TransportTransform SECOND EDITION ParadigmShiftingtheimpactofCovid-19ontransportplanning
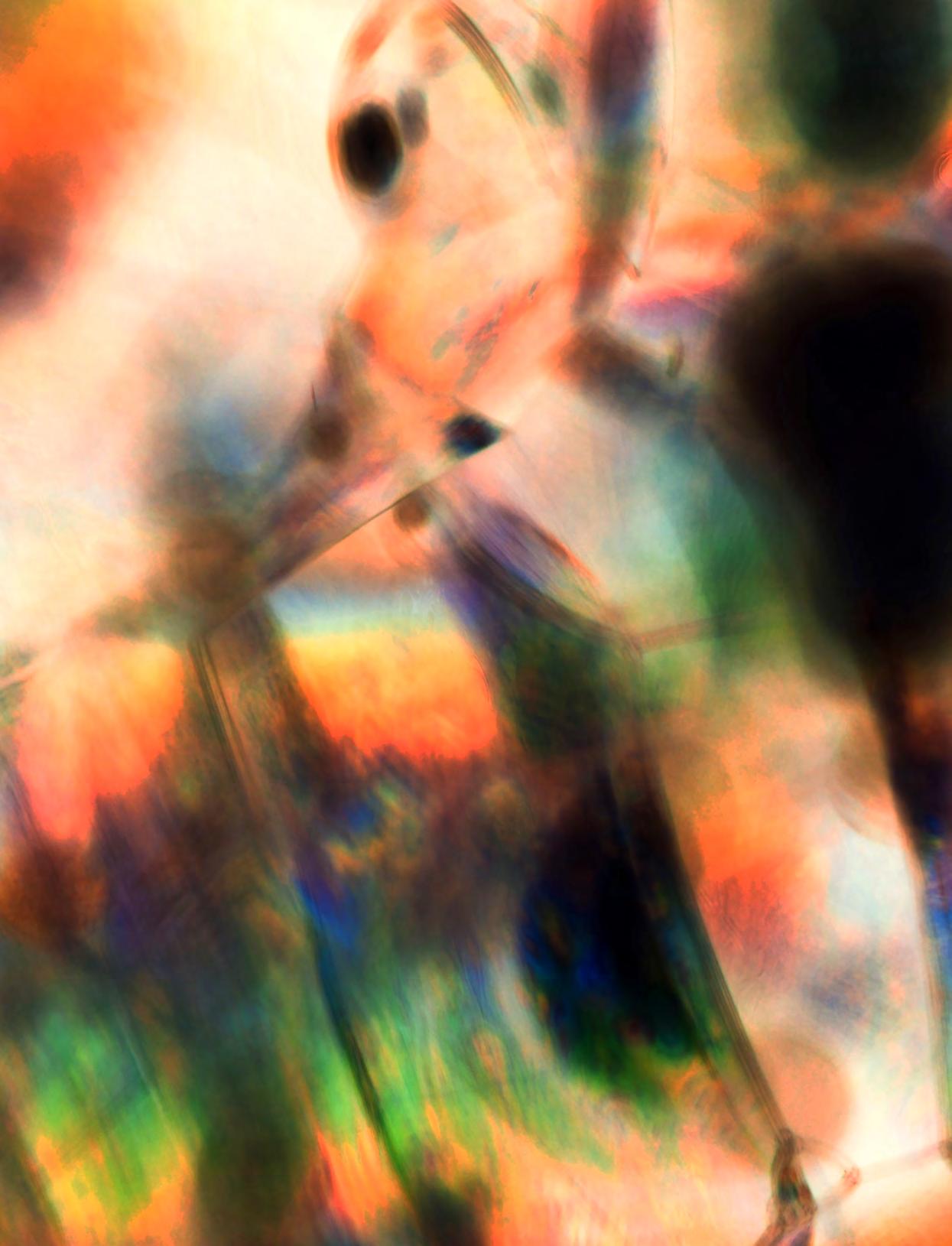
ansporttranspor
— Fabio Casiroli, Khrónopolis2008
"And who has never wondered if the rapid growth of large urban agglomerations could turn into a nightmare? I believe that it can be turned into a hope, if we know how to design a city able to guarantee essential rights of urban civilization to everyone, among which is the mobility right, always and everywhere."
Shifting Paradigm: the impact of Covid-19 on transport planning (second edition) Made in Milan by Transform Transport © 2022 Fondazione Transform Transport ETS All researches presented in this book are developed by Transform Transport. All rights reserved. Unauthorised use is prohibited. No part of this publication may be reproduced in any form or by any means without the written permission of Fondazione Transform Transport ETS Fondazione Transform Transport ETS Via Lovanio 8 20121 Milan, Italy +39 02 62 31 19 PrintedISBN:info@transformtransport.orgwww.transformtransport.org19788894713404inMilaninSeptember 2022 How to cite this book: Transform Transport (2022). Shifting Paradigm: the impact of Covid-19 on transport planning (second edition). Fondazione Transform Transport ETS. Via Lovanio, 8 20121 — Milan,Italy t +39 02 62 31 19 1 www.transformtransport.orginfo@transformtransport.orgFondazione Transform Transport Research and Innovation in Mobility and Transport Planning
Executive Summary p.6 Walkable Cities p.8 ● milan sidewalks map ● milan sidewalks network ● how covid-19 affected pedestrian modeling and simulation Living Local p.30 ● mapping micro-centers in milan ● access to green areas and the public realm ● walkabilty and the 15-minute city model ● stories: a platform built for place-based storytelling New MetricsUrban p.62 ● looking with machine eyes ● monitoring activity patterns in milan through wi-fi sensors ●datasensors data for unlocking hidden city metrics ● analysing conversation''twitterontube stations Way Forward p.90 Bibliography p.92 Table of Contents
This book is a structured collection of the most insightful investigations developed by Transform Transport since the Covid-19 outbreak. The unprecedented disruption caused by the pandemic has given rise to a large cultural and technical debate on new upcoming forms of urban development and mobility with the aim of envisioning potential trajectories of new urban paradigms and keeping up with the pace of our changing cities. Among the countless urban challenges, this book focuses on the issues of walkability, access to the public realm and barriers to enabling more localized living. Nearly 15 years after Systematica's founder, Fabio Casiroli's early investigations into chronocentric cities, we revisit the topic through the ’15-minute city’ paradigm, the latest perspective for fostering sustainable, inclusive and resilient cities, focusing on time as a shaper of urban
experiences. The book also aims to tackle technical challenges to read novel mobility patterns in the wake of the pandemic, addressing these through the investigation of advanced urban planning tools and new urban metrics. Through innovative city instruments, multi-dimensional metrics and deep learning techniques, this publication explores everything from the dense network of sidewalks to the actual accessibility to urban parks and investigates hidden people movement patterns through big data, video/ image analytics and data sensors, unveiling emerging correlations that could support future urban scenarios and strategies. Relying primarily on the city of Milan as the analytical testbed, these methodologies have the potential to be replicated in many global cities, to help bridge urban structural gaps and inform effective intervention strategies to address today’s complex mobility challenges.
6
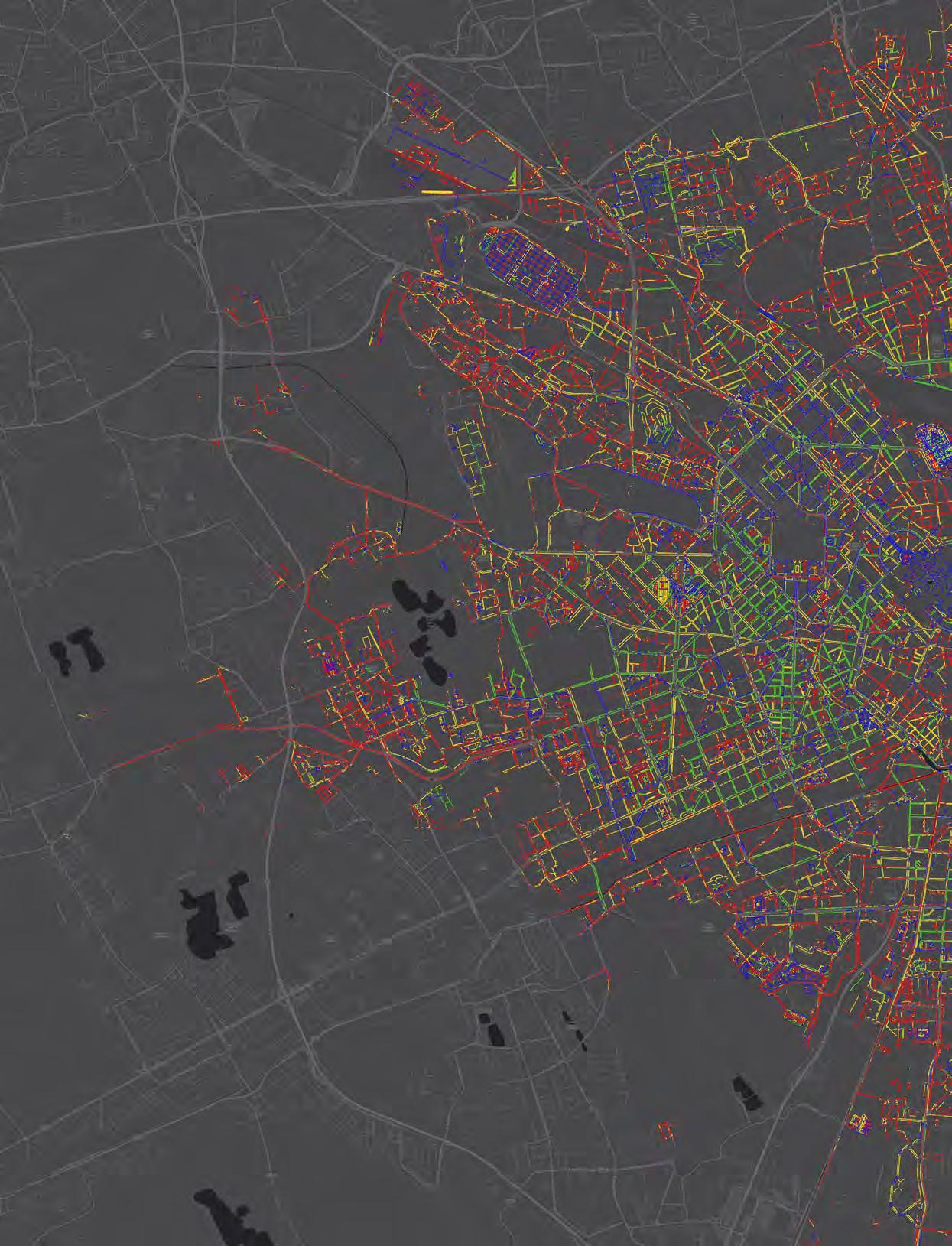
Thetravel.second chapter, Living Local is generally concerned with the theme of the 15-minute city. Closely related to the theme of walkability and often overlapping, the contributions of this chapter aim to test the practical application of the complete neighborhood concept as a viable way forward for an urban living model. It aims to explore pathways for bridging the theory with practice, presenting some initial technical methodologies for putting the model into practice. One of the contributions also focuses explicitly on the accessibility of public green spaces to city residents by foot living in different parts of the city, highlighting differences in access to open-air gathering spaces deemed especially crucial to urban life within the pandemic state.
This book is designed as a curated collection of research contributions conducted between March 2020 and March 2022 around the theme of the Covid-19 pandemic and its relation to urban and mobility planning. Contributions within the book range from academic research to thought pieces developed to test particular ideas or theories within a particular context or at a specific point in time during the unfolding Covid situation. Given the time span of the collection, some pieces are evolutions of earlier studies, reflecting, in part, the progression of the pandemic situation itself. Collectively, the contributions are categorized under 3 broad themes, which, in turn, make up the chapters of this book: (i) Walkable Streets, (ii) Living Local and (iii) New Urban Metrics. Shifting Paradigm: the impact of Covid-19 on transport planning is the second edition of the same publication first published in 2021. In the second edition, the research contributions are expanded to elaborate on each study in depth and present any new findings that have since been reached by the research team at Transform Transport. Moreover, the second edition includes three new contributions that have been developed over the past year: one on the topic of ‘Living Local’ and two under the theme of ‘New Urban Metrics’.
7
SummaryExecutive
It also presents a replicable methodology to evaluate urban pedestrian infrastructure under the lens of the pandemic situation, highlighting areas where interventions are needed to ensure safe pedestrian
Most of the applications covered in this book focus on the city of Milan, Italy – one of the first epicenters of the Covid-19 pandemic in Europe and the urban center in which the Transform Transport research foundation is based –. Each case, however, is supported by customizable and easily replicable methodologies that can be repurposed to study the varying situations in other global cities as the legacy of the pandemic situation continues to restructure and shape the way we inhabit the urban environment.
The final chapter, New Urban Metrics focuses on the application and use of new urban technologies to unearth hidden mobility patterns throughout the various pandemic phases, which could not have otherwise been studied using traditional transport planning instruments. From Wi-Fi sensors to video processing using pretrained deep learning models, to the analysis of geo-located, user-generated social media data, this chapter aims to demonstrate the capabilities of the Urban Informatics approach to extract meaning from unprecedented mobility situations.
The first chapter, Walkable Cities is focused on the theme of walkability. It gathers contributions that were developed to look at how Covid-19 impacted not just pedestrian behavior, but also pedestrian modelling tools used in the planning field, testing their applicability under conditions of a health crisis.
Chapter 1 8
Sidewalks becomingincreasinglyarethe new protagonists of the street. If not already for environmentalsocialphysical,and benefits, walkability is as important as ever due to the pandemic. Cities around the world urbanholisticintegratedresponsethecyclistsforreclaimingarestreetspedestriansandtoensurethatpandemiciswell-withinasustainablevision. 9
In a hyper-connected global world, emergency health risk situations are growing at an increasingly high rate. Overcrowding and person-to-person proximity are by definition critical factors in the subject of pandemic risk. To that end, shared public spaces became objects of contention during the COVID-19 pandemic situation.
Of all public spaces, sidewalks have a particular value as channels of pedestrian movement and carry a particular risk of proximity due to their intrinsically narrow bodies. This first article presents a mapping study aimed to analyze sidewalks across the city of Milan in order to test their capacity to accommodate necessary social distancing requirements to reduce the risk of contagion. It proposes a replicable methodology for any urban center, urban environment or metropolitan area to follow for similar purposes.
The preliminary results of this exercise reveal that about 45% of Milan's sidewalks by length are not wide enough to allow for safe travel during times of high pandemic risk based on gross pavement width. Further analysis at local scale is recommended to account for sidewalk fixtures and furniture and the impact they have on actual walkable width.
MapSidewalksMilan
10
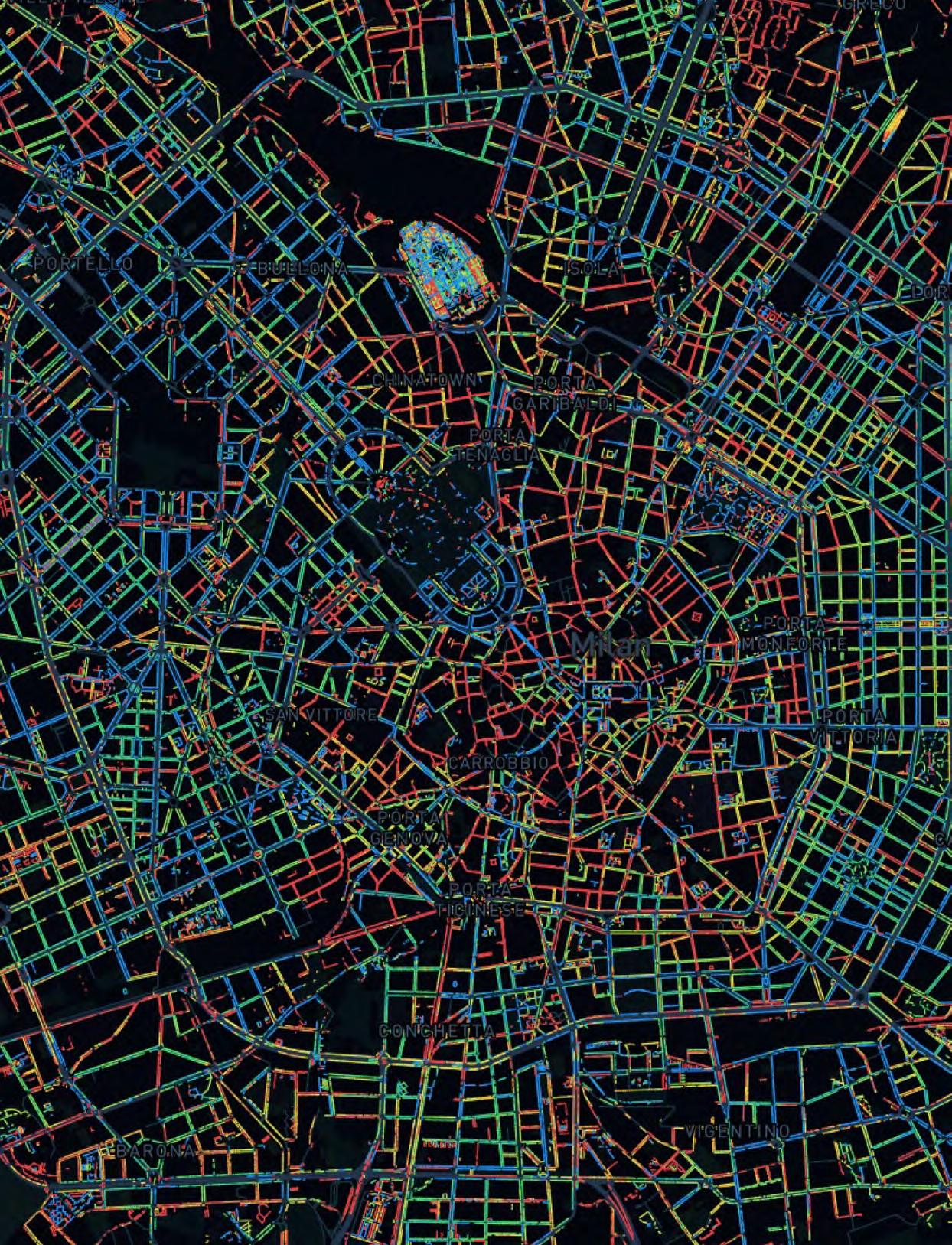
• Bolstering the capacity to analyze and plan pedestrian spaces;
The Importance of Mapping Pedestrian Infrastructure
UnsplashonPaoladeMickbyPhoto
The width of a sidewalk contributes to the level of comfort in using that infrastructure. Sidewalks that are too narrow can create zones of conflict where pedestrians encroach on road space, leading to dangerous situations.
• Quickly adapting infrastructure to emerging needs.
Cities have a huge amount of information on vehicular traffic in our cities: road hierarchy, number of lanes, vehicles per hour, level of congestion, etc. In contrast, the level of information cities have for their most prominent users - pedestrians - is weak. This study aims to highlight how the mapping of pedestrian infrastructure is fundamental to our understanding of urban mobility and to offer a useful tool for city administrations and planners.
Scaled up and diffused across wider territories, this tool has the potential to exponentially increase the ability to analyze and plan pedestrian spaces, serving the fundamental need for rapid adaptation, which is crucial not only under crisis conditions but beyond, that in line with global agendas for sustainable mobility (United Nations, 2016).
Once shared, mapping can be a fundamental decisionmaking tool for planners and citizens as well.
1,000 - 2,800/HR 7,500/ HR 4,000-8,000/ HR 9,000/HR Mixed traffic with frequent buses Two-waybikeway Dedicated transit lanes Sidewalks Figure 1 Capacity load of diverse transport modes in equal road segments Source: Freely taken from https://nacto.org/
Walking is considered a primary mode of travel with various personal and collective benefits. It is a healthy, free and sustainable travel choice and a fundamental component of daily urban life (Speck, 2013). Sidewalks are therefore crucial components in city planning as they support a primary mode of travel (walking) that in turn, supports all other travel options (see Figure 1).
• Prioritizing and defining the type of interventions required based on the existing situation;
Benefits for the Municipality
11
Mapping the pedestrian infrastructure inevitably brings significant advantages to multiple actors. First, administrations will gain a number of benefits, including:
• Verifying the suitability of pedestrian infrastructure with respect to the rules of social distancing;
Comfortable sidewalks instead support and encourage pedestrian traffic by increasing the attractiveness of the street and bringing widespread advantages.
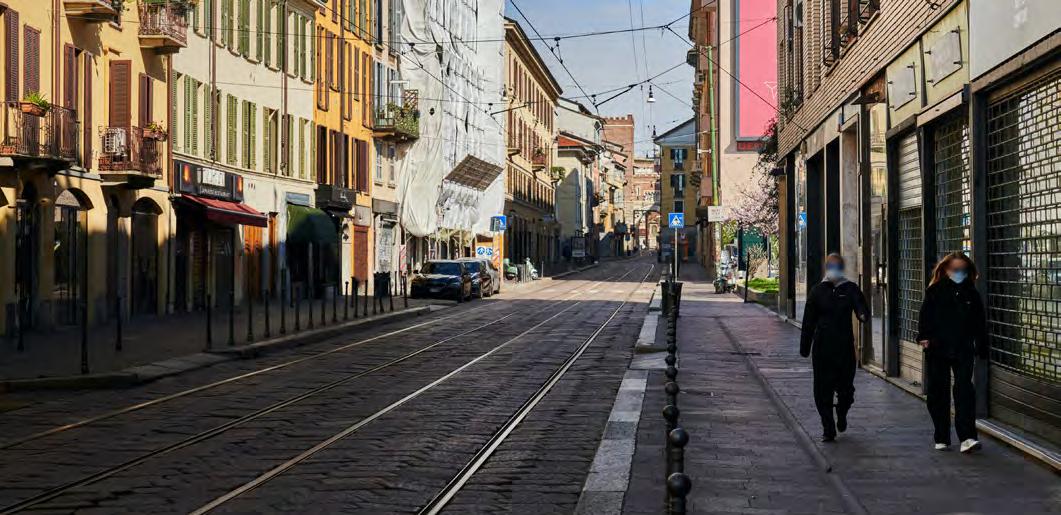
< 2,4 m – unsafe sidewalk >= 2,4 and < 3,3 m – acceptable sidewalk >= 3,3 and < 4,2 m – safe sidewalk >= 3,3 and < 4,2 m – ideal sidewalk 45% of the sidewalks are potentially unsafe and some interventions shold be put in place 17% of the sidewalks have ideal width Sidewalks width Milan
Figure 3 Distribution of sidewalk safety in the city of Milan
The research started from the methodology and results of the study presented by Meli Harvey1 and related to the sidewalk network of New York City. First, initial data from Milan was analysed to develop a sense of the existing situation of sidewalks on a local scale using the proposed ranking system (see Figure 2). The analysis revealed that particular attention should be paid to the sidewalks in the "ideal" category given that many of them are actually occupied by trees, cycle lanes or vehicles illegally parked on the sidewalk space.
Sidewalk < 2.4 m Extremely dangerous situation. At less than 2.4 meters of free width, it is not possible to maintain the required distance.
Sidewalk >= 2.4 and < 3.3 m Above 2.4 meters of free width, can work under emergency conditions when movements are limited and primarily individual.
Expanding the analysis to city scale, a total of 45% of sidewalks across the city were found to not meet the minimum requirements to guarantee adequate social distancing (see Figure 3). Of the remaining 65%, a significant portion requires intervention in the form of measures to increase the space available on wider sidewalks, such as removing parked cars on sidewalks. For Unsafe and Extremely Unsafe sidewalks, removing on-street parking or closing traffic lanes (temporarily
Sidewalk >= 3.3 and < 4.2 m Above 3.3 meters of free width, it is possible to move safely, not necessarily individually or in one direction of travel. Sidewalk >= 4.2 m Above 4.2 meters of free width, it is possible to move safely in groups of more than one and in both directions simultaneously. or permanently) may be needed to guarantee sufficient pedestrian space. Figure 4 shows a set of maps for each rank of sidewalk width to help identify cluster areas where intervention should be prioritized.
12
Figure 2 Sidewalk width safety ranking scale
Social Distancing as Criteria of Analysis
In the Lombardy region, the recommended interpersonal distance for contagion containment is one meter (Regione Lombardia, 2020). Starting from this assumption, the space occupied by an individual was taken into account (0.6 m + 0.2 m of comfort zone) and different sidewalk widths were ranked on safety based on their capacity (by width) to fulfill social distancinng requirements. The measurements refer to the net walkable width of the pavement. Ranking Sidewalks in Milan

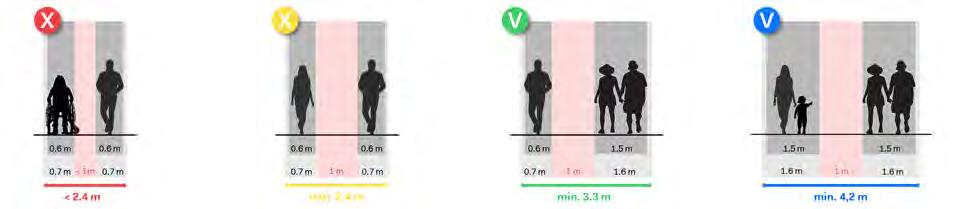
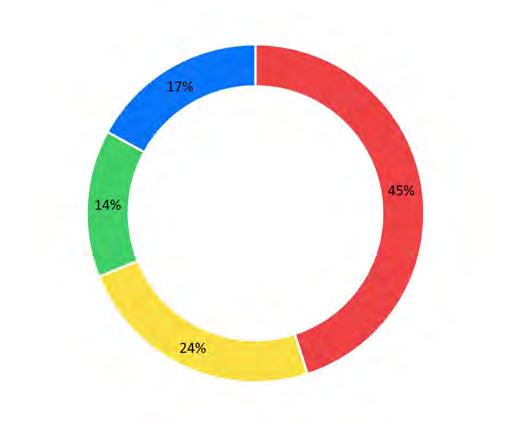
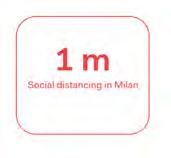
Figure 4 Mapping Milan's sidewalks by safety rank 13
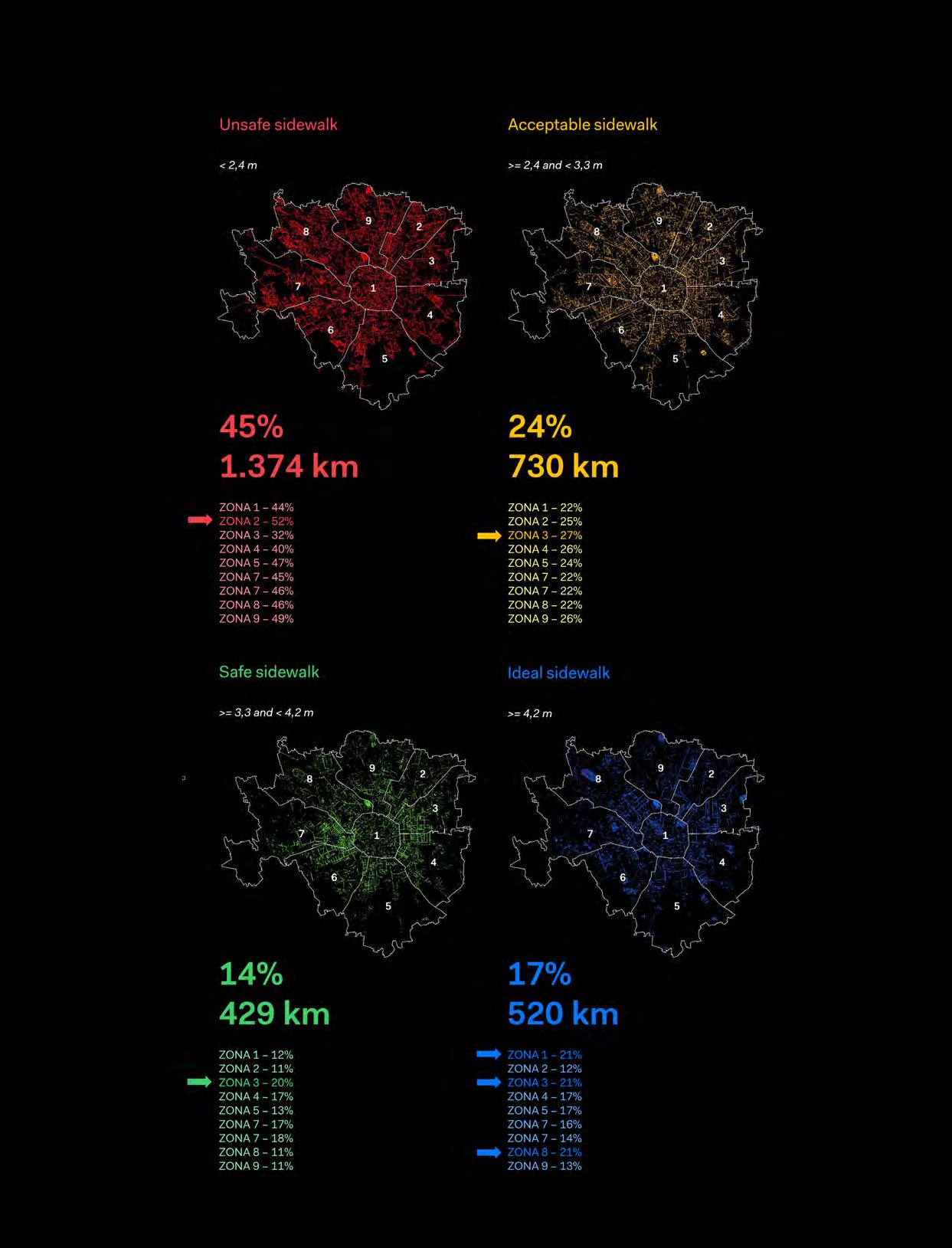
The Making of the Map
Step 1: The map was built starting from the polygons of the pavements available as open source on the Geoportal of the Municipality of Milan2.
Step 3: The data was then simplified and cleaned up to make it more comfortably usable by removing all segments shorter than 20 cm, considered not essential for visualization or analytical purposes.
Step 2: Starting from these polygons, the center line of each single sidewalk was obtained and the distance from the edges was calculated, namely the distance between the edge of the roadway and the buildings. The results obtained therefore show the width of each sidewalk not taking into account physical abstacles occupying sidewalk space.
Step 4: Once the new database was created, an interactive map3 was developed and published Figure 5 Mapping process divided into 4 steps
14
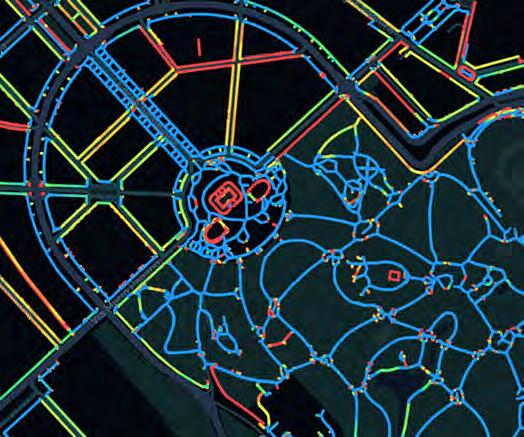
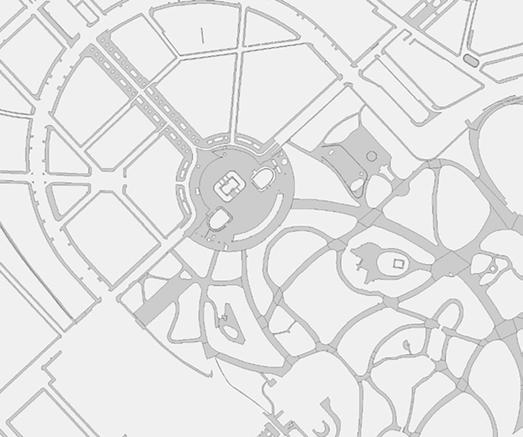
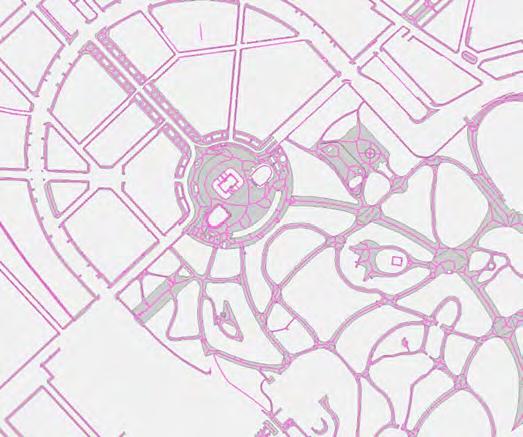
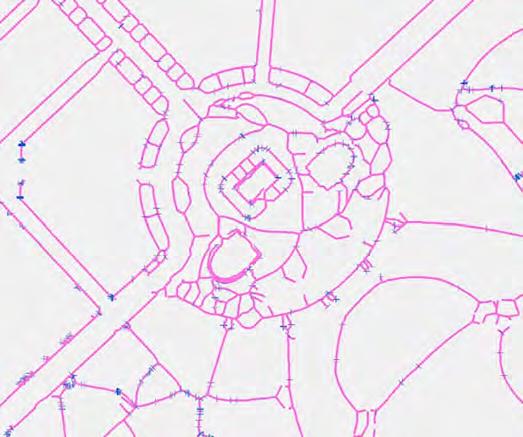
In sum, this mapping tool has the potential to become an aid for cities in decision-making by facilitating integrated mobility planning at urban and neighborhood scale, aiding in the implementation of walkability initiatives.
15
Undeniably, further studies looking at the distribution of these obstructive elements would provide deeper insights and allow for a more precise mapping of the real active widths of the pavements.
Next Steps Today, Milan's pedestrian infrastructure does not guarantee a homogeneous level of comfort to ensure pedestrian safety on sidewalks. It is therefore unsurprising that the Milan Municipality has launched wide-scale pedestrianization initiatives across the city during the course of the pandemic to reduce the associated risks (Comune di Milano and AMAT Agenzia Mobilità Ambiente Territorio, 2020).
Milan Sidewalks Map (www.milansidewalks.com)
Further Considerations to Refine the Map
The final resulting map3 (see Figure 6) is an intricate web of gross sidewalk widths across the city of Milan. It gives a sense of the distribution of widths by safety ranking as predefined in previous sections and it gives some indication of better performing and worse performing areas.
In any case, it is of fundamental importance to have access to a comprehensive mapping of our city's pedestrian infrastructure. Undoubtedly, further refinement and detailing of the map is required to ensure reliability and practical application. It is also important to collaborate with city administrations to enable constant upgrading of the database to reflect changing conditions. Furthermore, it would be very interesting in future phases to analyze this sidewalk database alongside other mobility information such as road hierarchy, vehicular traffic, public transport accessibility levels, etc.
A preliminary study based on the sample analysis of a limited number of Milanese streets indicates that, on average, about 0.3-0.5 m and 1.5-2 m of the gross width of sidewalks on neighborhood streets (for non-tree-lined streets and tree-lined streets, respectively) is occupied by street Parkingfurniture.onsidewalks is another prominent issue that reduces the attractiveness and comfort level for pedestrian users, while also contributing to a reduced level of safety. On Via Bazzini in Città Studi, for example, the gross width of the sidewalk is greater than five meters, yet in reality 70% of the space is occupied by parked cars.
Figure 6
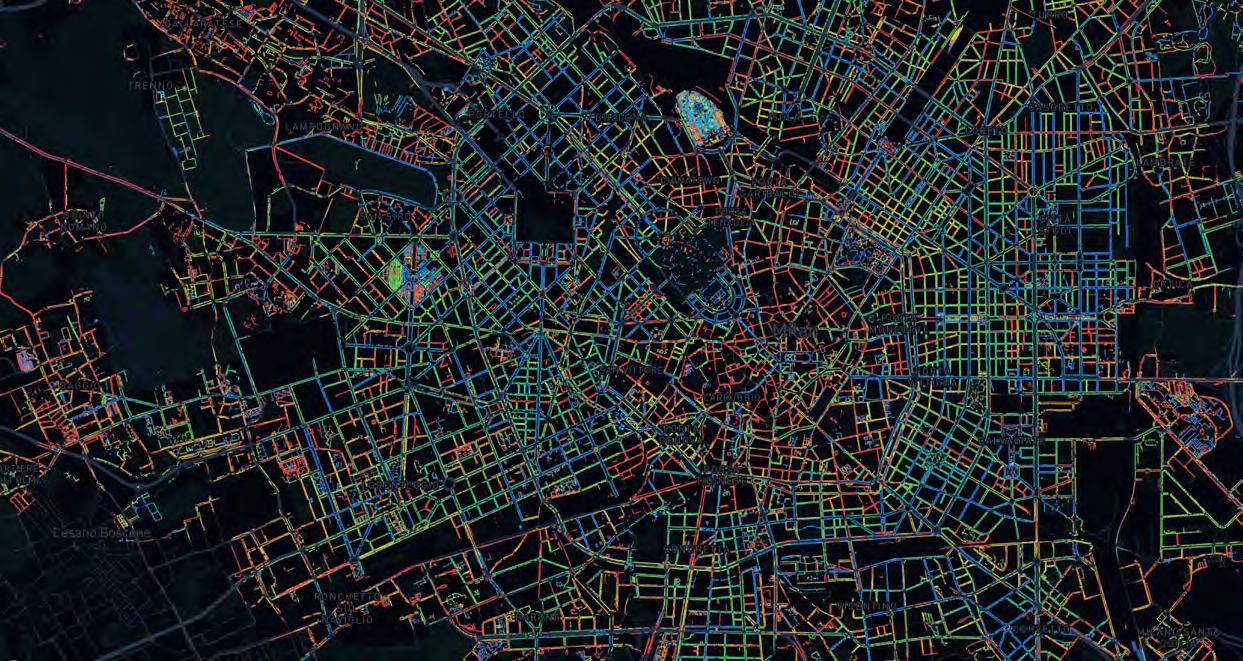
Milan Sidewalks Network: InterventionPriorityAreas
16
This article is based on the results of the study presented in the previous article, Milan Sidewalks Map in which 45% of all sidewalks in Milan were revealed to be insufficiently narrow for safe pedestrian travel. This share, which corresponds to approximately 1,400 km of sidewalk space is a massive extent to be tackled comprehensively for timely intervention. During the critical phase of the pandemic situation, policies often relied on priority-based indicators to effectively utilize resources towards the needs of the most at risk. To that end, this article proposes a useful methodology for identifying the most at-risk areas of the city on a first-need basis. The methodology proposed in this study is intentionally simplified, focusing on the most vulnerable populations in the pandemic state, namely the elderly and daytime populations (due to overcrowding risk).
The results of the study show that less than half of narrow sidewalks are in prioritized areas (21%); only 6% (169km) are of the highest priority level, i.e. are located in areas with higher than average ratios of both vulnerable populations identified in the course of the study.
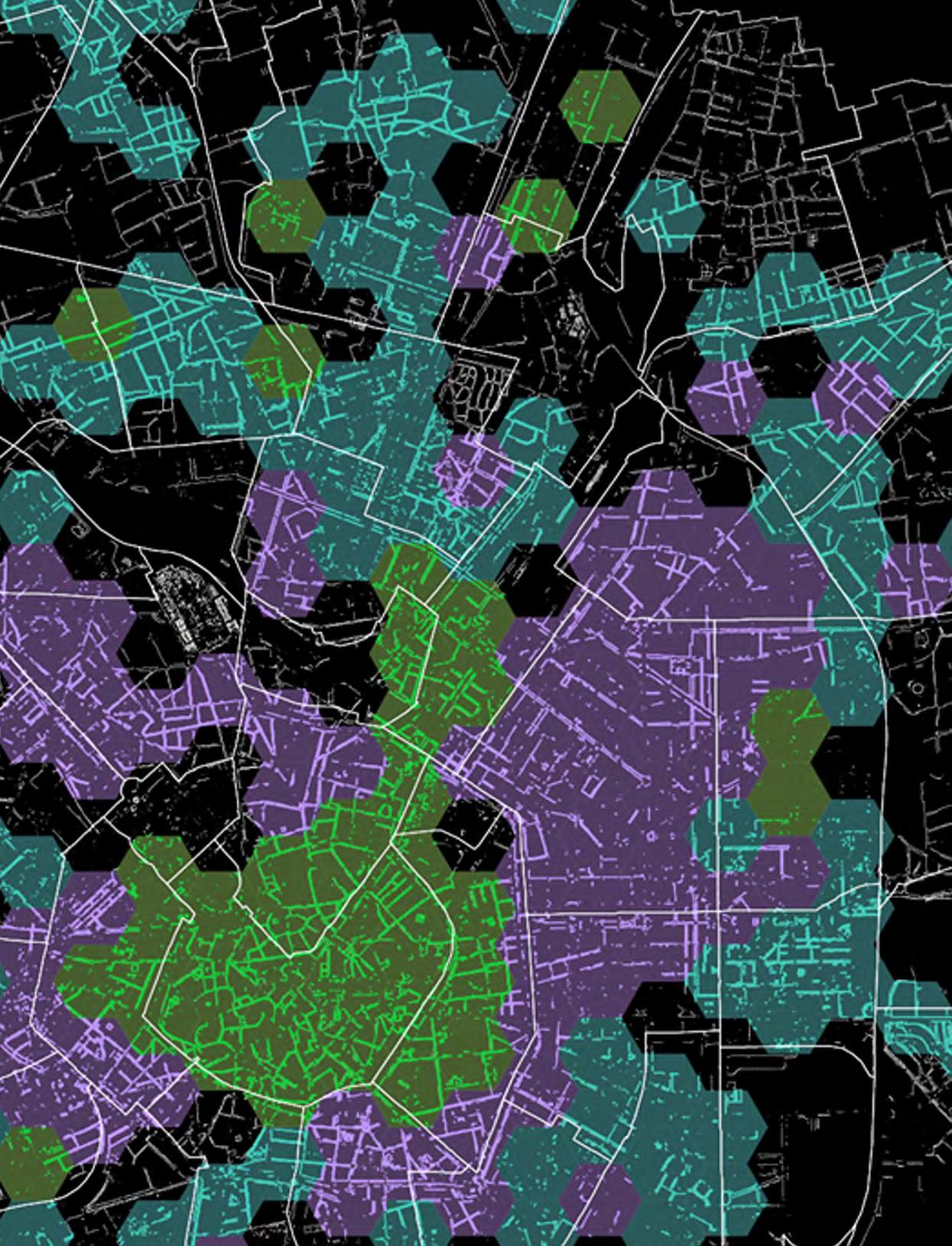
17
As previously discussed in the Milan Sidewalks Map article, the risks associated with inadequate sidewalk conditions are various. In the context of a pandemic, there is the additional risk of contagion resulting from overcrowding in areas where the sidewalks are not wide enough to accommodate pedestrian demand. In such cases, fears of contagion may prompt pedestrians to walk on the roadspace instead of the sidewalk, leading to significant safety and accident risks. In a context where nearly half of all sidewalks are considered too narrow for comfortable pedestrian movements, further criteria are brought into the analysis to determine a practical approach for prioritizing intervention areas. Focusing on population vulnerability, two main factors were considered in this study in order to define critical zones:
1. Elderly populations: defined as the density of the population over the age of 70 representing the slice of the population found to be most at risk of contracting the coronavirus. In Italy, 89% of the patients who died and were positive with Covid-19 fell under the age group of Over 70 (Istituto Nazionale di Statistica & Istituto Superiore di Sanità, 2020);
Residents Employees populationDaytime Retail PublicStopsTransport Vehicularflows Elderly Narrow sidewalks Sidewalks Figure 1 Overview of analyses Criteria of Analysis
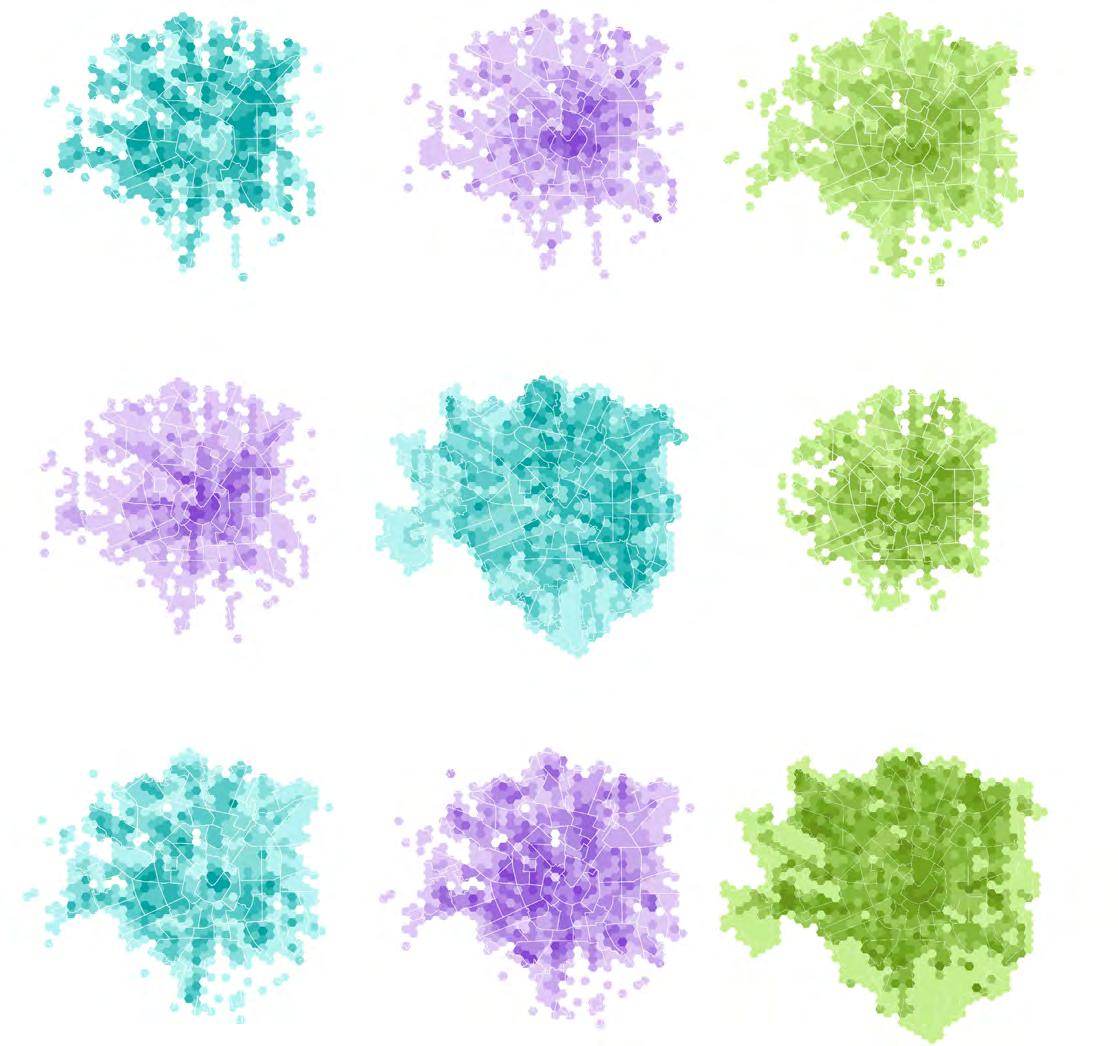
18
Figure 3 Comparison of density of vulnerable populations with the level of unsafe sidewalks by neighborhood Vulnerable Populations and Sidewalk Safety
2. Daily population: this term refers to the daily active population in a specific area including residents, students and employees who travel to places of workplace in each respective area. This factor essentially identifies the densest and most crowded areas during the day. The areas in which interventions are prioritzed will thus be those with greater Over 70 population density, areas with greater density of daily populations, or both (see Figure 2).
Elderly UnsafeDaytimepopulationpopulationsidewalks(<2.4m) some interventions shold be put in placeFigure 2 Mapping areas with high densities of vulnerable populations
The graph presented in Figure 3 is the result of the intersection of three pieces of information: the distribution of unsafe sidewalks, the density of the elderly population and the daily population by neighbourhood. This analysis allows us to observe that 65% of sidewalks that are narrower than 2.4 meters are concentrated where the majority of vulnerable populations are present (to be exact, where 80% of the population over 70; and 80% of the daily population are present).
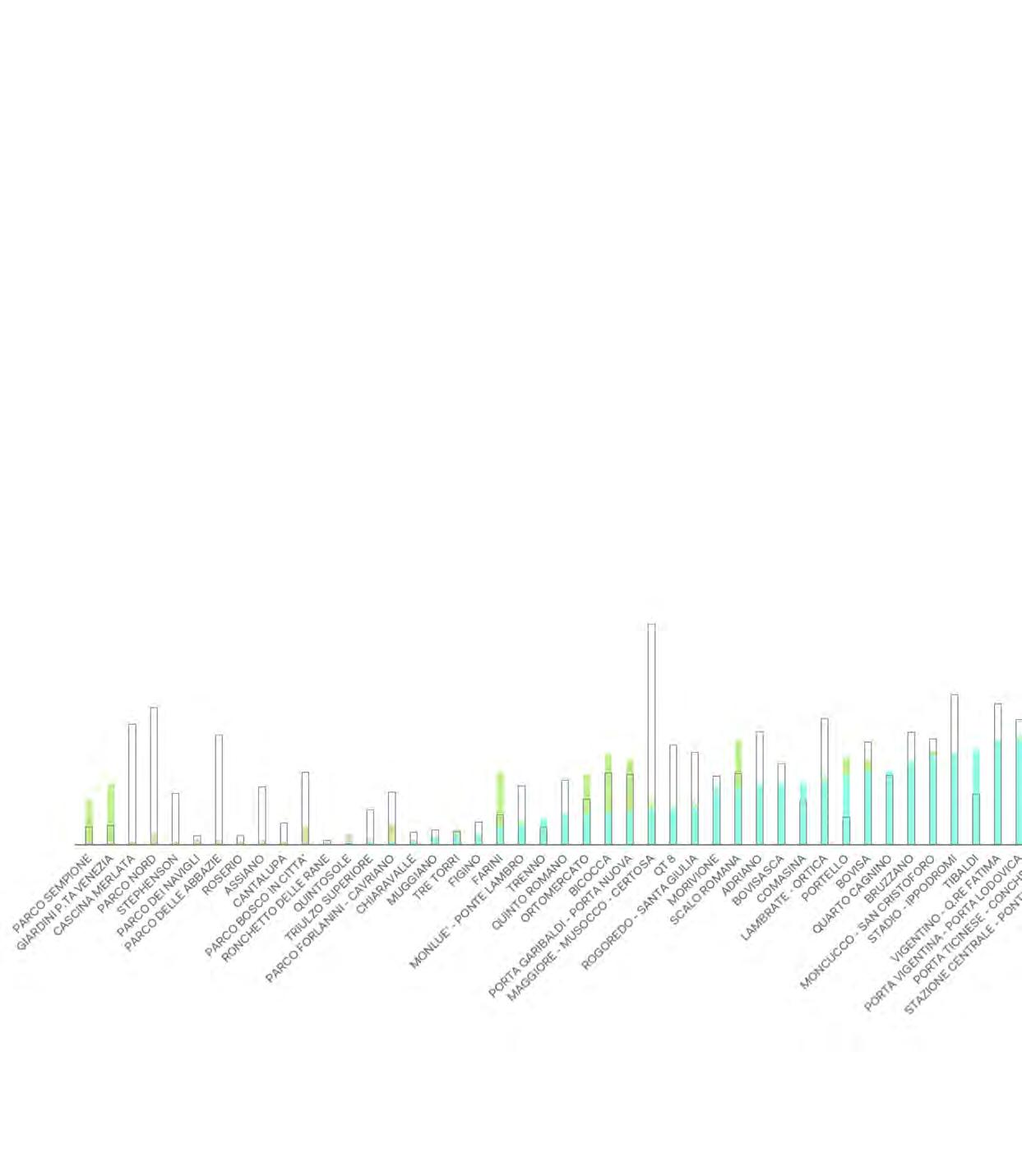
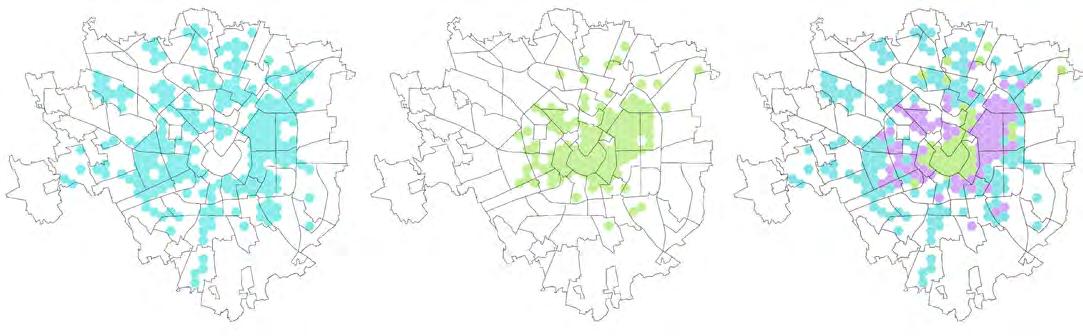
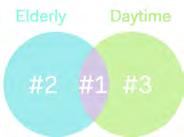
1,374 km of unsafe sidewalks in Milan. 65% of them are concentrated where: 80% of elderly population live; 75% of daily population concentrate. 19
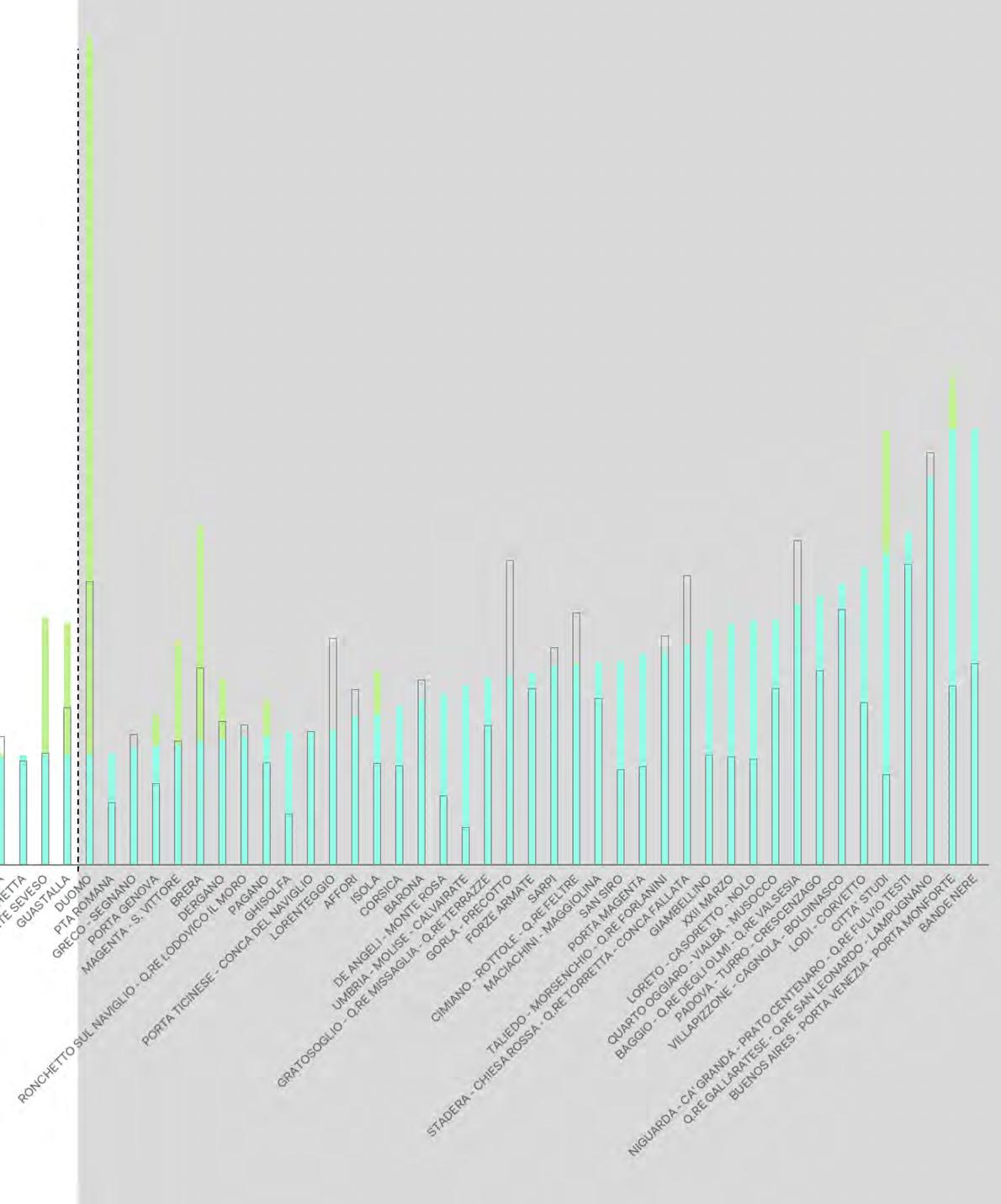
Narrow sidewalks / Daytime population Figure 4 Neighborhood distribution by vulnerable population group and unsafe sidewalk density Identification of Key Districts 20
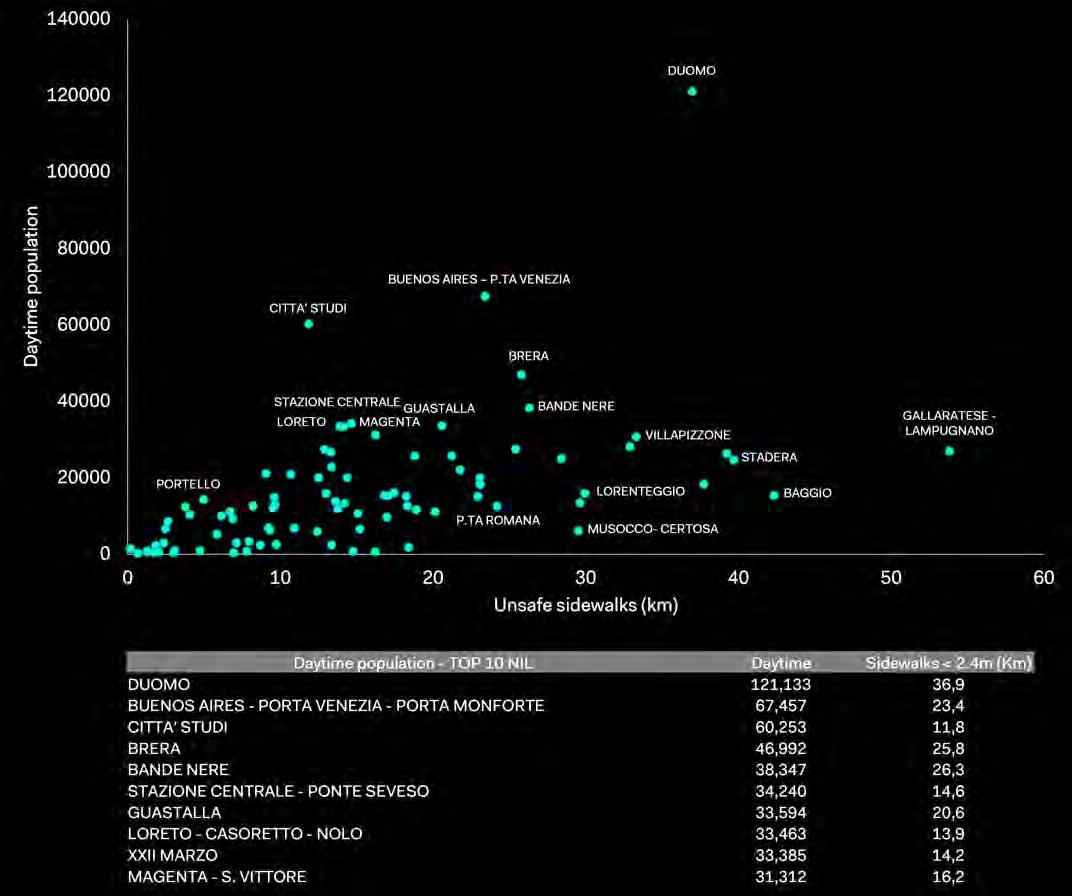
Narrow sidewalks / Elderly population 21
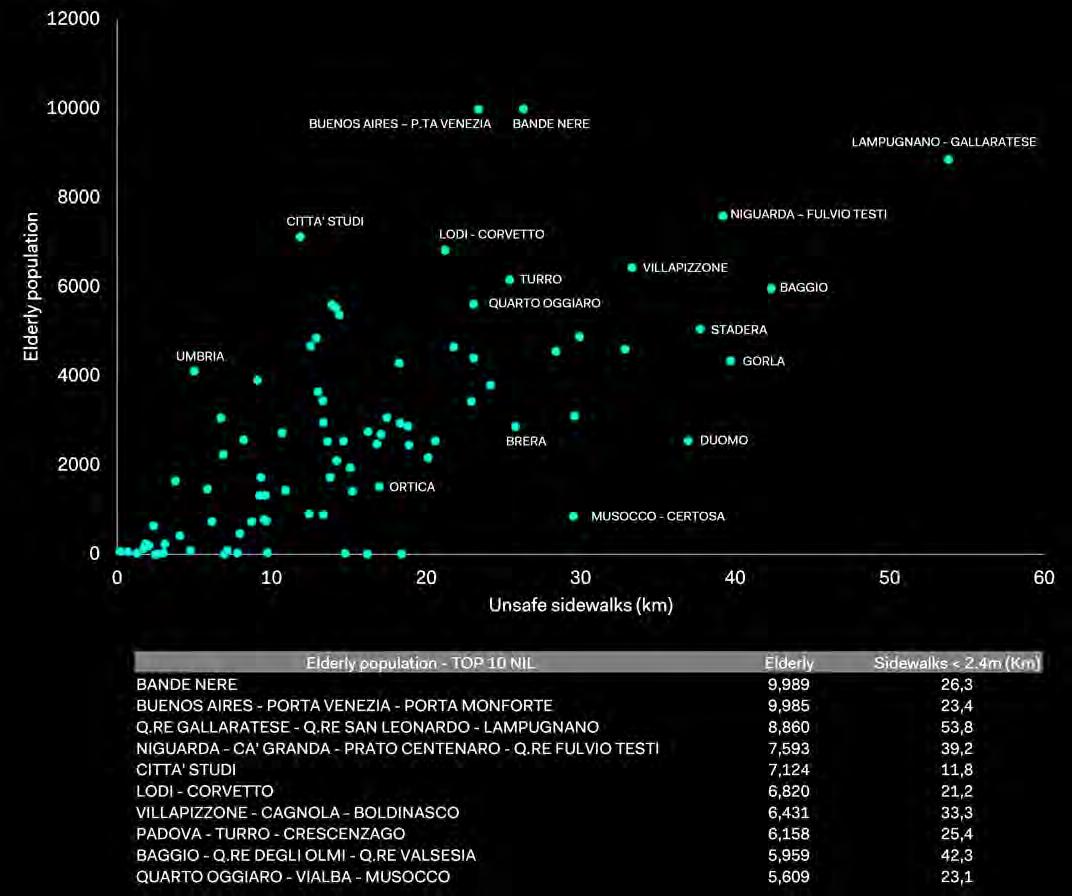
Priority 1: sidewalks located in very dense areas where there is a high density of both elderly and daily populations. Among these areas, the districts between the first and second ring roads stand out, such as Loreto, Porta Venezia, XXII Marzo, Porta Romana, Porta Genova, Sarpi; Priority 2: sidewalks in areas where there is a higher density of elderly populations than the city average. These include some historic and mainly residential neighborhoods such as: Gallaratese, Villapizzone, Musocco, Niguarda/ Affori, Maciachini, Lodi, Gratosoglio, Chiesa Rossa, Giambellino and Bande Nere; Priority 3: sidewalks in areas where there is a high presence of daily populations. Among these, areas that are particularly active during the day stand out, such as: Duomo, Città Studi and Porta Nuova.
22
As mentioned, these areas are generally clustered between the first and second rings of Milan, presenting an opportunity for targeted upgrading at the neighborhood level. To that end, tactical urbanism and long-term solutions may be explored at a more granular level to allow for the most effective transition towards safe and comfortable walking environments on the long run.
UnsplashonMyznikEgorbyPhoto
By overlapping areas with a high density of vulnerable populations and unfit walking conditions, intervention priority areas are mapped along three priority levels (see Figure 5):
Priority of Interventions
Of these, only a third (6% of all sidewalks or 169 km) was found to be of the highest priority, corresponding to areas where the most vulnerable populations reside more frequently than the rest of the city.
Following the criteria for high-risk areas for walking, 21% of the total sidewalk volume across the city have been found to be of some level of priority for intervention - less than half of sidewalks narrower than 2.4m (see Figure 6).
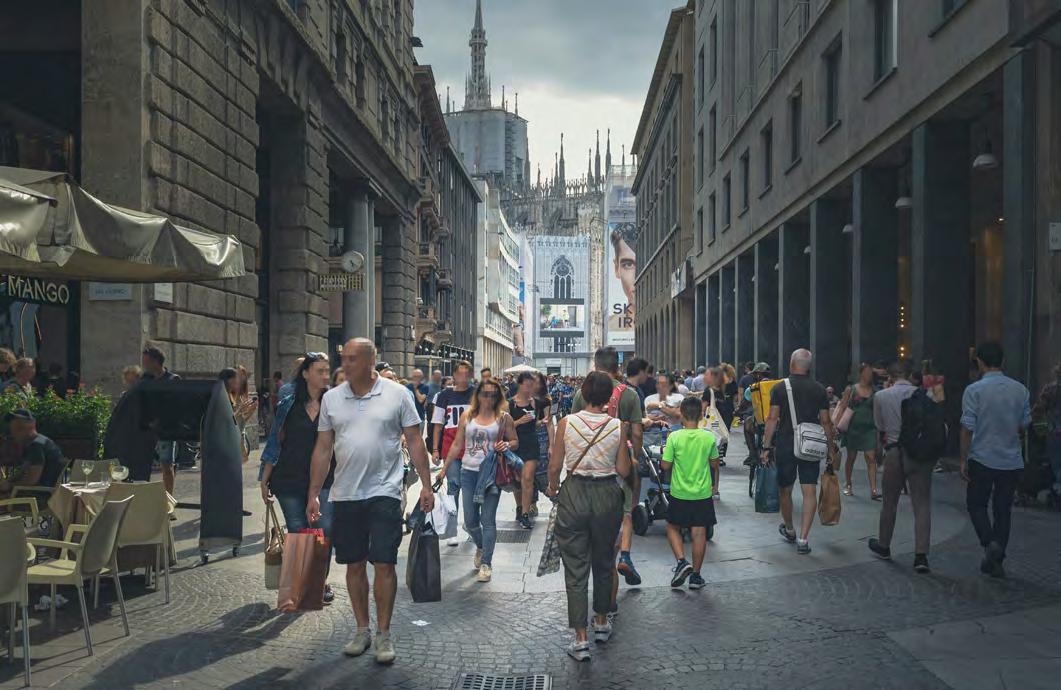
Narrow sidewalks with Priority 1 (elderly and daily pop) 169 km Narrow sidewalks with Priority 2 (elderly populations) 376 km Narrow sidewalks with Priority 3 (daily populations) 100 km Narrow sidewalks with no Priority 729 km Remaining sidewalks 1679 km Total sidewalks 3053 km Priority 1 Priority 2 Priority 3 Figure 6 Share of sidewalks by priority level of intervention Figure 5 Map of prioritized areas of intervention by priority level 23
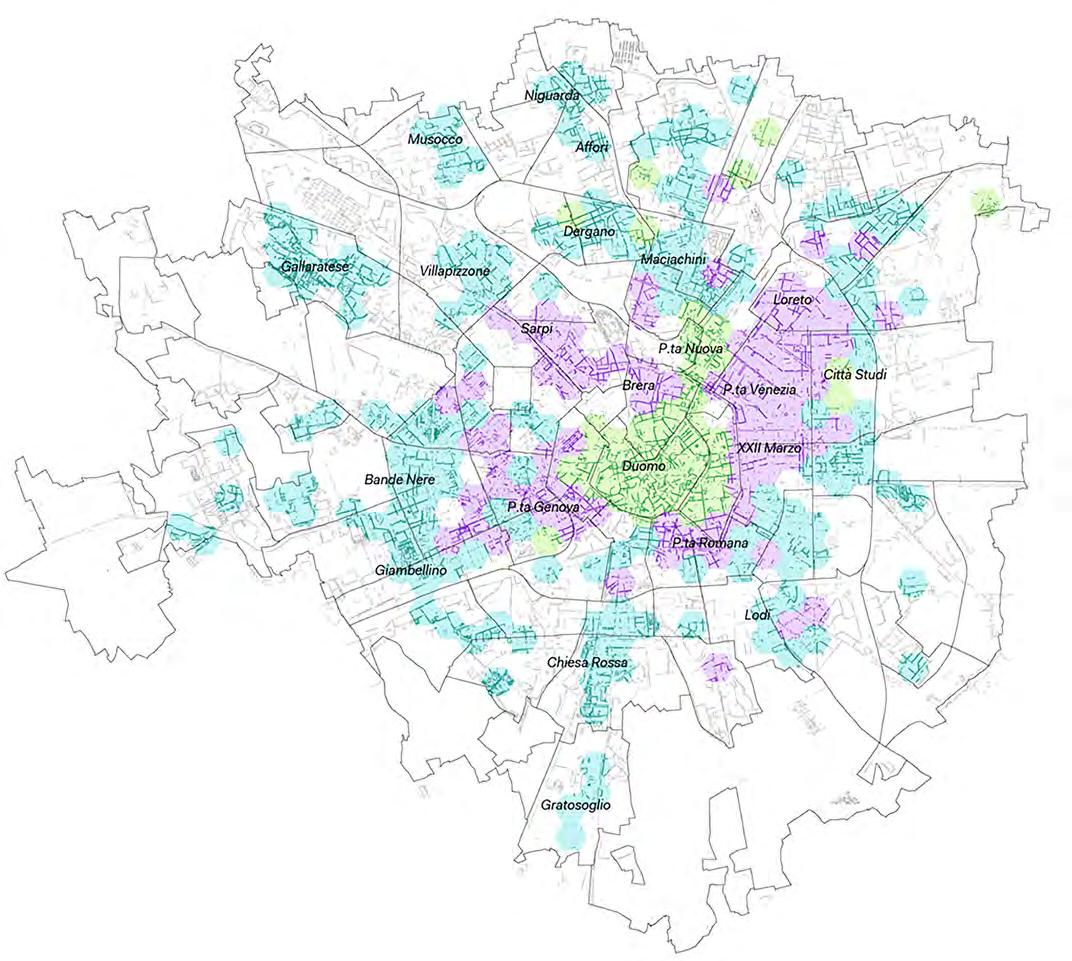
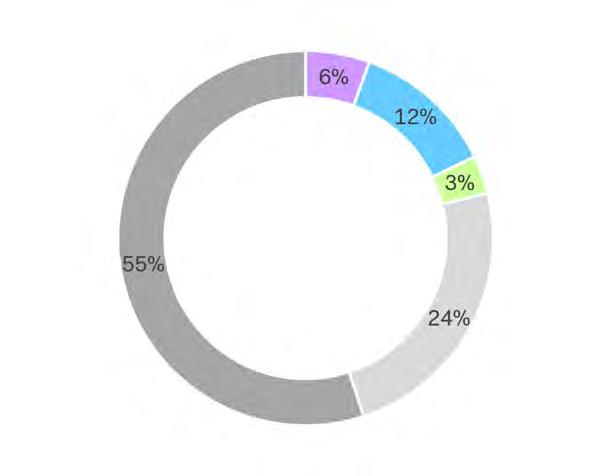
The relevance of computer-based pedestrian crowd simulations has become crucial in transport planning considering the unprecedented effects of the Covid-19 pandemic on urban mobility. However, there is still a lack of knowledge regarding the impact of social distancing on crowding, queuing, route choice and other pedestrian crowd phenomena.
The study presented herein applies the Social Force Model of the pedestrian simulation platform PTV Viswalk ® to investigate the effects of disruption of social distancing on pedestrian dynamics. First, a descriptive set of metrics and parameters is applied to calibrate interpersonal distances among pedestrians. Then, the plausibility of the proposed Social Distancing Model is evaluated against the socalled fundamental diagram to calibrate pedestrian Volume-Delay Functions. Finally, the proposed model is integrated into the PTV Visum ® simulation platform to evaluate the effect of social distancing on largescale pedestrian route choice. To do so, a macroscopic static model of the city of Venice was developed to test the effectiveness of alternative crowd management Resultsstrategies.about the historic center of Venice suggest that a significant reduction in the length of network with high density values can be achieved by finding a new user equilibrium, in which flows distribute among a wider set of paths. This is linked to the ability to set and validate crowd management strategies in advance, through transport modeling and well-calibrated monitoring programs.
How SimulationModelingAffectedCovid-19Pedestrianand 24
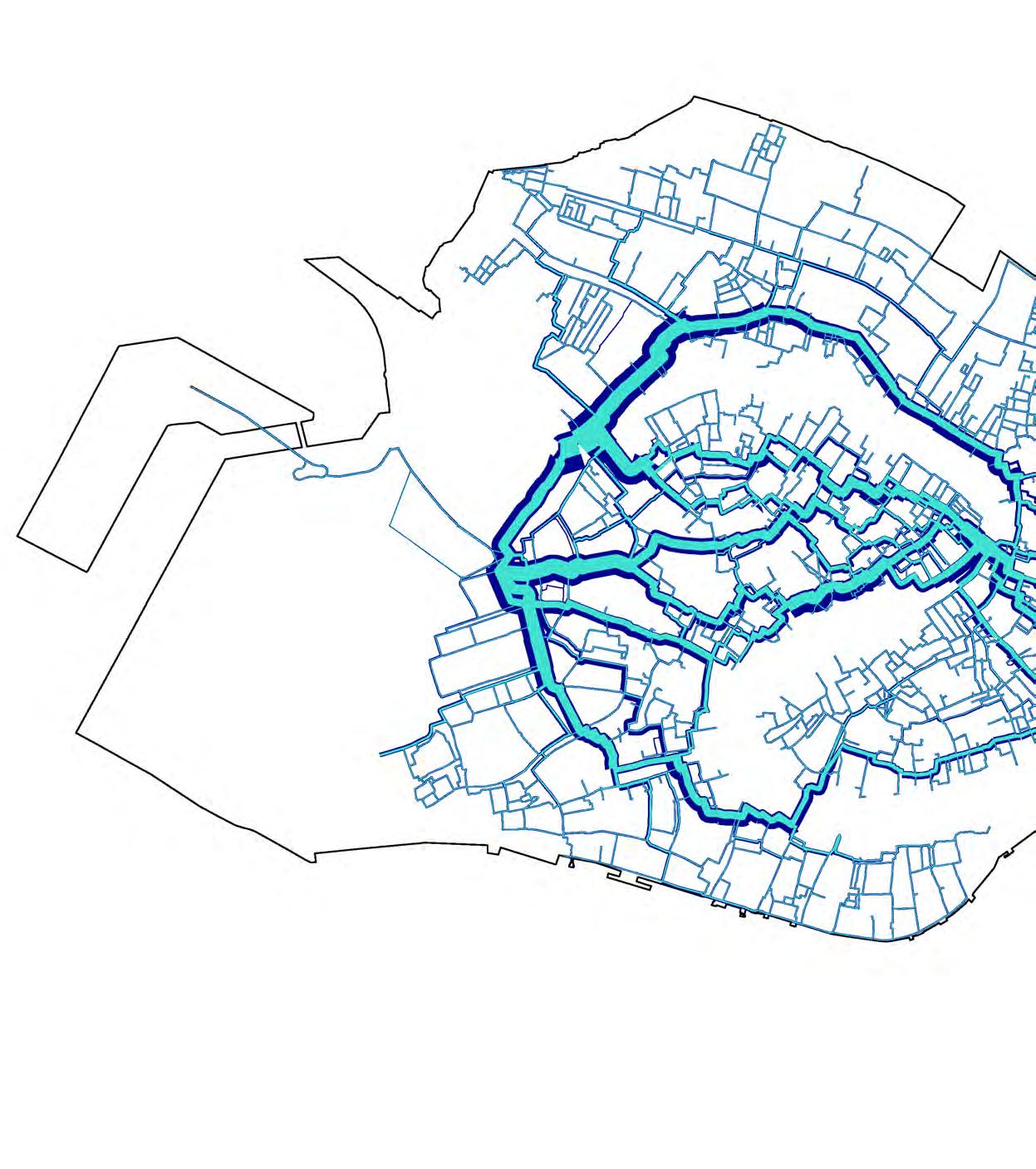
This research approaches the problem of pedestrian flow assignment under social distancing in a multilevel scale. First, a microscopic pedestrian model was developed to evaluate flow conditions at different demand levels. The microscopic model evaluated both BAU (Business-As-Usual) and SD (Social Distancing) pedestrian flow conditions by means of the Social Force Model (SFM) (Helbing and Molnar, 1995). By measuring flow, density and speed under different demand levels, the fundamental diagrams for both scenarios were built (BAU and SD). Then, Pedestrian Volume-Delay Functions (pVDFs) were calibrated by estimating the parameters that better fit the fundamental diagrams. Such functions were implemented in a large-scale macroscopic static pedestrian assignment model to evaluate the effect of social distancing on route choice.
UnsplashonSchwarzClaudiobyPhoto
25
Introduction Pedestrian movements are one of the most complicated dimensions when approaching transport planning, since they are strongly based on people behavior and personal perceptions of environment, social distancing and reasons at the basis of the trip. The use of computerbased systems for the simulation of pedestrian dynamics (e.g., Legion ®, MassMotion ®, PTV Viswalk ®, etc.) is a consolidated and successful domain, thanks to its scientific relevance and its capability to provide practical solutions for supporting transport planners in managing crowded facilities (Gorrini et al., 2018). The relevance of computer-based simulations in transport planning has become even more crucial considering the unprecedented effects of the Covid-19 pandemic on pedestrian dynamics, and urban mobility more in general. The activities of transport planners and decision makers have been projected ahead towards promoting pedestrian mobility and walkability in order to reduce risk of contagion associated with high contact and crowding conditions.
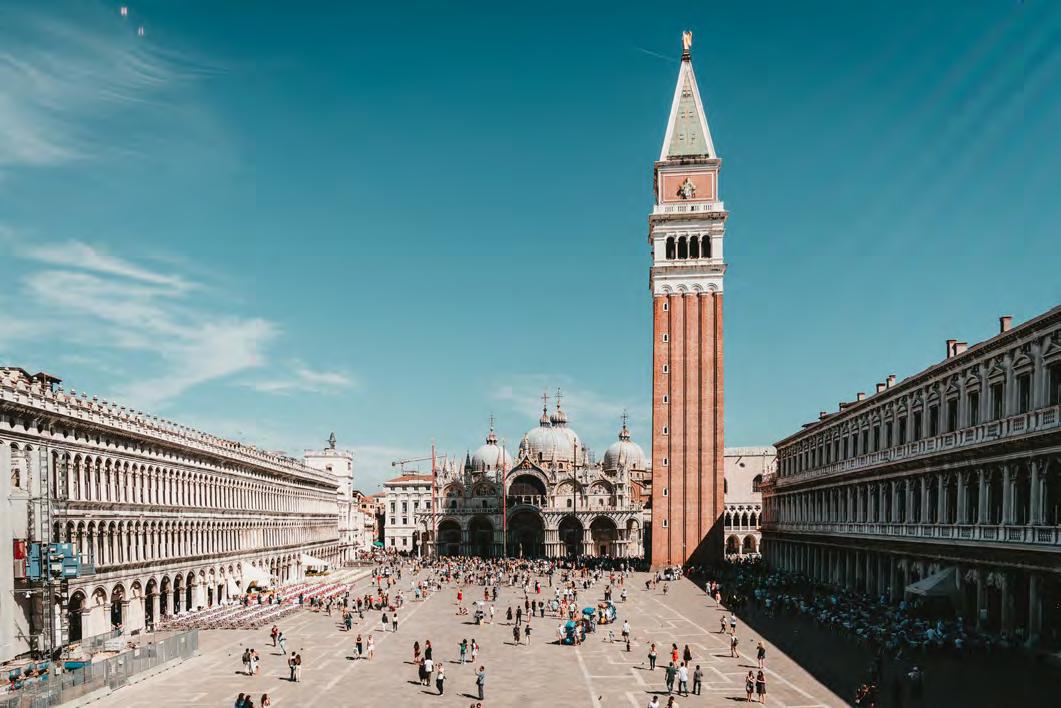
Social Force Model (BAU) Social Distancing Model (SD) Figure 1 Simulation results achieved through the Social Force Model and the proposed Social Distancing Model
Figure 2 Simulation set-up (above) and pedestrian density measurement (below)
Measurement area Loading Measurementareaarea
Microscopic Model A modification to the parameters of the default social force model implemented in the pedestrian simulation platform PTV Viswalk ® was proposed in order to simulate social-distancing conditions. The study focused on calibrating the dynamic regulation of interpersonal distances among pedestrians (i.e., social isotropic parameters related to repulsive force), to avoid conditions of inappropriate proximity and spatial restriction due to high density situations. The calibration process consisted of the iterative variation of the Social Force Model parameters until reaching a flow condition in which pedestrians would respect the majority of the time a predefined maximum density threshold (1 ped/ sqm), with a regular behavior and avoiding back and forth oscillations of pedestrians when approaching others (Kretz, 2015). This predefined maximum density is a proxy for a minimum interpersonal distance of 1 m, considering squared cells. Figure 1 shows a comparison of the time duration in which the density threshold is exceeded for a given input flow for the standard Social Force Model (BAU) and the Social-Distancing Model (SDM).
The calibrated SFM under social distancing (SD), and the default model (Business as Usual: BAU), were used to build the pedestrian fundamental diagrams for both conditions. The straight corridor set-up did not allow to observe the complete domain of densities of the fundamental diagram, since the flow input was limited by the release (loading) capacity. To overcome this limit, a closed loop set-up was implemented, as done by Seyfried et al. (2005), so it was possible to observe also hypercritical flow conditions (i.e., densities exceeding the density-at-capacity).
The following image shows the simulation set-up (see Figure 2).
26
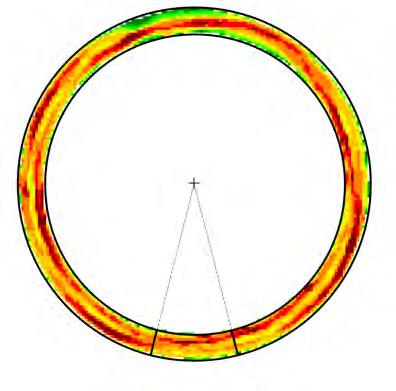
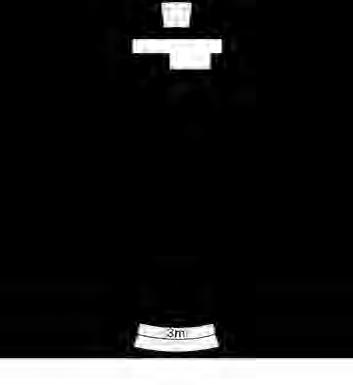
27
Functions From
Diameter = 22m Diameter = 12m Diameter = 4m Figure 3 SD Model Fundamental Diagrams: Flow-Density (a) and Speed-Density (b) 15.010.020.025.05.00.0[ped/m/min]FlowPedestrian 0.0 0.1 0.2 0.3 0.4 0.5 0.6 0.7 0.8 0.9 1.0 1.1 Pedestrian Density [ped/sqm] 0.60.20.41.01.20.0[m/s]Speed 0.0 0.1 0.2 0.3 0.4 0.5 0.6 0.7 0.8 0.9 1.0 1.1 Pedestrian Density [ped/sqm] 0.8 (a) Flow-Density (b) Speed-Density
Fundamental Diagrams and Volume-Delay the results of the microscopic model, the fundamental diagrams for the BAU Model and the SD Model were built (see Figure 3). A significant reduction in the capacity is observed under social-distancing conditions, going down from 63 ped/m/min in the BAU model to 21 ped/m/min in the SD model. The maximum density falls down from 4 ped/sqm to 1 ped/sqm, which was indeed the maximum density used as input for the calibration of the social-force model parameters. In order to apply the findings from the microscopic simulation model into a large-scale macroscopic pedestrian assignment model, both the hypo- and hypercritical parts of the fundamental diagrams were used to calibrate Pedestrian Volume-Delay Functions for BAU and SD models, through the least-squares method. In this work the standard BPR (Bureau of Public Roads, 1946) function formulation was used.
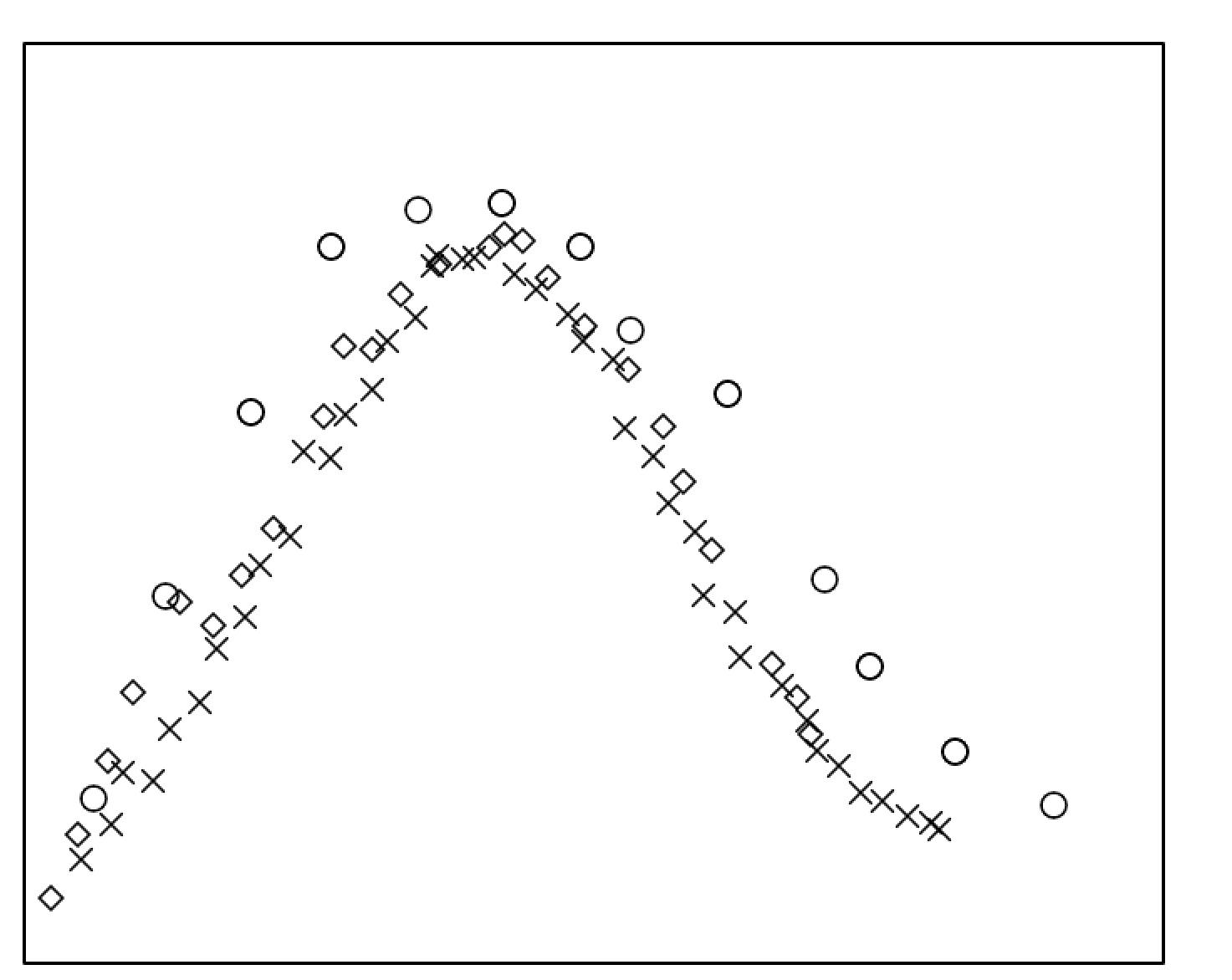
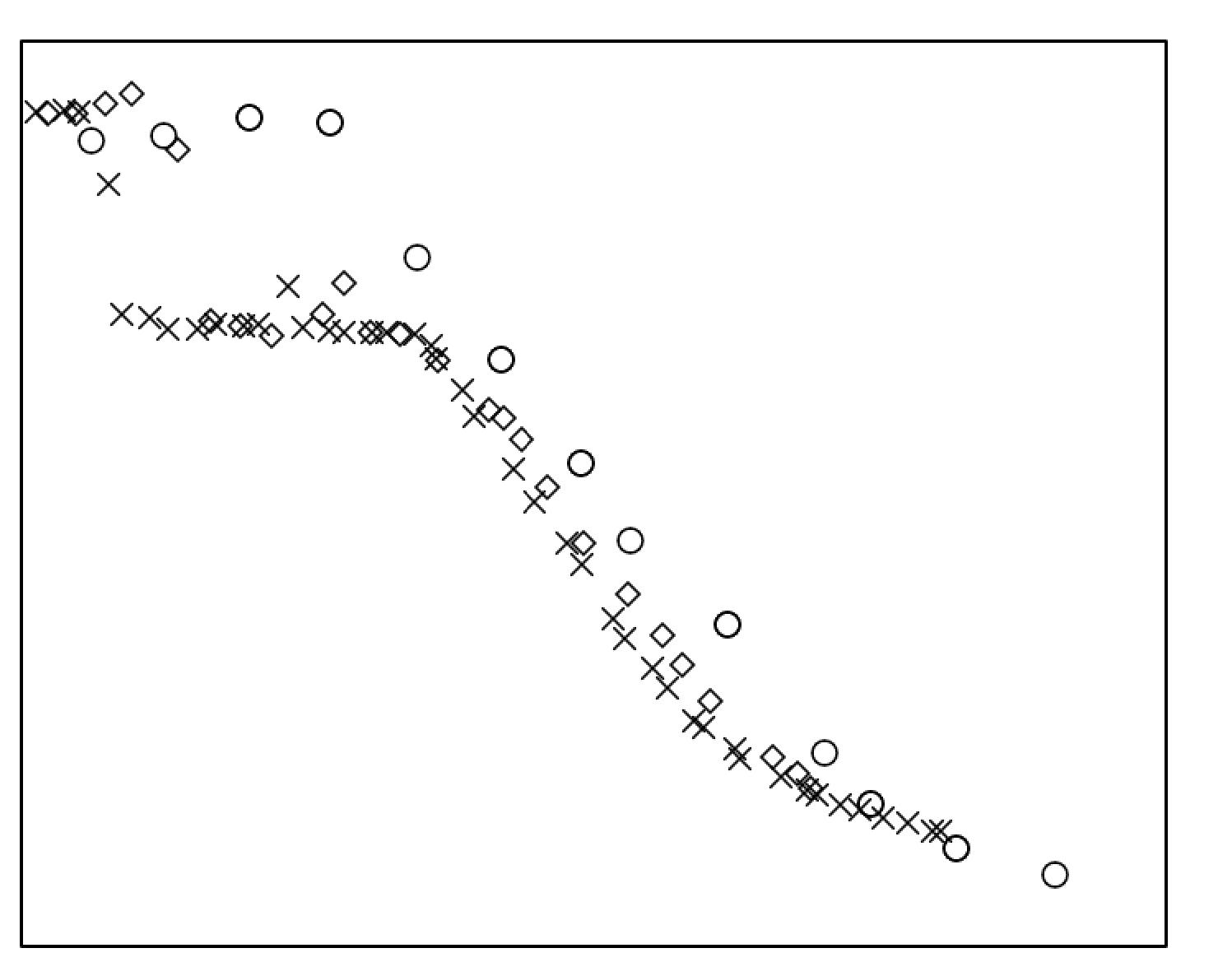
Pedestrian Assignment [ped/h] FLOW_SDFLOW_BAU Assignment flow comparison SD vs. BAU [ped/h] Increased Decreasedflowflow (a) Link Volume SD vs. BAU (b) Volume Difference SD vs. BAU Figure 4 Link Volume (a) and Volume Difference SD vs. BAU (b) 28
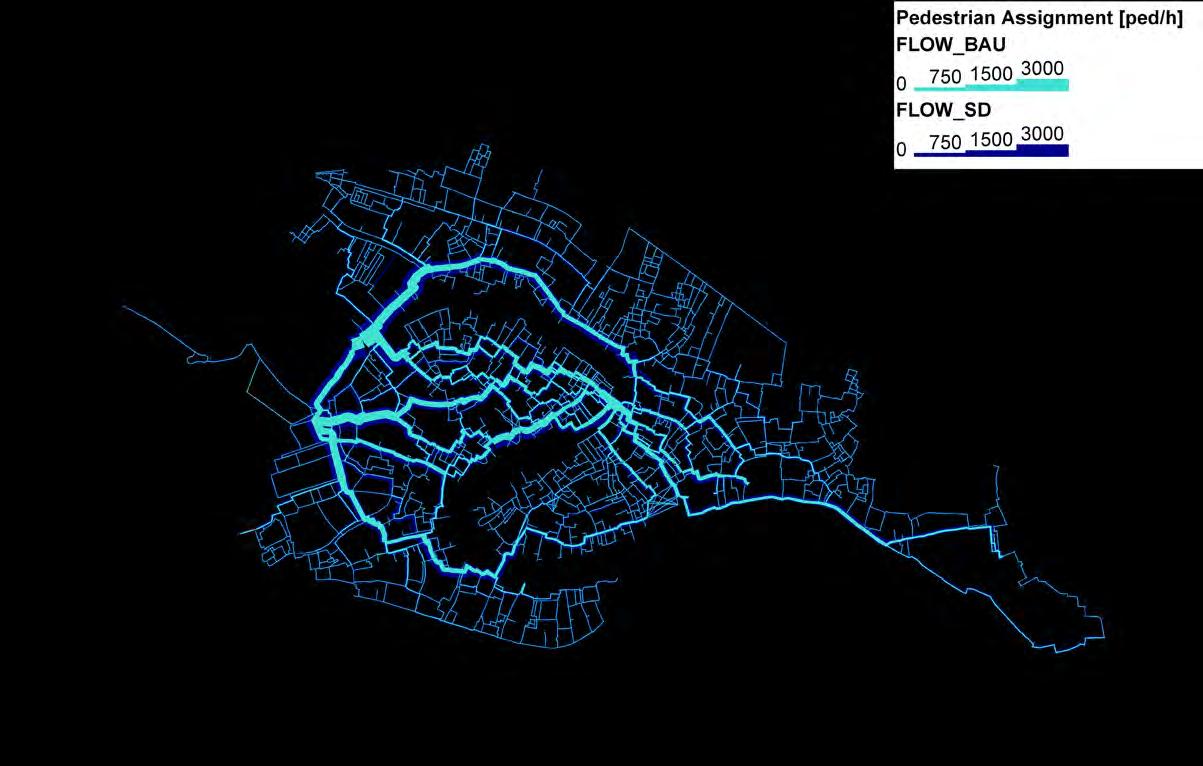
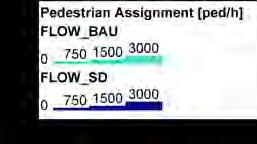
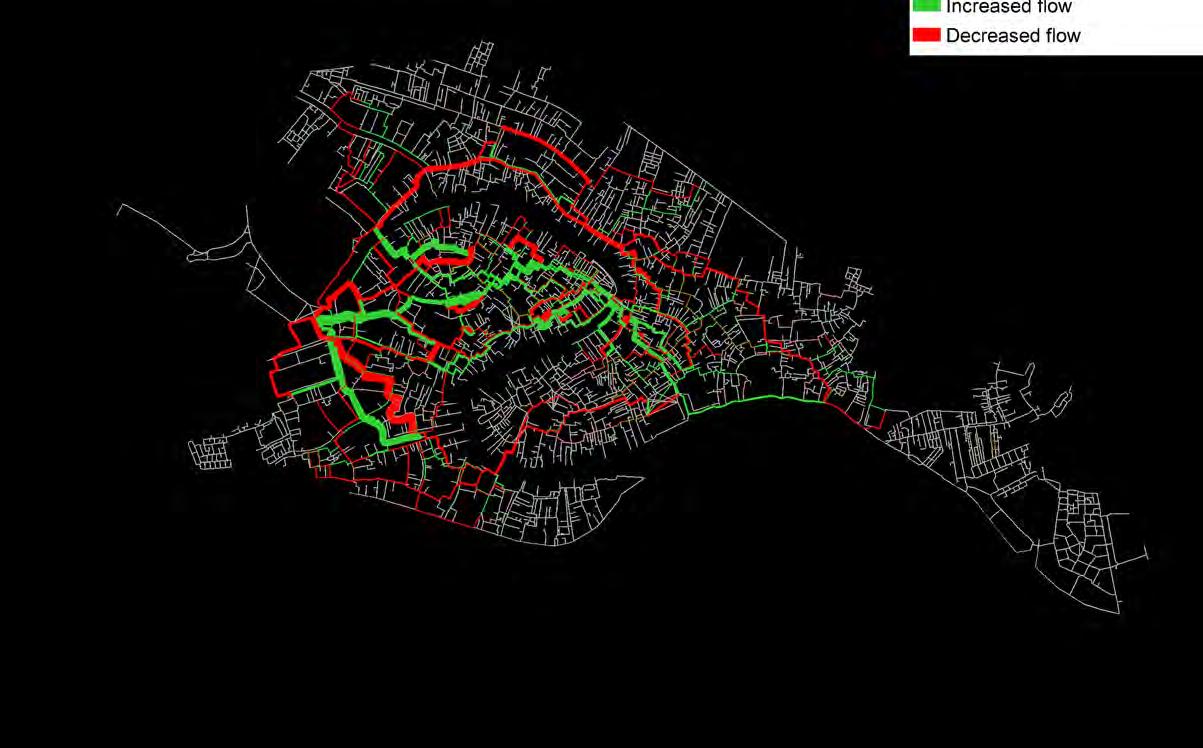
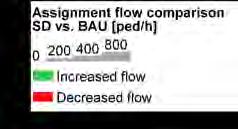
Case Study
29
Concluding Remarks
The calibrated Pedestrian Volume-Delay Functions were then used in a large-scale macroscopic pedestrian assignment developed in the software PTV Visum ® (PTV Group, 2021). The chosen case study was the City of Venice, Italy. The main island of the Venetian Lagoon is certainly a suitable example of a dense urban pedestrian network to apply and analyze people flow distribution across a complex urban context through the use of User-Equilibrium Pedestrian Assignment. The model implements an OD matrix including both external trips (between the island and the dry land) and internal trips (Origin and Destination within the island) for the morning peak hour of an average working day. The total estimated pedestrian OD matrix consists of 15,700 trips hourly.
The results of this research activity have been published in the following scientific Journals: Deponte, D., Fossa, G., Gorrini, A. (2020). Shaping space for ever-changing mobility. Covid19 lesson learned from Milan and its region. TeMA – Journal of Land Use, Mobility and Environment, 133-149. https://doi.org/10.6092/1970Espitia,9870/6857E.,Gorrini, A., Vacca, A., Deponte, D., Sarvi, M. (2022). How Covid-19 is Affecting Pedestrian Modelling and Simulation: The Case of Venice. Transportation Research Record. https://doi.org/10.1177/03611981221088224
Figure 4 (a) shows the pedestrian assignment flows in the network for both the BAU and SD models, while Figure 4 (b) shows volume differences in the SD assignment compared to the BAU model. Significant volume differences are observed in the network, not only at link-level (localized) but also at paths-level (large scale). Looking at the large-scale, in the SD assignment the pedestrian flows increase along the northern and southern sectors of the island, while the flow along the central connection (The Rialto Bridge) decreases significantly (-15%). This is the result of the redistribution of flows coming from Piazzale Roma and the Railway Station and going to the eastern part of the island. Detouring through the Cannaregio district (north) or through Dorsoduro district (south), allows to reach a new equilibrium by maintaining lower densities.
UnsplashonCarnelosLucreziabyPhoto
Beside the ability to support the definition and the operational assessment of effective responsive strategies to face the impact of the Covid-19 pandemic on urban mobility, the proposed analytical approach demonstrates the importance of pedestrian modeling and simulation. The use of advanced and robust pedestrian macroscopic models capable of capturing and replicating a larger variety of pedestrian dynamics allows to enrich the definition of innovative city walking plans with further valuable and evidence-based insights. In fact, the main goal of state-of-the-art walking plans, which consist of operational and actual implementation programs, is to understand, analyze, foresee and manage people movements in and around cities, allowing people to get access to urban opportunities, meet, and move safely and easily, by ensuring permeability, access for all abilities and planning for future growth.
We thank Prof. Giovanna Fossa (Politecnico di Milano) and Prof. Majid Sarvi (University of Melbourne, VIC, Australia) for their contribution. We also thank the team of PTV Group for their collaboration and for sharing information about how to calibrate the model of the Viswalk platform.
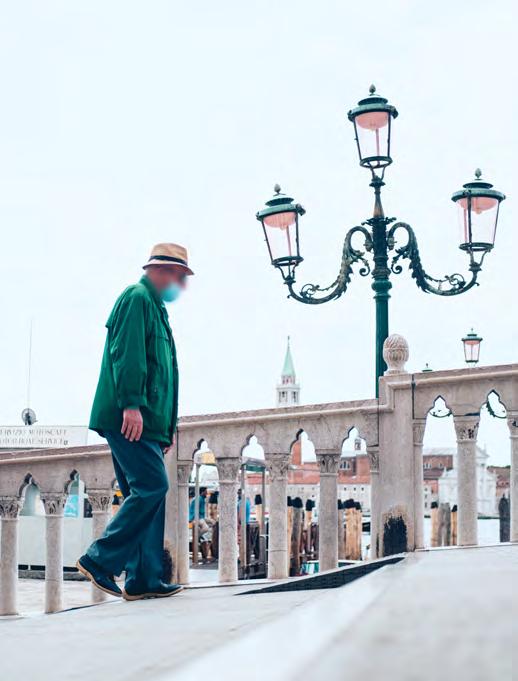
Chapter 2 LIVING LOCAL 30
The modelorneighbourhood’‘complete’15-minutecity’ispromoted as a framework for recovery from the COVID-19 pandemic situation. ofshapingarehomeaccessibility.spatialforhavelockdownsCityworldwideprovidedalensunderstandingtheinequalityofNear-environmentsthuspivotalinexperiencesurbanliving,as differences in service accessibility have profound effects on quality of urban life. 31
The movement restrictions experienced during the COVID-19 pandemic have challenged us to reconsider the way we inhabit and plan our cities. The lockdown state in particular has prompted questions about the capacity of the neighborhood scale to fully support daily life. Localized living potential is important not only during emergency situations, but also for long-term sustainability goals to reduce carbon emissions by urban transport. This study presents a preliminary analysis of Milan's neighborhood potential to support walking as the primary mode to meet daily needs. The analysis relies on the Voronoi tessellation method as a preliminary methodology to gauge neighborhood potential from a supply-side perspective by partitioning the city into regions where the size of each region determines whether each neighborhood classifies as what we call a The“micro-center”.resultsofthe analysis show that more than half (54%) of the city by area is made up of neighborhoods with low service proximity for pedestrian access. At the same time, it shows that these are areas where only 27% of the population of Milan reside. Low-performing neighborhoods generally tend to fall along the outer rings of the city; underscoring the importance of addressing peripheral urban neighborhoods in future urban agendas.
Micro-CentersMapping
in Milan 32
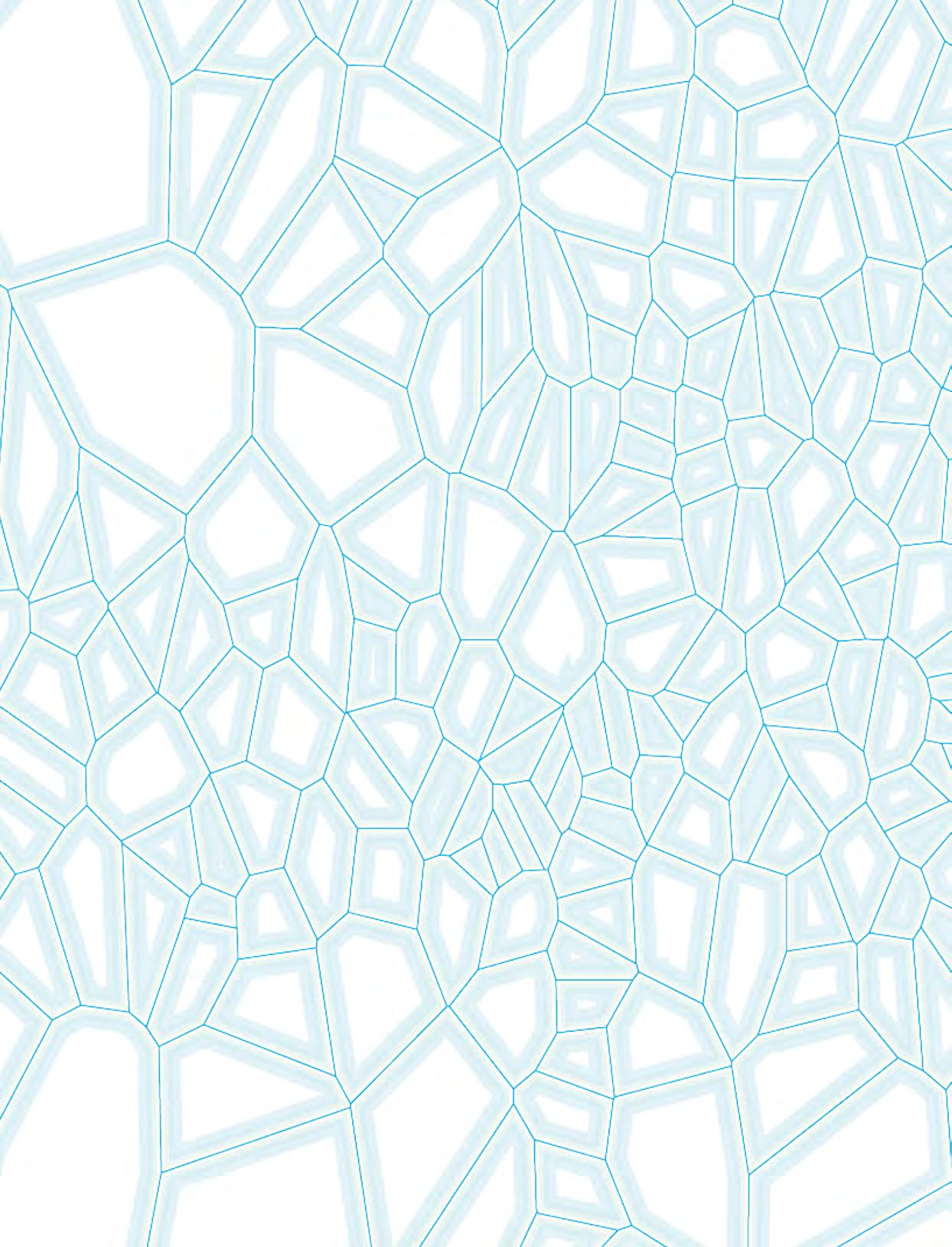
The "15-minute City" concept
The Role of the Municipality
The "15-minute City" is a term given to the vision proposed by urban academic Carlos Moreno and adopted by the city of Paris, whereby every resident is envisioned to be able to reach a number of essential services on foot or by bicycle within 15 minutes from their home (Moreno, 2021). This concept rests on the idea of localized living, whereby residents could satisfy the bulk of their daily needs without the need for long motorized journeys. Across the globe, numerous cities have adopted similar models, "20-minute neighbourhood" plans were already underway in Portland and Detroit in the United States, Ottawa in Canada and Melbourne, Australia. More and more cities are joining the movement. For example, the developed Open Streets plan promoted by the Municipality of Milan for ‘Phase 2’ of post-lockdown containment makes reference to the same principles of the "15-minute City": promoting the neighborhood scale guaranteeing services proximity, density and diversity (Comune di Milano and AMAT Agenzia Mobilità Ambiente Territorio, 2020). This study draws its inspiration from the abovementioned concepts of the 15-minute City and serves as an analysis framework with the aim of identifying “local micro-centers” within Milan (see Figure 1). We define micro-centers as those pedestrian-friendly areas of the city where the compresence, proximity and service diversity is achieved to a degree to which it becomes fairly easy to fulfill common daily trips on foot. The article proposes a replicable methodology to evaluate the state of the art and therefore the potential of a city in providing adequately ‘local centers’ with dense availability of services to its residents.
The "15-minute City" concept is highly ambitious because the idea of favoring functionally mixed areas goes against years of urban planning ideologies where the goal was to do the opposite: separate residential areas from areas of work and leisure. Local diversification and neighborhood scale living, necessitated by the continuous expansion of cities, has the potential to improve citizens’ quality of life by reducing travel times and reliance on heavy modes of Fortransport.thisconcept to materialize, city administrations must assume a proactive role in defining the array of ‘essential services’ needed to fulfill local micro-centers. In Paris, an initial categorization of these services was conceived on the basis of daily citizens needs groups such as work, nutrition, health and so on. These macro-categories must then be further detailed by municipalities, professionals, researchers and citizens into more concrete lists of services. Once a list of services is defined, municipalities can identify the neediest neighborhoods as those in which density - and therefore, accessibility to local centers - is weakest. This step will then inform strategic intervention and organize priority-based action plans for urban neighborhoods.
1
Proximity, Density and Diversity: Local Centers to Support Living at Neighborhood-Scale
33
Figure
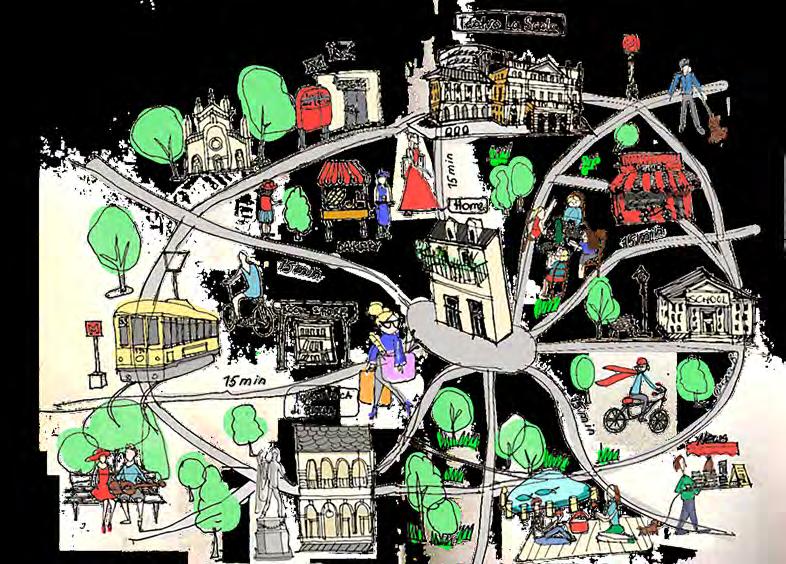
For each polygon, the maximum distance to travel to any particular point was then calculated to differentiate between different accessibility levels.
The Voronoi diagram is an aerial partitioning of space into "regions" based on the distance of the points from which they are generated with respect to all points on the plane.
34
The requirement to guarantee diversity was to verify, within the predefined pedestrian area, the presence of at least one service for each category considered. Starting from these identified local centers, the city was then mapped using the Voronoi tessellation method.
Food shop Bar Restaurant Metro Culture Education Park Shopping ATM Post service TransportPublic Newsstand Pharmacy Figure 2 List of defined essential service categories
In the absence of a comprehensive list of services to follow, the work was inspired by the documents disclosed by the city of Paris regarding the concept of “15 minutes City”. The same macro-categories (food, health, open spaces, sports, education, work, culture/leisure and mobility) were considered (see Figure 2).
Neighborhood Essential Services
The goal was to identify local micro-centers in Milan characterized by diversity, compresence and proximity of the aforementioned services. For this purpose, the analysis was set up considering the services with fewer presences (pharmacies) to which a 15-minute walk buffer was applied to guarantee the maximum proximity.
For each point of origin (in our case, for each local center identified) there is a corresponding "region” consisting of all points of services closest to that particular point.
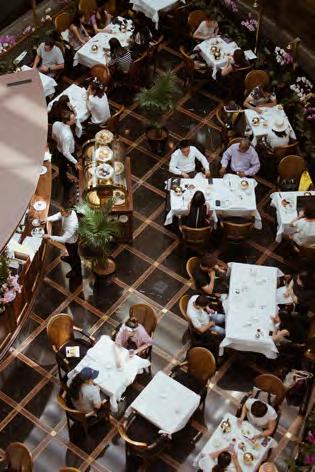
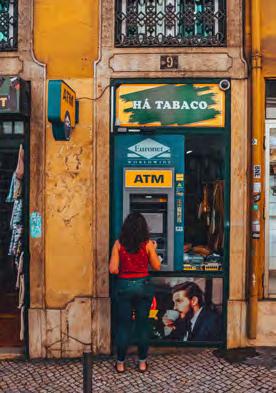
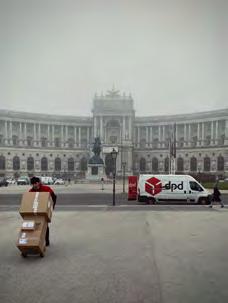
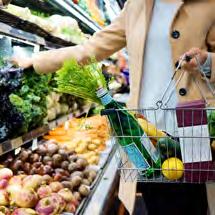
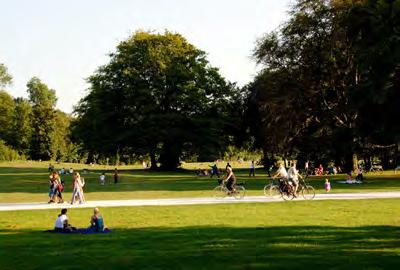
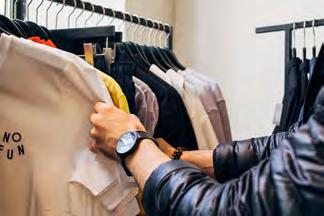
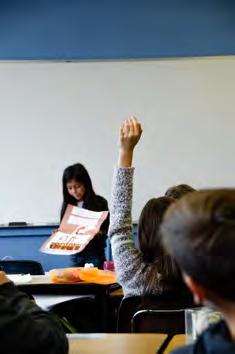
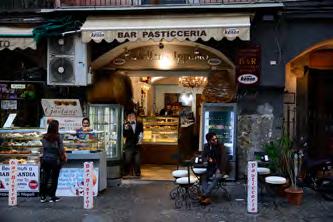
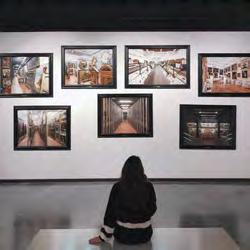
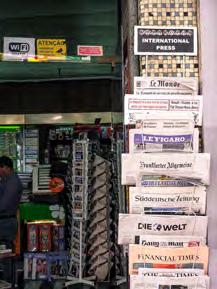
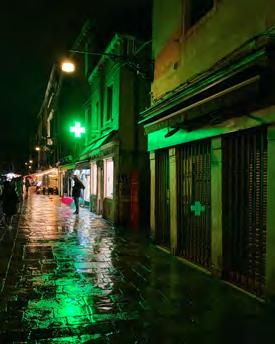
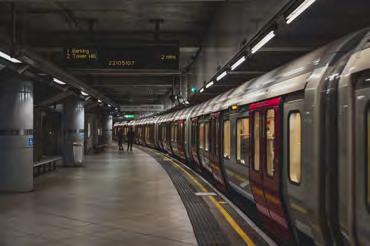
A quick reading of the mapping result shows a clear transitory pattern from inner city districts, where local centers are denser and therefore more accessible by foot, to peripheral areas, where there are few centralities and it takes longer than 15 minutes to reach these areas.
35
A number of central districts stand out for achieving diversity, proximity and density in services: these include Porta Garibaldi-Porta Nuova, Porta Venezia and Brera. These neighborhoods offer a model for local living that is further studied to gain insights into potential strategies to be adopted at city scale.
15 min. by walk 15 min. by bike 15 min. by car15 min. by walk 15 min. by bike Over 15 min.
Figure 3
The different scales of Milan's micro-centers De-constructing the City to Identify Its Local Centers
A mapping of the city using the Voronoi method described in the previous section can allow us to read the city as a tapestry of urban local centers of various accessibility levels (see Figure 3). All polygons highlighted in pink are of a dimension that allows reach to all service categories in less than 15 minutes on foot; those in orange in 15 minutes by bike; and light blue areas are those in which all essential services cannot be reached by either mode in the given timeframe.
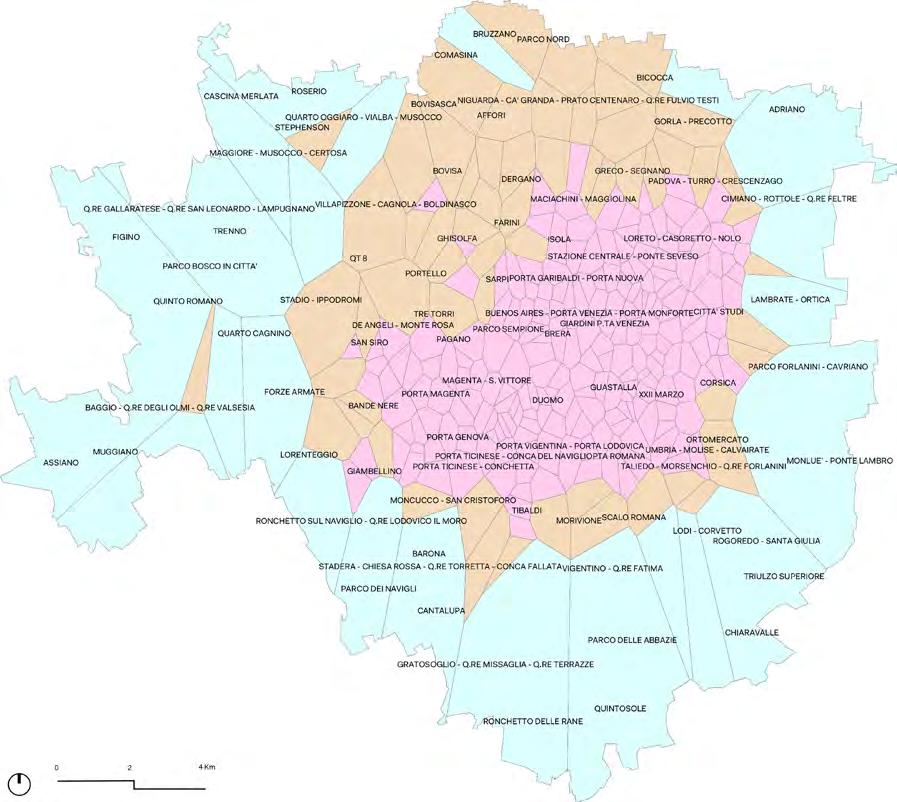
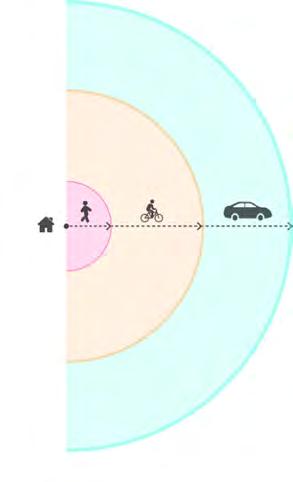
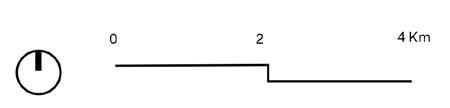
Figure
Figure
%
As shown in the mapping exercise in Figure 3, more than half of the surface area of the city (54%) is characterized by low-proximity and low density local centers. However, looking at these areas in terms of the distribution of residents provides deeper insight: in these low-proximity areas in which residents are required to travel more than 15 minutes to reach daily destinations, only 27% of the population reside (see Figure 4). In fact, the largest segment of the population (43%) lives in areas with high density and proximity of services accessible by foot. This data partly conveys the reality of high proximity being a function of high residential densities. It is not surprising that people have to travel shorter distances in high-density areas to reach the services they need. Careful consideration of the types of services available in underserved areas (light blue) is thus essential to understanding and compensating accessibility shortfalls.
If we take a closer look at the data, we find that an average micro-center in Milan is mainly composed of 330 shops (50% of the total), 170 bars/restaurants and 90 supermarkets (making up a 40% share). Unsurprisingly, fewer public transport stops are available in microcenters given the smaller surface area of these areas. However, it is notable that they are richer in cultural facilities than larger urban centers. It would be interesting to compare the service offer with the population distribution to understand per capita points of interest for each daily life category in micro-centers compared to more diffused urban nuclei.
Created by Nico Ilk from the Noun Project Areas within 15 min. by walk Areas within 15 min. by bike Areas over 15 min. Population in each area 4 Population distribution in urban centers of various scales Milan 5 Typical service distribution in urban centers of various scales in Milan
Local Centers and Population Distribution
Local Centers Composition In Figure 5, we analyze in more detail the typical distribution of services in the three broad accessibility categories of urban centers for daily living. We compare how services are subdivided in areas with high foot accessibility (micro-centers) in comparison with less dense and more sparse urban areas. The first thing that becomes clear is that high-performing areas, especially those that are the most central, are usually rich in shops. In fact, nearly 50% of the essential services available in areas with high foot accessibility fall under this category, in comparison with about 38% in centers with 15-minute cycling accessibility, and 33% in the lowest-performing areas. The second largest category of services is ‘eating’. In contrast, the distribution of this category is more homogenous across the 3 area types: food outlets and bars and restaurants collectively make up 40-45% of the service offering in all the areas analysed. On the other hand, large parks have a more notable presence in more peripheral, less walkable areas.
36

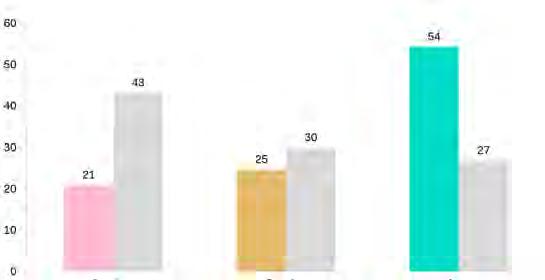
In Milan, the Territorial Government Plan (PGT) 2030 identifies, for example, a network of planned pedestrian areas where implementation of 'traffic calming' and urban care measures are designed to improve quality of life aspects, both from the environmental and social points of view (Comune di Milano, 2019). The network is conceived as a supporting structure of collective urban life in the center of neighborhoods, with the aim of facilitating the establishment and functioning of small businesses, crafts and creative activities and the connection of sociocultural and community services.
The study presented herein should be considered as an initial analysis to map functional micro-centers in the city. In this framework, a center is conceived as an atom of daily life activities whereby the smaller the atom, the more likely it is that residents in this area are able to complete their daily life activities on foot. By these measures, nearly half of the milanese population thereby lives in functional micro-centers. However, further analysis is required to understand the conditions under which people can reach and enjoy these destinations. In following articles, the level of walkability of these centers will be further analyzed, as well as the perceived level of crowding in public spaces across the city taking into account the diversity of public space types and the green area per capita in each respective area.
Efficient strategies to increase service accessibility in underserved areas can be derived from multiple initiatives. To increase network accessibility, the concept of the super-blocks in Barcelona presents a positive use case for encouraging walkability and socialization in public space. The precedent from Paris also in which road space dedicated to bicycles and pedestrians is expanded helps promote the reliance on these more sustainable travel modes. To increase service offering, inspiration can be drawn from initiatives to reuse abandoned spaces or temporarily unused spaces (such as school yards during evening hours) as places to host services and activities for the community that are otherwise deficient. To increase the supply of cultural spaces, for example, temporary shows and exhibitions could be hosted in public open spaces (e.g., plazas, etc.).
Discussion and Potential Intervention Strategies
Each category of urban centers (from micro-centers to large urban centers) calls for a different set of strategic actions to help bring each area type to its highest accessibility potential. Most of the areas in need, namely those in which essential services are not accessible either by foot or bicycle within 15 minutes, require the most intensive intervention in the form of diversification and densification of services. In contrast, in areas with sufficient foot accessibility, it suffices to improve pedestrian and cycling infrastructure to ensure easy and comfortable access to already existing services. For the category in between, a mix of both interventions can be optimized for each zone.
Pop-up infrastructure, such as kiosks and mobile service vans, are another way to rapidly extend services to underserved areas. These initiatives should, however, be supported by parallel long-term planning schemes for permanent infrastructures.
37
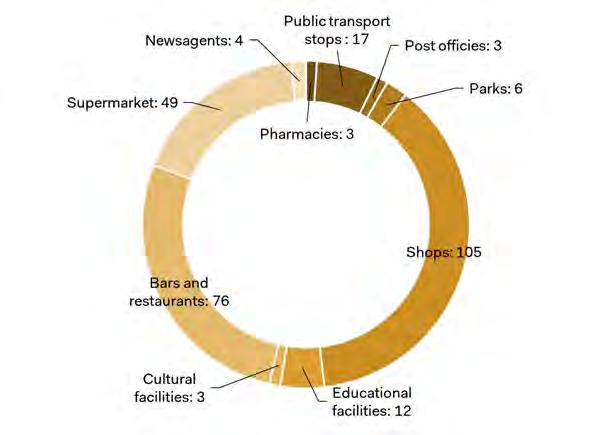
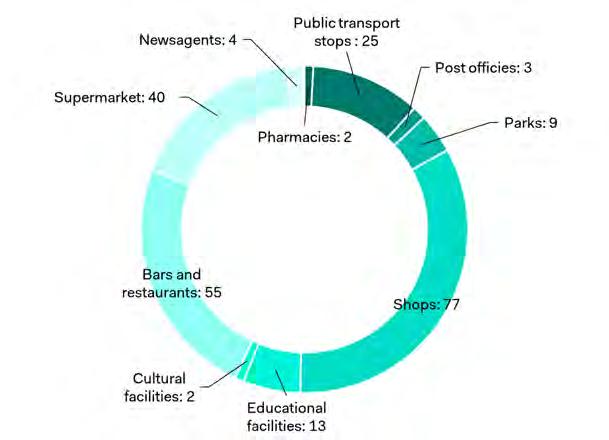
The following study follows an analytical approach aiming to address the transition of the city of Milan towards a wider and more homogeneous supply of public space. The mapping exercise is designed to measure the availability of easily accessible public open spaces on foot from home and their crowding risk based on distributional factors. In essence, it aims to study the effects of the pandemic situation on residents’ ability to safely enjoy public space in different zones of the city.
38
The pandemic situation has influenced social behaviors in space and placed emphasis on the importance of open-air gathering spaces as safer alternatives to indoor venues, adding pressure on often limited and scarce public spaces in the city, particularly during lockdown conditions when regulations made them the primary settings for active and community life. This noticeable transition highlighted the inequality of public space availability across the city.
Access to Green Areas and the Public Realm: The Case of Milan
The mapping analysis revealed that 35% of public parks found in Milan are potentially affected by crowding risk due to distributional differences and that these areas are mostly clustered along the second ring of Milan between city center and periphery. Under lockdown conditions, neighborhoods in these areas suffered lower levels of public space availability.
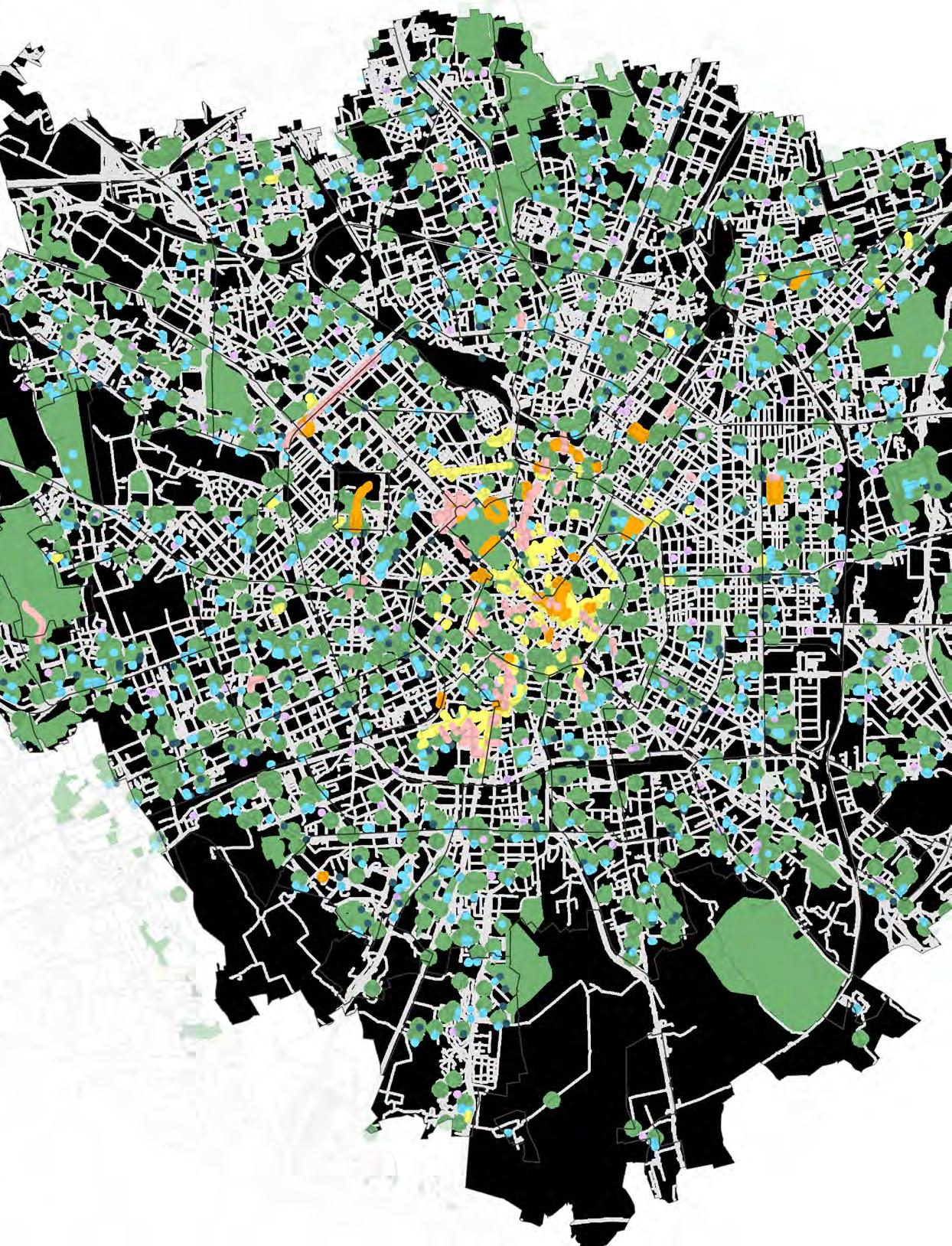
Public Realm Categories
Large Parks Small Gardens Medium Parks Playgrounds Dog Areas Oratories AreasPedestrian LEZSquares
Figure 1 Distribution of public spaces in Milan by type
The study originates from a comprehensive survey of Milan’s public space. Data collected from official sources and platforms are integrated to create an atlas of the main categories of public urban spaces found in Milan including parks, gardens, dog areas, oratories, plazas and pedestrian areas.
Figure 1 shows the cumulative result highlighting the concentrations of different types of public space across the city. The distribution of public open spaces in Milan by type are also measured quantitatively in shares by surface area. Large parks make up 70% of available open public spaces in the city.
39
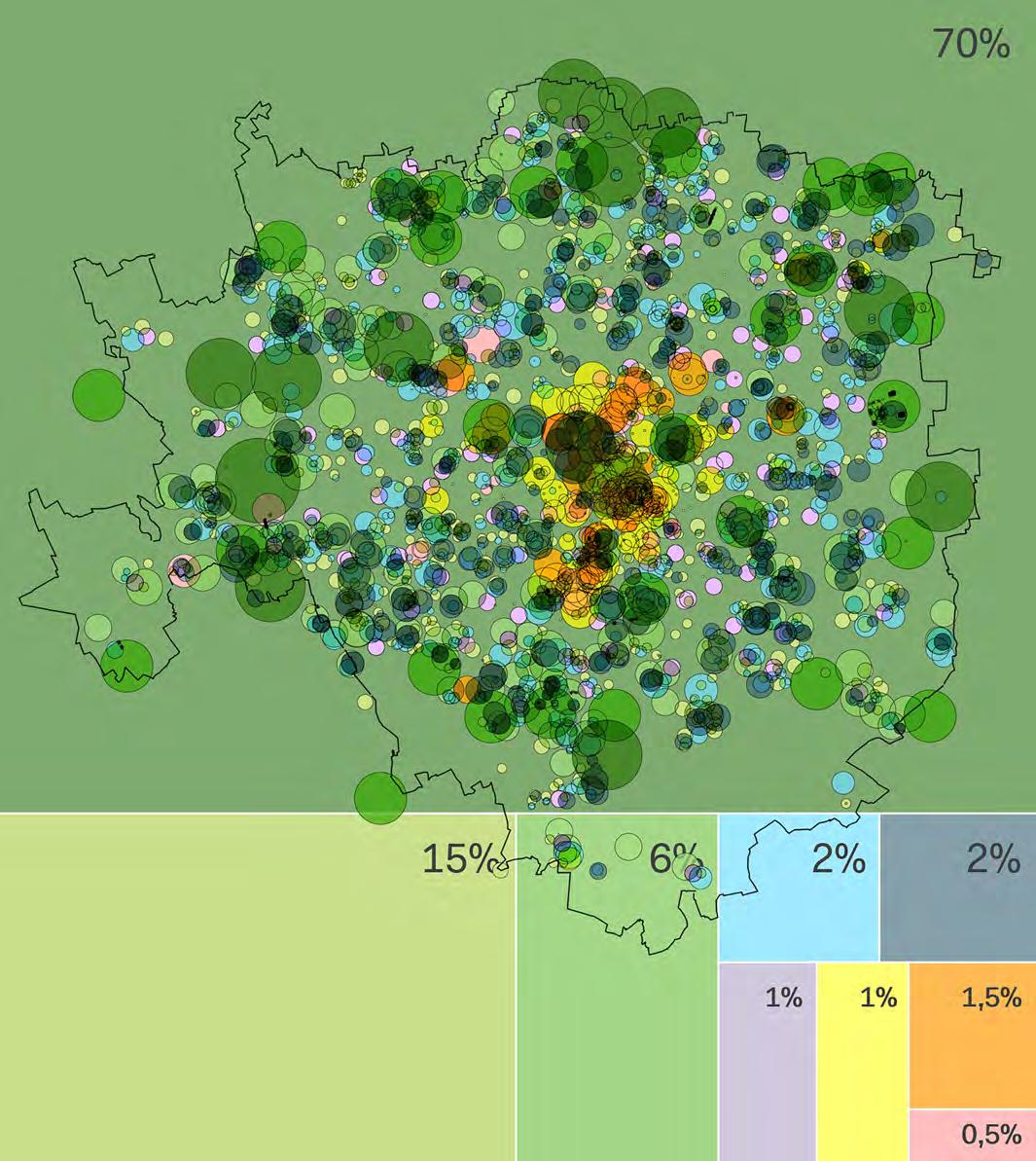
In light of what was inferred from the previous analysis, which highlights the predominance of green areas over other types of public space, the distribution of large public parks and gardens per neighborhood is analyzed (see Figure 3).
Figure 2 Distribution of parks and gardens across Milan Public Parks and Gardens
Public Parks and Gardens per Neighborhood
40
Large Parks Small Gardens Medium Parks Playgrounds Dog Areas Oratories AreasPedestrian LEZSquares
The overall extent of Milan’s public realm reaches 21.000.000 m2. Parks and gardens are predominant, occupying 11% of the municipal area, while playgrounds, pedestrian areas, dog parks, etc. tend to be smaller in size and more dispersed across the territory.
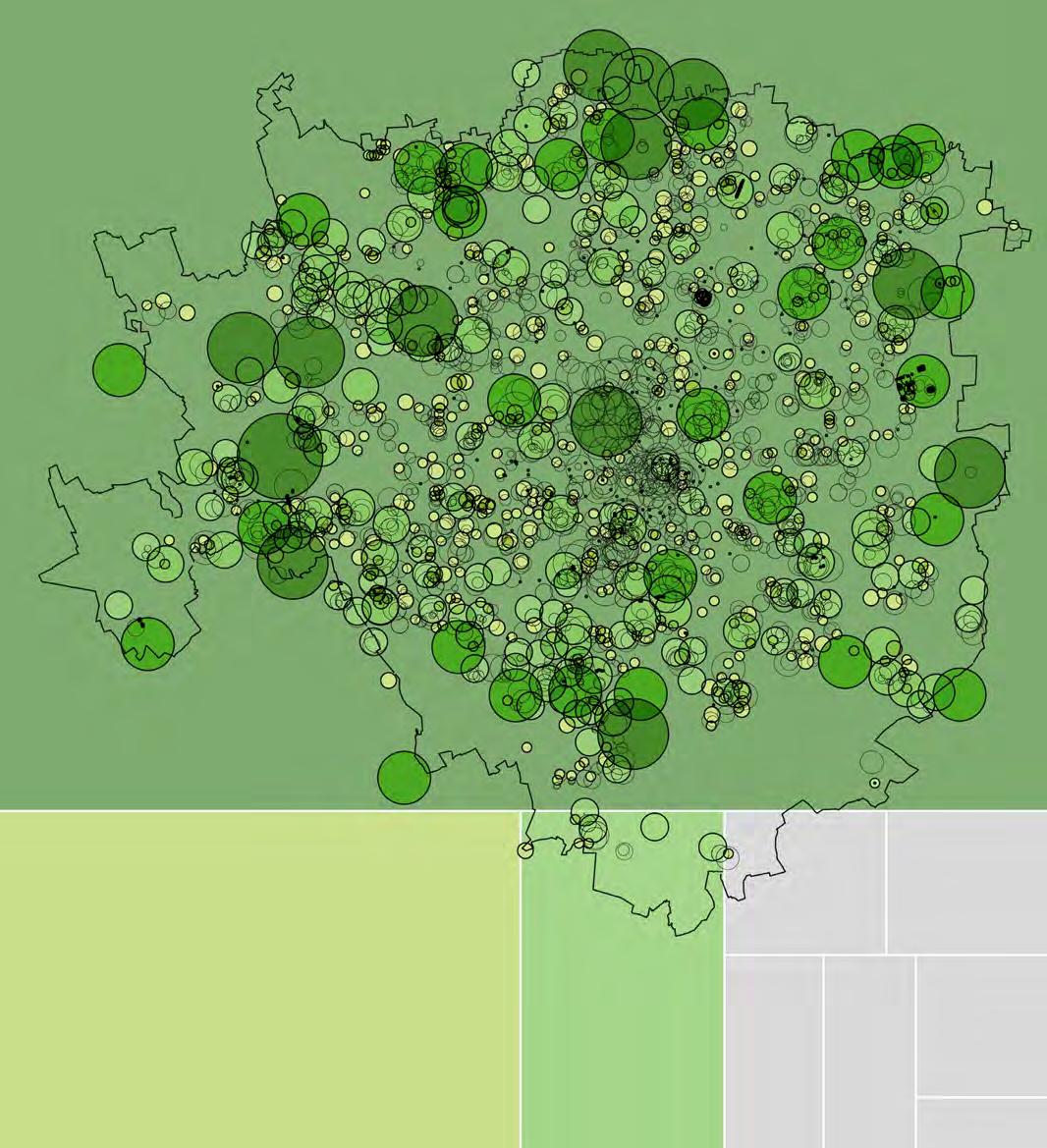
Neighborhoods
Park provision Large Parks
The isochronal analysis allows us to identify urban areas whose residents live less than 15 minutes away on foot from a specific point. This analysis is conducted for all categories of public spaces investigated in the study, as shown in Figures 4 and 5. This reading of the city reveals the number of inhabitants able to reach a recreational area in less than 15 minutes and the urban areas least served. In Figure 4, the map to the left shows that the distribution of medium and large public parks leaves some areas of the city uncovered. On the other hand, the diffusion of other recreational areas (right) partially compensates for these pockets. Nonetheless, the supply of these areas is more concentrated, resulting in a supply of 1.4 square metres per capita, on average.
Figure 3 Large parks distribution across Milan by neighborhood Figure 4 Catchment area to different types of public spaces across Milan Catchment area to the main public parks and gardens Catchment area for the other gathering areas
15-Minute Accessibility to Urban Parks
41
(m²)
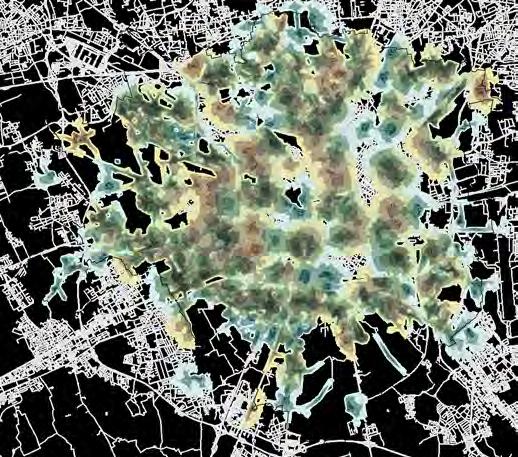
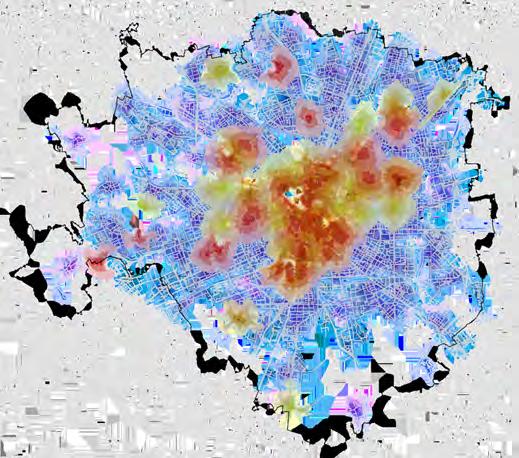
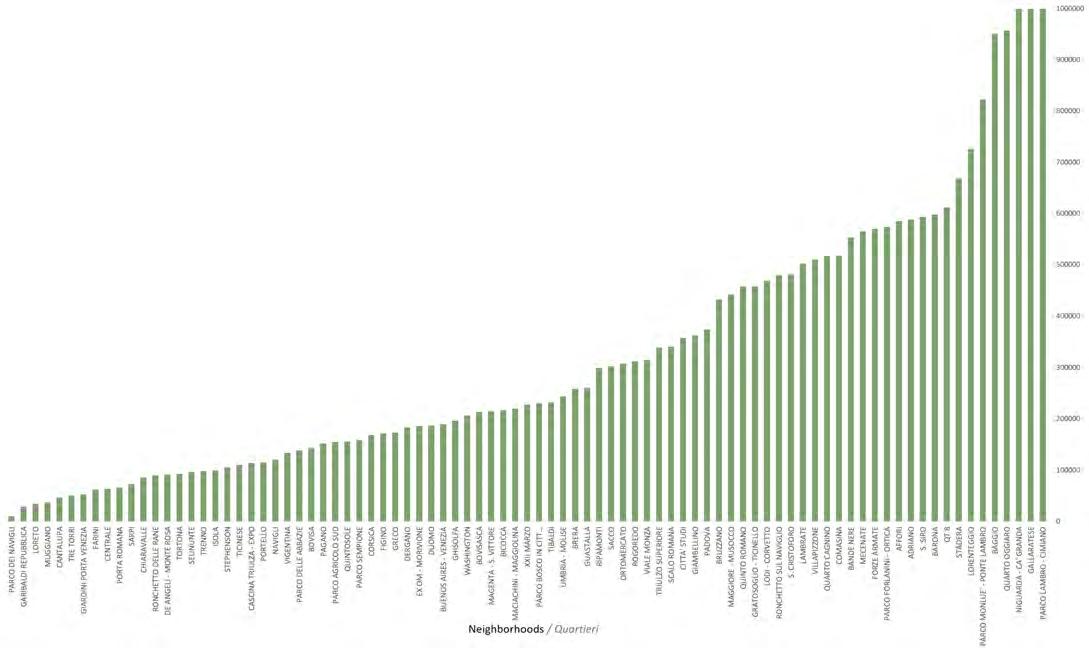
Large public parks (>12.000 m²) Pedestrian areas Medium public parks (6.000- 12.000 m2) Oratories m² / pers. m² / pers. m² / pers. m² / pers. Figure 5 15-minute accessibility to public space in Milan by public space category 15-Minute Accessibility per Category 42
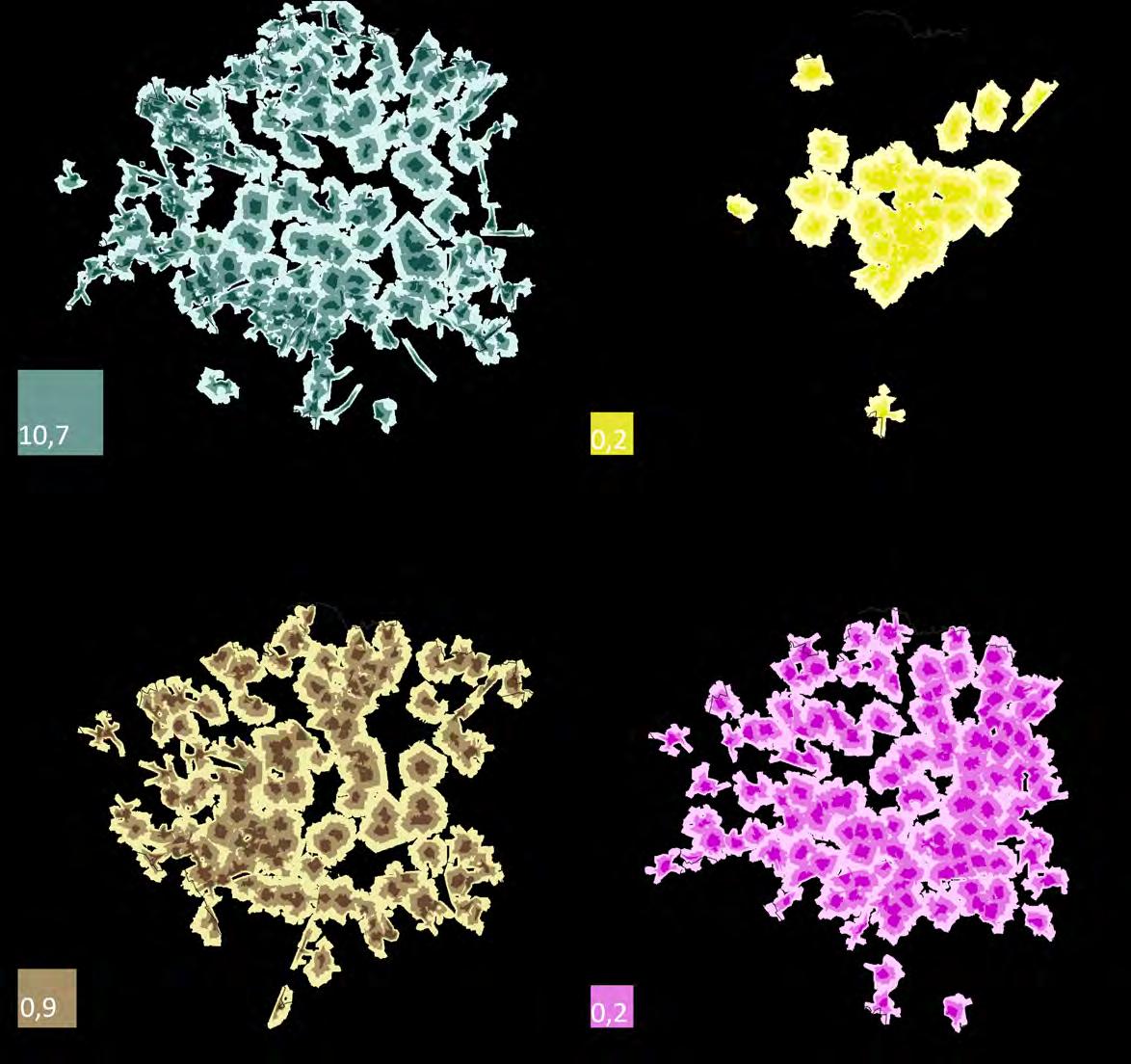
LEZ (Low Emission Zones) Main squares Dog areas Playgrounds m² / pers. m² / pers. m² / pers. m² / pers. 43
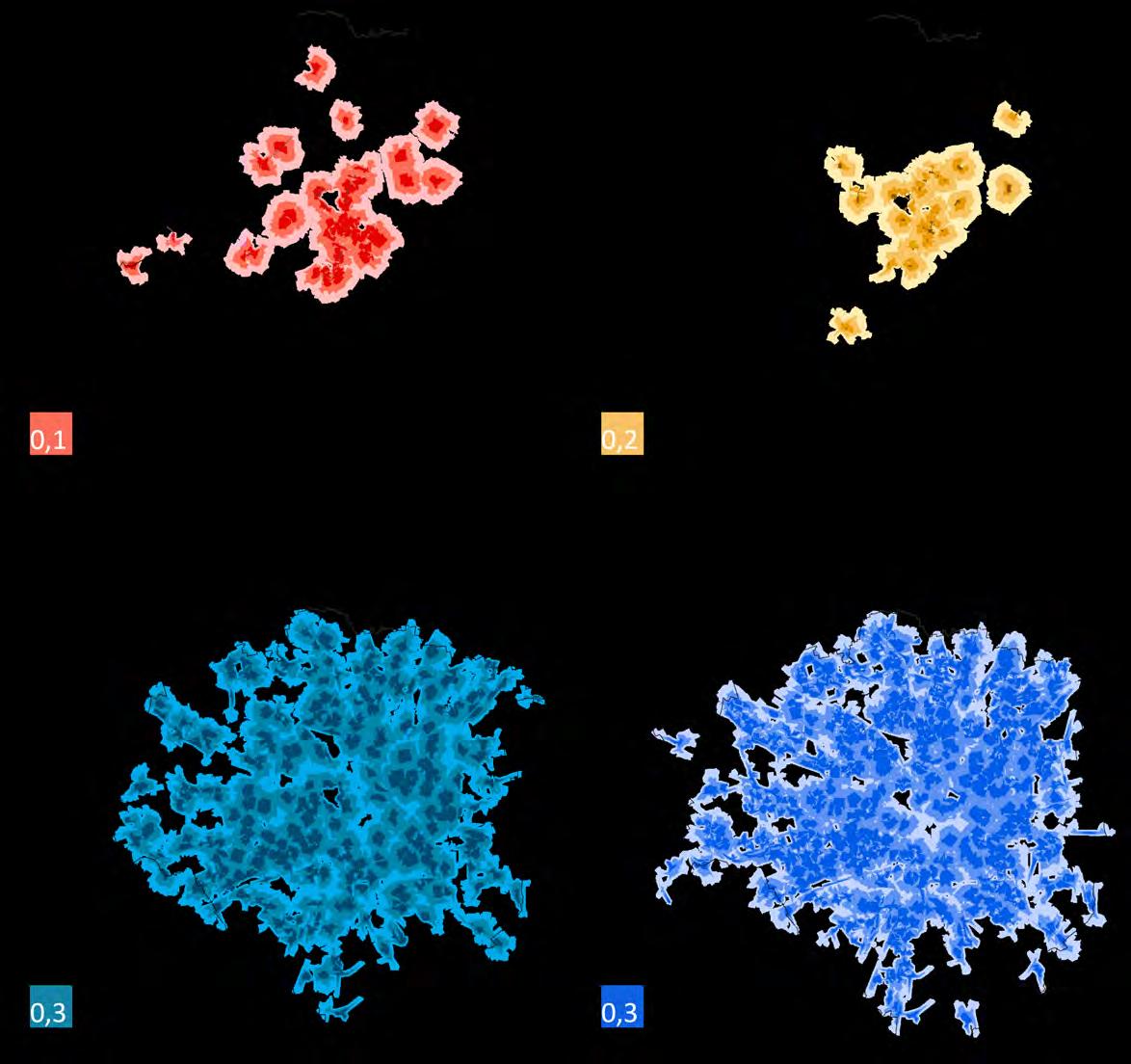
Gathering area provision (m²) Figure 6 Distribution of public space provision by surface area per capita across Milan Gathering Areas Reachable in 15 Minutes and Crowding Risk in Public Parks
Figure 6 shows the detailed processing of the results obtained through the isochronal analysis. The analytical process starts with the public space provision within 15 minutes from each census cell. Then, information about the residing population is analyzed. This methodology allows us to obtain a synthesized reading of the municipal area that compares the population density with the availability of recreational areas within 15 minutes from each census cell. The chromatic scale identifies the total amount of recreational areas available to each resident within 15 minutes. As shown on the map, more critical zones are located in proximity to the external ring-road, with episodic extensions along the north-east, south-east and west axial roads.
As highlighted through the survey of Milan’s public realm, parks play the greatest role among all urban recreational areas. Based on their size and proximity to residential areas, a map of crowding risk is produced (see Figure 7). The exercise is carried out considering the area provision per resident within 15 minutes from each park. Green areas providing less than 2 m2 per resident are considered potentially affected by crowding risk.
PoorIntermediate Sufficient Non residential areas Gathering areas 653,000 Inhabitants of scarely served areas 293,000 Inhabitants of medium served areas 294,000 Inhabitants of highly served areas
Emerging Behaviors of Historic Community Needs
The spread of the Covid-19 pandemic has placed the provision of public spaces in urban areas at the center of the global debate. In Milan, as in many other metropolitan areas, higher use of public spaces has been reported during the pandemic and especially during lockdown 44
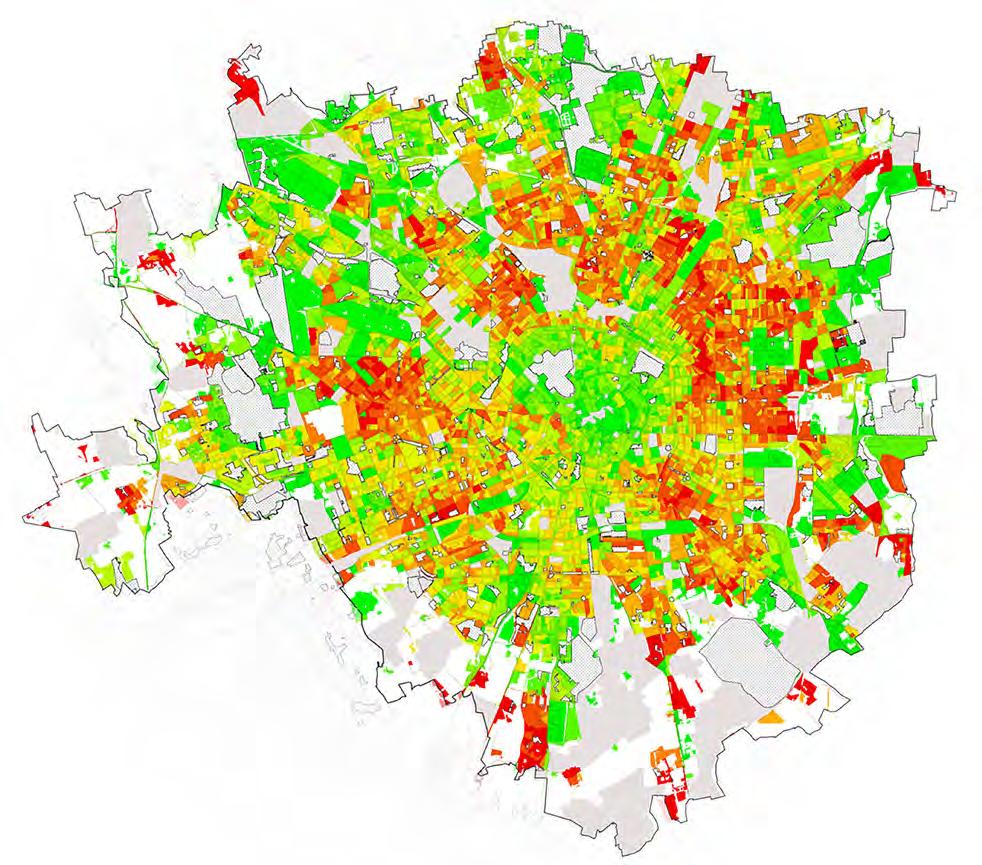
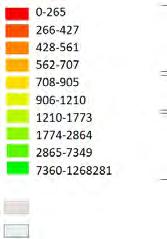
The conclusion of the study intends to provide preliminary guidelines for balancing public realm access across the municipal territory, as discussed in the following section.
Cumulative population within 15 minutes walking from census block 38% Of public parks potentially affected by crowding risk
Figure 7 Population distribution within 15 minutes reach of public spaces as indicator of crowding risk states (Geng et al., 2021). A careful reading of these behaviors would recognize them as a silent expression of the time-tested needs of the community to gather, socialize and to see and be seen in public. Since the '70s, the pioneering contributions of urban planning and environmental psychology scholars emphasized the role and importance of open spaces and natural settings for psychological wellbeing (Kaplan & Kaplan, 1989). The pandemic situation places even more emphasis on the need for restorative open spaces in the city, prompting the need to investigate new forms of reclaiming the public realm.
SystematicabyPhoto
45
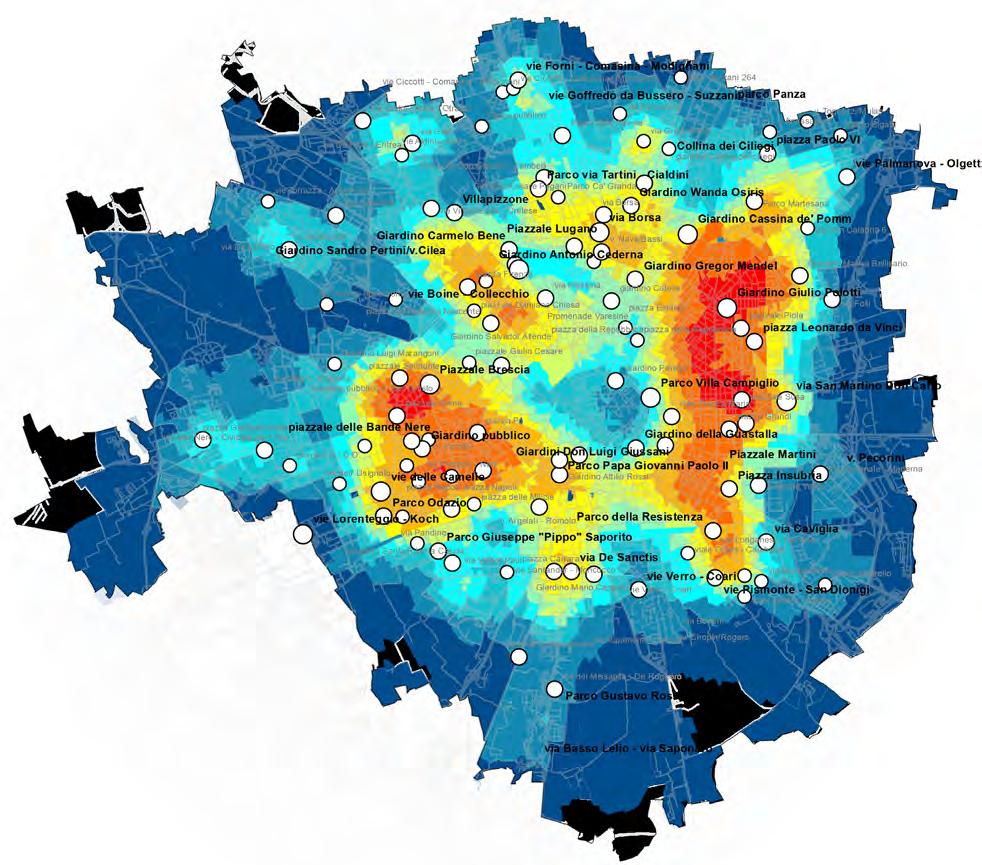
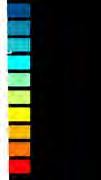
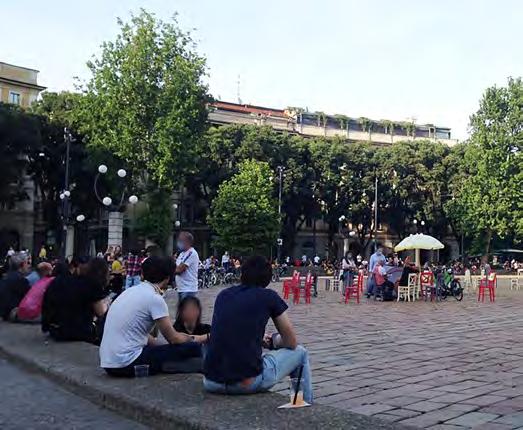
Cluster for enhancement Planned Open increase824.000m²GatheringNon-residentialSquaresareasareasRecommendedinpublicspaceprovision Figure 8 Clusters for intervention showing poor accessibility to public spaces 24%15%31% Improved connections with the surroundings
46
Main Clusters for Enhancement
The analytical process leads to the identification of some urban areas with less widespread and less extensive availability of public spaces in relation to the residing population. The map groups these areas into 8 main zones affecting 25 Milanese neighborhoods. For each of them an increase in public areas is suggested based on the intermediate urban provision, described in Figure 8. The increase reflects the possibility of citizens to have a total amount of 1,000 m2 of public space within 15 minutes from their residence. This value would bring the areas concerned closer to the public space provision level currently present in the southern area of the city (e.g., Porta Lodovica, Porta Ticinese, Porta Vigentina).
Recommended increase in public space provision
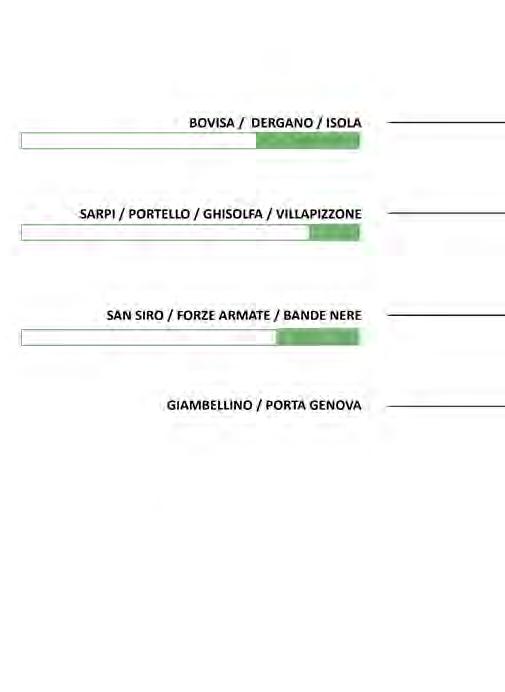
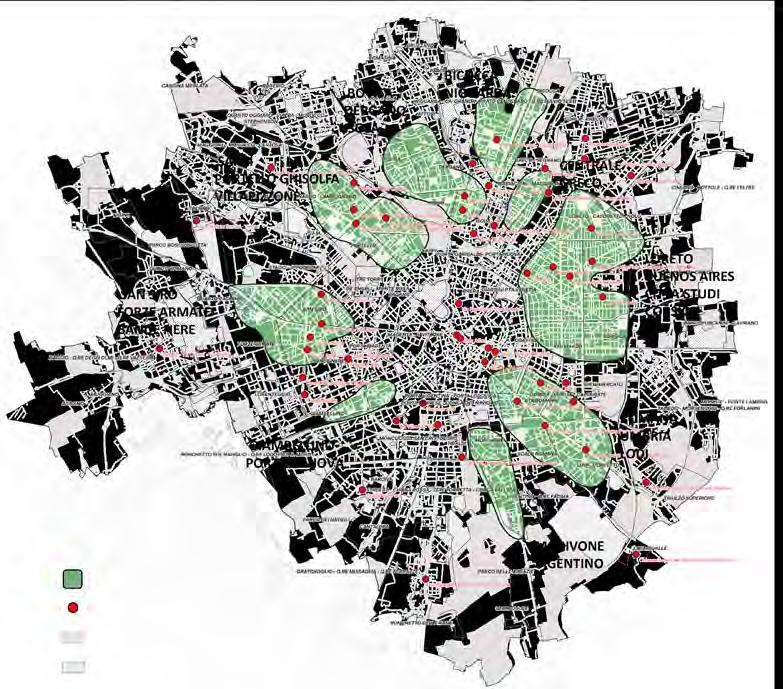
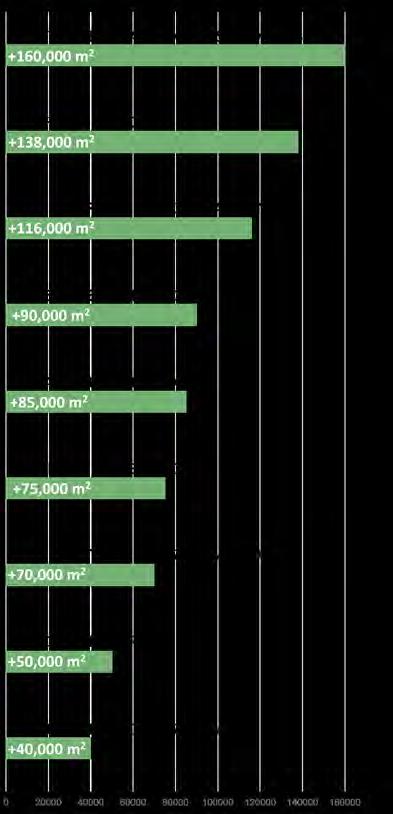
Guaranteeing a balanced level of accessibility to the public realm to residents across the city requires an integrated approach that considers network connectivity solutions as well as innovative public space creation in open pockets of the city and concerted policy efforts to ensure an adequate quota of public space in new and regenerated districts.
connections with the surroundings
Figure 9 The potential role of road connectivity in improving access to public spaces in clusters of interventionPhotobySystematica
Among the proposed interventions aimed at increasing public space access, enhancing network connectivity as a way to increase accessibility to already existing public spaces is a plausible first step and a cost-efficient solution in comparison to expanding the public space offer in the city. The capillary local roads networkrepresented in green in Figure 9 - can play a crucial role in that regard. The number of local roads potentially closed to vehicle traffic - shown in red - present opportunities for strengthening accessibility in line with the Open Streets plan by the Municipality of Milan (Comune di Milano & AMAT, 2020). In two of the identified clusters, namely Centrale/Loreto and Giambellino/Porta Genova, improved connections to the surrounding areas can have a big impact in overcoming accessibility limitations caused by the railroads.
47
Cluster for enhancement
Local Improvedroadsconnection with the surrounding
Enhancement Strategy
44%19%14%17%Improved
Network enhancement strategies for soft modes can also be implemented periodically (e.g., during weekends, etc.) to guarantee a decent level of access to public spaces during periods of high demand.
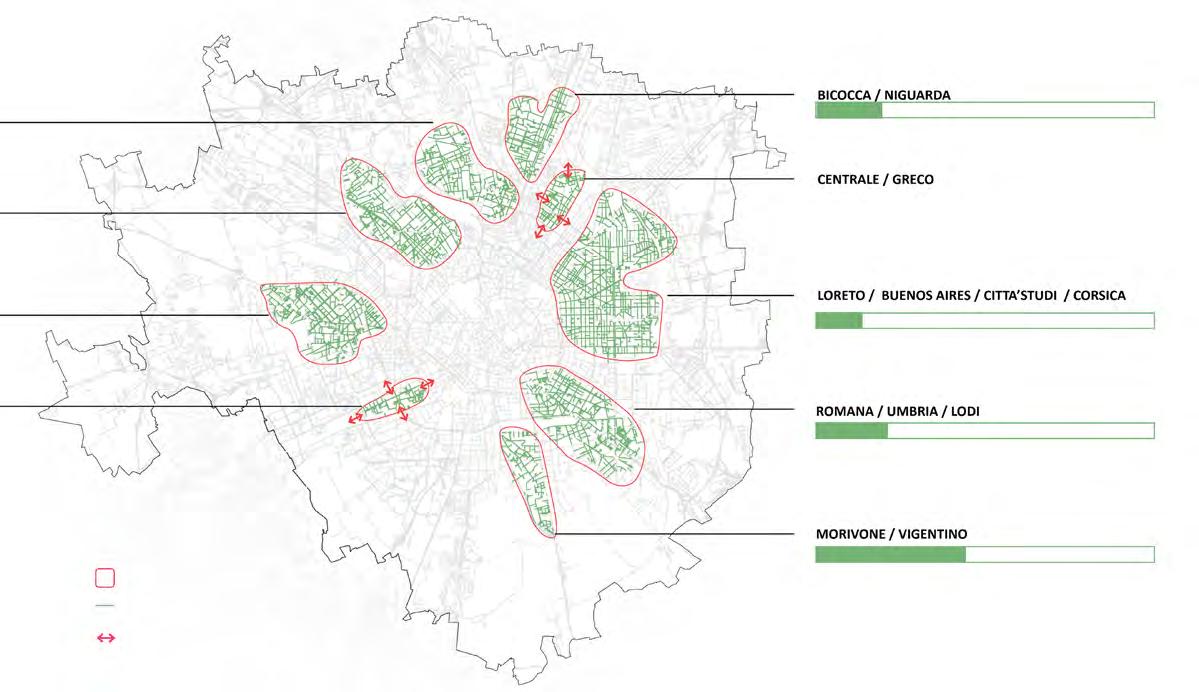
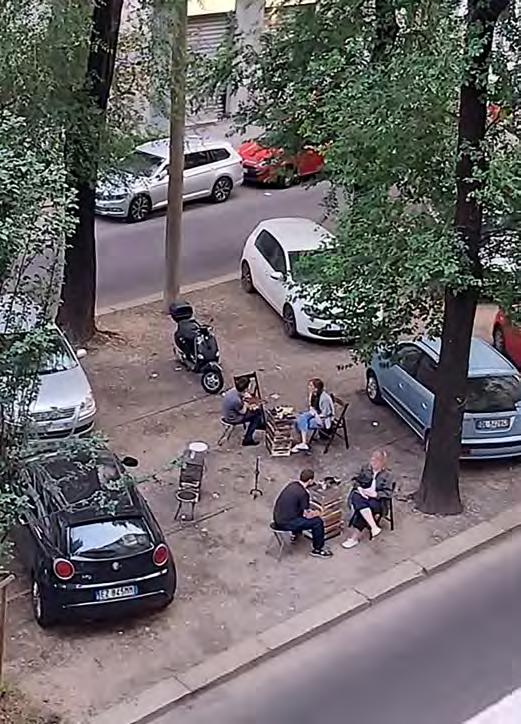
Among the many popular thematic areas in urban planning during the pandemic situation is that of the 15-minute city. The idea promotes hyper-proximity at the local scale as a vision for an equitable and livable urban system centralized around walking. Gaining support from the mobility restrictions of the pandemic situation, it aims to ensure that all essential daily life activities can be carried out within a short walk from home. In the following analysis, the chrono-centric ‘15-minute’ framework is used to evaluate the spatial distribution of walkable neighborhoods across the city of Milan. Experimenting with a multi-level spatial analysis using heat maps, this exercise is guided by a populationdriven narrative of the walkable city that considers resident and working population distribution, service distribution and walkability potential of the environment using Walk Score ® as a way to holistically approach the Overlappingmodel.
Walkability and the City15-MinuteModel 48
the multiple mapping analyses, it became possible to identify areas that enjoy a high level of population accessibility but suffer from low levels of service provision or low levels of walkability based on arrangement of the urban structure, and vice versa. These insights are useful in further defining a targeted intervention approach for each area based on its specific needs.
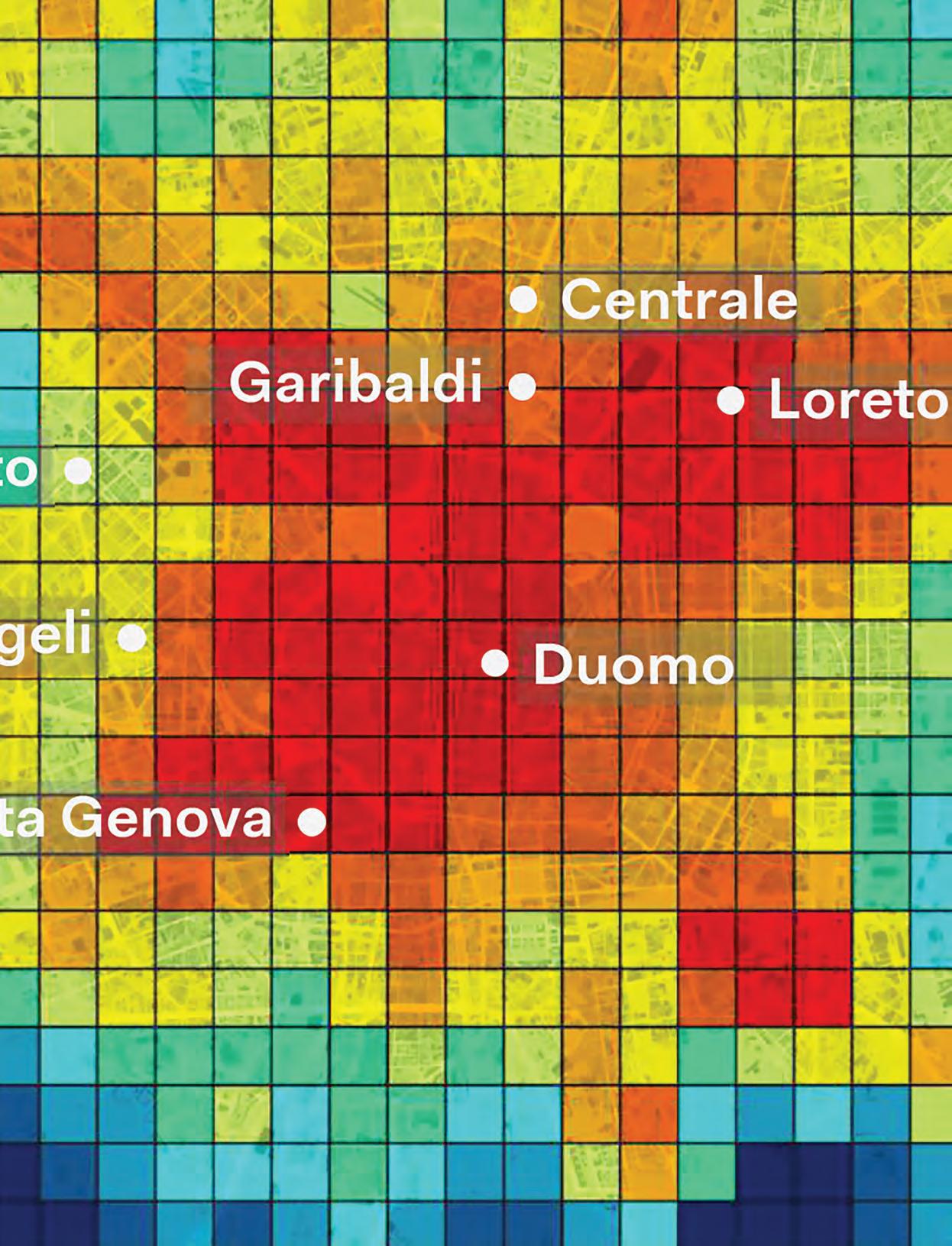
Our initial models (discussed at length in the article, Mapping Milan Micro-centers) focused on supply-side metrics: the model looked at the variety and quantity of important daily services in neighbourhoods across Milan and analysed the population distribution across the city as a way to gauge the share of residents reaping the benefits of these successful micro-centers.
49
SystematicabyPhoto
This study, in contrast, starts from the distribution of the population as a way to identify the areas accessible by walking by the largest number of people in Milan and comparing it to a static assessment of the distribution of daily services and walkable environmental conditions across the city. Pushing the envelope further, the demandbased model is dynamic in the sense that the ‘population density’ in a certain parcel/cell does not only represent people directly located in this unit, but also includes those able to access the unit within 15 minutes on foot. This shift in the approach allowed us to achieve two things: (a) by looking at both resident and working populations, we enlarged the concept of 15-minute access to include foot access from place of work as well as place of residence, accounting for the fact that the walkable environs of the working population on a typical week day is more realistically tied to their place of work as opposed to their residential address; and (b) by mapping population using the ‘ cumulative population’ method (i.e., people present in each cell as well as those able to reach this cell in a given timeframe), we draw attention to the areas of the city that are accessible to the largest share of the population as a basis for analysis, discussion and intervention.
Introduction Across the world, lockdown measures introduced at the start of the pandemic aimed to drastically restrict people’s movements to the most essential shortest trips from one’s place of residence. In Milan, as in other cities, there were ordinances that prevented inhabitants from traveling beyond their domiciled neighbourhood and effectively reduced trip purposes to essential trips such as grocery shopping and pharmacy stops. Far from the stringent early lockdown urban experiences, cities today have returned nearly to their 100% potential and inhabitants are generally allowed to roam freely within the city provided that they follow certain health requirements as mandated at the state level. Despite the major recovery of many urban mobility trends to pre-pandemic states in many parts of the world, the legacy of the lockdown state of near-immobility remains.
For many reasons, this interim phase has provoked questions about our collective mobility behaviours and placed emphasis on the long-studied neighbourhood scale and its importance in daily urban life, particularly as a vehicle for promoting walkability and particularly in the European context. Albeit for entirely different motives, the 15-minute city (Moreno et al., 2021) concept gained traction during this period for its similarities with the lived experiences of the lockdown period(s). As mobility experts, intrigued by the chrono-centric and walkability-based principles herein, we decided to test the concept’s practical application in a dense, midsized city such as Milan – incidentally, the first European epicentre of the pandemic in the early days of the crisis.
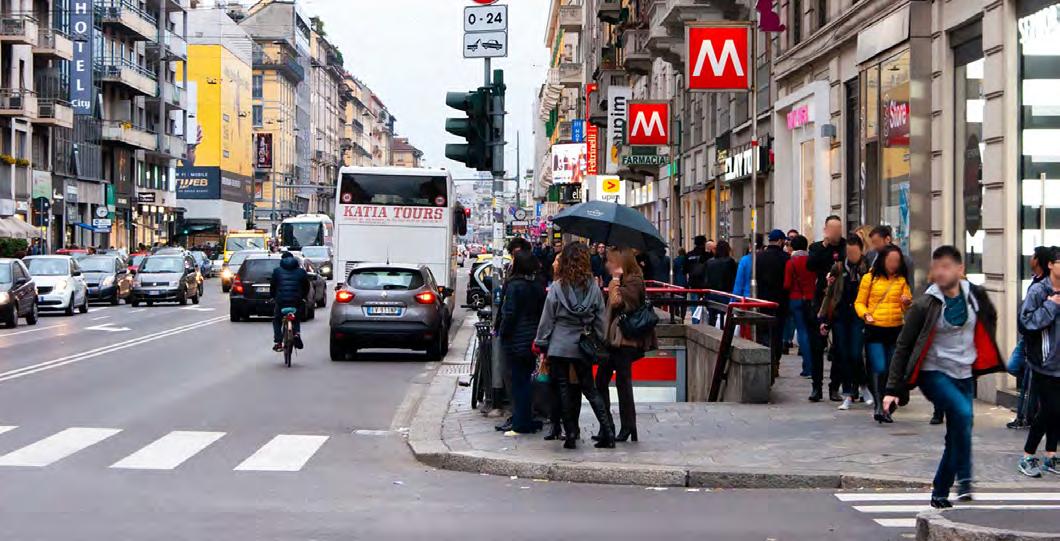
This study is organized on three levels of analysis: (i) mapping resident population and the density of workers/ employees at their place of work (workplace density) to give a first reading of the mobility character of its various districts (i.e., where trips are generated versus where they are attracted to most across the city); (ii) a static mapping of essential daily services to understand which areas have the highest variety and density of a predefined list of services which we identified as critical to the daily urban experience as based on a reinterpretation of the Parisian 15-minute city plan (Paris En Commun, 2020); and (iii) a compound walkability analysis using the patented Walk Score ® metric.
The Complex Nature of Walkability
The service proximity analysis (ii) is a pure compresence analysis calculated using simple distance buffers. It shows areas where residents can reach the majority of (at least 7 out of 9) service categories identified as crucial to support a balanced daily lifestyle of inhabitants. In total, the 9 service categories are: food/grocery stores, commercial stores (including clothes shops, electronics shops, etc.), cultural venues, educational facilities, parks and green spaces, restaurants, health facilities, sports facilities and other (post offices, banks, etc.).
50
Each of these service groups includes a number of services based on open-source datasets available for the municipality of Milan. For example, educational facilities include nurseries, primary schools, high schools, etc. A GIS-based analysis of the static compresence of these services was carried out based on a pure isometric analysis with respect to 3 walking buffers – 300, 600 and 900 meters – which roughly correspond to the 5, 10 and 15-minute timeframes.
A Demand-Driven Approach to the Chronocentric City
The Walk Score ® mapping (iii) measures the actual accessibility levels through isochronal analysis. It is based on the analysis of accessibility within 15 minutes to each group of services (there are 9 groups in total) calculated on a graph with the cost of the links based on travel time and inversely proportional to the slope of the road. The results of the 9 pedestrian accessibility analyses were then reported as indicators of accessibility to each service group on 150-meter grid. The final Walk Score ® value is a simple summation of these 9 individual analyses.
Population and workplace density Daily compresenceserviceWalkability(WalkScore)®
The path to achieve the 15-minute city is intricately tied to the potentials to deliver safe, comfortable and desirable walking environments. In other words, the idea is deeply intertwined with the concept of walkability. According to Speck’s General Theory of Walkability (2013), the level of walkability of an area is driven not only by ‘usefulness’ (i.e., the utilitarian gain of a walking trip – to get from point A to point B), but is also a result of the safety, comfort and attractiveness of this walking environment. It is driven by a complex set of tangible and intangible characteristics including the physical infrastructure conditions, the level of network connectivity, high land-use mix, vitality and distinction of urban character. As shown in the article Sidewalks Map in Chapter 1, 45% of Milan’s sidewalks do not adhere to the minimum width required for two-person movement following the one-meter social distancing recommendation by local and global authorities (Regione Lombardia, 2020; World Health Organization, 2020) and consistent with the standard for comfortable sidewalk conditions as recommended in the street design guidelines by the Global Designing Cities Initiative & NACTO (2016).
The first layer of analysis (i) aims to evaluate the density of the resident population and workplace population across Milan in relation to the pedestrian accessibility levels as allowed by the morphology of the urban fabric. The main result is therefore mapped as a “cumulative” value in the sense that it represents not only the resident or workplace population present in each cell, but also includes those who are able to reach this cell in a given timeframe (i.e., 5, 10 and 15 minutes).
However, in order to gain a holistic understanding of walkability as described herein, a walkability index (i.e., Walk Score ®) is used in this study to reflect the complex nature of walkability. Walk Score ® is a compound metric that considers various factors including both proximity and variety of services accessible by foot in a definite amount of time. Among the various indices used to measure walkability, the Walk Score ® index is chosen here for its consideration of travel time as a key variable, allowing us to tailor the analysis to reflect the 15-minute city approach.
Figure 2 Pedestrian access of the working population to different census sections of the city in 5/10/15 minutes
Results of the Analysis: A Mismatch Between Supply and Demand
A similar interpretation can be drawn from the distribution of the workplace population. The worker pedestrian access maps show a more scattered pattern of highly accessible areas within 5 minutes of walking. At 15 minutes of walking, central districts of Milan emerge as the main cluster and a center-to-periphery gradation becomes clear. The centers with the highest pedestrian access to workplace populations at 15 minutes are the Duomo district followed by secondary concentrations in the north-east districts of Loreto, Centrale and Porta Garibaldi (see Figure 2). Areas scoring lowest in both clustering scenarios (resident population and workplace population) are peripheral neighborhoods and agricultural non-built areas, such as those on the southern end of the municipality map.
High values Low LowHighvaluesvaluesvalues
The resident and workplace pedestrian access maps offer an alternative approach to chrono-centric mapping based on population density as opposed to service density, highlighting distributional differences at different time scales, giving an indication of the urban character of each district. This conceptual analysis demonstrates how isochrones change shape and intensity depending on the given timeframe. In the resident pedestrian access map, micro-clusters of local centralities emerge from the 5-minute map. As the timescale is stretched, the donut shape of Milan’s second ring-road area in which the highest concentration of the resident population is located, begins to emerge (see Figure 1).
Figure 1 Pedestrian access of the Milanese population to different census sections of the city in 5/10/15 minutes
51
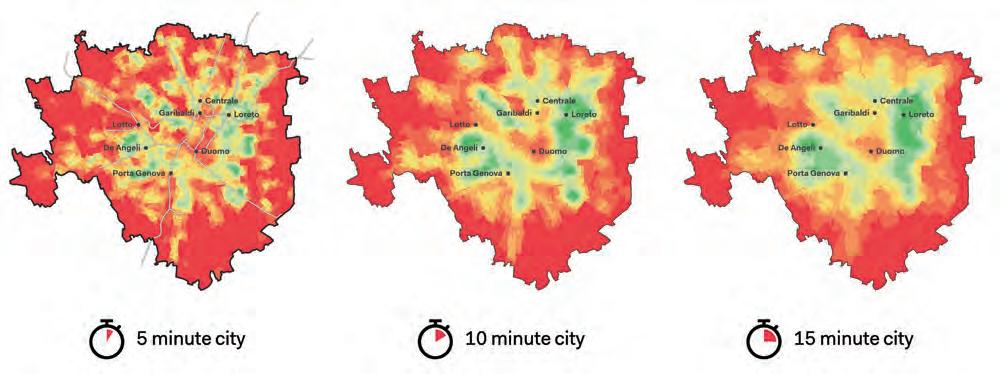
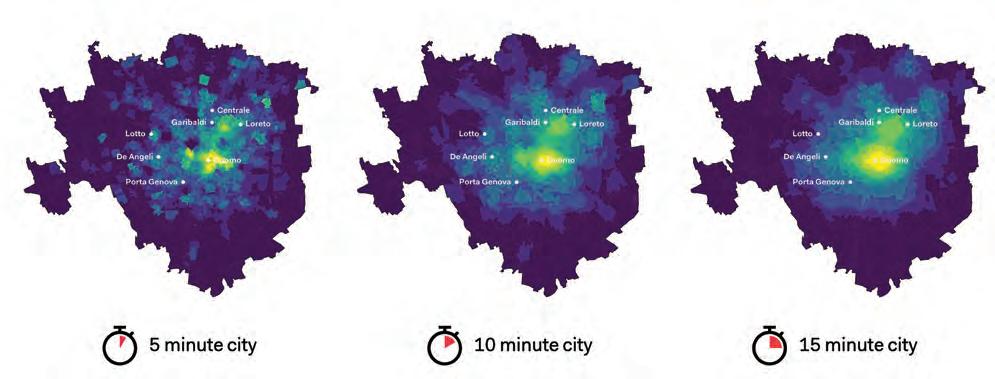
The Walk Score ® analysis set at a 15-minute radius confirms the static findings of the service compresence map. In addition, it highlights further lower scoring areas in the north of Milan that, despite adequate service density, are potentially less walkable for the failure of the urban structure to support comfortable and efficient walking trips (see Figure 3).
52
Cumulative resident population density (15 minutes) Cumulative workplace density (15 minutes) High values Low values High values Low values
Figure 3 Different analytical components of the 15-minute city model
When compared to the demand-side maps, it is possible to observe that despite the high number of residents within 15 minutes reach of the districts of De Angeli, Lotto and Porta Genova, they are not adequately covered by all primary service groups. Central areas of Milan predominantly outnumber peripheral areas in terms of density and variety of their basic service offering.
In contrast to the demand-based models, the service compresence accessibility analysis offers a first look at supply side distribution of services across the city. The map highlights the distributional differences in service diversity and density in 5, 10 and 15-minute ranges. It is clear from the map that a large portion of the city by area does not guarantee the minimum access to 7 basic service groups within a 15-minute walk.
As shown in the same chart, apart from a few spikes in the number of people in close reach of wellperforming and walkable districts such as Duomo and Porta Garibaldi-Porta Nuova, there is no clear trend in population distribution amongst the remaining neighborhoods. In fact, many neighborhoods with high population accessibility have low walkability scores such as Gorla, Cimiano and Gallaratese. These neighborhoods have high attraction potential but low pedestrian accessibility to services, signifying a need for intervention to promote equal access to services on foot across the city.
Overlaying the Walk Score ® results with the NIL (Nuclei di Identità Locale) neighborhood classification allows us to study the results of the analysis in relation to typically recognized neighborhood units at the city level. The result of this comparison reveals a striking correlation between nearness of a neighborhood to the city center and high walkability levels as illustrated on the chart below (see Figure 4). This correlation does not translate, however, to population distribution trends.
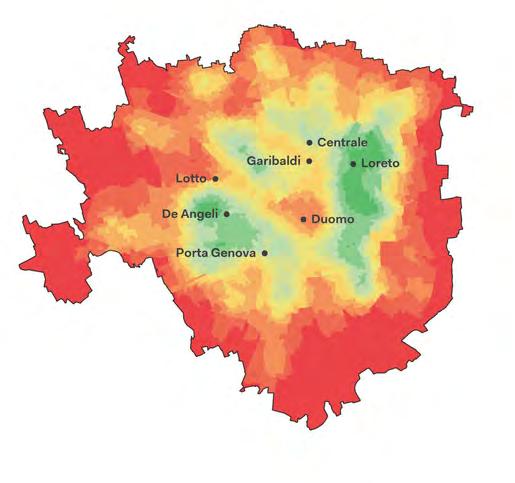
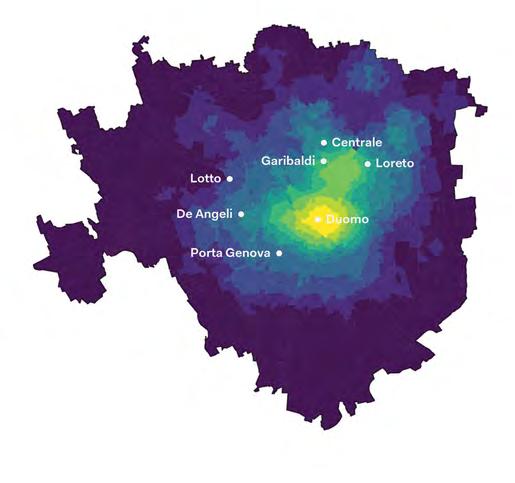
>=7 within 300m buffer >=7 within 600m buffer >=7 within 900m buffer <7 service macro-categories Service (300-600-900Compresencebuffers) (workplaceWorkingPopulationPopulationdensity) Walk Score ® (15 minutes) High values Low values Figure 4 Walk Score ® and 15-minute population accessibility by NIL neighborhoods 53
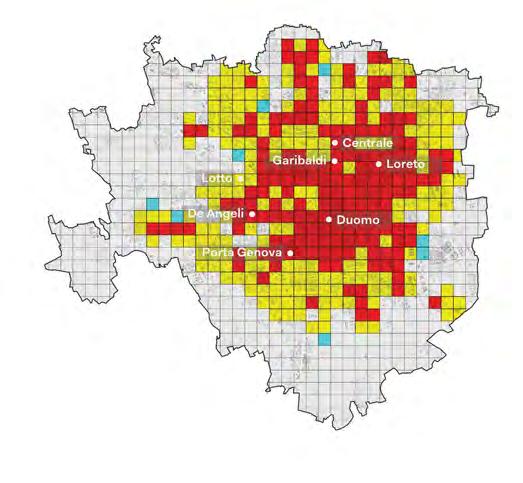
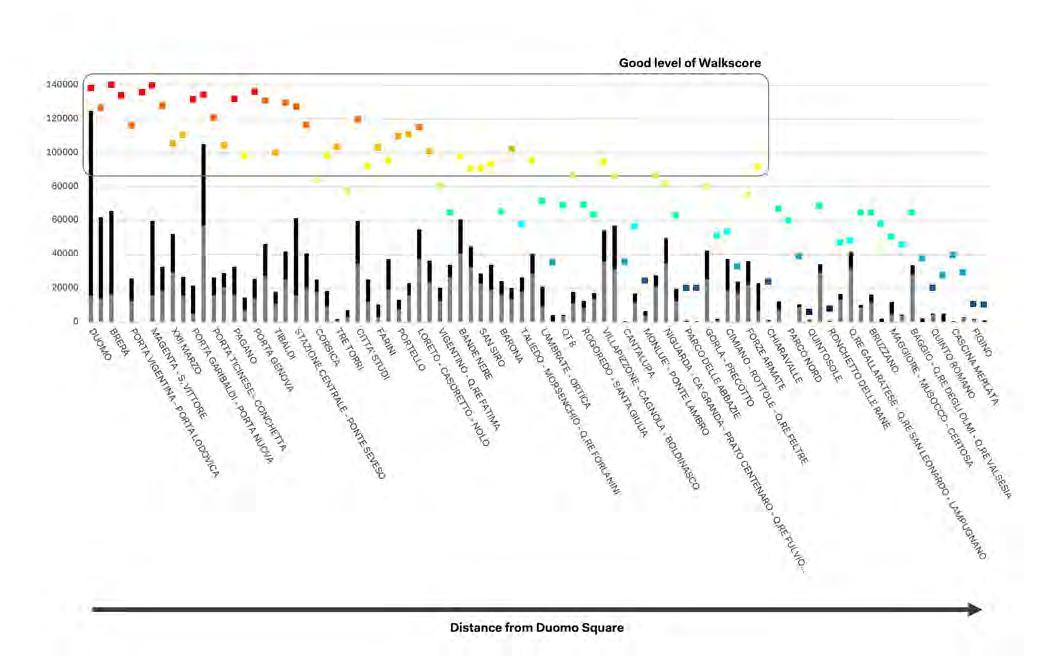
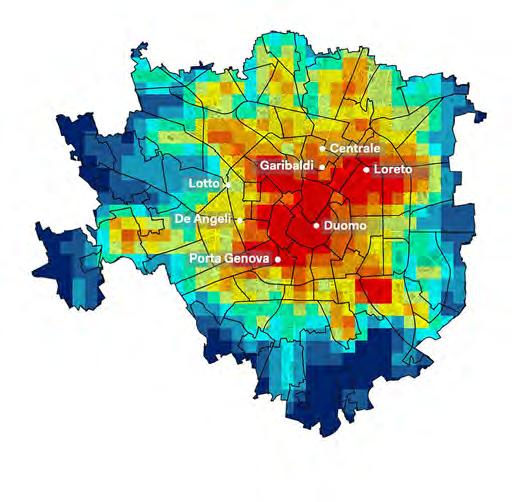
Final Discussion
54
The mapping analysis shows that Milan’s humanscale spatial structure has great potential to support walkability, should distributional differences and the mismatch between supply and demand in certain areas be addressed. From a demand perspective, the highest concentration of the resident population is within reasonable walking distance to the districts of the second ring road area, whereas the distribution of the working population cluster strongly in central districts.
Micro-mobility services and on-demand transit can help alleviate the burdens of a low walkable environment in the short run. Currently, only half of Milan benefits from the ease and convenience of a 15-minute city. A practical solution to support the model could be to boost firstand-last-mile mobility solutions to extend accessibility ranges while maintaining limited trip times and energy costs. Global trends show that micro-mobility devices have a significant role in reducing the first-and-last-mile gap and are often paired with public transport (Heineke et al., 2019; Zagorskas & Burinskienė, 2020; Boglietti et al, 2021). One of the main limitations of micro-mobility, however, is its demographic exclusion of higher age groups (ibid.). For older citizens and users with assisted mobility needs, on-demand transport may be a more suitable intermodal solution.
Intermodal Support for the Transitional Phase
This research highlights the diversity of approaches to address walkability in cities and proposes a demanddriven approach to measure the 15-minute city. It progresses the view that 15-minute cities can only work if cities are not only efficient but are also designed to encourage more walking from a multi-angular perspective that includes both functional and experiential factors. Walking infrastructure shortages could be overcome by broadening the perspective of the public realm as a whole and adopting a holistic approach of the walkable ‘urban living’ model. Short-term flexible interventions could focus on upgrading existing open spaces (through sidewalk redesign and integration of new micro-mobility modes) whereas long-term strategies should extend to policy design promoting egalitarian access opportunities across different districts in the city – and in particular in peripheral neighborhoods – while placing the walkability agenda at the center of neighborhood design. Ongoing local plans in the City of Milan reflect the shift to prioritize walkability as a sustainable growth principle. Both the Territorial Government Plan (PGT) 2030 and the Open Streets plan developed in the wake of the pandemic crisis highlight the importance of pedestrian-focused interventions to improve urban connectivity and accessibility (Comune di Milano, 2019; Comune di Milano and AMAT Agenzia Mobilità Ambiente Territorio, 2020).
On the other hand, if we look at the supply side of the equation (i.e., the walkability of the pedestrian network and the distribution of services), we find that there is missed potential in several pockets of high demand that are not adequately served by everyday destinations and are not sufficiently walkable from a multi-dimensional standpoint. By considering both sides of the equation, the combined series of maps captures not only the current state of the city, but the potential to extend its reach based on its intrinsic morphological capacity to attract daily users (i.e., residents and workers).
SystematicabyPhoto
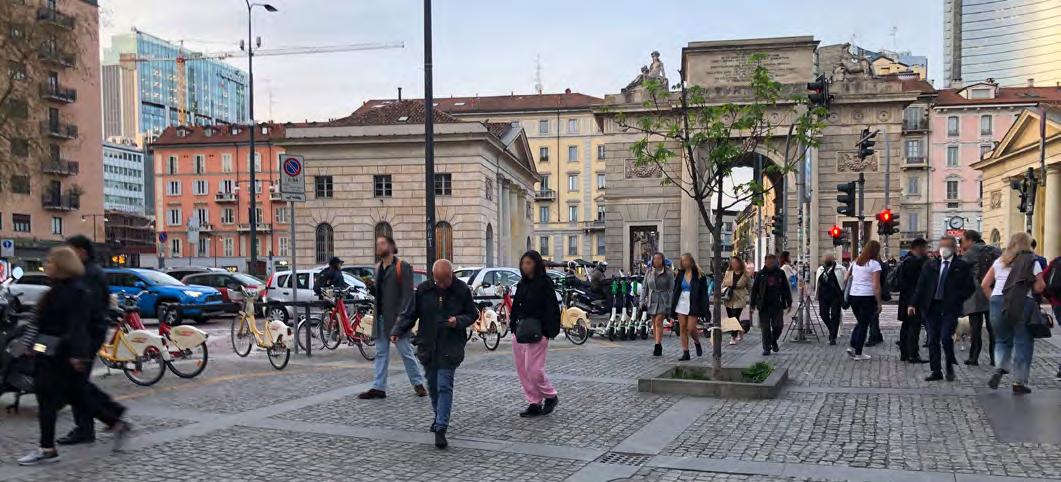
Moving Forward
A comprehensive view of mobility that considers the augmented role of digital infrastructure in fulfilling daily urban needs is essential for a critical analysis of mobility systems in the contemporary urban landscape. Notwithstanding the potentials offered by digital infrastructure substitutions, we also caution that an excessive reliance on these solutions could lead to the denial of what we believe is the key role of public space from a contemporary interpretation: fertile ground for triggering opportunities of relationship and aggregation, which is the basis of the concept of walkability.
55
Abdelfattah L., Deponte D., Fossa G. (2022). The 15-minute city as a hybrid model for Milan. TeMA - Journal of Land Use, Mobility and Environment, 71-86. https://doi.org/10.6093/1970-9870/8653
A critical understanding of mobility under contemporary circumstances, which considers digital as well as physical infrastructure is essential for accurately representing the 15-minute city. There is potential in exploring the introduction of ‘zero-minute trips’ as part of a new hybrid conception of the 15-minute city that considers the contributions of digital services in replacing physical trips. Especially in the context of unprecedented growth of remote working since the start of the pandemic, urban mobility models stand to benefit from reductions in work commutes and thereby traffic congestion, which can both directly (hyper-proximity) and indirectly (road safety, reduced noise and environmental benefits) improve walkability prospects in cities. On the other hand, the marked drop in retail and replacement with online shopping has myriad effects on the urban mobility system. Whereas on the one hand it reduces user trips, it is replaced by increased logistics and delivery trips which has various implications on traffic and curbside utilization in urban areas. Both of these trends have been largely influenced by the pandemic situation.
The results of this research activity have been published in the following scientific Journals:
Abdelfattah, L., Deponte, D., Fossa, G. (2022). 15-Minute City: Interpreting the Model to Bring Out Urban Resiliencies. Transportation Research Procedia, 60, 330-337. https://doi. org/10.1016/j.trpro.2021.12.043
The mobility standstill brought about by the coronavirus pandemic in early 2020 provided a real-life simulation of what it would mean to live entirely within a few short blocks from home, generating interest in local urban communities and what the neighborhood context can offer residents across different areas in the Thiscity.article discusses an idea for a digital platform that addresses these urban user questions using a storytelling approach. Co-created with Accurat, a leading data-driven innovation firm, expert in digital storytelling, the “Stories” app was developed as an open-source and decentralized tool designed to engage citizens on urban initiatives transforming the way they live and move around the urban context.
Based on data-driven stories, the digital platform allows a two-way framework of communication between people and local entities, who can mutually collect and share -on a voluntary base – georeferenced information. Built on open-source technologies – such as OpenStreetMap – the app allows private and public stakeholders to freely design, personalize and deploy their own place-based stories.
In this sense, Stories doesn’t rely on centralized data owned by private actors, but is backed by aggregated and anonymous data and includes offline capabilities to allow the platform to be used in areas with poor network connectivity.
AStories:Platform
Built for StorytellingPlace-Based 56
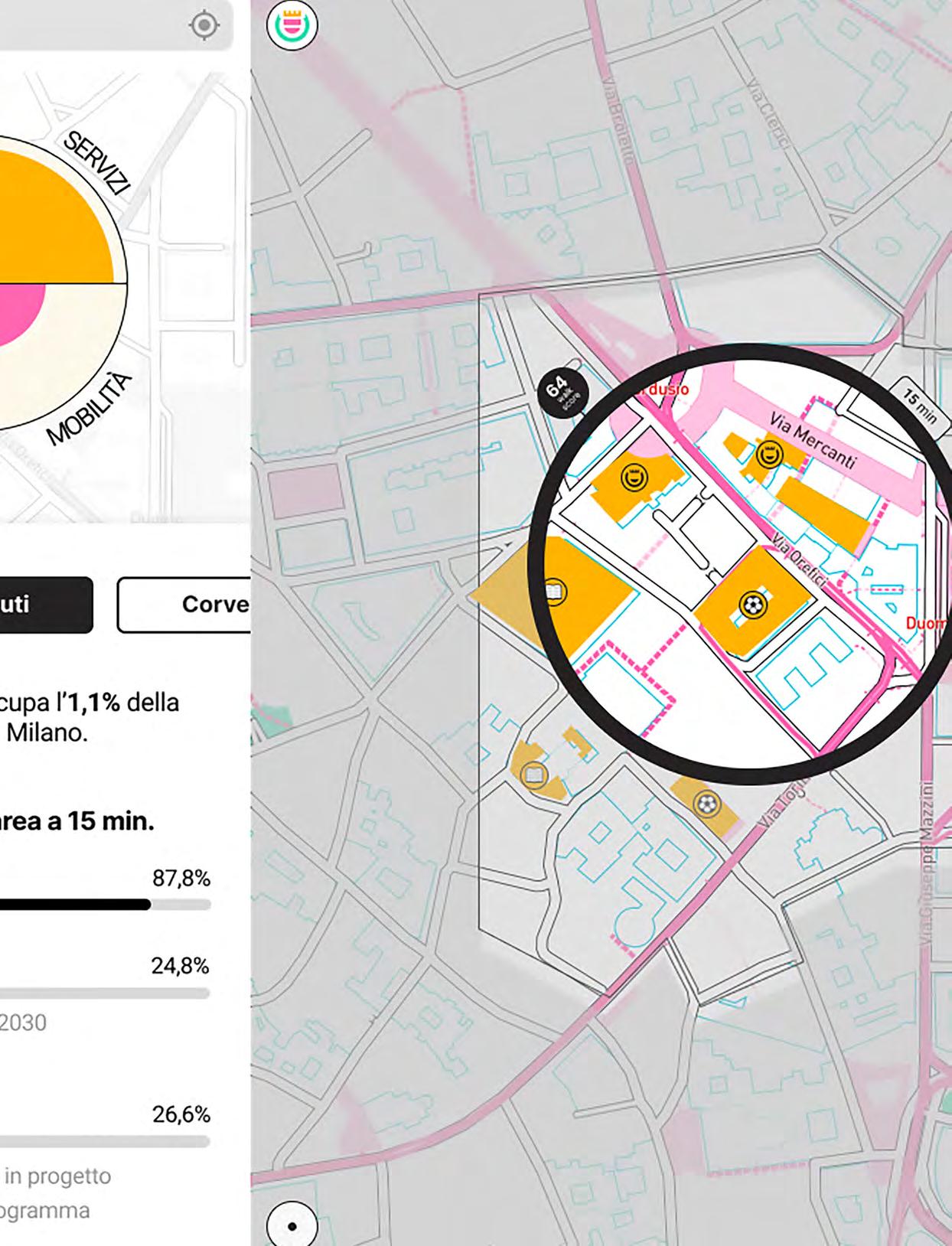
The explosion of location-based data and data collection tools in recent decades has led to a paradigm shift for urban and mobility planning. For the first time, movement patterns happening all around the city could be gathered passively at a large scale and synthesized in ways that give us insight about changes in mobility behavior in realtime. Not only that, but the ubiquity of geo-referenced data (in large part owing to digital miniaturisation) has led to the decentralization of data collection to billions of data sharers, significantly broadening the type and depth of movement data we can collect; a phenomenon we like to call "data in every pocket" (Choubassi & Abdelfattah, 2020). Simply put, Big Data (and in particular locationbased data) is transforming how we document, collect and understand mobility patterns. In the words of Batty, "This revolution in tracking human and other motion in digital form enables the collection of multiple attributes at the finest of scales of urban observation" (Batty, 2010, p. 576).
People Places InformaticsUrban Technologies People & Places Interaction & Experience Design Community Engagement & Development Humanities Communication StudiesInformation Networks Data Science Human-ComputerInteractionSocialMediaUrban Sociology UrbanArchitectureUrbanDesignStudiesUrban&RegionalPlanning ComputingUrban TechnologyPervasive Location-Specific Services SystemsDesign MakingPlace People & Technologies Places & Technologies Figure 1 Urban Informatics relational chart Using Urban Informatics to Understand and Respond to Citizens’ Needs
57
It is within this framework that this project is conceived. Conceptually speaking, Urban Informatics operates at the intersection of three domains: People, Places, and Technologies. The Stories App project was executed in collaboration with Accurat, a leader in the field of Data Science and data visualization, with a deep-rooted philosophy of "Data Humanism" (Lupi, 2017), which is an approach that attempts to deliver data to people in tangible and approachable ways. In that sense, the Stories project is one that combines the expertise of two industry experts into a multi-disciplinary approach to reimagine urban mobility as a customized, simplified and humanized daily experience.
The discipline through which urban planners employ these technology-enabled spatial analysis methods has come to be known as Urban Informatics. Urban Informatics is an evidenced-based approach that, thanks to the recent development of advanced Information and Communication Technologies (ICTs) and the increasing availability of digitally widespread data sources, has led to innovative assessment tools and metrics that can provide valuable support to city decision makers (Foth et al., 2011). Due to unprecedented data volumes, granularity and diversity, problems of sampling bias associated with traditional data collection methods can be sufficiently overcome, allowing the data to be manipulated to focus on specific communities, their behaviors and needs (Batty, 2013; Milne and Watling, 2019).
Stories’ ethos—to put users’ needs first—is embedded into the prototype’s architecture. Open source, location–based data made available through the Comune di Milano’s geoportal powers this version, and represents a compelling use case. The prototype demonstrates how municipal data can be put to use as the foundation for a handy mobile app, equally valuable to locals and visitors.
At a higher level, the Milan prototype shows how Stories can be leveraged by local governing bodies to create a positive feedback loop for information–sharing. We anticipate that users who find the app valuable will, in turn, be more likely to contribute tips, ultimately improving the accuracy of city data. The end–product of this scenario is a strong offering, unique in its ability to serve sponsoring entities and users, both as a practical navigational tool and an ethical way to solicit data.
58
The app was designed as an alternative to tech giants’ map–based, navigational offerings, which are supported by (and thus, favor) local business interests.
Figure 2 A screenshot from the Stories App The Stories App When users log in, they are greeted with a map of a locale marked by visual indicators. These icons show quality of life measures: proximity to green spaces, transportation, areas of culture and recreation, and demographics. The placement of these data visualizations shows the quality of amenities in the corresponding area across the four aforementioned categories. Users can customize Stories to reflect specific considerations. For example: the mobility category can be adjusted to only display bus lines, or bike racks. This visual system gives users an at–a–glance view of how well–suited an area is to meet their needs, whether they’re exploring as tourists or evaluating places to live. After gathering their bearings, users are invited to take a tour by choosing a “Story” from a menu. In this example, the "15-minute-city" is the theme. When activated, the guided overview plays, taking users through the municipalities’ neighborhoods and highlighting places relevant to the Story via audio narration. The experience unfolds through a series of cards, which will feel familiar to users of Instagram Stories. Those who wish to deepen their exploration can do so with granular analysis capabilities, replete with data visualizations. Lastly, as a send–off, users are invited to participate through contributing tips to maintain the accuracy of data, or by simply signing up to receive notifications for new features and Stories.
Stories’ initial prototype was developed for Milan. The 15 Minute City concept served as a source of inspiration and a framework for ideating location–based narratives within the city (incidentally, the concept is also available as one of four options to activate the storytelling functionality).
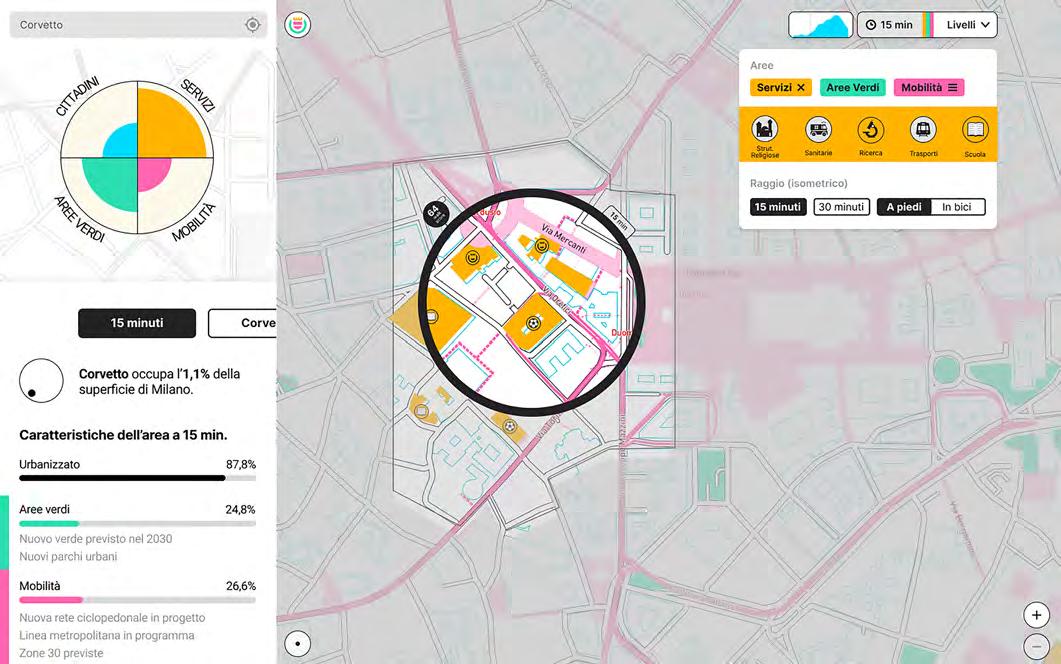
Figure 3 Series of screenshots from the Stories App From top to bottom / left to right: list themes - geolocation - filter content - play story - deepening - NIL comparision 59
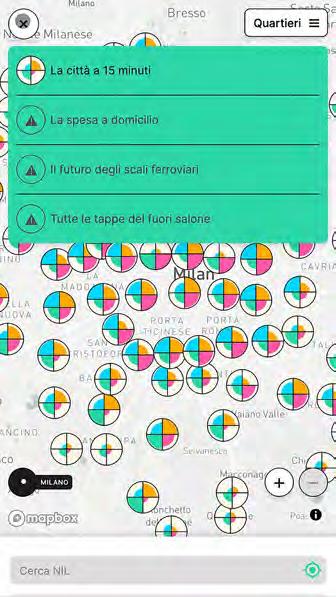
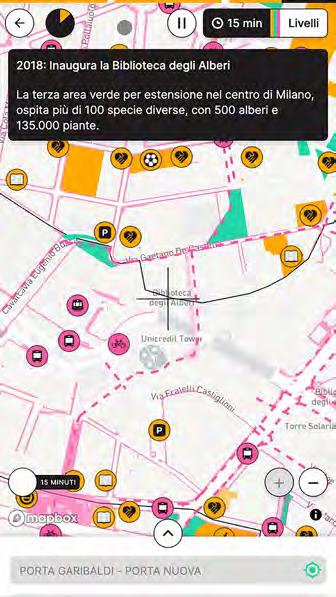
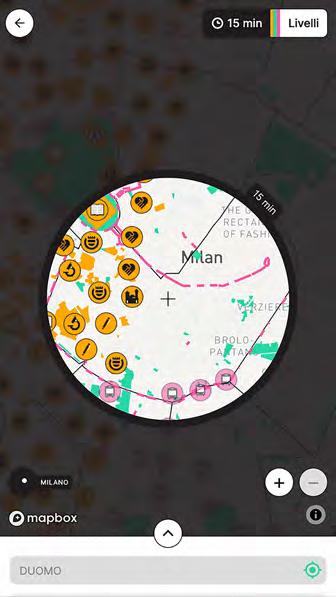
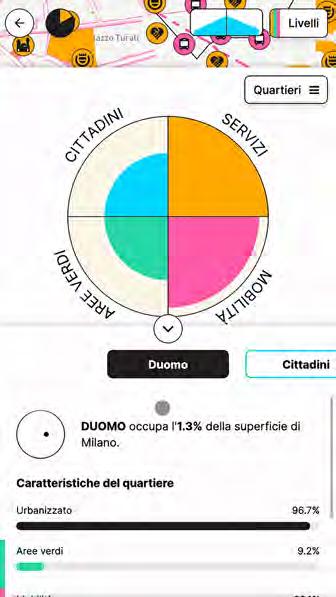
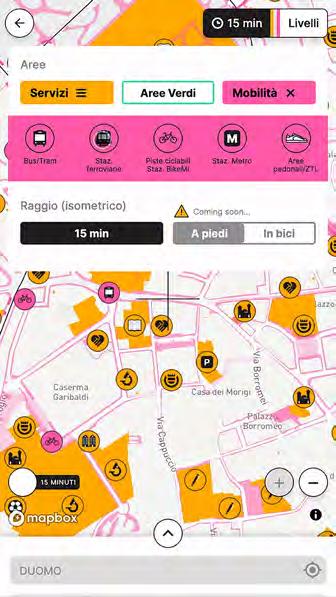
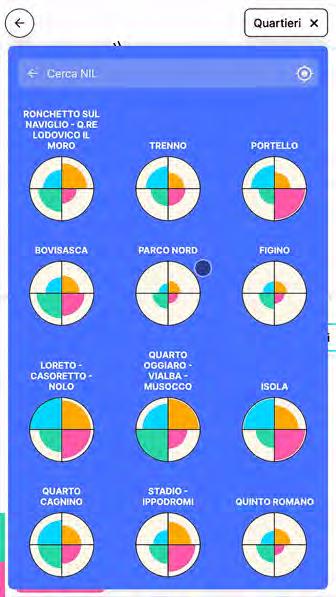
One of the main goals of European cities is to promote active modes of travel providing Sustainable Urban Mobility Plans or SUMPs for short (Buhrmann et al., 2019). Within a short time frame and following the impact of the Covid-19 pandemic, city administrations have adopted and implemented short and long-term plans to redistribute vehicular road space towards cyclist and pedestrian infrastructure. Milan, a city that was hit hard since the outbreak of the virus becoming one of the early epicentres of the pandemic is nowadays one of the forefront cities embracing this approach, adding 35 kilometres of cycle lanes in the city center under its Open Streets plan (Comune di Milano and AMAT Agenzia Mobilità Ambiente Territorio, 2020). In Milan as around the world, initiatives showing clear aims to favour pedestrian movement and active travel options quickly rose to the top of local mobility agendas.
According to the Territorial Government Plan of the Municipality of Milan (Comune di Milano, 2019), the territory of the City of Milan is divided into 88 Neighborhoods or Nuclei of Local Identity (NIL): a system of areas connected by means of mobility infrastructures and services, and characterized by urban vitality, distinctive features, historical heritage, but also by ongoing renovation projects. Thus, NIL have been considered as the most appropriate spatial units for the proposed GIS analysis, in terms of historical peculiarity, granularity, average area.
The unprecedented disruption caused by the Covid-19 pandemic has given rise to a large cultural and technical debate on upcoming forms of urban development and mobility, in the attempt to envision potential trajectories of new paradigms and keep up with the pace of our changing cities. City administrations around the world are recognizing walkability, universal accessibility, public realm and living locally as crucial planning dimensions. In particular, the recent focus on the "15-minute city" model represents a new perspective for evaluating sustainable, inclusive, and resilient urban organisms, meeting the human desire for community and place identity. The model envisions access to the urban life experience, such as essential facilities and services, in a timeframe of 15 minutes from home, by walking or cycling, as defined by Moreno et al. (2021). The recent pandemic clearly showed this potential as soft mobility modes were allowed for social and recreational life even during lockdown periods. Having said that, encouraging the shift towards a sustainable urban mobility with focus on public transport, shared-micro mobility, and active modes such as walking and cycling is one of the main challenges European cities nowadays have to face (Buhrmann et al., 2019).
In particular, the selected datasets were analyzed considering the spatial distribution of punctual and areal vectors on NIL. For comparing the various indicators among them, each one has been normalized on a 0-100 scale, creating Z-scores that follow the normal distribution of the values.
60
The methodological approach which sets the current research is based on the application of Geographic Information Systems (GIS) for the analysis of a series of relevant location-based data which were retrieved, sorted and filtered from the Geoportal of the City of Milan2
Among all possible urban challenges, a recent focus on walkability, which contributes to the quality of life of its citizens by enhancing physical activity, well-being, and social inclusion (Speck, 2013) is shaking all advanced urban planning activities. Taking up this challenge, cities worldwide are improving pedestrian mobility, including barrier-free and safe sidewalks, but also human-scale environments which allow people to enjoy walking and gather in comfort (Gehl, 2013).
Despite recent efforts towards sustainable and inclusive mobility in urban contexts, the needs of vulnerable city users are still largely overlooked and/or not explicitly addressed through mainstream planning instruments.
As highlighted by the 2030 Agenda for Sustainable Development adopted by all United Nations Member States (i.e., SDG 11.2-Sustainable Transport for All), public transport should be designed to be inclusive of diverse types of users: "By 2030, [transport systems should] provide access to safe, affordable, accessible and sustainable transport systems for all, improving road safety, notably by expanding public transport, with special attention to the needs of those in vulnerable situations, women, children, persons with disabilities and older persons" (United Nations, 2016, p. 24).
The retrieved open data were used to design a series of multi-layered maps of the City of Milan, considering the territorial boundaries of the above described NIL. This was aimed at estimating the spatial distribution of each data set, and to identify and characterize the NIL where to prioritize interventions focusing on the accessibility of relevant services within a comfortable walking distance.
How Covid-19 Impacted Urban Mobility and Everyday Life
Open Data for Unlocking Hidden City Metrics
A user-centered approach to mobility planning is central to the Stories App vision. Customized transit planning is etched into the DNA of the project with the aim to ensure transport inclusivity and promote equal access to opportunity in the city.
Finally, the considered dataset for the calculation of the Mobility Index spans several domains: (i) subway stations, railway stations, and local public transport stops (TP); (ii) cycling infrastructures (CI); (iii) sidewalk infrastructures (SI). This was based on estimating the number of inhabitants and workers served by transport services and infrastructures, and on the spatial distribution of cycling (m) and sidewalk infrastructure (m). Then, the index was defined by the weighted summation of the Z-scores of each preliminary sensitivity analysis on the considered data, the constant parameters KTP (corresponding to 0.8), KCI (corresponding to 0.1), and KSIS (corresponding to 0.1) were weighted to accentuate the impact of subway, railway and local public transport service on the proposed index (Σ constant parameters = 1).
Scalability and integration of the app in future research projects
In particular, neighborhoods characterized by a lack of accessibility of proximity services, green areas and public services and infrastructures could be further investigated through audit tools and survey questionnaires focused on the subjective evaluations of the city users about the level of walkability of a specific area.
The research represents a preliminary step aimed at identifying the areas of the City of Milan where it is suitable to execute a series of data collection activities.
One of the next steps will be aimed at transforming the results of the proposed in-depth data collection and analysis activities into an interactive communication tool, which could raise public awareness of the research findings. Thus, results will be exploited by using opensource platforms for data visualization to produce webbased, fully accessible and interactive thematic maps. Moreover, the app will include new functionalities related crowd source collaborative mapping through the direct contribution of the users, based on the possibility to incorporate feedback from the users and to collect data about their mobility patterns (Bolognesi and Galli, 2017).
In conclusion, the study represents a valuable example of the possibility to analyze digitally widespread open data sources to support decision-makers. According to the 2030 UN Agenda for Sustainable Development (i.e. SDG 11.2-Sustainable Transport for All), the presented results could be of notable interest to public institutions involved in the design of sustainable and inclusive mobility strategies.
The Stories App project was executed in collaboration with Accurat. The team thanks Comune di Milano and Agenzia Mobilità Ambiente Territorio for their support.
Stories is built to scale to accommodate environments big and small, spread–out and dense. Its application is equally relevant to college campuses and metropolitan areas even bigger than Milan. Additionally, beyond opportunities in the public sector, Stories can be sponsored by private entities. The 2022 Olympics committee, for instance, could leverage Stories for the 2022 games in Milan and Cortina. Other types of companies that rely on users to self–navigate might find value in Stories’ potential for customization. Sponsors are invited to contribute expertise as material for audio narration. A museum seeking to highlight particular collection items, or a real estate firm looking to give users an overview of a neighborhood, are prime examples.
61
Throughdata:a
Data analysis started from the definition of four indexes, as follows: 1. Demographics; 2. Proximity services; 3. Green areas; 4. TheMobility.calculation of the Demographics Index was based on the density distribution (inhab/sqm) of the number of inhabitants living in the census sections of each NIL. The Proximity Services Index was based instead on the spatial distribution of social, religious, educational, security, health, administrative, and cultural services on each NIL. The Green Areas Index was based on estimating the aerial extension (sqm) of public parks and gardens on each NIL.
The results of the analysis are then visualized considering both the proposed indexes and the possibility to explore the localization of each layer through an isometric buffer of 1 km, which approximately corresponds to 15 minutes by walking.
Users of Stories encounter the fruits of these calculations as objective scores, visualized within iconography that calls to mind navigational tools, such as a compass or sonar radar. The data visualizations - circular discs divided into four, color-coded sections - display an area’s performance across the four indexes. The consolidated visualization also allows users to easily weigh a location’s performance in one index versus another.
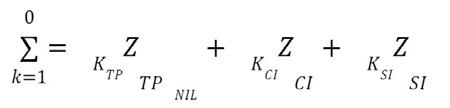
Chapter 3 METRICSURBANNEW 62
The development of new technologies is shaping the way we read and plan our cities. indispensibletheseongoingcausedmobilityunprecedentedInurbanforpossibilitiesofgrowingcombinedtechnologyWheniswiththeavailabilityopendata,newemergeunderstandingconditions.thecontextofdisruptionbythepandemic,toolsbecomefor quick, effective and widescale analysis. METRICSURBANNEW 63
64
The results of the analysis, which focused on traffic data collected at Corso Buenos Aires – one of the longest and most well-known retail streets in Europe - outline patterns in the street use, with particular attention to the Covid-19 lockdown periods, and highlight trends in sidewalk choice due to thermal comfort, depicting the average monthly share of pedestrians per sidewalk and the mean detected temperature.
The development of new technologies is shaping the way we read and plan cities. When technologies such as Big Data and Artificial Intelligence (AI) are combined with the growing availability of open data, new possibilities emerge to develop new understandings of the urban fabric and use. These technologies are particularly useful in the context of emergency situations, such as that of the Covid-19 pandemic as they help in quickly understanding the shape and impact of mobility disruption.
This project focuses on the analysis of pre-trained deep learning models - a subset of machine learning algorithms – for object detection, image segmentation and crowd counting and on their applications on research topics linked to the understanding of temporal patterns related to the development of Covid-19, climate analysis and thermal comfort, and their integration with transport modelling.
Looking
SpacesPatternsUnderstandingMachinewithEyes:inUrban
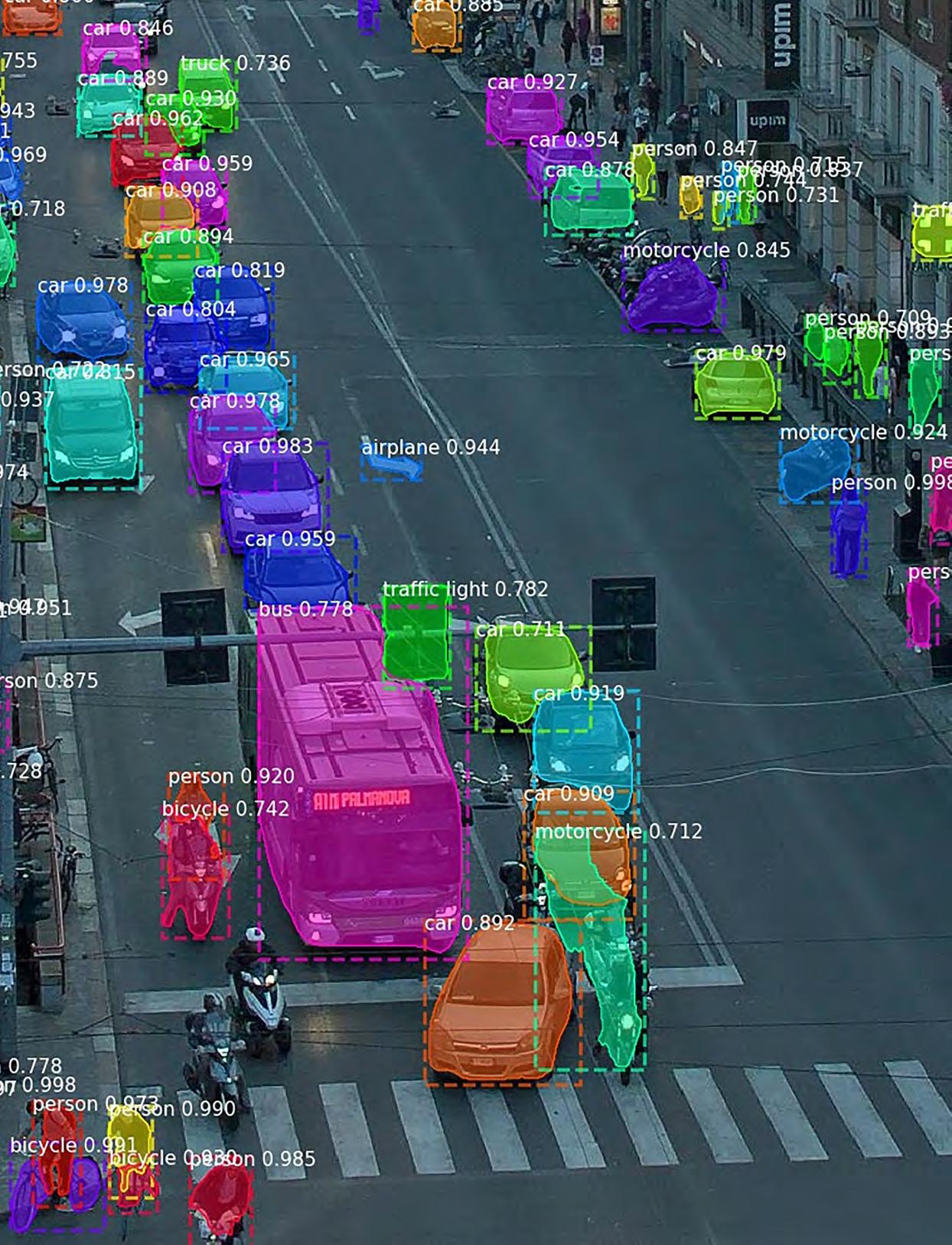
People/Bicycle/Motorcycle/Car Mask R-CNN1008060402001/9/1927/9/1923/10/1918/11/1912/12/199/1/204/2/20 22/4/201/3/2027/3/20 18/5/2013/6/209/7/20 4/8/2030/8/20 Car Motorcycle Bicycle People Figure 1 Main temporal trends and patterns for each mode Table 1 Algorthms tested within phase 1 Table 2 Mean deviation between automatic and manual counts for each algorithm
Furthermore, in the past years, the availability of pretrained weights for deep learning algorithms has grown. Dataset and First Steps
Deep learning algorithms are of particular interest in the field of computer vision, enabling the manipulation of large datasets in an automatic way. In the past years, the rising availability of deep learning techniques led to new frontiers in the automatic understanding of images and estimating the number of objects within an image. Deep learning methods obtained state-of-the-art results for image classification, object detection (Zhao et al., 2019) and instance/semantic segmentation tasks.
The study area centers around Corso Buenos Aires, Milan, one of the longest and most well-known retail streets in Europe (Transform Transport, 2018). The dataset consists of a collection of images (from MilanoCam4) depicting the street with a strong perspective view, showing the flow of vehicles and people from Piazza Lima to Porta Venezia. These are taken at one-hour intervals, with a high potential for comparative analyses of changes in the street use behavior at peak times and different seasons.
65
The current study builds on previous research presented in Shifting Paradigm 1st edition, which was structured in two phases. In the first phase, a set of algorithms with pre-trained weights were selected based on their popularity and tested on a selection of 16 images. The results were then measured against the manual counts performed on each image. The goal was to define the usability of these models out-of-the-box, for the given dataset. Table 1 outlines algorithms tested within Phase 1 of the research and Table 2 lists the mean deviation between automatic counts and manual ones for each algorithm, computed as: In the second step, the analysis of the images for a yearlong period was carried out. The initial focus was on the performance of the algorithms with respect to weather and light conditions as well as business activity of the Finally,street.the study aimed to identify the patterns in the street use every day at 19:00, the moment when people leave their offices, with particular attention to the Covid-19 lockdown period. Figure 1 outlines the main temporal trends and patterns for each mode.
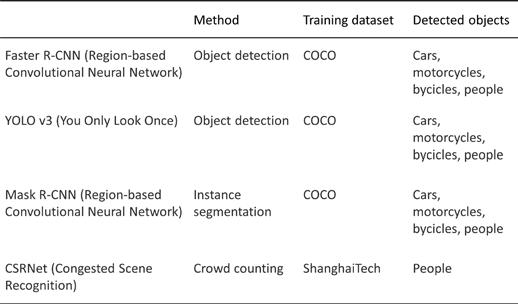
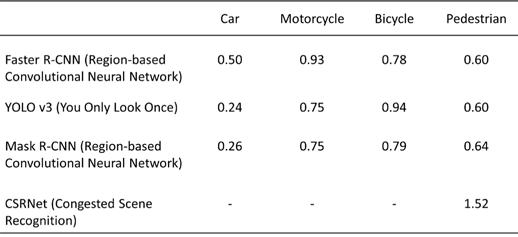
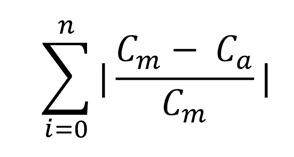
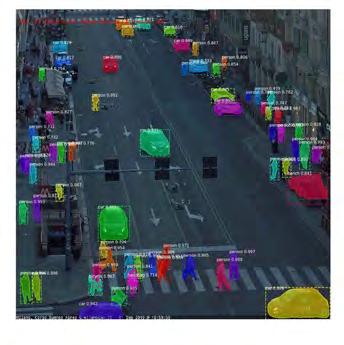
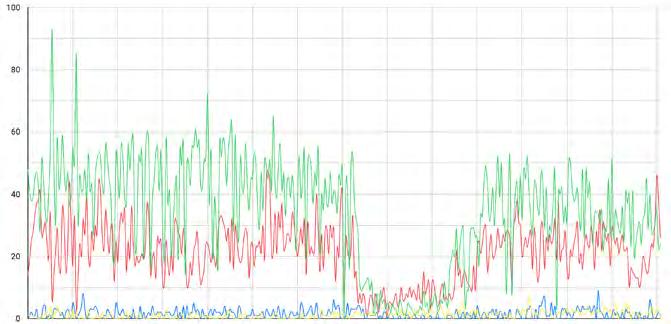
An open-source model was used to detect objects in the images, which was trained on CCTV cameras in Montreal. The dataset represents vehicles, pedestrians, constructions, cyclists, and bus instances, obtaining a mean Average Precision (mAP) equal to 0.809 in pedestrians’ recognition on the original training set (City of Montreal, 2021). Figure 2 shows results on a sample image of Corso Buenos Aires.
Figure 2
Sample image of Corso Buenos Aires Data and Tools
Analysis Building on knowledge obtained from previous research, the study was then structured in four steps, which focus on the analysis of pedestrian patterns in Corso Buenos Aires with increasing temporal granularity (i.e., months, day types, hours) and spatial accuracy (i.e., whole image, sidewalks).
Figure 5 shows how splitting 2019 data into 5 random groups leads to a deviation from the mean lower than 5% for all moments of the day, with an exception for 7 and 8 am. This may be due to differences in footfall patterns during the weekday and weekends. Instead, splitting data into 5 random groups leads to a higher deviation from the mean, above the 5% threshold at multiple moments throughout the day.
66
The first one aimed at quantifying the deviations of the model’s performance for different weather and lighting conditions, identifying systematic inaccuracies in the detections. Here, a manual validation methodology was implemented on 10% of all images, comparing the amount of automatically detected pedestrians with those counted manually. Results shown in Figure 4 demonstrate how darkness and rain worsen overall accuracy, leading to a mean absolute difference with manual counts above 10%. Instead, shadow and sun conditions affect results slightly, leading to a mean absolute difference equal to roughly 7.5%.
First, an exploratory analysis was performed on the dataset (see Figure 3). The goal was to visualize overall trends in registered pedestrian footfall in 2019. Figure 3 shows average monthly profiles for weekdays and weekends. These present expected patterns, with anomalies in June and September, when extraordinary events (i.e., Fashion week, Giro d’Italia, etc.) occurred in the street. Then, the internal validity of the dataset was assessed from two perspectives.
First, a mean hourly number of detected pedestrians was computed. This should be the baseline value for comparisons. Then, hourly detections were grouped in random clusters. For each, the mean hourly value was computed to assess its deviation from the mean value.
In the last phase, an extensive dataset of 14,956 images was constructed, representing 12 daily timeslots (6am – 10pm) between January 2019 and May 2021. These were then analyzed on the fly using Yolov5 (You Only Look Once) (Jocher et al., 2021) as an object detection algorithm. The goal was to recognize pedestrians and locate them in an image. YOLOv5 represents an evolution of the YOLO architecture, which enables faster and more accurate class predictions. This architecture relies on CSPDarknet53 as the backbone and PAN as an aggregator level. Furthermore, it uses image augmentation methods in training to improve accuracy in results (Gutta, 2021).
The second validity check aimed at defining the reliability of the dataset through a sensitivity analysis on data robustness and outliers. Data for the year 2019 was analyzed to ensure consistency in the analysis.
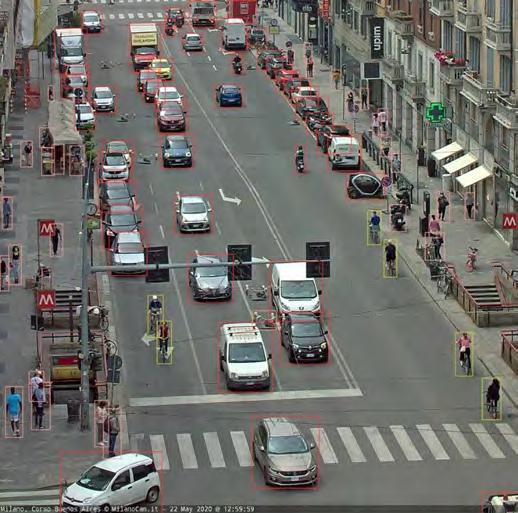
0 - Workdays 1 - Weekdays Mean absolute difference – 8% Optimal conditions Low sun light, bright colors, homogeneous pedestrian distribution on sidewalks Critical conditions Reflection of lights, high contrast, dark images, high pedestrian densities on sidewalks Random groups Deviation from mean Figure 3 Preliminary analyses & data visualization Workdays and Weekends, Pedestrians, 2019 Figure 4 Definition of systematic inaccuracies in outputs – how images are affected by lighting and weather condition Figure 5 Representativity study - minimum number of images to describe a moment within a fixed deviation from the mean value and outliers' impact 67
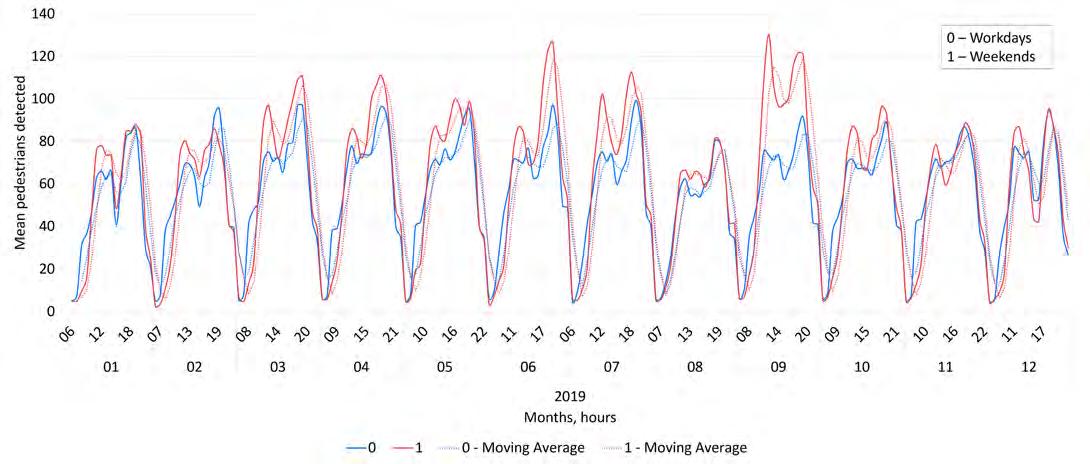
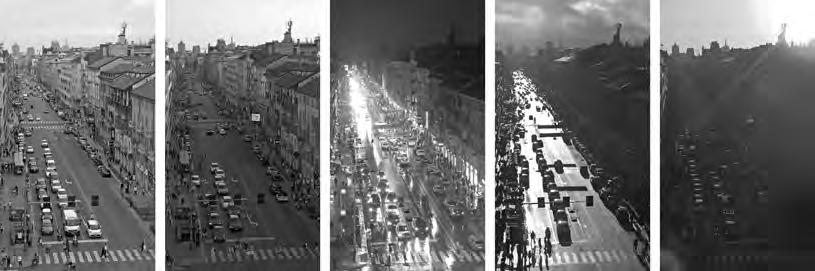
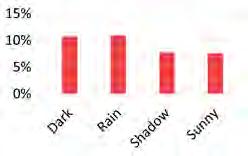
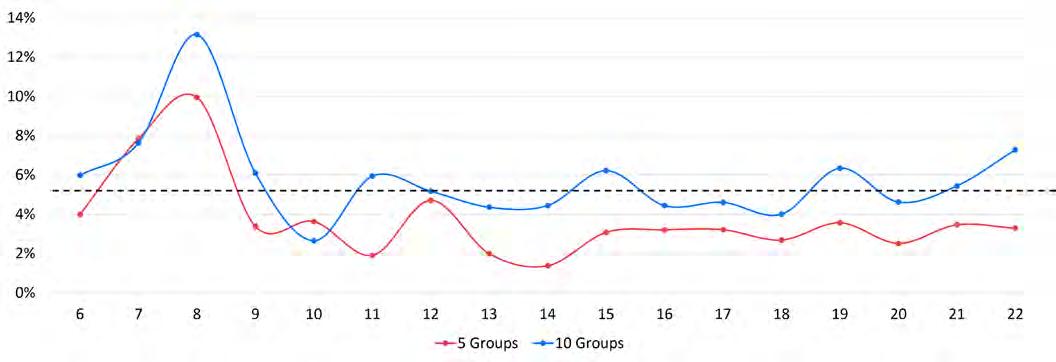
Covid-19 monitoring Daily pedestrian counts through Covid-19 restriction phases
Figure 7 outlines detected pedestrian volume trends by day type and hour. The top graph shows how mean pedestrian counts never reached pre-covid levels during the study period, with slight differences registered between “necessity” and “soft” restrictions phases. The bottom graph depicts hourly patterns for each restriction phase. Lower volumes were registered at lunch time and in the evening in “necessity” phases, while higher volumes can be seen in the weekends during “soft” phases. Furthermore, the effects of the curfew implementation in the city at 10pm can be seen from “necessity 2” to “necessity 4” phases.
Figure 6
The second phase of the study investigated specific patterns in the use of Corso Buenos Aires. The dataset was divided into five subsets, considering the Covid-19 pandemic restrictions. Then, hourly trends for workdays and weekends and for different day types were studied and compared among different restriction phases (see Figure 6). This analysis aimed at extending the one carried out in the first phase, with a focus on post lockdown restrictions. These can be classified as "necessity" restrictions (namely, movements were limited to necessity reasons, as work and grocery shopping), and "soft" restrictions (namely, leisure movements were allowed, such as shopping). The goal was to determine whether differences among the two phases could be recognized by means of pedestrian counting. Figure 6 gives an overview of the restriction phases and of the overall footfall trends.
68
The third step of the study consists in converting instantaneous pedestrian densities captured in images into pedestrian flow and related walking speed. To do so, pedestrian fundamental diagrams, specifically derived from an innovative application of dynamic microscopic model, were applied on the detections. The Social Force Model represents pedestrians as particles subject to forces (i.e., attraction and repulsion) in an analogy with fluid dynamics, quantifying pedestrian flows under business-as-usual conditions. The Social Distancing Model is a modification of the default parameters to simulate social distancing conditions (Espitia et al., 2022).
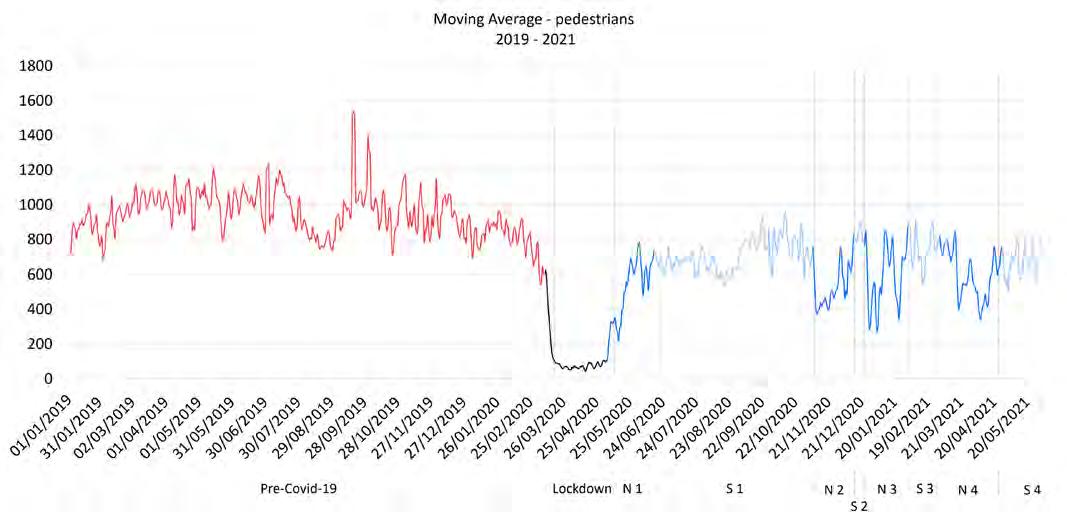
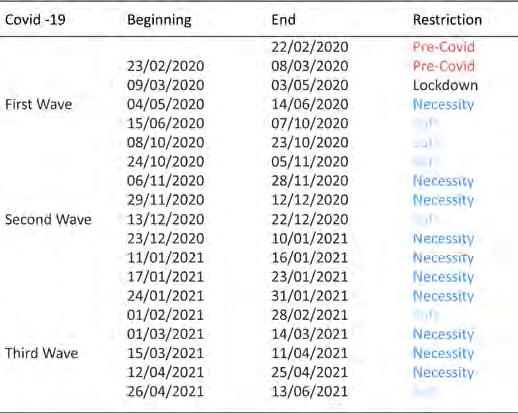
52% pedestrian volume occurrence is above 35 Figure 7 Covid-19 monitoring Daily pedestrian counts through Covid-19 restriction phases Figure 8 Transport modelling integration
69
As can be seen, in 52% of the images in the dataset, the number of detected pedestrians would lead to a difference between business-as-usual and social distancing hourly flows greater than 8%. While images cannot be used to compute hourly flow, the graph gives insights on possible flows scenarios in different crowding conditions in Corso Buenos Aires, enabling a visual comparison with the occurrences of detected densities in images.
Figure 8 outlines the preliminary results on the application of these models to determine the overall influence of social distancing in the study area. The graph depicts Social Force Model and Social Distancing Model curves (red and blue curves), which quantify the hourly pedestrian flow in Corso Buenos Aires, given increasing pedestrian densities (x axis). These can be compared with the occurrences of the detected densities in the image dataset (black curve).
Definition of conditions that determine decrease in pedestrian flow

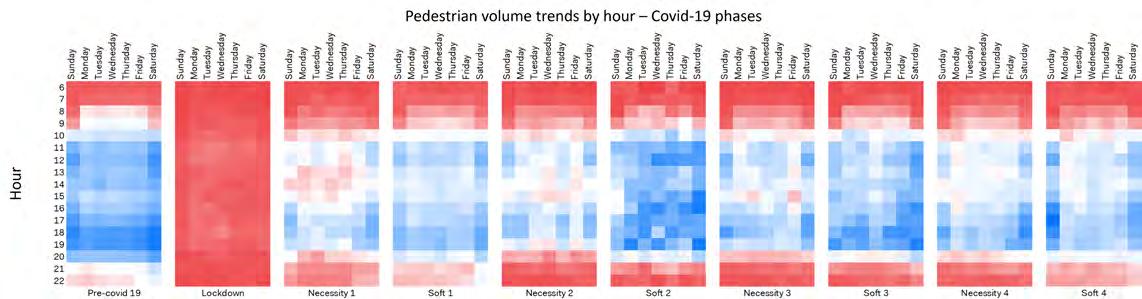
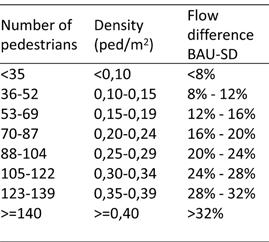
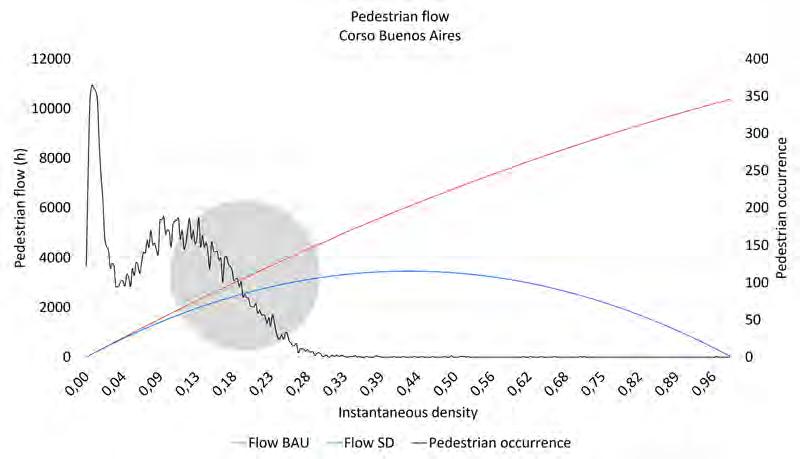
Figure 10 Climate analysis: Analysis of shading conditions in Corso Buenos Aires on August 6th, 2021
Lastly, a preliminary analysis on pedestrians’ distribution on sideways was carried out. As Corso Buenos Aires has a north-south orientation, its shading conditions change gradually during the day, allowing for a thorough pedestrian comfort study. In this phase, temperature and cloud conditions were researched by using an opensource meteorological dataset. These may influence the sidewalk choice. Figure 9 shows the shading conditions in Corso Buenos Aires on August 6th, 2021, between 12am and 5pm. The left sidewalk (east) is in the shade from 12am to 2pm, while the right one (west) is shaded from 3pm to 6pm.
Figure 9
Climate analysis: Images showing shading conditions in Corso Buenos Aires on August 6th, 2021
70
In this phase, images were masked to detect pedestrians on the sidewalk of interest. Then, the share of pedestrians for each sidewalk was computed to obtain a comparable metric. Figure 10 outlines trends in sidewalk choice in 2019, depicting the average monthly share of pedestrians per sidewalk and the mean detected temperature. While a general preference for the left sidewalk can be observed, curves abruptly change between 2 and 3 pm in summer months with temperatures above 30° Celsius. These patterns are consistent with 2020 ones, proving potential for further analyses (see Figure 11).
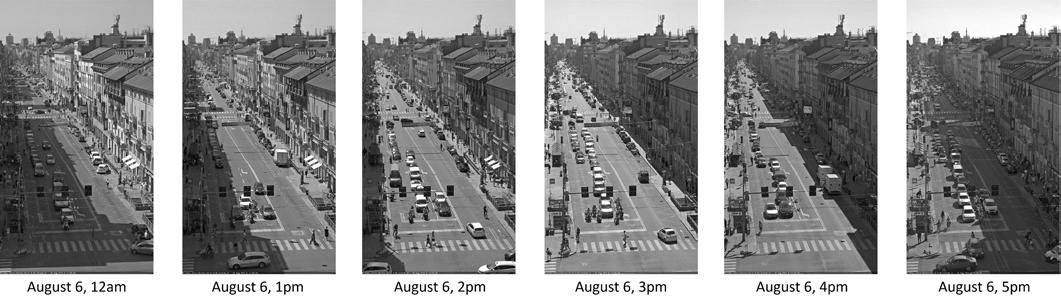
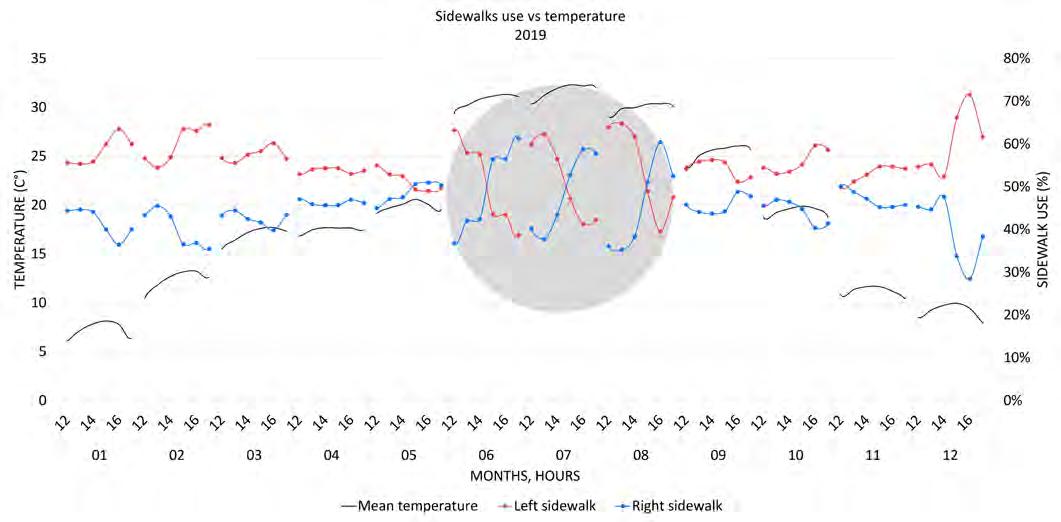
This research aims at studying different methods to identify and count objects in images. The goal is to understand the potential of each method with available pre-trained weights. Then, the purpose is to analyse the street use of Corso Buenos Aires by means of ongoing studies.
Future works include the growing integration of modelling outputs with real data, the replication and forecast of complex pedestrian patterns at both urban district and city levels, integrating multiple datasets and deepening the study on pedestrian comfort in different environmental conditions. An example is represented by the usage of this information and other data from different sources (wi-fi hot spots, mobile and telco data, etc.) to implement innovative predictive models able to replicate and forecast complex pedestrian flow patterns at both urban district and city levels, as implemented in the city of Melbourne (City of Melbourne, 2021) and in the city of Liverpool, Australia (Barthélemy et al., 2019).
Figure 11 Climate analysis: Sidewalk patterns, Pedestrians, May – September, 2019 - 2020 Conclusions and Future Research
71
The evolution in results obtained between the first steps and phase three highlight possibilities in development of new applications and studies involving image datasets. In fact, while models that were trained on broad scope datasets (i.e., COCO) (Lin et al., 2014) would lead to modest results for small instances (i.e., pedestrians and cyclists), results showed a great improvement using a model specifically trained on the classes of interest (i.e., vehicles, pedestrians, cyclists) and on images with similar orientation. Further improvements could be obtained through data augmentation, limiting the effects of meteorological factors on images quality, and by finetuning the open-source models. In general, the methodology proves to be time and costefficient with respect to the level of accuracy and details inferred while guaranteeing the privacy of individuals (Bernas et al., 2018). The use of an extensive dataset led to statistically significant results on aggregated temporal periods, overcoming the uncertainties of a single image. Furthermore, it gives the possibility to detect meaningful moments to be further analyzed by means of video analytics.
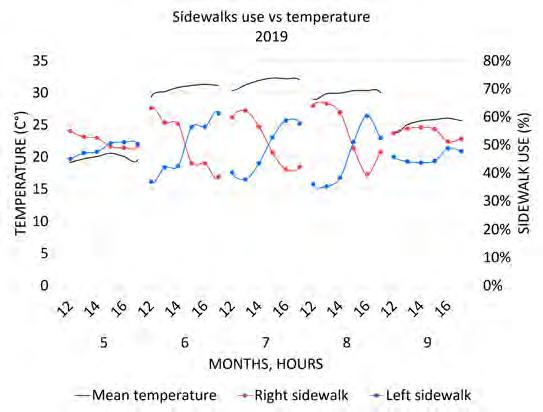
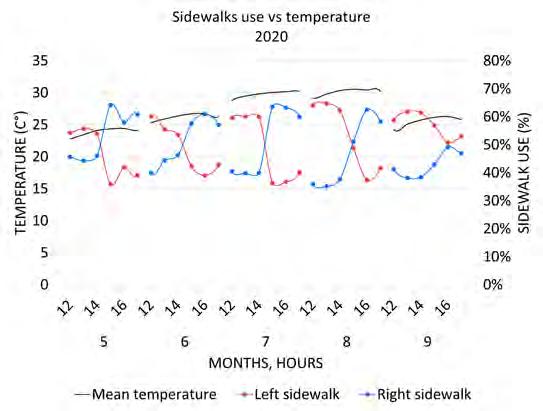
Patterns in Milan Through Wi-Fi Sensors Data
The recent development of location detection systems enables the monitoring, understanding and prediction of activity patterns of city users. The Internet of Things (IoT), in particular, is a valuable city resource that when adequately installed across the city, can provide valuable insights into the daily movements of urban users. This infrastructure becomes a strong asset in times of abrupt change, where unpredictable activity patterns could be monitored and analyzed to provide deep insights about user behavior. In this framework, the following research focuses on the analysis of a sample of aggregated traffic data based on the number of mobile devices detected through a network of 55 Wi-Fi Access Points in Milan. Data was collected over a period of 7 months (January to July 2020), allowing for a study on the impact of the Covid-19 pandemic on activity patterns. Data analysis was based on merging: (i) time series analysis of trends, peak hours and mobility profiles; (ii) GIS-based spatial analysis of land data and public transport data. Results showed a significant correlation between Wi-Fi data and the density distribution of residential buildings, service and transportation facilities, entertainment, financial amenities, department stores and bike-sharing docking stations. In this context, a Suitability Analysis Index was proposed, identifying the areas of Milan which could be better monitored through the installation of additional Wi-Fi sensors.
72
Monitoring Activity
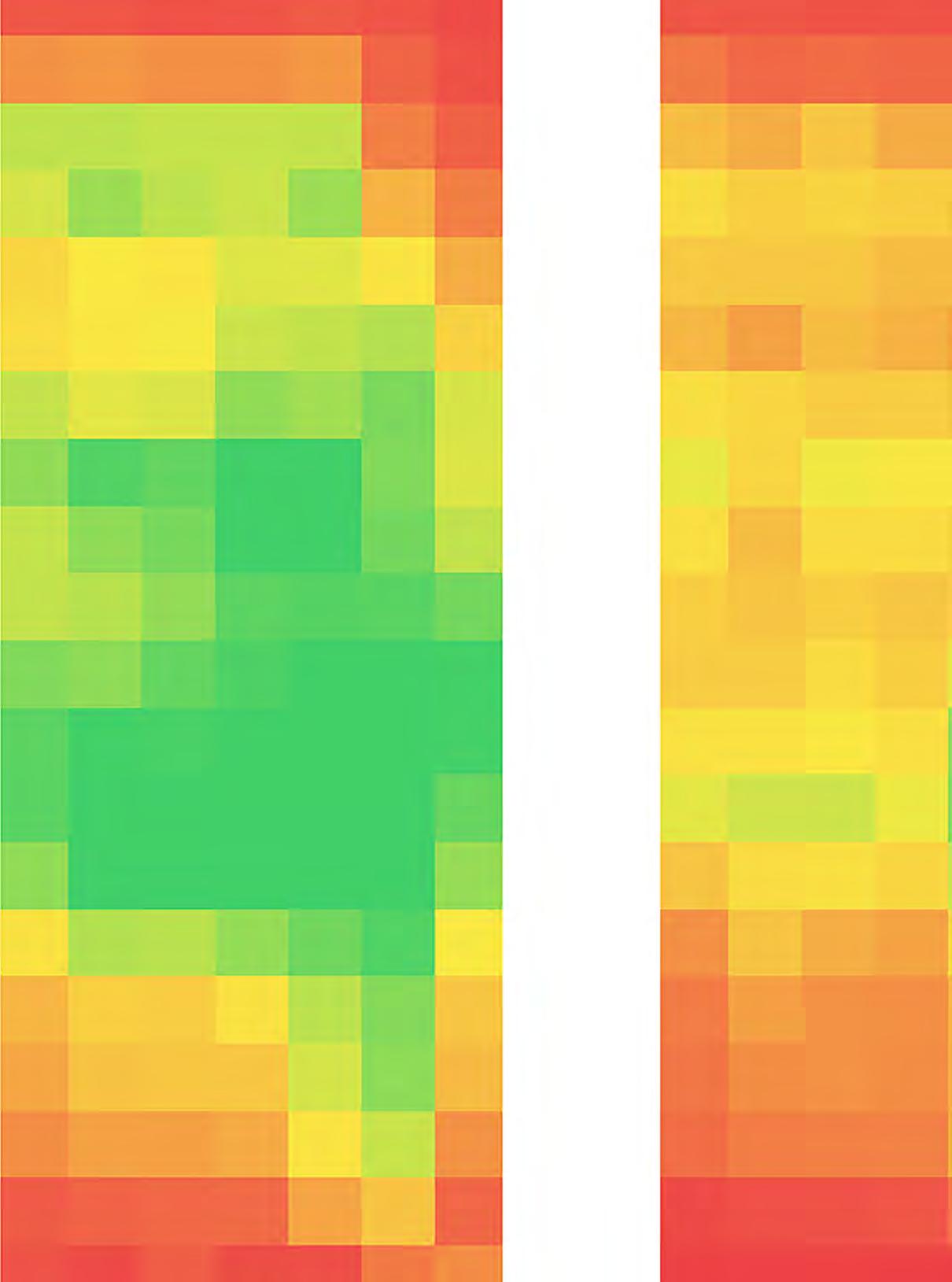
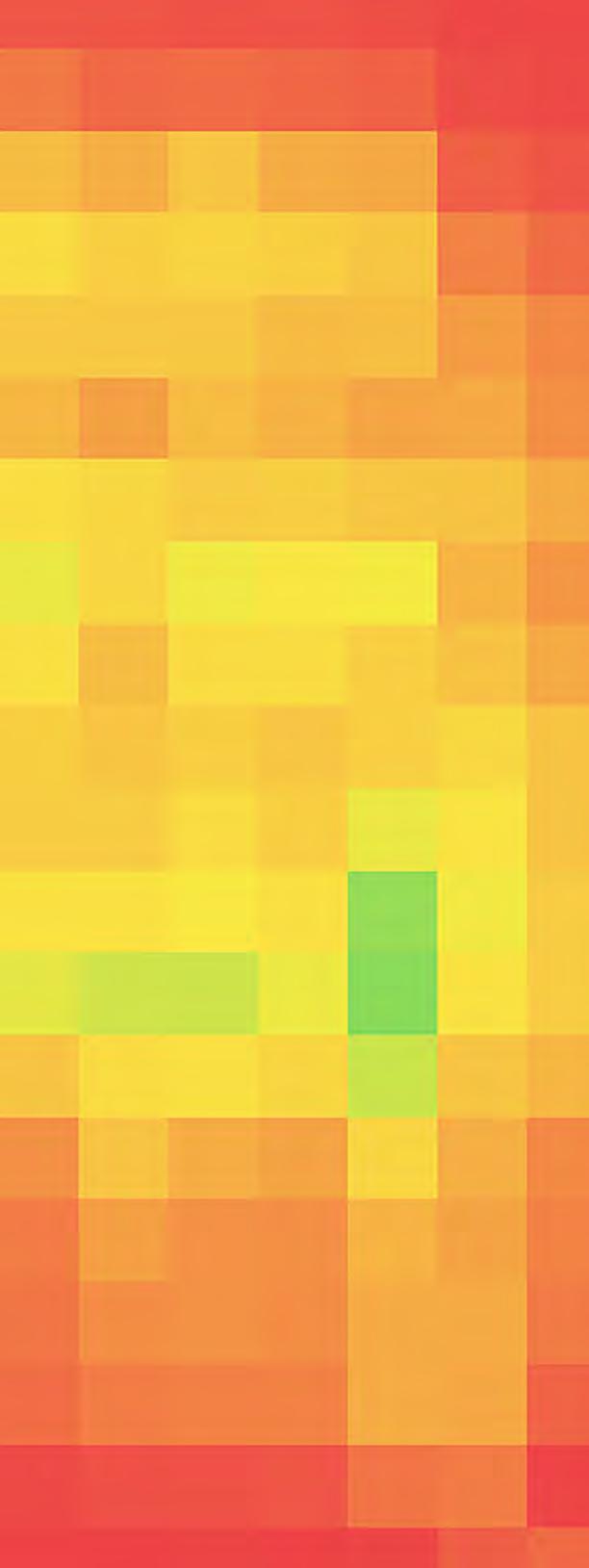
In this framework, the research is based on the analysis of a large sample of structured proprietary data gathered during a 7-month period (January-July 2020) through a network of 55 Wi-Fi sensors distributed in several department stores, shops and public services in Milan, Italy. The objective of the analysis is twofold. First, the research aimed to test the effectiveness and reliability of Wi-Fi technology to collect aggregated data about activity patterns in large-scale urban scenarios. Second, the research aimed to correlate the number of detected mobile devices (i.e., time-variant data) with the density distribution of relevant land and public transport data (i.e., time-invariant data) such as building typologies, green areas, amenities and public transport. Moreover, the results of the analysis were used to define a Suitability Analysis Index, aiming to identify the areas of Milan that could be exploited for more extensive data collection campaigns by means of the installation of additional Wi-Fi sensors.
Thanks to the collaboration with the Wi-Fi service provider FreeLuna5, a large sample of proprietary data was collected through a network of 55 Wi-Fi sensors (see Table 1) from the beginning of January 2020 to the end of July 2020. First, the proposed time series analysis was focused on the number of mobile devices detected per day per hour through the Wi-Fi sensors, in order to highlight trends, peak hours and mobility profiles during the entire period of reference. Then, results were compared against an extensive GIS-based analysis focused on relevant land and public transport data (see Table 1), which were retrieved, sorted and filtered from geoportals and open data repositories. This information was analyzed to design a multi-layer map of Milan and to estimate the spatial distribution of each dataset considering the localization of the Wi-Fi Access Point (APs).
A catchment area with a radius of 280 m (circular buffer areas of 0.246 km2) was defined, considering the radius of action of installed Wi-Fi sensors (80 m) and the travel distance allowed from place of residence within the restricted territories during the first phase of the lockdown measures (200 m), according to the national regulation enforced by the Italian Government6
• Images stream analysis and acquisition systems (Buch et al., 2011): AI and computer vision techniques for processing a sequence of photographs or a video clip collected through CCTV cameras or drones;
• Software development kit, Beacon and bid stream systems (Lin and Hsu, 2014): data derived from GPS signal of navigation devices and Apps for smartphones;
• Cell network data acquisition systems (Schläpfer et al., 2021): data derived from SIM cards and cell network technology;
Urban Informatics (Foth et al., 2011) provides innovative assessment tools and metrics to support an effective planning of mobility services, within an evidencedbased and multi-disciplinary approach. Thanks to the recent development of advanced ICT solutions and the increasing availability of digitally widespread data sources, Big Data is becoming a valuable tool to unveil hidden mobility patterns in cities (Batty, 2013; Crist et al., 2015). In particular, the development of location detection systems allows us to monitor, understand and predict the travel behavior of city users, considering both micro and macro scale scenarios (Xhafa et al., 2017). This has become even more crucial considering the need to investigate the unprecedented effects of disruption of the Covid-19 pandemic on urban mobility and to assess the effectiveness of immediate and longer-term actions that cities have developed to respond to the crisis (Deponte et al., 2020; European Platform on Sustainable Urban Mobility Plans, 2020). Data collection techniques include the following:
• Radio frequency systems based on Wi-Fi or Bluetooth technology (Sapiezynski et al., 2015): antenna type sensors for scanning wireless devices through the MAC address;
The proposed GIS analysis was based on various attributes and characteristics of the urban area surrounding each Wi-Fi sensor. To do so, raw data related to the urban scale were extracted about surrounding areas of each Wi-Fi AP (see Figure 1).
• Occupancy Detection Systems (Odat et al., 2017): light pulse type remote detection techniques, including LIDAR and PIR sensors.
Urban Informatics and Mobility Patterns
Enabling Data and Methodology
73
A_Su_caA_DS_caA_Sh_caA_He_caA_Fi_caA_En_caA_Ed_caNReB_ST_caNReB_In_caNReB_Co_caNReB_Ad_caReB_ca PT_Su_caPT_Su_caPT_Bi_caGA_ca (stops) PT_Tr_caPT_Tr_ca(stops)PT_Bu_caPT_Bu_ca(stops) Amenities Residential and Non-residential Buildings PublicGreenTransportAreas Table 1 The list of proprietary and open datasets Figure 1 The procedure applied to extract the Land and Public Transport data on catchment areas 74
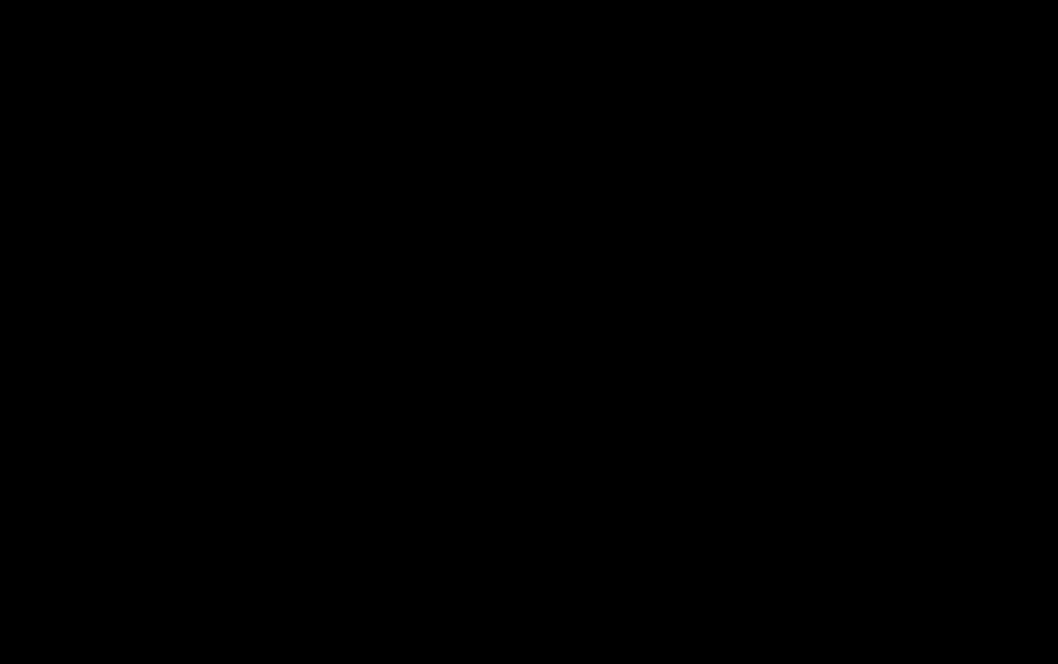
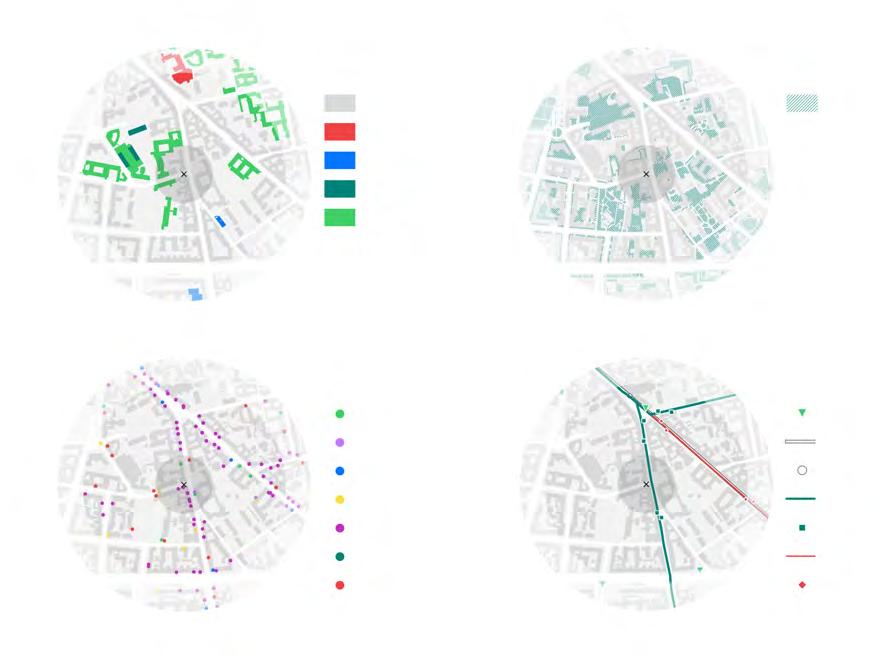
• Phase 1 (9 March 2020 - 3 May 2020): Milan was defined by the Italian government as a “red zone” along with the entire Lombardy Region. In order to avoid gatherings, the access to green areas, educational, entertainment, sport, sustenance, religious and non-essential public commercial services was prohibited. Public transport capacity was reduced to 25% to allow for social distancing.
• Phase 3 (15 June 2020 - 31 July 2020): Further easing of the containment measures took place in Phase 3. Public entertainment venues were reopened and public transport capacity was increased to 50%.
• Phase 0 (23 February 2020 - 8 March 2020): Milan was defined by the local government as a “yellow zone”. The Duomo cathedral, the Scala theatre and other cultural sites were temporarily closed, as well as schools and universities; bars and clubs closed at 6 pm.
• Phase 2 (4 May 2020 - 14 June 2020): Gradual easing of the lockdown containment measures in response to a downward trend of the epidemic curve. Green areas, sustenance, commercial and religious public services were reopened but strictly limited in terms of number of persons allowed and services hours. Public transport capacity was kept at 25%.
The analysis of Wi-Fi data trends (see Figure 3) highlighted a statistically significant difference between the datasets related to the Pre-Covid-19 period and 75
Phase23/02/2020009/03/2020Phase1 Phase04/05/20202 Phase15/06/20203 -100%-50%0%50% MA - CAMobile devices 01/01/2020 01/02/2020 01/03/2020 01/04/2020 01/05/2020 01/06/2020 01/07/2020 [Milion]DataTraffic 00.511.5 [%]CA-MA Figure 2 The analysis of Wi-Fi data trends Time-Series Analysis and Circadian Rhythm The analysis was aimed at identifying significant variations in the number of mobile devices detected through the Wi-Fi sensors during the Pre-Covid-19 period and the lockdown phases 0, 1, 2 and 3. The definition of the lockdown phases is based on the containment measures enforced by the local and national governments, which gradually prohibited any movements to, from and within the restricted territories to counter the spread of contagion.
Any movement to and from the restricted territories was prohibited, as well as within the territories themselves. Apart from health-related and emergency trips, the maximum travel distance allowed from place of residence was limited to 200 meters.
• Pre-Covid-19 period (1 January 2020 - 22 February 2020): The first Covid-19 case was confirmed in Italy on January 31st, 2020. A cluster of cases was later detected in the Lombardy Region on February 21st, 2020.
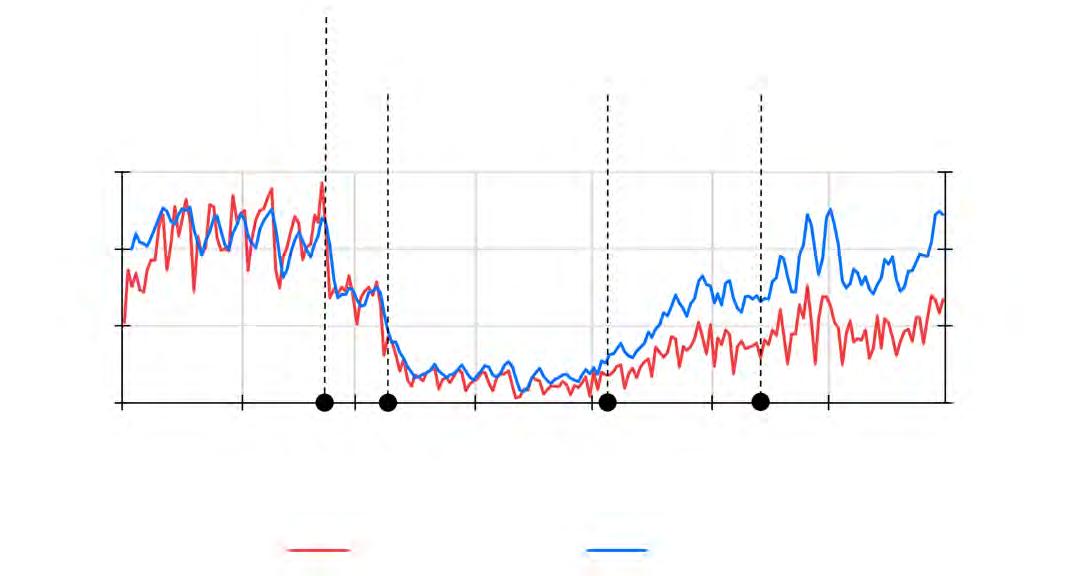
trends
The proposed extensive GIS-based analysis was based on employing spatial correlation matrices for each variable to jointly measure the dependence between the number of detected mobile devices (namely, traffic data) and the density distribution of building typologies, green areas, amenities and public transport.
The results of the analysis showed a statistically significant correlation between Wi-Fi data and the density distribution of residential buildings, service and transportation facilities, entertainment, financial amenities, department stores and bike-sharing docking stations. This was further analyzed to identify the areas of Milan that could be suitable for more extensive traffic data collection campaigns. Thus, a Suitability Analysis Index (SAI) was defined through the density distribution of relevant data on the entire territory of Milan: Results (see Figure 4) showed the areas of Milan which could be effectively exploited for extensively monitoring high traffic data by means of the installation of additional Wi-Fi sensors (areas characterized by SAI ≥ 0.8). The granularity and widespread coverage of results showed the suitability of both central and peripheral areas of the city, as well as the overlap of the modelled data with the major transport infrastructures.
Pre-Covid-19 Phase 1 Phase 2 Phase 3 Mo
Phase 0 Figure 3 The
50th perc. (971 mobile devices) 90th perc. (1430 mobile devices) Tu We Th Fr Sa Su Mo Tu We Th Fr Sa Su Mo Tu We Th Fr Sa Su Mo Tu We Th Fr Sa Su Mo Tu We Th Fr Sa Su 10th perc. (79 mobile devices) analysis of Wi-Fi data
76
the lockdown phases caused by the evolution of the containment measures put in place to counter the spread of the virus. Moreover, the number of detected mobile devices was further analyzed to characterize peak hours (i.e., circadian rhythm of the city) (see Figure 3). Results showed that the Pre-Covid-19 period was characterized by peak hours in the working day afternoons (highest peak hour on Friday afternoon at 5 pm) and on Saturday evening and Sunday afternoon. Phase 0 was characterized by an overall decrease of traffic volumes, with relative peak hours in the afternoon. Phase 1 was characterized, instead, by a flattened temporal distribution of values during the entire week. Phases 2 and 3 were characterized by an increase of traffic volumes. The analysis of weekday mobility profiles was based on the segmentation of the lockdown phases and on the calculation of the average hourly distribution of values. Results highlighted consistent peaks for the PreCovid-19 period and all phases except Phase 1 (e.g., home-to-work and home-to-school mobility patterns, occasional demand related to leisure trips, etc.).
Spatial Analysis and Suitability Analysis Index
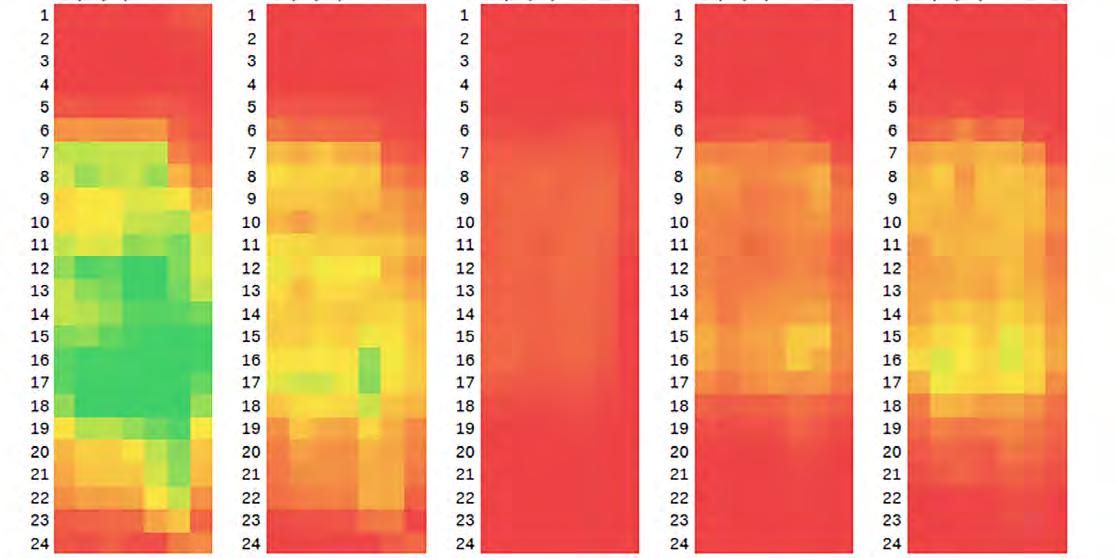
Almost 70 years after Jacobs (1961) argued for smaller and connected neighborhoods, the world is beginning to fully grasp the importance of mixed-use urban planning (Song et al., 2013). Such plans are also in keeping with the General Theory of Walkability proposed by Speck (2013), which states that the level of pedestrian friendliness of urban areas is directly related to their level of usefulness and attractiveness, pinned down in metrics of land-use mix, street connectivity and commercial density as well as polycentricity to ensure service coverage within short walkable distances. Future work will be based on the development of WiFi sensing applications for monitoring traffic data in near real-time (Li et al., 2020), considering that the triangulation of APs enables both outdoor and indoor data collection. The planned activity could support the The authors thank the team at FreeLuna for their collaboration and for sharing proprietary data.
77
managementorg/10.6093/1970-9870/7886ofcrowddynamics during big events (e.g., trade exhibitions, music, art and cultural festivals, sport events, etc.), which tend to be characterized by the scarcely predictable impact of extraordinary touristic flows. In analogy with the pilot study proposed by Transport for London (2019), the application of the Wi-Fi technology to monitor traffic data in real-time in mass transit and gathering facilities could enable the assessment of contextual conditions of service, detecting anomalies, preventing service disruption and guaranteeing the comfort and security of users.
The results of this research activity have been published in the scientific Journal TeMA: Gorrini, A., Messa, F., Ceccarelli, G., Choubassi, R. (2021). Covid-19 pandemic and activity patterns in Milan. Wi-Fi sensors and location-based data. TeMA - Journal of Land Use, Mobility and Environment, 14(2), 211-226. https://doi.
Wi-Fi Access Points SAI - Suitability Analysis Index 0 - 0.3 0.3 - 0.4 0.4 - 0.5 0.5 - 0.6 0.6 - 0.7 0.7 - 0.8 0.8 - 0.9 0.9 - 1 Figure 4
Results of proposed Suitability Analysis Index
The results of the analysis open up the debate on the need to design cities to have a polycentric structure, with several and distinctive areas of attraction for city users (i.e., 15-minute city concept) in order to be resilient to extraordinary events such as the Covid-19 pandemic.
Conclusions and Future Work
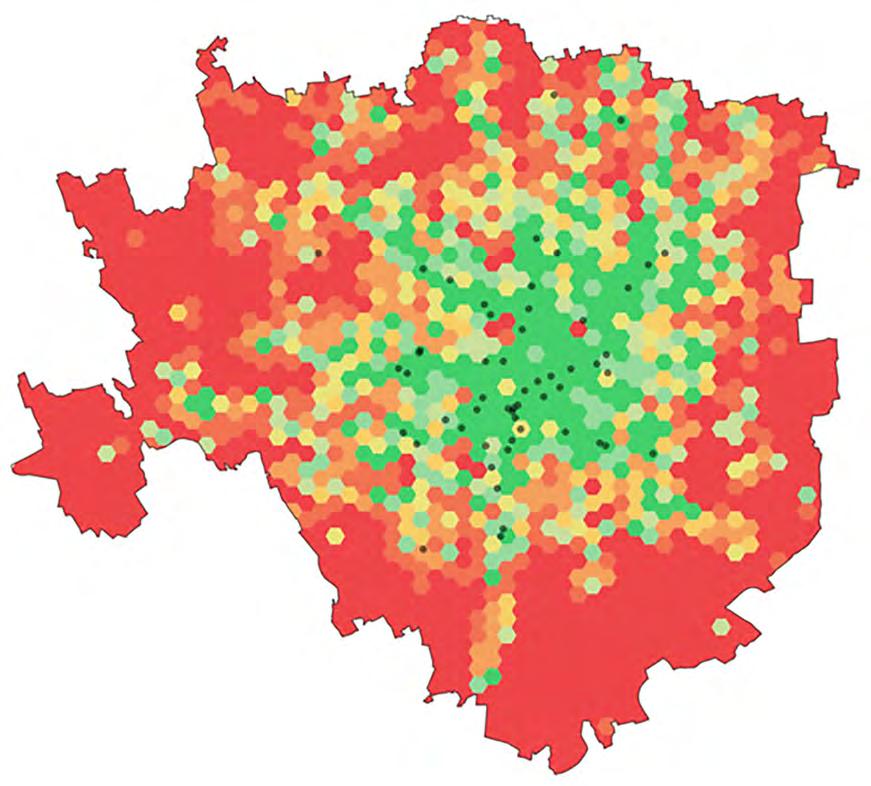
Sensors Data for Unlocking Hidden City Metrics
78
Thanks to the collaboration with Blimp.ai5, the following research is based on the analysis of proprietary data on pedestrian counts collected in Milan from November 2020 to July 2021 through a network of sensors. Data is used to assess the impact of the Covid-19 restrictions on pedestrian dynamics with a time series analysis. This is further analyzed through an extended GIS-based analysis of potential attractors of city users to assess the interplay between pedestrian traffic, city characteristics and restrictions. The results of the analysis focused on the average pedestrian count per sensor showing significant trends related to the impact of Covid-19 restrictions enacted under the considered scenarios on mobility patterns. Furthermore, the results related to geospatial data about land use, urban amenities and services show that public transport services are the most important factors for predicting pedestrian flows, with particular reference to tram stops and bike-sharing docking stations.
The recent developments of ICT tools for collecting traffic data in the urban environment have allowed a tremendous increase in the quality and amount of easily accessible data, not only for vehicular traffic (as is possible using traditional methods), but for more complex mobility modes such as pedestrian traffic as well. In the context of COVID-19, such information is critical given the predominance of walking as a mode of transport under strict vehicular mobility restrictions.
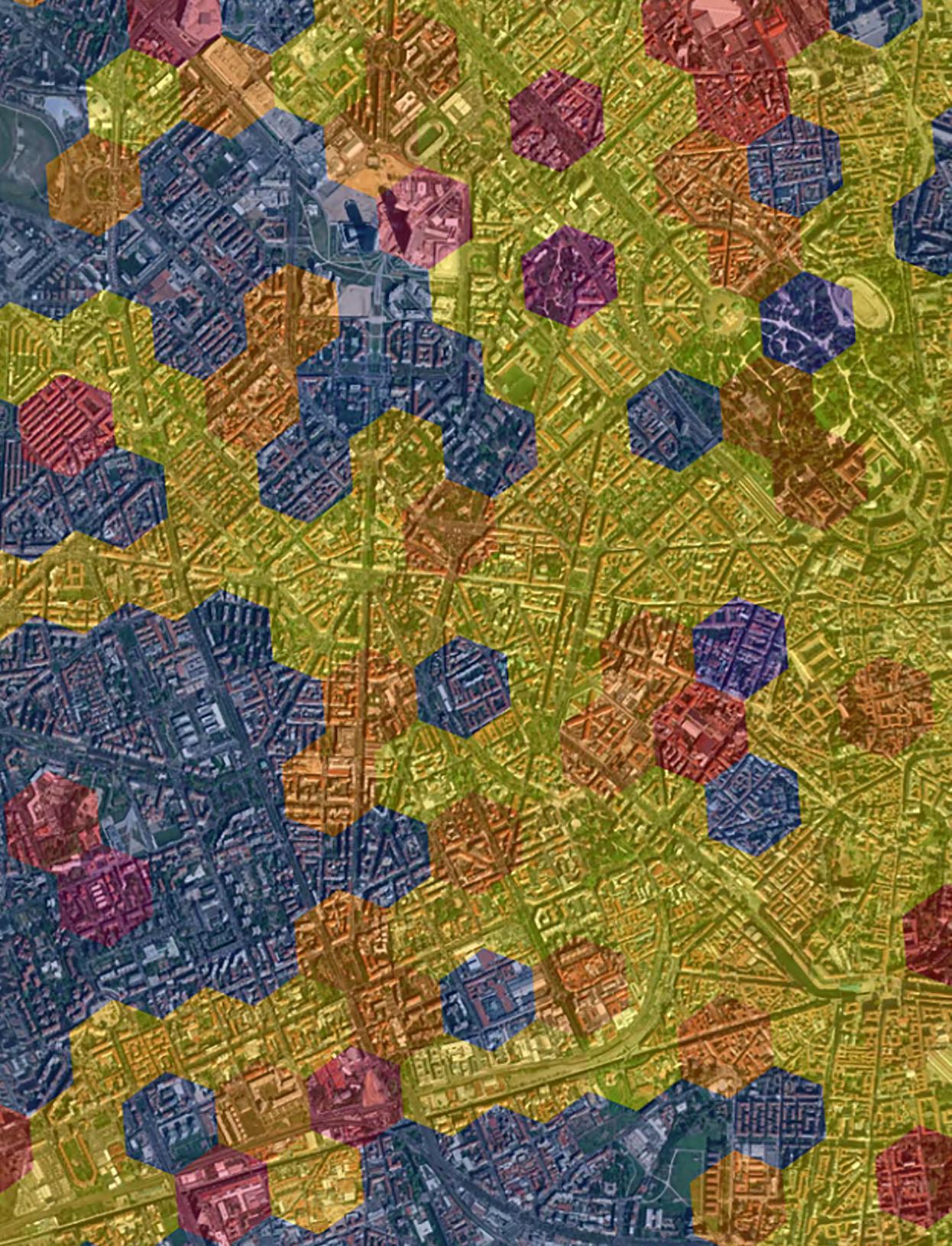
Blimp.ai5 developed a sensor (aided by an A.I.) which collects disaggregated traffic data (e.g., vehicular traffic, pedestrian and bicycle flows). Thanks to the collaboration with them, Transform Transport analyzed pedestrian activity data collected through a network of sensors installed in advertising billboards. Sensors are located next to newspaper stands, typically on larger sidewalks or plazas, and they count passersby in a cone shape.
Urban Informatics for Transport Planning
Being a robust and easy technique (Schwertman et. al., 2004), the interquartile range method was chosen. Values detected as being outliers were then replaced by null values. Moreover, sensors that had too high a frequency of removed or missing values were excluded from the study, as they would likely be unreliable. This process led to a final selection of 47 sensors.
79
Average pedestrian counts of each sensor over the 9-month study period
Figure 1
A few values were missing throughout the data collection period, likely due to malfunctioning of sensors. Moreover, some values were inexplicably high or low relative to others, therefore, a data validation process was necessary to find and remove outliers. Those values were removed and replaced by null values. However, some other strange values were scattered over the study period highlighting the need for a more systematic way to detect outliers.
Enabling Data and Methodology
The growth of urban data produced by sensors scattered across our cities allows better management and optimization of transport services in real time (Li et al., 2020). Within the Urban Informatics approach (Foth et al., 2011), the aim is to assess the operational conditions of mobility services, detecting anomalies, and avoiding service disruptions. The more powerful ICT solutions for analysis of travel patterns can be enabled by several different types of location detection systems. This study uses sensors-based analysis-acquisition systems (Buch et al., 2011), in which data is processed by an Artificial Intelligence to count pedestrians. In this context, the Covid-19 pandemic has revealed a crucial application of traffic data monitoring: to examine the effects of the pandemic on urban mobility and to measure the effectiveness of the cities’ responses to the crisis (European Platform on Sustainable Urban Mobility Plans, 2020; Comune di Milano and AMAT, 2020).
This research proposes a combined spatial and temporal analysis of pedestrian count data in the City of Milan to assess the impact of Covid-19 lockdown restriction measures on pedestrian traffic and the interplay with city attractors. By analogy with a methodology presented by Transport Transport for the analysis of Wi-Fi data (Gorrini et al., 2021), pedestrian counts data is used to estimate hidden mobility patterns in the City of Milan, focusing on the latter waves of the pandemic (from the beginning of November 2020 to the end of July 2021) (see Figure 1).
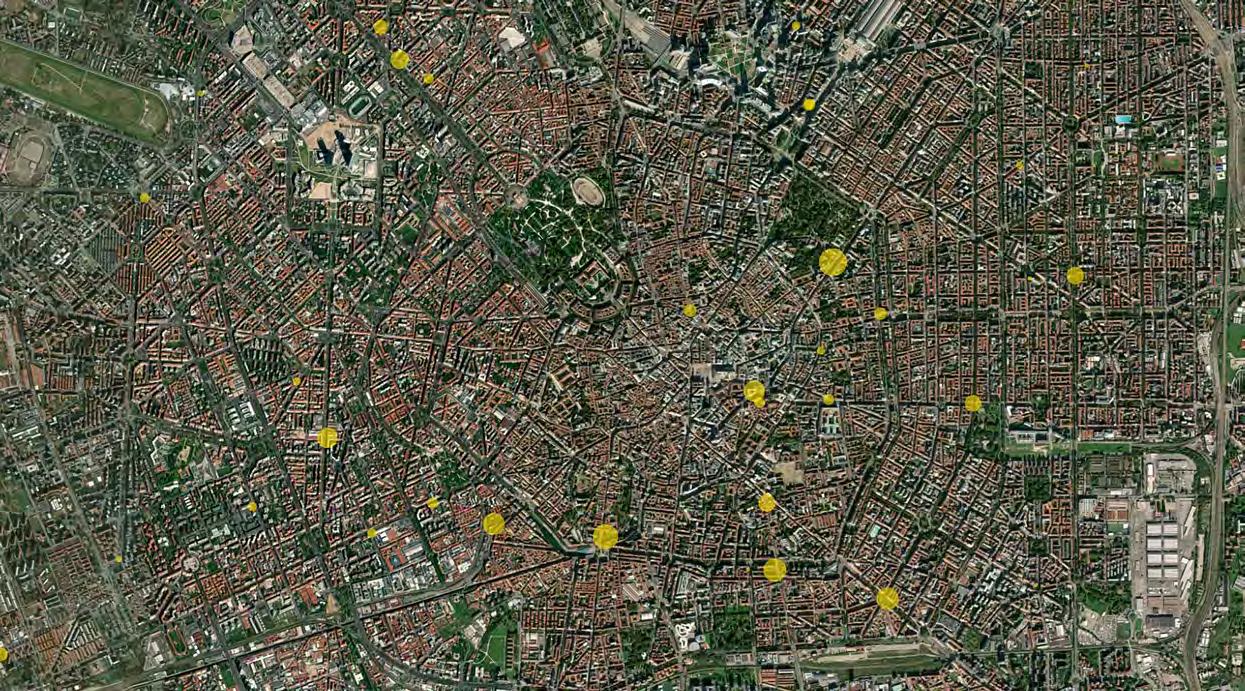
countpedestrianAverage Date M_AVG7AVG_COUNTPhases
Figure 3 shows the average count of pedestrians per day of the week, for the various phases. Values are more homogeneous the stricter the Covid-19 measures get, but Wednesday remains the least active day of the week for all phases.
Figure 3 Correlation analysis, circadian Table 1 Types of Covid-19 restrictions
80
The chart (see Figure 2) presents the result of the time series analysis of the average values per sensor from November 1st, 2020, to July 29th, 2021. The types of Covid-19 restrictions enacted under each scenario are highlighted in the chart, and described in Table 1 by ascending order of strictness: A 7-day moving average smoothes out short-term variations, showing longer-term trends. However, the rapid evolution of covid restrictions limits the potential to interpret the precise effect they had. For this purpose, a series of t-tests were conducted, resulting in a significant (p < 0.05) difference between the number
of pedestrians detected on a daily average during all scenarios. Therefore, it can be deduced that the Covid-19 restrictions had a sizable effect on pedestrian activity.
Figure 2 Trend analysis (count and moving average)
Results of Temporal Analysis
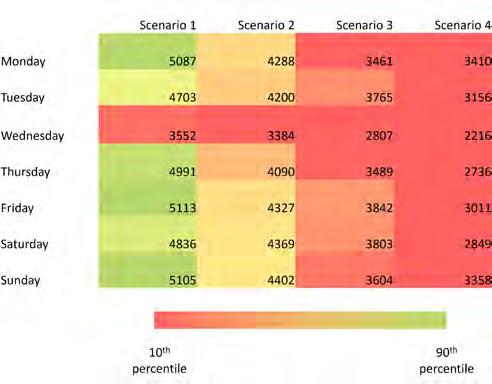
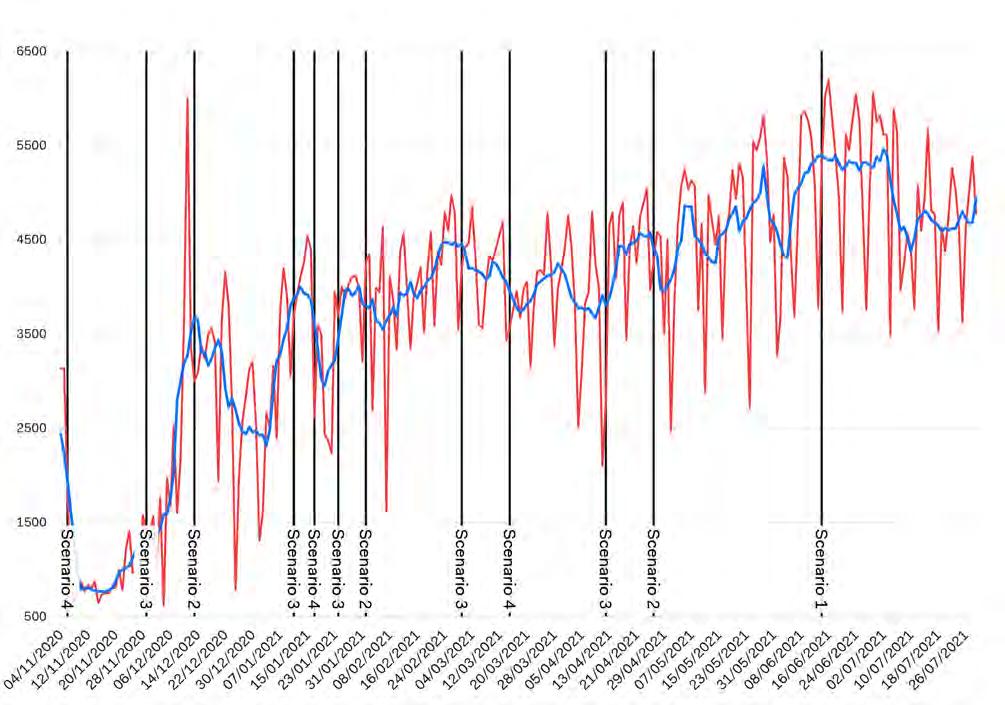
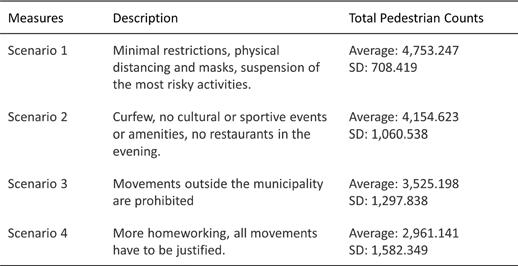
Table 2 Correlation analysis, explanatory factors selected Table 3 Results of correlation analyses Results of Spatial Analysis
A series of geospatial data about land use, urban amenities and services was collected thanks to open data from local authorities (i.e., Geoportal of the City of Milan2, Geoportal of Lombardy Region8, Italian National Institute of Statistics9), and from OpenStreetMap10. These are listed in Table 2. A series of correlation analyses (Pearson’s Correlation Coefficient) revealed a positive correlation between pedestrian counts and attractors, summarized in the table and in Figure 4. Moreover, results show that public transport services are often the most important factors for pedestrian flows, with particular reference to tram stops and bike-sharing docking stations (see Table 3).
81
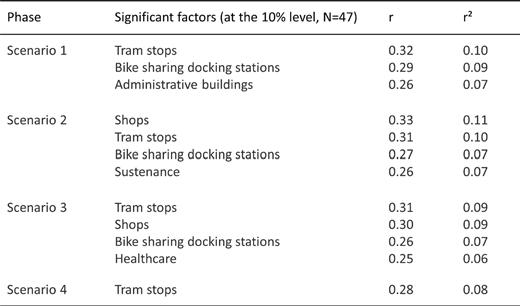
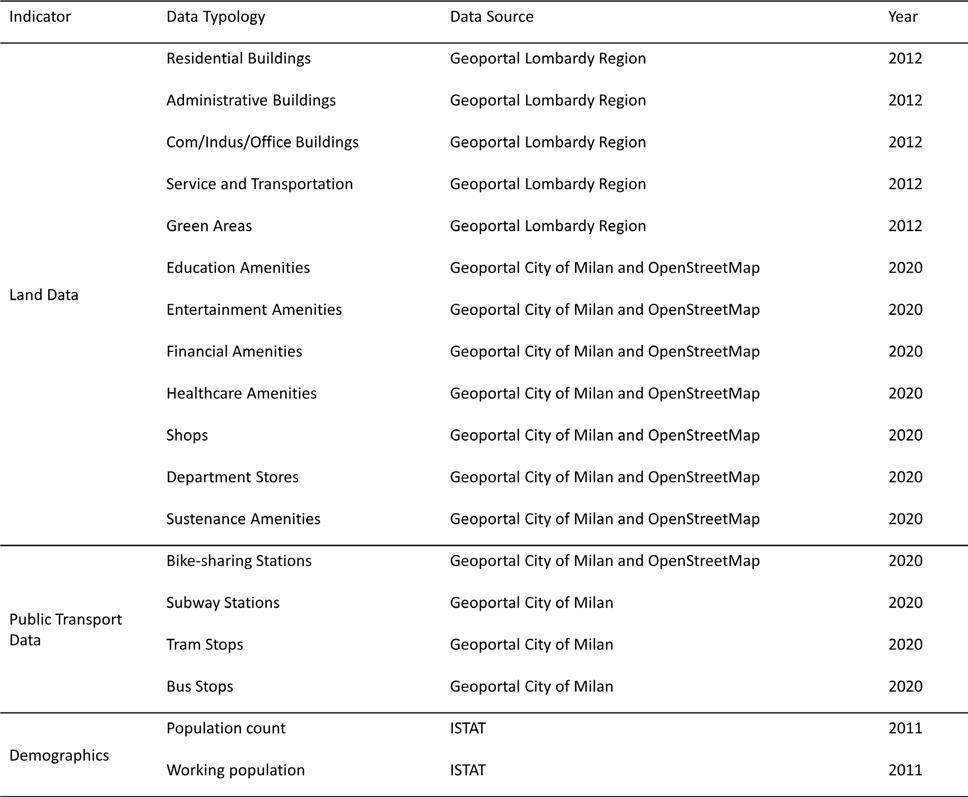
Bus Bike-sharingTramSubwaystopsstationsstopsstations Department stores PopulationFinancialEducationHealthcareEntertainmentSustenanceShops095,000density (residents/km²) + Selected sensors 400m buffers GreenServicesCommercialAdministrativeResidentialareas + Selected sensors 400m buffers + Selected sensors 400m buffers + Selected sensors 400m buffers Figure 4 Correlation analysis, explanatory factors selected. The count or area of these factors were measured in a 400m radius around each sensor (namely 5 minutes walking distance). 82
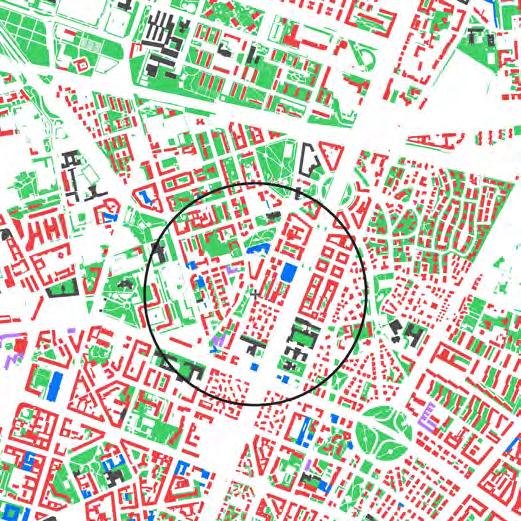
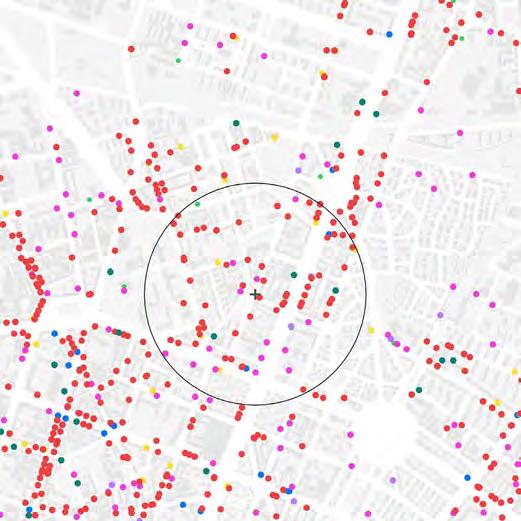
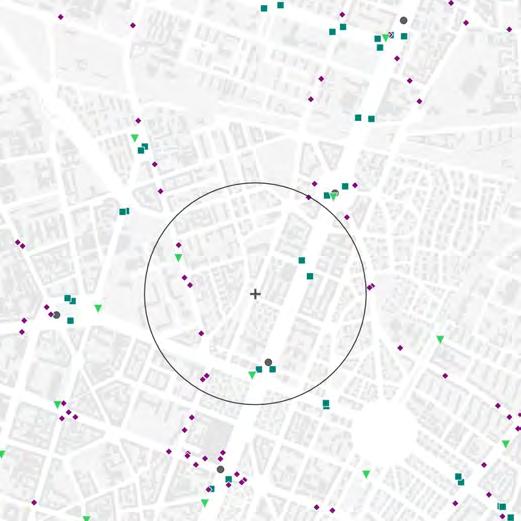
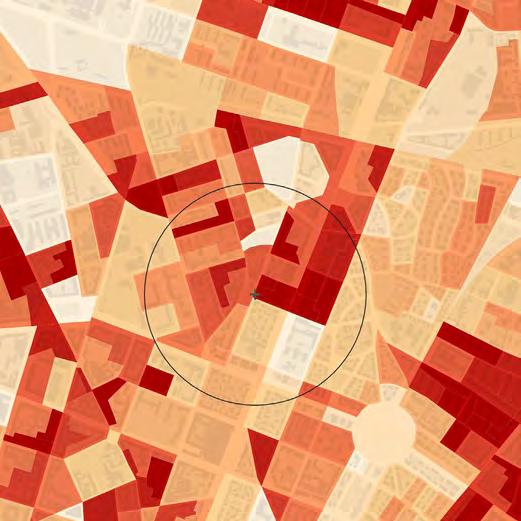
A spatial statistical analysis showed that sensors close to tram stops, bikesharing stations and administrative buildings had higher pedestrian activities. Given that this spatial data is easily collectable for major cities, this study can be replicated in different contexts across the world. Future research could make use of more sensors and advanced analytical tools to accurately estimate the expected pedestrian activity in a city.
SAIi = rTram ZTram,i + rBike,i ZBike,i + rAdmin ZAdmin,i The Z values are obtained by evaluating the amount of selected amenities (i.e., tram stops, bike sharing docking stations and administrative buildings), normalized on a 0-1 scale using a normal cumulative probability distribution function. The formula aboved is then used with i standing for every cell, resulting in the map below of the potentialities for relevant pedestrian activities around newspaper stands.
83
The team thanks Blimp.ai (an e-Novia company) for their fruitful collaboration and for sharing data.
Sensors-based techniques have been shown to be very effective for collecting traffic data of different users in urban areas, with decreasing costs and great progress being made on the A.I. powering those tools (Buch et al., 2011). However, their compliance with the General Data Protection Regulation (National Centre IOT and Privacy, 2020) is crucial for privacy and acceptability of this technology.
Conclusions and Future Work
Figure 5
This research work was conducted with a large proprietary dataset of pedestrian counts, spanning a 9-month period (from November 2020 to the end of July 2021), collected by Blimp.ai through 47 sensors across Milan. Statistical analysis showed the sizable impact of the Covid-19 restriction measures on pedestrian activity. This method could be applied in real time to assess the effectiveness of those kinds of policies in the future.
Results of Suitability Analysis Index
To assess and forecast potential pedestrian activity patterns a Suitability Analysis Index11 (see Figure 5) was constructed from the r values of Scenario 1, the one that likely resembles the medium-term future of Covid-19 restrictions.
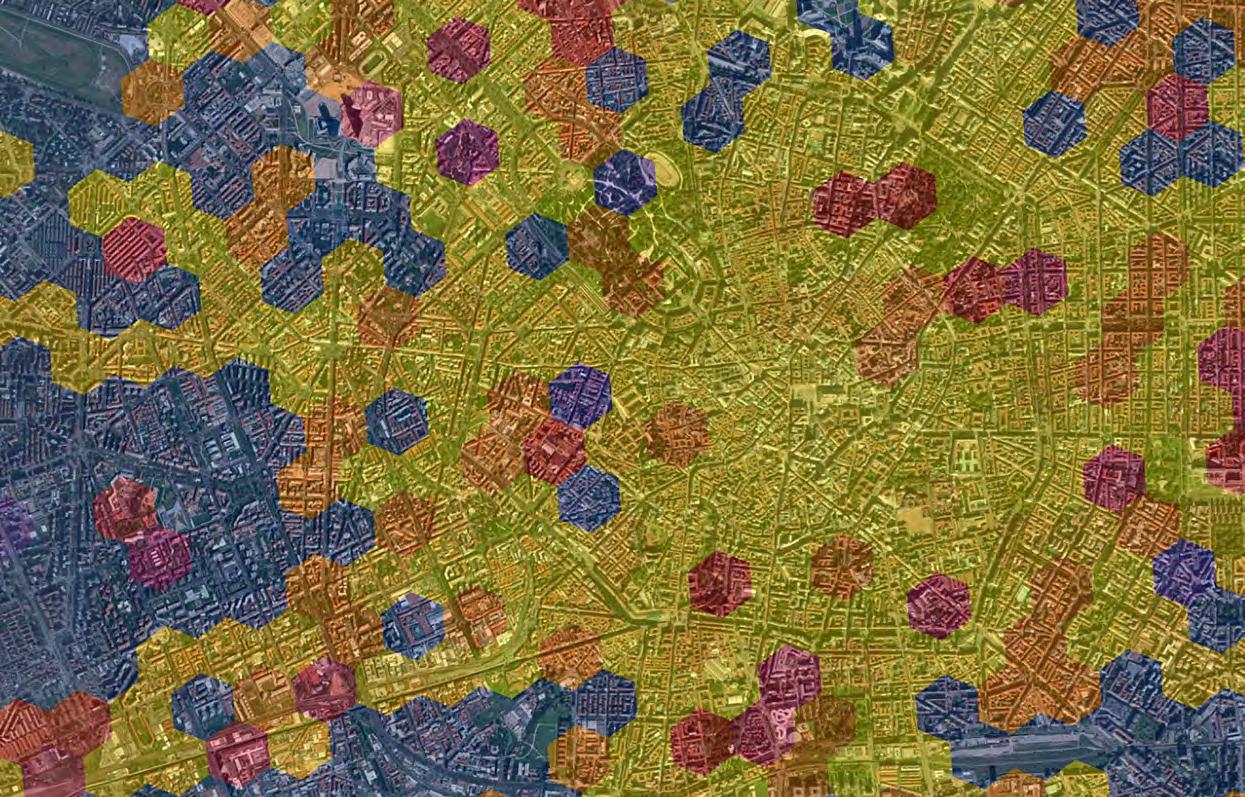
To investigate this phenomenon, the following research proposes an Urban Informatics approach to understand passengers’ opinions and expressed polarity on public transport during different phases of the pandemic through user-generated socialmedia data (Twitter) in the Greater London Area. The analyzed corpus consists of 27,700 tweets posted between January 13th, 2020 and May 17th, 2021, and geolocated in immediate proximity of a short sample of selected Tube stations. Data is segmented in several phases, based on the restriction measures enforced by the UK National Authorities. Each subset is then analyzed through texts structuring and semantic analyses, with LDA topic modelling and Sentiment Analysis. Finally, the outcomes of these processes are interpreted in their chronological succession and compared to demand data and servicedisruption data. The research shows the possibility to exploit social media data mining techniques to develop a comprehensive model of an urbanized area, able to describe and simulate phenomena happening in the urban environment because in addition to locationbased information.
At an urban level, the Covid-19 pandemic has deeply affected the perception of public transport as an urban mobility choice: the social-distancing rules adopted to cope with the virus transmission pushed the majority of city users into avoiding public transport services in favor of contactless (and often private) travel options.
TubeConversation''TwitteronStations
I Really love these Railway Stations! COVID19 is a shame ���� #pandemic #staysafe Fondazione @transformtransport 11:30 AM • Sep 13, 2021 Wondering if the train will today �� #service Fondazione @transformtransport 11:30 AM • Sep 13, 2021 I wonder if I will manage to arrive on time... #delay Fondazione @transformtransport 11:30 AM • Sep 13, 2021 Really looking forward to have a drink while waiting for the subway to arrive Fondazione @transformtransport 11:30 AM • Sep 13, 2021 P0 Baseline Phase (13/01/2020 - 17/03/2020) (18/03/2020 - 11/05/2020) [12.744] [1.637] P1 Lockdown 1 covid corona coronavirus virus pandemic lockdown quarantine staysafe stayhome distance distancing mask facemask vaccine covidvaccine vaccination nhsPreventionLockdownPandemicVaccineOther
Analysing
84
and flows.
User-generated Social-media Data Tool Understanding City Data is at the foundation of modern planning practice and among other tools that enable collection of mobility patterns In particular, user-generated socialmedia data has the potential to represent both nationalscale (Jurdak et al., 2015) and urban-scale mobility patterns (Plunz et al., 2019). This opportunity has become even more crucial considering the need to investigate the unprecedented effects of disruption of the Covid-19 pandemic on urban mobility. In particular, the nation-wide lockdown and post-lockdown phases have drastically changed citizens behaviours and mobility patterns related to the use of public transport services (Buhrmann et al., 2020), due to the need to avoid crowded transport infrastructures in favour of safe and contactless travel options (e.g., private vehicles, cycling, walking, etc.). In this context, the article presents an extended analysis of a Twitter-data corpus, collected by geographical location and specific timeframes, and further filtered by removing non-relevant tweets and non-human posting. The acquired corpus consists of 27,700 tweets geolocated in immediate proximity of selected Tube stations of the Greater London Area (UK) and posted between January 13th, 2020 and May 17th, 2021. The social-media data is segmented in 9 phases, shown in Figure 1, to compare the outcomes of the analyses in timeframes that have a mobility-demand significance, but also a social connotation. The goal of these analyses is to offer a comprehensive view of the relation between public transport and usergenerated data. Hence, the process is twofold: firstly, the analysis is focused on understanding the impact of the pandemic on the public transport mobility patterns through daily demand data and daily service-disruption data. Secondly, the social-media data is investigated with two different methodologies based on texts structuring and semantic analyses, specifically: (i) identification of tweets’ subjects through LDA Topic Modelling, and (ii) assessment of the conversation emotional polarity through Sentiment Analysis.
85
be on time (12/05/2020 - 14/09/2020) (15/09/2020 - 05/11/2020) (05/11/2020 - 02/12/2020) (03/12/2020 - 20/12/2020) (21/12/2020 - 05/01/2021) (06/01/2021 - 12/04/2021) (13/04/2021 - 17/05/2021) [4.884] [2.938] [940] [1.051] [403] [1.820] [1.283] P2 Transition Phase P3 Pre Lockdown 2 P4 Lockdown 2 P5 Tier 2 / Tier 3 P6 Tier 4 P7 Roadmap Out of Lockdown 1 P8 Roadmap Out of Lockdown 2 Figure 1 Distribution of reference set words during the phases
as a
for
the
The raw outcome of this process is a dataset comprising of 95,463 tweets, which was then post-processed in several steps in order to harmonize tweets’ text to be input in further semantic analyses, removing noise and superfluous information, by:
P0 P2P1 P3P4P5P6P7 P8 13/01/2017/03/2011/05/20 14/09/2005/11/2002/12/2020/12/2005/01/21 12/04/2116/05/21 Figure 2 Restriction and phasing definition Figure 3 Location of selected Tube stations Social Media Data Gathering
All these processes contribute to the harmonization of text and the removal of words with no semantic meaning, enhancing the performance of the topic modelling, specifically: (i) stop words removal was based on the NLTK English stop-words list (e.g., a, an, the, etc.) (Bird et al., 2009), which was extended with custom words (e.g., London, etc.) that appeared ubiquitously in the corpus; (ii) lemmatization consists of a reduction of each word to its root and allows a further selection of allowed tokens, in this case leading to the selection of nouns, verbs, adjectives and adverbs in each tweet. The data cleaning process was differentiated for topic modelling and sentiment analysis and it's shown in Figure 4.
• Natural Language Processing (NLP) methodologies, such as stop words removal and lemmatizations (Bird et al., 2009).
• Text content pre-processing with characters-based simplifications removing punctuation signs, emojis, URLs and converting all the text to lowercase format;
• Detecting repetitive and non-human posts in the overall corpus, the presence of which could lead to biased and inaccurate topic modelling;
In accordance with the research scope, the phasing structure is derived from the evolution of the pandemic; the phases were selected by considering the national and local policies imposing restrictions affecting Greater London. The calendar, comprising of 25 policy milestones, was reduced to 9 phases in order to avoid an excessive segmentation of the outputs, considering only the major self-distancing measure changes. In addition to the pandemic period, a Baseline Phase was added for the purpose of providing a reference scenario for the assessed indicators (see Figure 2). This practice is common in a before-after analysis and the timeframe chosen was in line with the Coronavirus publications produced by Transport for London (TfL).
86
Similarly, a selection of Tube stations (see Figure 3) was performed in order to identify the locations to look for geo-located tweets. This process was achieved by using demand data from 2019 published by TfL, which represents the daily entry/exit for the typical weekday of a pre-pandemic year. The stations were selected by considering the 85th percentile of the demand, resulting in 40 stations located in the Greater London Area. The selected stations’ locations are used as centroids to calculate buffers of 400 meters of radius. The measure of the proposed catchment area around stations was chosen since it can be considered as a 5-minute walking distance in the analysis setting. Moreover, the small catchment area represents a location bias able to represent the content posted in proximity of public transport infrastructure.
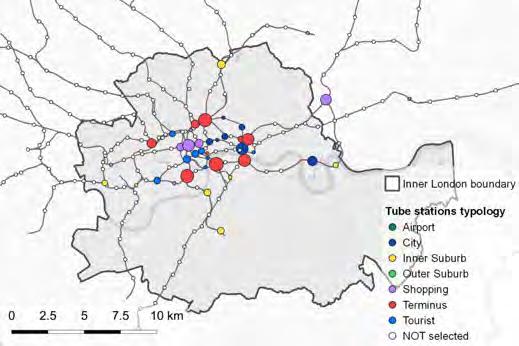
Topic Modelling Analysis
• Other: nhs.
Text Semantic Analysis
• Vaccine: vaccine, covidvaccine, vaccination;
Topic AnalysisModelling
Figure 4 Data collection and cleaning process - From left to right: collection in stations' buffer; filtering of non-user posts; text content pre-processing; Natural Language Processing (NLP)
Furthermore, Sentiment Analysis was performed using Vader (Valence Aware Dictionary and sEntiment Reasoner) (Hutto et al., 2014), a lexicon and rule-based analysis tool developed to estimate the polarity of social media texts. The tool outputs three values divided into positive, neutral and negative scorings and a compound value to summarize the sentiment’s results.
The set consists of 17 terms that also include variation of the same lemma, divided thematically into five categories:
The two analyses performed to comprehend the content of the corpora were (see Figure 5): • Topic modelling: to gain knowledge on the main tweets’ subjects; • Sentiment analysis: to investigate the expressed message polarity.
This set was built considering the overall corpus content by manually evaluating a random subset of tweets, Figure 1 shows the distribution of these words.
SentimentAnalysis
• Pandemic: covid, corona, coronavirus, virus, pandemic;
Topic Models are statistical language models used to understand the theme structure of a document. In particular, the used model was Latent Dirichlet Allocation (LDA), which is a probabilistic generative model that takes as input a collection of lemmatized texts and outputs topics’ probability distribution over each document (Kapadia, 2019). For each phase of the analysis timeframe, topic modelling was used to identify 10 topics per Theperiod.model parameters were hypertuned to obtain best fitting topics, looping over LDA’s main parameters. Subsequently, the significance of the results was quantified using the topics’ coherence metric, which measures the degree of semantic similarity between high-scoring words in the topic, helping to distinguish artifacts from semantically valid outcomes. In order to measure the influence of pandemic-related posts in the analysed corpora, a reference control set built around Covid-19 related words was defined and compared against the previously obtained topics. By using the reference set as an unseen document to query the models, the output was the probabilistic distribution of the queried control words among the phases’ topics set.
• Lockdown: lockdown, quarantine, staysafe, stayhome;
Figure 5 Text semantic analysis - From left to right: LDA topic modelling; Reference set models querying; Sentiment analysis
• Prevention: distance, distancing, mask, facemask;
The relation between the reference set and the phases’ corpora is expressed by measuring how many terms of the set fall into the dominant topic (i.e., the topic with highest semantic similarity with the queried text) and by the overall topic weight (i.e., the probability distribution of the topics with respect to the queried text).
87
Since the topic modelling analysis was performed on diverse corpora and the LDA model is generative, the reference was devised to infer the relation between Covid-19 related terms during the phases.
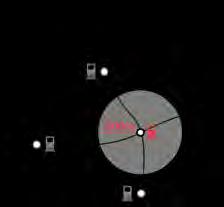
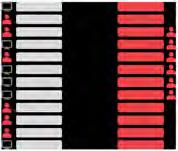
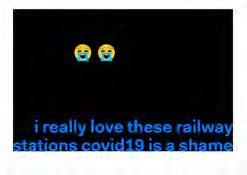
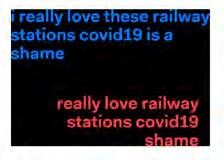
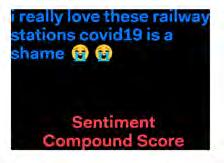
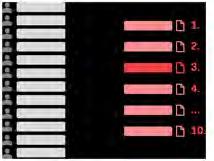
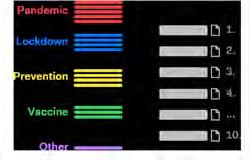
Figure 7
Service Disruption Analysis and Sentiment Analysis
In addition to the demand used to select the stations, which was referred to the pre-pandemic year (2019), the daily demand of the selected timeframe was analysed in order to understand the actual impact of Covid-19 on the Tube’s mobility. The variation between the phases is substantial to the extent that during the last phase (P8) the average daily demand is -67% compared to the Baseline Phase (see Figure 6).
Finally, the sentiment analysis can be interpreted using the threshold proposed by the tool creators (Hutto et al., 2014): typical compound limits are positive (score ≥ 0.05), neutral ( - 0.05 > score > 0.05) and negative (score ≥ - 0.05). Considering these values, all the phases are characterized by a positive average compound score.
Findings While the demand and service data describe a currently well-known phenomenon, the text semantic analysis allows the gathering of more insights on the perception of the pandemic. The distribution of covid-related terms in the different phases allow to understand the evolution of the conversation: during the Lockdown 1 phase, the conversation was more focused on the actual situation, with the largest part of the conversation focused on the “Pandemic” theme. This was followed by an interest more related to the “Lockdown” theme and the restriction measures, during Lockdown 2 phase. Finally, the discussion converged towards the “Vaccine” theme during the last analysed phases. By comparing these considerations to the topic modelling outputs, it is possible to confirm some of these insights. During the Lockdown 1 phase (P1), the dominant topic relates to “Pandemic” and “Lockdown” themes and it shifts toward “Lockdown” in P4 and to “Vaccine” during the last phases. However, the Dominant topic weight metric shows how relevant the topic is in relation to the generated model. A more or less relevant topic can be influenced by several factors, such as an effective topic modelling process or the semantic significance of the analysed corpus. However, for the scope of this research, it can be considered as a reference-set independent weight metric describing the relevance of the outputs.
Service quality index per phase
In order to structure a comprehensive view of the public transport usage during the pandemic evolution, the service disruption was taken into consideration. In this regard, the disruptions caused by diverse sources were categorized and aggregated per phase. The service interruptions and delays were considered for all the Tube lines since the selected stations are distributed among all the subway lines and a service quality index was calculated in term of minutes of delay per day, as shown in Figure 7. While the Topic Modelling Analysis is performed separately on each corpus, the Sentiment Analysis is used to interpret each single tweet, then the results are aggregated and interpreted per phase. VADER tool expresses the polarity of each item by three values, defined as positive, neutral and negative and a normalized weighted Compound Score to summarize the overall results (Hutto et al., 2014). For the scope of this research the value considered is the Compound Score, which expresses a single comparable score among the entries.
Figure 6 Timeseries of number of tweets per day (left); Timeseries of passengers demand per day (right) Demand Data and Tweets
However, social-media data is usually influenced by a positivity bias (Waterloo et al., 2018) because negative 88
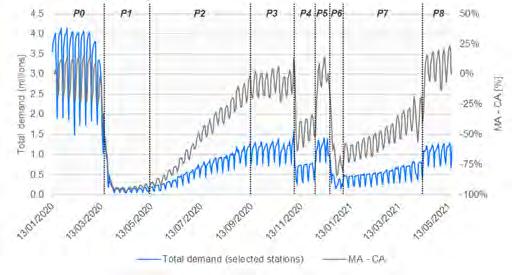
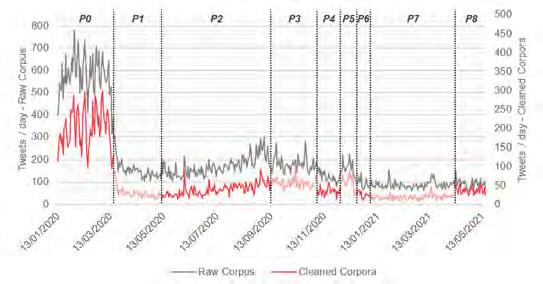
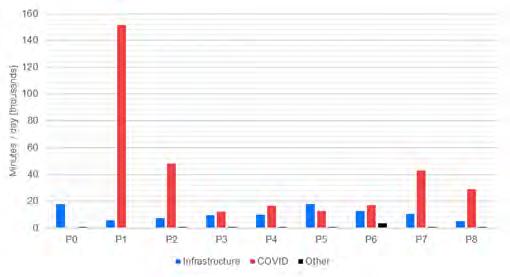
Figure 8 Variation of the Dominat Topic Weight and included reference set terms (up); Variation of the average Compound Score in comparison with the Baseline Phase (bottom) [%]topicdominantinincludedTerms 101520253050 Pandemic (5) Vaccine (3) Lockdown (4) Other (1) Prevention (4) Dominant topic weight P0 P1 P2 P3 P4 P5 P7P6 P8 1][0,topicdominantofWeight0.0000.1000.2000.3000.4000.5000.600 [%]VariationSoundCompound -1212840-4-8 P0 P1 P2 P3 P4 P5 P7P6 P8 Compound Score Trend (with P0) Average Compound Score Compound Score Trend (with previous) 1][0,ScoreComoundAverage0.1500.2500.0000.0500.1000.2000.3000.3500.400
The results of this research work has been published on Zenodo: Messa, F., Ceccarelli, G., Gorrini, A., Presicce, D., Deponte, D. (2021). Analysing ‘Twitter Conversation’ of London Tube Stations: The Case of the COVID19 Pandemic. In: Proceedings of the 49th European Transport Conference 2021 (ETC 2021), 13-15 September 2021 –https://doi.org/10.5281/zenodo.6493631Online. 89
The team thanks Transport for London (TfL) for sharing data.
emotions are often considered as not appropriate to publicly share on social media. For this reason, the trend metrics are proposed, and it is possible to appreciate how the general sentiment per phase is always lower than the Baseline Phase and especially how the variation closely follows the demand data, hence the restrictions implementation calendar. Even if in a qualitative fashion, these sets of data manage to add information that is otherwise difficult to obtain without the utilization of surveys or time-consuming and cost-impactive methods. For this reason, the analysis of social media data is steadily increasing its applications, ranging from sociological and medical studies to mobility-related analyses. Crowd-sourced data could be one of the potential data feeds needed to develop a comprehensive model of an urbanized area (digital twin) able to describe and simulate phenomena happening in the urban environment, because in addition to locationbased information, they allow to identify social and cultural patterns.
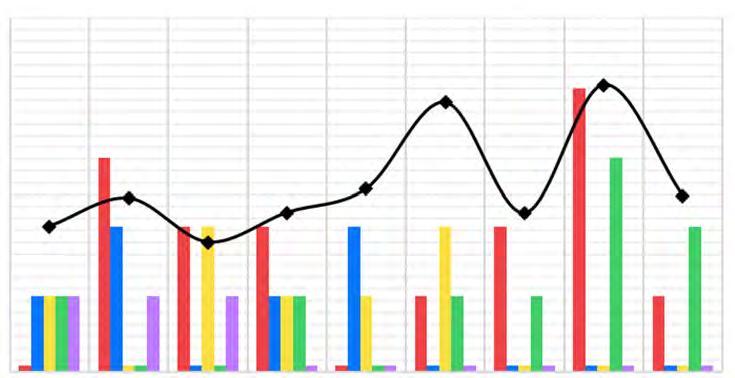
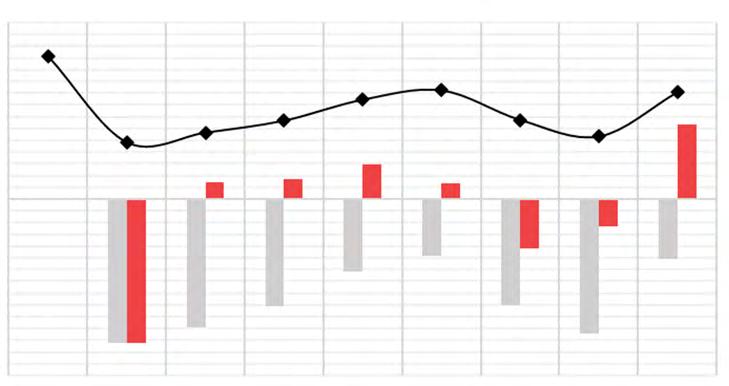
Way Forward 90
Covid-19 has triggered a series of theoretical reflections and urban experiments, whether through research or physical urban interventions, which we, at Transform Transport, could not but be part of. We have witnessed these changes steered by the need to adapt to the new conditions that the pandemic imposed on us. Kicking off our research days after the outbreak of the pandemic, today, more than two years later, our efforts are persistent towards our aim to bring this urban research endeavour to the next level – looking beyond the current moment to envision a more resilient future for our cities. The pandemic pushed cities worldwide to change the way to read and plan cities, reprioritizing certain assets, such as walkable networks and alternative modes of transportation, capitalizing on changing working habits and recognizing the immense
capability of open space and the public realm in enhancing the quality of urban life.
The vision for the experiments and studies presented herein is to become a living legacy while planning our future cities, as new parameters and evolving technologies continue to provide new opportunities for exploration. These studies will therefore continue to be tested and updated as part of an ongoing process, with the aim to transform them from simple analytical frameworks into robust urban design principles. We strongly believe that the development of new methods for reading urban conditions has the potential to increase the sensitivity of our planning tools, paving the way for a gradual and everlasting change to the practice of urban and transportation planning.
91
Above much else, the Covid-19 premise for the book provided a new perspective with which to view already existing urban issues, thereby exposing more dimensions to be investigated and inducing innovative methodologies.
92 Bibliography
Bernas, M., Płaczek, B., Korski, W., Loska, P., Smyła, J. and Szymała, P. (2018). A Survey and Comparison of Low-Cost Sensing Technologies for Road Traffic Monitoring. Sensors, 18(10), 3243. https://doi. Bird,org/10.3390/s18103243S.,Klein,E.andLoper, E. (2009). Natural language processing with Python: analyzing text with the natural language toolkit. O’Reilly Media, Boglietti,Inc.S., Barabino, B. and Maternini, G. (2021). Survey on e-Powered Micro Personal Mobility Vehicles: Exploring Current Issues towards Future Developments. Sustainability, 13(7), 3692. https://doi. Bolognesi,org/10.3390/su13073692C.andGalli,A. (2017). Mapping Socials: A Voluntary Map of a Great Event in Monza Park. In: Multidisciplinary Digital Publishing Institute Proceedings, 1(9), 917. https://doi.org/10.3390/ proceedings1090917
Batty, M. (2010). The Pulse of the City. Environment and Planning B, 37 (4), 575–577. https://doi.org/10.1068/b3704ed
Deponte, D., Fossa, G. and Gorrini, A. (2020). Shaping space for everchanging mobility. Covid-19 lesson learned from Milan and its region. TeMA-Journal of Land Use, Mobility and Environment, 133-149. Espitia,https://doi.org/10.6092/1970-9870/6857E.,Gorrini,A.,Vacca,A.,Deponte, D. and Sarvi, M. (2022). How Covid-19 is Affecting Pedestrian Modelling and Simulation: The Case of Venice. Transportation Research Record. https://doi. Europeanorg/10.1177/03611981221088224PlatformonSustainableUrban Mobility Plans (2020). COVID-19 SUMP Practitioner Briefing. CIVITAS SATELLITE CSA. Available online: Foth,sumppractitionersbriefing_final.pdfhttps://www.eltis.org/sites/default/files/covid-19_(accessedon1March2021)M.,Choi,J.H.J.andSatchell,C.(2011).Urbaninformatics.In: Proceedings of the ACM 2011 Conference on Computer Supported Cooperative Work, 1-8. https://doi.org/10.1145/1958824.1958826 Gehl, J. (2013). Cities for people. Island press. Geng, D., Innes, J., Wu, W. and Wang, G. (2021). Impacts of COVID-19 pandemic on urban park visitation: a global analysis. Journal of Forestry Research, 32, 553–567. https://doi.org/10.1007/s11676Global020-01249-wDesigning Cities Initiative and National Association of City Transportation Officials (2016). Global street design guide. Island Press. Available at:
Batty, M. (2013). Big data, smart cities and city planning. Dialogues in Human Geography, 3(3), 274-279. https://doi. org/10.1177%2F2043820613513390
Bureauguidelines_2019_interactive_document_1.pdfofPublicRoads(1946).
Crist, P., Greer, E., Ratti, C., Humanes, P., Konzett, G., Tijink, J., Figuero, D. and Lax, R. (2015). Big Data and Transport: Understanding and assessing options. International Transport Forum. Available at: https://www.itf-oecd.org/sites/default/files/docs/15cpb_bigdata_0.pdf
Gutta, S. (2021). Object Detection Algorithm — YOLO v5 Architecture. Medium. Available at: 15,000-mileHeineke,detection-algorithm-yolo-v5-architecture-89e0a35472efhttps://medium.com/analytics-vidhya/object-K.,Kloss,B.,Scurtu,D.andWeig,F.(2019).Micromobility’scheckup. McKinsey & Company, Automative & Assembly Available at: https://www. mckinsey.
Buch, N., Velastin, S.A. and Orwell, J. (2011). A review of computer vision techniques for the analysis of urban traffic. IEEE Transactions on Intelligent Transportation Systems, 12 (3), 920–939. https://doi. Buhrmann,org/10.1109/TITS.2011.2119372S.,Wefering,F.,Rupprecht, S. (2019). Guidelines for Developing and Implementing a Sustainable Urban Mobility Plan. Second Edition. Rupprecht Consult-Forschung und Beratung GmbH. Available at: https://www.eltis.org/sites/default/files/sump_ Traffic Assignment Manual US Department of Commerce, Urban Planning Division. Choubassi, R. and Abdelfattah, L. (2020). How Big Data is the Way We Plan Our Cities. FEEM Policy Brief, 20, 1-16. at SSRN: City of Melbourne (2021). Pedestrian Counting System. Available images taken from the video stream of traffic cameras. Information Technology Department. Available at: Comuneannotees-cameras-circulation.https://donnees.montreal.ca/ville-de-montreal/images-diMilanoandAMAT(2020).
Barthélemy, J., Verstaevel, N., Forehead, H. and Perez, P. (2019). EdgeComputing Video Analytics for Real-Time Traffic Monitoring in a Smart City. Sensors, 19(9), 2048. https://doi.org/10.3390/s19092048
Citystatistics/city-population/Pages/pedestrian-counting-system.aspxhttps://www.melbourne.vic.gov.au/about-melbourne/research-and-at:ofMontreal(2021). Annotated
Jacobs, J. (1961). The Death and Birth of Great American Cities. Penguin. Jocher, G. et al. (2021). ultralytics/yolov5: v5.0 - YOLOv5-P6 1280 models, AWS, Supervise.ly and YouTube integrations (v5.0). Zenodo https://doi.org/10.5281/zenodo.4679653
Available
Transforming
Open Streets: Milan 2020 Adaptation Strategy. Comune di Milano. Available at: Comuned9be0547-1eb0-5abf-410b-a8ca97945136?t=1589195741171comune.milano.it/documents/20126/7117896/Open+streets.pdf/https://www.diMilano(2019). Milano 2030 Visione, Costruzione, Strategie, Spazi. Piano di Governo del Territorio (PGT). Comune di Milano. Available at: baba55b0-c49a-ce8a-d9d2-68380cce21d2?t=1554722056097it/documents/20126/2053134/01DP_Relazione_Generale.pdf/https://www.comune.milano.
results.estimationGorrini,global-street-design-guide/https://globaldesigningcities.org/publication/A.,L.Crociani,L.Vizzari,G.,andS.Bandini.(2018).Stressinpedestriancrowds:Experimentaldataandsimulations
Web Intelligence, 17(1), 85-99. http://dx.doi.org/10.3233/ Gorrini,WEB-190403A.,Messa, F., Ceccarelli, G. & Choubassi, R. (2021). Covid-19 pandemic and activity patterns in Milan. Wi-Fi sensors and locationbased data. TeMA-Journal of Land Use, Mobility and Environment, 14 (2), 211-226. https://doi.org/10.6093/1970-9870/7886
https://ssrn.com/abstract=3757431
dynamics.Helbing,andassembly/our-insights/micromobilitys-15000-mile-checkupcom/industries/automotive-D.andP.Molnar(1995).Socialforcemodelforpedestrian Phys. Rev. E, 51(5), 4282-4286. https://doi.org/10.1103/ Hutto,PhysRevE.51.4282C.andGilbert, E. (2014). Vader: A parsimonious rule-based model for sentiment analysis of social media text. In: Proceedings of the International AAAI Conference on Web and Social Media, 8 (1). Available at: https://ojs.aaai.org/index.php/ICWSM/article/ Istitutoview/14550Nazionale di Statistica and Istituto Superiore di Sanità (2020). Impatto dell’epidemia Covid-19 sulla mortalità totale della popolazione residente primo quadrimestre 2020. Istituto Superiore di Sanità. Available at: Iss_3Giugno.pdfhttps://www.epicentro.iss.it/coronavirus/pdf/Rapp_Istat_
Jurdak, R., Zhao, K., Liu, J., AbouJaoude, M., Cameron, M. and Newth, D. (2015). Understanding human mobility from Twitter. PloS one, 10 (7), e0131469. https://doi.org/10.1371/journal.pone.0131469
Review of the TfL WiFi pilot. Our findings. Mayor of London. Available online: http://content.tfl.gov.uk/review-tflwifi-pilot.pdf (accessed on 1 March 2021)
Physical Audience Measuring Technologies and Privacy Concerns. National Centre IOT and Privacy. Available at: Privacy-Concerns-ENG.pdfWhitepaper-Physical-Audience-Measuring-Technologies-and-https://iotprivacy.it/wp-content/uploads/2020/06/ Odat, E., Shamma, J.S. and Claudel, C. (2017). Vehicle classification and speed estimation using combined passive infrared/ultrasonic sensors. IEEE transactions on intelligent transportation systems, 19(5), 1593-1606. https://doi.org/10.1109/TITS.2017.2727224 Paris En Commun (2020). Ville du 1/4h. Le Programme d’Anne Hidalgo. Available at: https://annehidalgo2020.com/le-programme/ Plunz, R. A., Zhou, Y., Vintimilla, M. I. C., Mckeown, K., Yu, T., Uguccioni, L., and Sutto, M. P. (2019). Twitter sentiment in New York City parks as measure of well-being. Landscape and urban planning, 189, 235-246. https://doi.org/10.1016/j.landurbplan.2019.04.024 PTV Group (2021). PTV Visum ®. PTV Group. Regione Lombardia (2020). Ordinanza n. 604 del 10/09/2020. Ulteriori misure per la prevenzione e gestione dell’emergenza epidemiologica da Covid-19. Available at: wps/wcm/connect/209b7430-4bbf-4203-b9ab-89c97bb599a2/https://www.regione.lombardia.it/ ORDINANZA+604+del+10+settembre+2020.pdf?MOD=AJPERE S&CACHEID=ROOTWORKSPACE-209b7430-4bbf-4203-b9ab89c97bb599a2-nhUNJzC
1
Schläpfer, M., Dong, L., O’Keeffe, K. et al. (2021). The universal visitation law of human mobility. Nature, 593, 522–527. https://doi. org/10.1038/s41586-021-03480-9
Schwertman, N. C., Owens, M. A. and Adnan, R. (2004). A simple more general boxplot method for identifying outliers. Computational statistics & data analysis, 47(1), 165-174. https://doi.org/10.1016/j. csda.2003.10.012
Xhafa, F., Leu, F.Y. and Hung, L.L. (2017). Smart sensors networks: Communication technologies and intelligent applications
Speck, J. (2013). Walkable city: How downtown can save America, one step at a time. Macmillan. Transform Transport (2018). Re-imagining Corso Buenos Aires Systematica Srl. Available at: Transportresearch/re-imagining-corso-buenos-aires/https://research.systematica.net/forLondon(2019).
2
United Nations (2016). Transforming Our World: The 2030 Agenda for Sustainable Development. UN Publishing. Waterloo, S. F., Baumgartner, S. E., Peter, J. and Valkenburg, P. M. (2018). Norms of online expressions of emotion: Comparing Facebook, Twitter, Instagram, and WhatsApp. New media & society, 20 (5), 18131831. https://doi.org/10.1177/1461444817707349
Zhao, Z., Zheng, P., Xu, S. and Wu, X. (2019). Object Detection With Deep Learning: A Review. IEEE Transactions on Neural Networks and Learning Systems, 30, 3212-3232. https://doi.org/10.48550/ arXiv.1807.05511 Websites Measuring sidewalk widths for NYC using sidewalk outlines: https://github.com/meliharvey/sidewalkwidths-nyc Geoportal of the City of
World Health Organization (2020). Coronavirus disease (COVID-19) advice for the public. World Health Organization. Available at: www.who.int/emergencies/diseases/novel-coronavirus-2019/advice-https:// for-public (Accessed: 20 July 2020)
Sapiezynski, P., Stopczynski, A., Gatej, R. and Lehmann, S. (2015). Tracking human mobility using wifi signals. PloS one, 10(7), e0130824. https://doi.org/10.1371/journal.pone.0130824
Lin, T. et al. (2014). Microsoft COCO: Common Objects in Context. In: Procedings of the European Conference on Computer Vision, 740755. Lupi,https://doi.org/10.1007/978-3-319-10602-1_48G.(2017).Datahumanism:Therevolutionaryfuture of data visualization. PrintMag. Available at: inMilne,data-humanism-future-of-data-visualization.https://www.printmag.com/post/D.andWatling,D.(2019).Bigdataandunderstandingchangethecontextofplanningtransportsystems. Journal of Transport Geography, 76, 235-244 Moreno, C., Allam, Z., Chabaud, D., Gall, C. and Pratlong, F. (2021). Introducing the “15-Minute City”: Sustainability, Resilience and Place Identity in Future Post-Pandemic Cities. Smart Cities, 4(1), 93–111. Nationalhttps://doi.org/10.3390/smartcities40100066CentreIOTandPrivacy(2020).
https://www.istat.it/en/https://www.geoportale.regione.lombardia.it/http://www.governo.it/it/coronavirus-misure-del-governohttps://geoportale.comune.milano.it/sit/open-data/Milan:3MilanSidewalksMap:www.milansidewalks.com4MilanoCam:https://www.milanocam.it/5FreeLuna:https://www.futur3.it/en/6NationalregulationenforcedbytheItalianGovernment:7Blimp:https://blimp.ai/8GeoportalofLombardyRegion:9ItalianNationalInstituteofStatistics:10OpenStreetMap:https://www.geofabrik.de/11ResultsofSuitabilityAnalysisIndex(interactivemap):https://app.transformtransport.org/suitabilityindex/milan.html
Seyfried, A., B. Steffen, W. Klingsch, and M. Boltes (2005). The fundamental diagram of pedestrian movement revisited. Journal of Statistical Mechanics: Theory and Experiment, 10, P10002. https:// Song,doi.org/10.1088/1742-5468/2005/10/p10002Y.,Merlin,L.andRodriguez,D.(2013).Comparing measures of urban land use mix. Computers, Environment and Urban Systems, 42, 1-13. https://doi.org/10.1016/j.compenvurbsys.2013.08.001
Academic Press. Zagorskas, J. and Burinskienė, M. (2020). Challenges caused by increased use of e-powered personal mobility vehicles in European cities. Sustainability, 12(1), 273. https://doi.org/10.3390/su12010273
93 Kapadia, S. (2019). Topic Modeling in Python: Latent Dirichlet Allocation (LDA). Medium. Available at: Kaplan,lda-35ce4ed6b3e0com/end-to-end-topic-modeling-in-pythonlatent-dirichlet-alhttps://towardsdatascience.location-R.andKaplan,S.(1989).
The experience of nature: A psychological perspective. Cambridge University Press. Kretz, T. (2015). On oscillations in the social force model. Physica A: Statistical Mechanics and its Applications, 438, 272-285. https://doi. Li,org/10.1016/j.physa.2015.07.002W.,Batty,M.andGoodchild,M.F. (2020). Real-time gis for smart cities. International Journal of Geographical Information Science, 34 (2), 311-324. https://doi.org/10.1080/13658816.2019.1673397
Lin, M. and Hsu, W.J. (2014). Mining GPS data for mobility patterns: A survey. Pervasive and mobile computing, 12, 1-16. http://dx.doi. org/10.1016/j.pmcj.2013.06.005
It grounds on 30 years of Systematica’s work and explores how disruptive technologies, increasingly and rapidly influencing urban mobility, can have a positive impact on cities, neighborhoods, and buildings, collaborating closely with municipalities and companies, and using Big Data for greater insights.
Transform Transport is a non-profit research foundation based in Milan (Italy) and focused on innovation in mobility and transport planning. It provides innovative, inclusive, and sustainable mobility solutions for shaping the future of society and cities worldwide in line with the UN’s SDG 11 (Sustainable cities and communities).
Transform Transport
Transform Transport actively participates in lectures, talks, and hackathons in partnership with Universities, mentoring and sharing methodologies with students. It also promotes and develops research studies, disseminating them through books, publications, conferences, public talks, and events.
www.transformtransport.org
Established in 1989, Systematica is a Milanbased transport planning and mobility engineering consultancy with subsidiary offices in Mumbai and New SystematicaYork. operates at multiple scales - national, urban/metropolitan, and development-scale - and provides a wide array of integrated consultancy services in the transport and urban planning sectors, such as strategic advisory and due diligence for infrastructure investments; traffic analysis and management; mobility engineering in complex buildings and events venues with a focus on pedestrian flows; parking design; vertical transportation, and application of advanced mobility systems and technologies.
Systematica
Committed to its mission to provide innovative, inclusive, and sustainable solutions, Systematica also seeks new approaches to overcome the ever-changing challenges of mobility and transport planning and support sustainable growth through scientific research.
www.systematica.net
The analyzed data were treated according to the GDPRGeneral Data Protection Regulation (EU, 2016/679). This research received no specific grant from any funding agency in the public, commercial, or not-forprofit sectors.
Credits Transform Transport (2022). Shifting Paradigm: the impact of Covid-19 on transport planning (second edition). Fondazione Transform Transport ETS. ISBN: 9788894713404 Team: Lamia Abdelfattah, Filippo Bazzoni, Giulia Boni, Oxana Borovkova, Simone Castelnuovo, Giulia Ceccarelli, Ana Gaby Chavez, Rawad Choubassi, Diego Deponte, Eduardo Espitia, Andrea Gorrini, Jonelle Hanson, Matteo Marconi, Federico Messa, AntoineMarie Portalier, Dante Presicce, Caroline Purps, Nicola Ratti, Davide Tamiozzo, Alessandro Vacca, Marianna AZuretti.special thanks to all collaborators of Transform Transport who contributed to this book. Acknowledgement
anspor t t
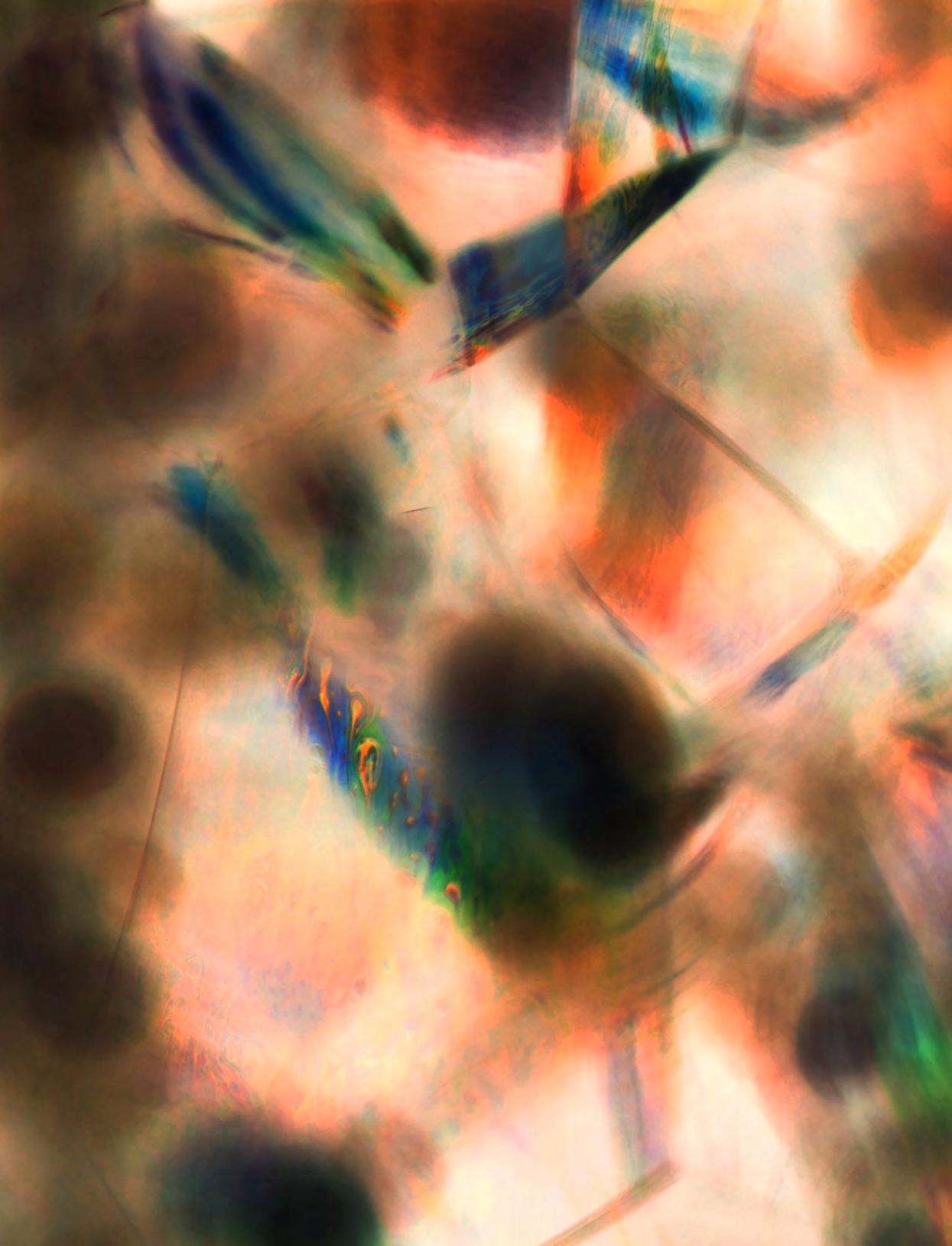