: 231. 15. Kumar N, Golhar R, Sharma K et al. Rapid Single Cell Evaluation of Human Disease and Disorder Targets Using REVEALTM: SingleCell, bioRxiv 2020.06.24.169730; doi: https://doi. org/10.1101/2020.06.24.169730
Marilyn Matz
Marilyn Matz is CEO and co-founder of Paradigm4. She completed an MS degree at the MIT AI lab and was one of three co-founders of Cognex Corporation. Marilyn received the sixth annual Women Entrepreneurs in Science and Technology (WEST) Leadership Award and was a corecipient of the SEMI industry award for outstanding technical contributions to the semiconductor industry; and a 2020 NACD Directorship 100.
Zachary Pitluk
Zachary Pitluk is the Vice President of Business Development, Life Sciences and Healthcare at Paradigm4. He has 23 years’ experience in sales and marketing ranging from being a pharmaceutical representative for BMS, to management roles in life science technology companies since 2003. His academic positions include Yale University Department of Molecular Biophysics and Biochemistry: Associate Research Scientist, Postdoctoral Fellow and Graduate Student.